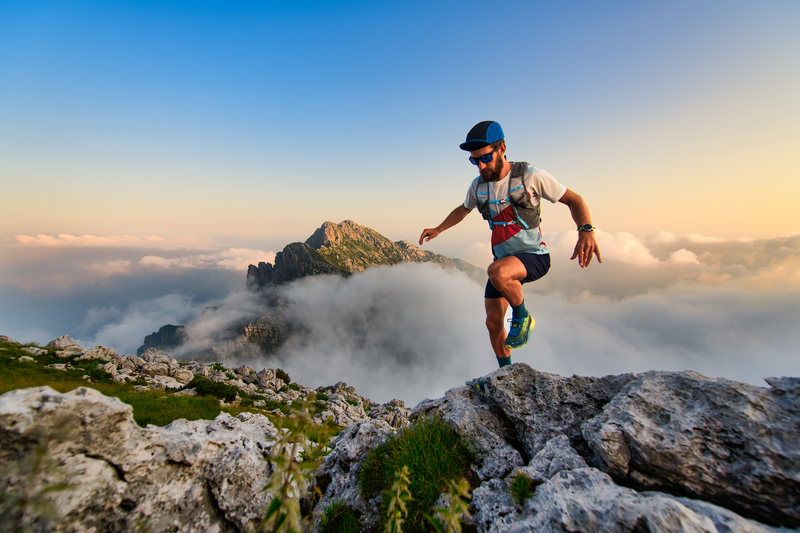
95% of researchers rate our articles as excellent or good
Learn more about the work of our research integrity team to safeguard the quality of each article we publish.
Find out more
ORIGINAL RESEARCH article
Front. Earth Sci. , 26 November 2024
Sec. Solid Earth Geophysics
Volume 12 - 2024 | https://doi.org/10.3389/feart.2024.1472303
To obtain more accurate full waveform inversion results, we present a forward modeling method with minimal phase error, low numerical dispersion, and high computational efficiency. To solve the 2D acoustic wave equation, we utilize a finite-difference (FD) scheme with optimized coefficients for spatial discretization, combined with a phase-preserving symplectic partitioned Runge-Kutta method for temporal discretization. This results in the development of the optimized symplectic partitioned Runge-Kutta (OSPRK) forward modeling method. We further apply the OSPRK method in conjunction with a recurrent neural network (RNN) for full waveform inversion (FWI). Our study explores the impact of various loss functions, Nadam optimizer parameters, and the incorporation of physical information operators on inversion performance. Numerical experiments demonstrate that the OSPRK method significantly reduces numerical dispersion compared to traditional FD methods. The Log-Cosh loss function offers superior stability across different learning rates, while the Nesterov-accelerated Adaptive Moment Estimation (Nadam) optimizer with optimized parameters greatly enhances convergence speed and inversion accuracy. Furthermore, the inclusion of physical information operators markedly improves inversion outcomes.
Full waveform inversion (FWI) is widely used in oil and gas exploration, geotectonic research, and infrastructure development. This technique relies on the analysis of seismic waveforms, which propagate through subsurface media and are recorded by receivers, to infer the material properties of the Earth’s interior (Tarantola, 1984; Virieux and Operto, 2009; Tong et al., 2014). In this process, the wave equation is typically employed to simulate the propagation of seismic waves through the medium, producing synthetic waveform data. An optimization algorithm is then applied to iteratively refine the subsurface parameters, improving the accuracy of the inversion with each iteration.
