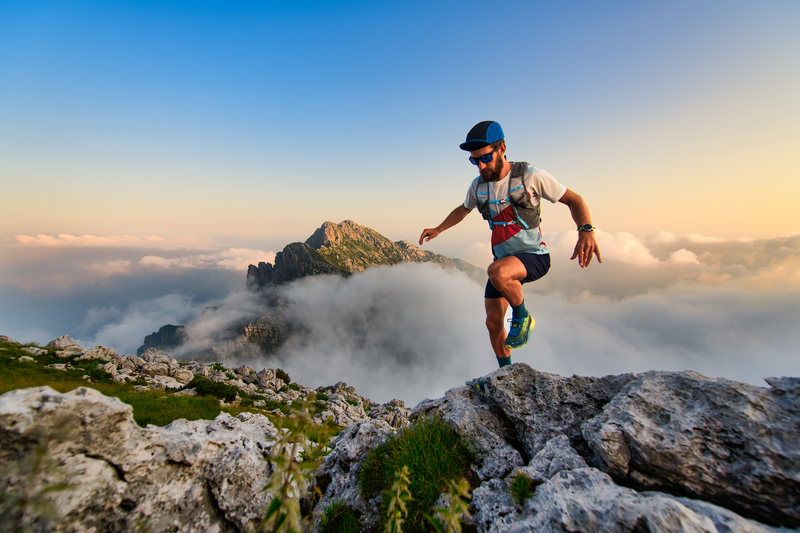
95% of researchers rate our articles as excellent or good
Learn more about the work of our research integrity team to safeguard the quality of each article we publish.
Find out more
ORIGINAL RESEARCH article
Front. Earth Sci. , 08 August 2024
Sec. Geohazards and Georisks
Volume 12 - 2024 | https://doi.org/10.3389/feart.2024.1420425
This article is part of the Research Topic Risk Assessment and Resilience of Extreme Weather-Induced Disasters View all 9 articles
On 8 June 2017, a heavy storm struck the parallel ridge-valley area of western Chongqing, resulting in serious urban waterlogging and landslides, which led to severe impacts on infrastructure and damage to private property. Based on high-resolution optical satellite images, this paper comprehensively identified the landslides triggered by this rainfall event, and established a corresponding landslide database. The database takes the landslide area density and landslide number density as the main indicators, and combines the lithology characteristics to analyze the spatial distribution of landslides. The results show that this event triggered 487 landslides in an area of 583 km2, involving an area of about 485,587 m2, accounting for about 0.083% of the study area. The average landslide number density is 0.84 num/km2, the highest value of landslide number density can reach 55.6 num/km2, and the maximum landslide area density is about 6.4%. These landslides are mainly distributed in the southern foothills of the Huaying Mountain, especially in the weak interlayer lithology area. The database provides scientific reference and data support for exploring the mechanism of landslides in western Chongqing and reducing the risk of landslide disasters under the background of rapid development of local society.
Landslide disasters occur frequently, drawing extensive research from numerous scholars (Huang et al., 2022; Huang et al., 2023), with the aim of better addressing this challenge. Particularly in the current scenario of severe global climate change, the impact of climate change on the frequency of landslides has become a matter of great concern (Patton et al., 2019; Kirschbaum et al., 2020). The frequent incidence of extreme events such as heavy rainfall and droughts, caused by climate change, aggravates the erosion and loosening of the earth surface and increases the possibility of landslides occurrence. As a natural disaster, landslides are extremely destructive, posing a serious threat to both human society and the environment. Between 1 January 2004, and 31 December 2010, there were 2,620 fatal landslide events globally (excluding co-seismic landslides), resulting in a total of 32,322 deaths, with the number of recorded landslides showing an increasing trend each year (Petley, 2012). Landslide disasters can cause direct or indirect damage to local communities (e.g., destruction of infrastructure, power outages, and water supply interruptions), significantly impacting the local economy and social stability. The post-disaster reconstruction costs are enormous, encompassing clearance and reconstruction of facilities, imposing a heavy burden on local governments and social resources (Vranken et al., 2013). Moreover, landslide disasters can change the local landscape. Large-magnitude landslides may result in drastic changes in topography, including collapses, river diversion, etc., bringing profound impacts on surrounding environments and ecosystems (Thapa et al., 2024). Compared to post-disaster remedies, preventive measures beforehand are particularly crucial (World Bank and United Nations, 2010). By upgrading monitoring and warning systems, adjusting land use plans, enhancing infrastructure resilience, etc., thereby the frequency of landslides and the resulting losses and impacts can be effectively reduced.