In FWI, solving the wave equation represents the primary computational burden. As a result, the development of numerical methods that can solve wave equations with both high accuracy and efficiency has been a key area of research. Numerical methods for wave equation solutions involve spatial discretization, time discretization, and boundary condition handling. Spatial discretization methods include the traditional finite difference (FD) method (Virieux, 1986; Moczo et al., 2002; Saenger et al., 2004), the finite element method (Eriksson and Johnson, 1991; Yang et al., 2008), the nearly analytic discrete (NAD) method (Yang et al., 2003), the optimized finite difference format (Zhang and Yao, 2013), the optimized expansion method (Wu and Alkhalifah, 2014), among others. The FD method tends to perform well when using a fine mesh but suffers from significant numerical dispersion when applied with a coarse mesh (Yang et al., 2006; Ma et al., 2020; Wu and Alkhalifah, 2018). The NAD method improves on this by utilizing both displacement and gradient information from grid points and their neighbors to approximate higher-order spatial derivatives, thereby reducing numerical dispersion. However, this method requires substantial computational resources, which limits its efficiency. Zhang and Yao (2013) introduced an optimized FD method that minimizes wave number errors using the maximum norm. The optimized coefficients for this method are obtained via the simulated annealing technique (Kirkpatrick et al., 1983), resulting in lower numerical dispersion and greater computational efficiency. For temporal discretization, methods include the symplectic partitioned Runge-Kutta (SPRK) method (Qin and Zhang, 1990), the phase-preserving SPRK (Ma and Yang, 2017), and the modified SPRK format (Liu et al., 2017). Each method has distinct advantages depending on the application. For instance, Liu et al. (2017) introduced a modified SPRK method that achieves higher-order accuracy with reduced computational cost. Ma and Yang, (2017) developed optimized coefficients for the phase-preserving SPRK format, which significantly reduces phase errors and improves overall accuracy.
In recent years, with the rapid advancement of deep learning technologies, researchers have begun integrating deep learning with forward modeling and inversion techniques in geophysics. Richardson (2018) was the first to combine forward modeling with the recurrent neural network (RNN) framework in deep learning. His numerical experiments showed that the Adam optimizer in deep learning outperformed the traditional L-BFGS-B optimizer for inversion tasks. Wu and McMechan (2019) applied convolutional neural networks (CNNs) to generate initial velocity models by utilizing a weighting parameter, resulting in inverted velocity models that were more accurate than those produced by conventional FWI methods. Raissi et al. (2019) introduced Physics-Informed Neural Networks (PINN) to tackle both forward and inverse problems involving nonlinear partial differential equations, a method later extended by Song and Alkhalifah (Song and Alkhalifah, 2021). Lu et al. (2021) further refined this approach by integrating high-precision operators into the RNN framework and enhancing the loss function of FWI through the incorporation of physical information operators, leading to improved inversion accuracy. Li et al. (2021) improved the resolution of the elastic full waveform inversion (EFWI) by using a deep neural network to learn the statistical relationship between selected features in the inversion model and the lithology interpreted from downhole logs. Li et al. (2023) designed a deep learning (DL) framework that combines full waveform inversion (FWI) on seismic data and downhole logging information to construct a subsurface model, which ultimately improves the resolution and accuracy of the velocity model.
The innovation of this study lies in the use of the OSPRK method as the forward modeling approach for FWI, along with the Log_Cosh function enhanced by physically informed operators as the loss function. In cases involving coarse grids, traditional FD methods tend to introduce significant numerical dispersion, resulting in greater numerical errors with each iteration of the inversion process. In contrast, the OSPRK method is characterized by its low numerical dispersion and minimal phase error, making it superior to the FD method. The Log_Cosh loss function combines the advantages of both the Mean Squared Error (MSE) and Mean Absolute Error (MAE) loss functions. By incorporating a physical information operator that adheres to the wave equation into this loss function, we impose a constraint based on physical principles, thereby improving the accuracy of the inversion results.
A 2n-dimensional linear separable Hamiltonian system can be written in the following form (Feng and Qin, 2010):
where
Solving Equation 1 in kth order SPRK format (Ma and Yang, 2017) can be expressed as:
where
In a two-dimensional isotropic medium with constant density, the acoustic wave equation can be expressed as:
where
where
We employ the fourth-order SPRK format for temporal discretization. Equation 4 is then expressed as:
For the temporal discretization, Ma and Yang, (2017) derived a vector of coefficients corresponding to the fourth-order phase-preserving SPRK for the individual coefficients of
For second-order derivatives in
where the coefficients of the sixth-order optimized finite difference operator (Zhang and Yao, 2013) are as follows:
To prevent unwanted reflections at the edges of the simulation domain, we incorporate the perfectly matched layer (PML) (Wang, 2003) into Equation 5. Consequently, the complete process for solving the wave equation involves temporal discretization, spatial discretization, and the implementation of the PML to absorb outgoing waves effectively.
FWI is essentially an optimization problem that aims to minimize the discrepancy between the seismic waveform data recorded by the receiver and the synthetic waveform data generated by forward modeling. Typically, FWI consists of three key components: the forward modeling method, the choice of the loss function, and the selection of the optimizer. In this study, we combine the OSPRK method with an RNN for forward modeling, creating the OSPRK-RNN method for FWI. This approach leverages the similarity between traditional forward modeling algorithms and the computational process of RNNs (Richardson, 2018). Figure 1 illustrates the flowchart for the OSPRK-RNN method for full waveform forward and inverse modeling, where
In this paper, we introduce the Log_Cosh loss function and compare its performance in inversion results with that of the MAE and MSE loss functions through numerical experiments (Peng et al., 2022). The Log_Cosh loss function is defined as follows:
where
where
By identifying these three components, we establish a foundational framework for FWI based on deep learning. To further enhance experimental results, we also incorporate additional deep learning techniques, including the use of a small batch strategy, regularization, and grid search for hyperparameter optimization. These techniques help to improve model performance, reduce overfitting, and fine-tune parameters, ultimately leading to more accurate and reliable numerical outcomes.
In a previous study, Rassi et al. (2019) introduced physical information operators into the loss function to guide the training process, yielding promising results. In this study, we also incorporate physical differential operators into the loss function. Specifically, we define the physical differential operator for the two-dimensional acoustic equation as:
where
In this section, we present five numerical experiments to evaluate the effectiveness of combining the OSPRK method with RNN for full waveform forward and inverse modeling. The first experiment demonstrates the accuracy and efficiency of the OSPRK method in forward modeling. The second and third experiments investigate the impact of different loss functions and various Nadam optimizer parameters on FWI performance. In the fourth experiment, we compare the inversion results obtained using the traditional FD method with those from the OSPRK method. Finally, in the fifth experiment, we illustrate the capability of the OSPRK-RNN method in inverting complex velocity models, and we show how the inclusion of physically informed operators significantly improves inversion accuracy.
In this numerical experiment, we evaluate the effectiveness of combining the OSPRK method with RNN and compare it to the conventional sixth-order FD method combined with RNN for forward modeling. The computational domain for the homogeneous velocity model is set to 4.8 km × 4.8 km, with a spatial step of
We then compare the forward simulation results of the OSPRK method with those of the traditional FD method combined with RNN. As shown in Figure 3, the wavefield snapshot in Figure 3B, obtained using the FD method, displays significant numerical dispersion, whereas the snapshot in Figure 3A, produced by the OSPRK method, exhibits minimal numerical dispersion. Furthermore, the wavefield snapshots in Figures 3C, D confirm that the added PML effectively absorbs boundary-reflected waves as the wave propagates out of the region. In terms of computational efficiency, the time required to compute the entire wavefield is 2.57 s for the OSPRK method and 2.61 s for the conventional FD method. These results demonstrate that the OSPRK method significantly reduces numerical dispersion, while the PML effectively mitigates boundary reflections.
Figure 3. (A, C) and (B, D) Snapshots of the wavefields at t = 0.319 s and t = 0.469 s by OSPRK and FD, respectively.
In this experiment, we apply FWI using the OSPRK method combined with RNN, focusing on the effects of three different loss functions and varying learning rates on the inversion results. The two-layer velocity model has a computational domain of 0.25 km × 0.25 km, with a spatial step of
Figure 4. Layered velocity model with [0 km, 0.125 km] × [0 km, 0.25 km] for region I and [0.125 km, 0.25 km] × [0 km, 0.25 km] for region II.