In recent years, the prominent geographic advantage of the northwest part of Chongqing has garnered attention due to the development of the Chengdu-Chongqing economic circle. Positioned at the intersection of the Belt and Road and the Yangtze River Economic Belt, this region holds a unique geographical advantage in connecting the southwest and northwest of China domestically, and bridging East Asia, Southeast Asia, and South Asia internationally. Not only does this area boast abundant ecological resources and energy, including mineral deposits, but it also features densely populated urban areas and picturesque landscapes. It stands as one of the most densely populated regions in western China, with robust industrial foundations, strong innovative capabilities, broad market potential, and high levels of openness, playing a crucial strategic role in the country’s overall development. However, the rapid development of the Chengdu-Chongqing region also brings a series of challenges and risks, including frequent landslide disasters. For instance, an extreme rainfall event could trigger widespread landslides, severely impacting the local socioeconomic and ecological environment. Previous research in the region mainly focused on slope stability assessment (Wang L. et al., 2019; Wu et al., 2023), landslide susceptibility (Sun et al., 2020; Wei et al., 2021) and landslide movement processes (Zhang et al., 2014; Guo et al., 2020), while there is little research on the database of landslide triggered by a single rainfall event.
Compiling a landslide inventory serves as a crucial data foundation for further landslide studies, driven by multiple factors. Firstly, it marks the spatial and temporal occurrences of landslides (Shao et al., 2023a; Chen et al., 2023), providing fundamental data for subsequent research. Through landslide inventory, the mechanism, morphology, and mode controlled by the lithology or geology can be deeply understood (Zhang, 2020; Li et al., 2021). Conducting susceptibility (Ciurleo et al., 2021; Razavi-Termeh et al., 2021) and hazard assessments (Thiery et al., 2020; Lin et al., 2021) are of significant importance for geological disaster management. Developing landslide early warning systems (Lagomarsino et al., 2013; Calvello and Piciullo, 2016; Magrì et al., 2024) and taking proactive measures aids in reducing economic losses and casualties caused by landslides.
The advancement of science leads to technological innovations, offering various methods for landslide detection and inventory compilation. For instance, SAR data, characterized by all-weather capability and low cost, is utilized for landslide identification and displacement monitoring (Handwerger et al., 2022; Zhang et al., 2023). However, rapid deformation rates can lead to decorrelation, rendering it unsuitable for detecting landslides triggered by extreme rainfall events. Field surveys are commonly employed for individual landslides and on-site verification of landslide databases, but with high time and economic costs. Text mining based on big data extracts landslide event locations and times from social media (Franceschini et al., 2022), yielding abundant but potentially redundant and incomplete landslide-related data, posing huge challenges to researchers. Rapid development in optical satellite technology, with high precision, wide coverage, low cost, and multi-temporal, has received extensive attention. Using high-resolution optical satellite images, Sun et al. (2024a) identified 10,968 landslide traces in the Yinshan area; He et al. (2021) found 167 landslides triggered by the Qiaojia Mw5.1 earthquake on 18 May 2020 in Yunnan, China; Huang et al. (2021) established a database of earthquake-triggered landslides in Milin, Tibet, including 3,130 co-seismic landslides. Xie et al. (2023) took an extreme rainfall event in Jiexi County, Guangdong Province in August 2018 as the research subject, and established a database containing 1,844 landslides. Compared with the great progress in earthquake-triggered landslide database construction, the establishment of a rainfall-triggered landslide database using optical satellite images is relatively slow. As of 2022, there are only 16 public databases (Ma et al., 2022) of heavy rainfall-triggered landslides worldwide. Primarily because optical satellites are often hindered by cloud cover during adverse weather conditions, making it challenging to extract rainfall-triggered landslides occurring on cloudy days.