For the initial velocity model used in the inversion, we reduce the velocity by a factor of 0.9. The real velocity model and the initial velocity model are shown in Figure 5. We then perform FWI using three commonly used loss functions—MAE, MSE, and Log_Cosh—across different learning rates. Figure 6 presents the inversion results obtained using these loss functions at learning rates of 10, 20, 30, 50, and 70. Since the magnitudes of the three loss functions differ, we define the relative error
where N and M are the numbers of rows and columns of the mesh, respectively.
Figure 6. Inversion results obtained by Log_Cosh, MAE, and MSE loss functions at different learning rates.
Table 1 presents the relative errors for the three loss functions at the specified learning rates of 10, 20, 30, 50, and 70. Figure 7 illustrates the line graphs of the relative errors for each loss function across the different learning rates, highlighting the characteristics of each loss function and the influence of learning rates on the inversion results. When
Figure 7. The relative error of the Log_Cosh, MAE, and MSE loss functions at different learning rates in the coordinate system.
In the following, we will consider the placement of receivers and seismic sources under real full-waveform inversion conditions. We place the receivers vertically every 5 m on the leftmost side of the model area and the sources horizontally every 5 m on the surface, for a total of 51 receivers and 51 sources. Figure 8 shows a schematic diagram of the placement of the seismic sources and receivers.
In the training process, we choose 40 sources as the training set and the remaining 11 sources are used as the test set. We set the batch size to 3, the learning rate to 20, and the number of traversals to 5, for a total of 65 iterations. As can be seen in Figure 9, the OSPRK method also gives good inversion results in real receiver and seismic source placement scenarios.
In this experiment, we conduct FWI using the OSPRK method combined with RNN, focusing on the effects of the parameters
First, the values of the parameters are detailed in Table 2, with β1 ranging from 0.9 to 0.1 in steps of 0.2, and β2 ranging from 0.999 to 0.1 in steps of 0.3. This results in a total of 20 sets of inverse numerical simulations.
The Ricker wavelet sources, with a frequency of 10 Hz, are positioned at the bottom of the model and distributed horizontally across 51 grid points. Receivers are placed on the surface of the model at 6-meter intervals. Out of the 51 sources, 39 are selected for training, while the remaining 12 are used for testing. The mini-batch size is set to 3, with an epoch count of 4. This configuration results in a total of 52 batch iteration steps to complete the inversion process. The relative errors for these 20 sets of inverse numerical simulations are displayed in Figure 9.
From Figure 11, it is clear that the default Nadam parameters are not optimal. Among the various parameter configurations, the lowest relative error is achieved with
As shown in Figure 12, the loss function curves with the optimized parameters are relatively smooth throughout the descent process and converge after 20 iterations. In contrast, the loss function curves with the default parameters exhibit significant oscillations during the descent process and only converge after 30 iterations.
In this experiment, we will use OSPRK-RNN and FD-RNN for full waveform inversion and then compare the inversion results of the two methods. For the three-layer velocity model, the computational domain is 0.25 km × 0.25 km, the spatial step is
Figure 13. The location of region I is [0 km, 0.075 km] × [0 km, 0.25 km] and the location of region II is [0.14 km, 0.19 km] × [0.1 km, 0.15 km].
For the initial velocity model of the inversion, we choose a Gaussian perturbation of 10% of the true velocity model to generate the initial velocity model. In Figure 14, we give the true velocity model and the initial velocity model. Figure 15 gives a comparison of the inversion between the OSPRK method and the FD method after 65 iterations. From the inversion results in Figure 15, both methods are able to roughly invert the basic shape of the real velocity model. However, the inversion result of the OSPRK method is closer to the real velocity model in terms of the dividing line between regions I and III and the shape of region II. For the relative errors of the velocity model after inversion, the relative errors of the OSPRK method and the FD method are 1.275% and 2.137%, respectively. As a result, our proposed OSPRK will be more accurate than the FD method in the inversion results.
In the final numerical experiment, we perform an inversion on a complex Sigsbee velocity model. The computational domain is 0.488 km × 0.808 km, with velocities ranging from 1,400 m/s to 4,500 m/s. The model features a high-velocity anomaly of 4,500 m/s in the central region. The spatial step is
For the first 80 iterations, we use only the data information in the loss function. In the last 80 iterations, we incorporate physical information into the loss function. As shown in Figure 16, the value of the loss function with physical information added during the final 80 iterations is significantly lower than that of the loss function without physical information. This demonstrates that incorporating physical information into the loss function enhances the inversion accuracy. Figure 17 shows that the OSPRK method, when augmented with physical information, successfully inverts the high-velocity anomalies, indicating that this approach is highly effective for inverting complex velocity models.
In this study, we combined the OSPRK forward modeling method with an RNN for full waveform forward and inverse modeling. Additionally, we introduced the Log_Cosh loss function and the Nadam optimizer from deep learning, conducting one forward modeling and four inverse numerical simulations to demonstrate the effectiveness of our approach. The first experiment, which involved uniform medium forward modeling, showed that the OSPRK method effectively suppresses numerical dispersion compared to the conventional FD method. In the second experiment, using a two-layer velocity model for inversion, we confirmed that the Log_Cosh loss function offers greater stability across various learning rates compared to MSE and MAE. The third experiment, which focused on a central velocity model, demonstrated that optimized Nadam parameters result in faster convergence and higher inversion accuracy compared to default settings. The fourth experiment revealed that the OSPRK method yields more accurate inversion results than the FD method. Finally, in the inversion of a complex Sigsbee velocity model, we utilized an optimized Nadam optimizer and a loss function incorporating physical operators, highlighting the high efficacy of combining deep learning techniques with the OSPRK method. All these numerical simulations are based on the acoustic wave equation, a simplified mathematical-physical model of seismic wave propagation. In future research, we plan to integrate deep learning techniques with more complex elastic wave equations. Additionally, since the RNN framework may face challenges such as gradient vanishing and explosion, another key focus of our future work will be exploring neural network frameworks with improved performance.
The original contributions presented in the study are included in the article/supplementary material, further inquiries can be directed to the corresponding author.
YZ: Writing–review and editing. XL: Methodology, Validation, Writing–original draft, Writing–review and editing. XuH: Writing–review and editing. XiH: Writing–review and editing. TC: Writing–review and editing.
The author(s) declare that financial support was received for the research, authorship, and/or publication of this article. This work was supported by the National Natural Science Foundation of China under Grant 42330801, and Grant 42374143.
We thank Alan Richardson for providing his open-source code at https://arxiv.org/abs/1801.07232. His code provides an important basis for the progress of this study.
The authors declare that the research was conducted in the absence of any commercial or financial relationships that could be construed as a potential conflict of interest.
All claims expressed in this article are solely those of the authors and do not necessarily represent those of their affiliated organizations, or those of the publisher, the editors and the reviewers. Any product that may be evaluated in this article, or claim that may be made by its manufacturer, is not guaranteed or endorsed by the publisher.