The parallel ridge-valley region in Chongqing, being one of the world’s three major fold mountain systems, provides a unique setting for examining how rainfall initiates landslides within its geological context. The area’s pronounced geological features greatly intensify the need for research into a database on rainfall-triggered landslide occurrences in this region and its vicinity. This study focused on a localized heavy storm event that occurred in the Huaying Mountains (in the parallel ridge-valley region of western Chongqing) on 8 June 2017. It revealed the spatiotemporal characteristics of this rainfall event. Using satellite images, we extracted landslides triggered by this event to establish a landslide database. This work not only enriches the landslide database of the Chongqing, but also provides accurate data support for subsequent analysis of landslides triggered by the event. It will also directly contribute to the protection of residents’ lives and property, reduce potential losses caused by landslide disasters, and thereby ensure the long-term stability and development of the community.
The study area is located in the northwest of Chongqing (Figure 1). The coordinates of the study area’s corners are: 106.396° E, 30.075° N; 106.192° E, 29.917° N; 106.321° E, 29.75° N; 106.525° E, 29.909° N. The study area covers approximately 583 km2. The climate in the region is subtropical humid monsoon. It’s characterized by early springs, hot summers, rainy autumns, and mild winters. The average annual temperature is 18.2°C. The average annual precipitation is 1156.8 mm, concentrated from May to September each year. The study area is situated at the southern foothills of the Huaying Mountain, which lies within the parallel ridge-valley region adjacent to the eastern Sichuan Basin. The study area exhibits a terrain marked by anticlines forming hills and synclines forming valleys, with elevations ranging from 120 to 1,000 m. Flowing through the heart of the area, the Jialing River provides abundant water resources. The strata of the study area span a wide range from the Jurassic to the Quaternary. Jurassic covers over 50% of the total area, mainly distributed in flat valleys. These areas are composed of mudstone interbedded with siltstone and feldspathic sandstone. In the mountainous regions, the main underlying strata are Triassic, accounting for over 30%, consisting of quartz sandstone, shale, and limestone (Figure 2).
From June 8th to 10th, 2017, Chongqing experienced an unprecedented rainfall event, resulting in significant economic losses for the region. The severely affected Hechuan District has become the focus of media attention (CCTV, 2017; Lin and Wu, 2017). In response, we utilized the GPM IMERG Final Run product (Huffman et al., 2023) to analyze the precipitation patterns during these three days in Chongqing. Through spatial analysis, we identified the region with the highest precipitation. According to the rainfall distribution, media attention, terrain characteristics, and population density observed from satellite images, we determined the study area, and then carried out research on rainfall-triggered landslides in the study area.
In recent years, the field of landslide identification technology has undergone significant technological innovation, particularly with the application of deep learning techniques (Wang et al., 2021; Yang et al., 2022), which have provided efficient means of identification and high-precision results for disaster emergency response. While the accuracy of deep learning in landslide identification is satisfactory for simple surface environments, its recognition accuracy significantly decreases when dealing with complex surface environments, such as areas near roads. Therefore, at the current stage of technological development, the human-machine interactive method for landslide identification maintains its irreplaceable position.
Our team has made substantial strides in the realm of remote sensing interpretation for event-induced landslides, with a wealth of experience specifically in extracting landslide data from optical remote sensing images. We have successfully developed interpretation criteria for earthquake-induced landslides (Xu et al., 2015; Sun et al., 2024b; Shao et al., 2024) and have also achieved significant breakthroughs in identifying rainfall-induced landslides (Ma et al., 2023a; Cui et al., 2024; Gao et al., 2024). The insights and standards developed for earthquake-induced landslides are readily applicable to the recognition of rainfall-induced landslides in this study, as both types of landslides present marked differences from their surroundings on optical remote sensing images, which is a key indicator for identification. Drawing from our previous research, we have compiled an expert knowledge framework that is a core to the process of landslide interpretation. This framework combines the analysis of optical remote sensing imagery, terrain and geomorphological characteristics, and the mechanisms that trigger landslides, offering a robust scientific foundation for precise landslide identification. Especially for shallow landslides triggered by rainfall events, their distinctive morphological traits (such as compact size and elongated forms) are vital for enhancing the accuracy and efficiency of landslide interpretation.