Bubeck, S., Lee, Y. T., and Singh, M. (2015). A geometric alternative to nesterov's accelerated gradient descent. Mathematics. doi:10.48550/arXiv.1506.08187
Eriksson, K., and Johnson, C. (1991). Adaptive finite element methods for parabolic problems. I.: a linear model problem. Soc. Industrial Appl. Math. 28, 43–77. doi:10.1137/0728003
Feng, K., and Qin, M. (2010). Symplectic geometric algorithms for Hamiltonian systems. Germany: Springer. doi:10.1007/978-3-642-01777-3
Kingma, D., and Ba, J. (2014). Adam: a method for stochastic optimization. Comput. Sci. doi:10.48550/arXiv.1412.6980
Kirkpatrick, S., Gelatt, C. D., and Vecchi, M. P. (1983). Optimization by simulated annealing. Science 220, 671–680. doi:10.1126/science.220.4598.671
Li, Y. Y., Alkhalifah, T., Huang, J., and Li, Z. (2023). Self-supervised pretraining vision transformer with masked autoencoders for building subsurface model. Trans. Geoscience Remote Sens. 6, 11–10. doi:10.1109/TGRS.2023.3308999
Li, Y. Y., Alkhalifah, T., and Zhang, Z. (2021). Deep-learning assisted regularized elastic full waveform inversion using the velocity distribution information from wells. Geophys. J. Int. 226, 1322–1335. doi:10.1093/gji/ggab162
Liu, S. L., Yang, D. H., and Ma, J. (2017). A modified symplectic PRK scheme for seismic wave modeling. Comput. and Geosciences 99, 28–36. doi:10.1016/j.cageo.2016.11.001
Lu, F., Zhou, Y. J., He, X. J., Ma, X., and Huang, X. Y. (2021). Full waveform inversion based on deep learning and optimal nearly analytic discrete method. Appl. Geophys. 18 (4), 483–498. doi:10.1007/s11770-021-0912-4
Ma, S., Li, D., Hu, T., Xing, Y., and Nai, W. (2020). Huber loss function based on variable step beetle antennae search algorithm with Gaussian direction. Int. Conf. Intelligent Human-Machine Syst. Cybern. (IHMSC) 1, 248–251. doi:10.1109/IHMSC49165.2020.00062
Ma, X., and Yang, D. H. (2017). A phase-preserving and low-dispersive symplectic partitioned Runge-Kutta method for solving seismic wave equations. Geophys. J. Int. 209, 1534–1557. doi:10.1093/gji/ggx097
Moczo, P., Kristek, J., Vavrycuk, V., Archuleta, R. J., and Halada, L. (2002). 3d heterogeneous staggered-grid finite-difference modeling of seismic motion with volume harmonic and arithmetic averaging of elastic moduli and densities. Bull. Seismol. Soc. Am. 92 (8), 3042–3066. doi:10.1785/0120010167
Peng, K., Guo, H. Y., and Shang, X. Y. (2022). Microseismic source location using the Log-Cosh function and distant sensor-removed P-wave arrival data. J. Central South Univ. 29 (2), 712–725. doi:10.1007/s11771-022-4943-7
Qin, M. Z., and Zhang, M. Q. (1990). Multi-stage symplectic schemes of two kinds of Hamiltonian systems for wave equations. Comput. Math. Appl. 19 (10), 51–62. doi:10.1016/0898-1221(90)90357-P
Raissi, M., Perdikaris, P., and Karniadakis, G. E. (2019). Physics-informed neural networks: a deep learning framework for solving forward and inverse problems involving nonlinear partial differential equations. J. Comput. Phys. 378, 686–707. doi:10.1016/j.jcp.2018.10.045
Richardson, A. (2018). Seismic full-waveform inversion using deep learning tools and techniques. doi:10.48550/arXiv.1801.07232
Saenger, E. H., Krüger, O. S., and Shapiro, S. A. (2004). Effective elastic properties of randomly fractured soils: 3d numerical experiments. Geophys. Prospect. 52 (3), 183–195. doi:10.1111/j.1365-2478.2004.00407.x
Saleh, R. A., and Saleh, A. K. M. E. (2022). Statistical properties of the Log_Cosh loss function used in machine learning. Arxiv. doi:10.48550/arXiv.2208.04564
Song, C., and Alkhalifah, T. (2021). Wavefield reconstruction inversion via physics-informed neural networks. IEEE Trans. Geoscience Remote Sens. 60, 1–12. doi:10.1109/TGRS.2021.3123122