In this study, based on high-resolution satellite images, all landslide data were extracted by human-computer interaction visual interpretation. We use the Planet satellite image with a resolution of 3 m as the main satellite image. Pre-event imagery dates back to May 2017, captured in a global monthly composite image, while post-event imagery was obtained in July 2017 (Figure 3). Given the spatial resolution of the Planet and extensive traces of human activity in the study area, precautions were taken to avoid misidentifying. For a more accurate identification of landslides, we supplemented the analysis with detailed validation using Google Earth imagery from August 2016 and August 2017 (Figure 4).
Figure 3. The Planet images of the study area pre- and post-rainfall event. (A) May 2017 (B) July 2017.
Figure 4. The Google Earth image depicts the study area marked in yellow in Figure 3 (29.9°N, 106.341°E) pre- and post-rainfall event. (A) August 2016 (B) August 2017.
The lithology data used in the study are from the China Geological Survey (http://dcc.cgs.gov.cn/, accessed on 19 March 2024).
This study collected GPM IMERG Final Run daily precipitation products from June 8th to 11 June 2017, to conduct spatial analysis of the rainfall event in Chongqing (Figure 5). The results indicate that the Hechuan District, Beibei District, Bishan District, Yubei District, and Tongliang District are the areas with the highest precipitation. This rainfall event caused flooding, leading to waterlogging and road inundation in urban areas. Notably, all 27 towns and streets within Hechuan District experienced significant flooding, resulting in severe submersion of agricultural lands. Due to ongoing river diversion construction in Huangjin Village, river surged, causing 30 workers were trapped (Tianqi Network, 2017). Although all trapped workers were successfully rescued by the firefighters, this incident raised concerns about geological disaster prevention. Hechuan and its surroundings, due to their unique geographical and climatic conditions, are prone to geological disasters. The heavy storm induced landslides pose a serious threat to public safety. Therefore, studying the landslides triggered by this rainfall is critical. Through scientific research and effective prevention measures, authorities can better safeguard lives and property and mitigate the recurrence of such disasters.
Figure 5. Spatial distribution of accumulation precipitation in Chongqing from 8 June 2017 to 10 June 2017.
The rainfall process of the study area was analyzed temporally using the 0.5-hour rainfall data from the GPM IMERG Final Run. It was observed that the intense rainfall mainly occurred from 3:00 p.m. on 8 June 2017, to 12:00 a.m. on 9 June 2017. According to the standards outlined in the National Standard of the People’s Republic of China (GBT28592-2012 Grade of precipitation) and the rainfall intensity classification criteria issued by the China Meteorological Administration (Table 1), the total rainfall on June 8th was approximately 85 mm, reaching the level of a heavy storm. Figure 6 shows that the rainfall intensity reached the maximum at 17: 30 on the 8th, about 30 mm/h. The event spanned three days, with a total precipitation of about 87 mm.
Table 1. Precipitation intensity grading standards promulgated by the China Meteorological Administration.
The occurrence of rainfall resulted in the initiation of 487 landslides, covering a total area of about 485,587 m2. This biggest landslide covered an area of about 8,608 m2, while the smallest was 76 m2, with an average landslide area of around 997 m2. Based on the data presented in Figure 7, there was a total of 205 landslides with sizes less than 500 m2, which represents about 42% of the total area of landslides. There were a total of 227 landslides, with sizes ranging from 500 to 2,000 m2, which accounted for around 46% of the total. Furthermore, a total of 45 landslides occurred, with sizes varying between 2,000 and 5,000 m2. There were ten landslides with areas of more than 5,000 m2, all of which were situated on the right bank of the Jialing River (Figure 8). Empirical relationships of landslide area-volume proposed by Guzzetti et al. (2009) were utilized for calculations. The findings reveal that the largest volume of an individual landslide was roughly 35,574 m3, the smallest was around 38 m3, and the average volume was about 2,197 m3.