Tarantola, A. (1984). Inversion of seismic reflection data in the acoustic approximation. Geophysics 49 (8), 1259–1266. doi:10.1190/1.1441754
Tong, P., Zhao, D., Yang, D. H., Liu, Q., and Chen, J. (2014). Wave-equation based traveltime seismic tomography – part 1: method. Solid Earth Discuss. 6 (2), 2523–2566. doi:10.5194/sed-6-2523-2014
Virieux, J. (1986). P-sv wave propagation in heterogeneous media velocity-stress finite-difference method. Geophysics 51, 889–901. doi:10.1190/1.1442147
Virieux, J., and Operto, S. (2009). An overview of full-waveform inversion in exploration geophysics. Geophysics 74 (6), WCC1–WCC26. doi:10.1190/1.3238367
Wang, S. D. (2003). Absorbing boundary condition for acoustic wave equation by perfectly matched layer. Oil Geophys. Prospect. 38 (1), 31–34. doi:10.1007/BF02873153
Wu, Y. L., and McMechan, G. A. (2019). Parametric convolutional neural network-domain full-waveform inversion. Geophysics 84 (6), R881–R896. doi:10.1190/geo2018-0224.1
Wu, Z. D., and Alkhalifah, T. (2014). The optimized expansion based low-rank method for wavefield extrapolation. Geophysics 79 (2), T51–T60. doi:10.1190/GEO2013-0174.1
Wu, Z. D., and Alkhalifah, T. (2018). A highly accurate finite-difference method with minimum dispersion error for solving the Helmholtz equation. J. Comput. Phys. 365, 350–361. doi:10.1016/j.jcp.2018.03.046
Yang, D. H., Liu, E., and Zhang, Z. (2008). Evaluation of the u-W finite method in anisotropic porous media. J. Seismic Explor. 17 (2-3), 273–299. doi:10.1093/petrology/egn007
Yang, D. H., Peng, J. M., Lu, M., and Terlaky, T. (2006). Optimal nearly analytic discrete approximation to the scalar wave equation. Bull. Seismol. Soc. Am. 96, 1114–1130. doi:10.1785/0120050080
Yang, D. H., Teng, J., Zhang, Z., and Liu, E. (2003). A nearly analytic discrete method for acoustic and elastic wave equations in anisotropic media. Bull. Seismol. Soc. Am. 93 (2), 882–890. doi:10.1785/0120020125
Keywords: OSPRK method, FWI, RNN, nadam optimizer, Log_Cosh loss, the physical information operator
Citation: Zhou Y, Leng X, Huang X, He X and Cao T (2024) Full waveform inversion based on deep learning and the phase-preserving symplectic partitioned Runge-Kutta method. Front. Earth Sci. 12:1472303. doi: 10.3389/feart.2024.1472303
Received: 29 July 2024; Accepted: 04 November 2024;
Published: 26 November 2024.
Edited by:
Tariq Alkhalifah, King Abdullah University of Science and Technology, Saudi ArabiaReviewed by:
Gang Yao, China University of Petroleum, Beijing, ChinaCopyright © 2024 Zhou, Leng, Huang, He and Cao. This is an open-access article distributed under the terms of the Creative Commons Attribution License (CC BY). The use, distribution or reproduction in other forums is permitted, provided the original author(s) and the copyright owner(s) are credited and that the original publication in this journal is cited, in accordance with accepted academic practice. No use, distribution or reproduction is permitted which does not comply with these terms.
*Correspondence: Yanjie Zhou, emhvdXlhbmppZTlAMTYzLmNvbQ==
Disclaimer: All claims expressed in this article are solely those of the authors and do not necessarily represent those of their affiliated organizations, or those of the publisher, the editors and the reviewers. Any product that may be evaluated in this article or claim that may be made by its manufacturer is not guaranteed or endorsed by the publisher.
Research integrity at Frontiers
Learn more about the work of our research integrity team to safeguard the quality of each article we publish.