The studied region has an average landslide number density (LND) of 0.84 num/km2. The area affected by landslides accounts for approximately 0.083%. Based on the search radius of 1 km, the raster resolution was set to 12.5 m, and the Kernel density method was used to plot LND (Figure 9A) and landslide area density(LAD, Figure 9B) maps of landslides. The highest LND value reaches up to 55.6 num/km2. According to the distribution of LND, it is divided into six levels. Specifically, the LND of 40–60 num/km2 covers about 2.5 km2, which represents 0.43% of the total study area; the 20–40 num/km2 group takes up an area of about 4 km2, accounting for 0.67% of the total; the 10–20 num/km2 group occupies 8.0 km2, and its proportion is 1.4%; the region of 5–10 num/km2 is around 10.2 km2, with a ratio of 1.8%; the area and proportion of 2–5 num/km2 are 12.6 km2and 2.2%, respectively; the group with less than 2 num/km2 hold an area of 545.6 km2 which is 93.6% of all. Correspondingly, the analysis of LAD shows that its maximum is 6.4%. The area where LAD is less than 0.5% covers approximately 562.2 km2, which accounts for 96.4% of the total study area; the area with LAD between 0.5% and 1% is about 9.0 km2, representing 1.5% of all; the 1%–2% group takes up 4.8 km2 in area and 0.82% in proportion; the 2%–4% covers an area of about 3.8 km2 which is 0.65% of the study area; the area of 4%–6% in LAD is around 3.1 km2, and its ratio is 0.54%; the region whose LAD is greater than 6% cover approximately 0.3 km2, accounting for about 0.04%.
Figure 9. Spatial density of landslides triggered by this rainfall event. (A) Landslide number density (LND); (B) landslide areal density (LAD).
In this study, we focused on showcasing localized areas of high landslide density within the research zone, aiming to investigate the phenomenon of rainfall-triggered landslides. As depicted in Figure 10, we presented the topography at two different periods (Planet images from May and July 2017), with locations of landslides triggered by rainfall marked by red dashed lines. Comparing these two images, we observe significant color changes in the marked areas, primarily transitioning from vegetated regions to exposed soil. Upon further observation, unlike deep-seated landslides, rainfall-triggered shallow landslides exhibit elongated fluid-like forms. Additionally, shallow landslides tend to have relatively smaller areas, highlighting distinct differences compared to deep-seated landslides. These observations emphasize the varying impacts of different landslide trigger types on morphology, resulting in different landslide morphologies and distribution characteristics. This diversity serves as crucial evidence for landslide identification, aiding in a deeper understanding of landslide formation mechanisms and their significance in geological hazard management.
The interplay of various geological structures and climatic backgrounds under different triggering events leads to diversification of landslide phenomena (Tatard et al., 2010). Furthermore, the ongoing warming of the Earth’s climate system adds complexity and challenges to the understanding of landslide processes (Gariano and Guzzetti, 2016). In this context, establishing a landslide database is crucial for advancing landslide research. Santangelo et al. (2023) have created a database of landslides triggered by extreme rainfall events in the Marche-Umbria region of Italy through field reconnaissance, which includes records of 1,687 landslide events. Martha et al. (2015) mapped a total of 3,472 landslides in the Bhagirathi and Alaknanda river valleys in India following an extreme rainfall event from June 15 to 17, 2013, using satellite remote sensing imagery. This study focuses on the parallel ridge-valley region in Chongqing, one of the world’s three major fold mountain systems, which offers a new perspective for landslide research due to its unique geographical location, geological structure, and climatic environment. The rainfall patterns, geological activity, and topographical features of this region significantly differ from other study areas, most notably in the scale of landslides. The establishment of this database not only enhances our understanding of the characteristics of landslides in the parallel ridge-valley region in Chongqing, but also provides valuable data support and theoretical basis for global landslide research, especially in exploring the triggering mechanisms and evolutionary processes of landslides in fold mountain systems.
A preliminary statistical analysis of the rainfall-triggered landslide was conducted, and its spatial distribution characteristics were discussed. During this rainfall event, the maximum precipitation reached 226 mm, which was recorded in the Baohe (Wang Z. et al., 2019). The area with a high incidence of landslides is primarily situated on the right bank of the Jialing River, exhibiting a northeastward distribution trend, consistent with the orientation of the central mountain range in the study area (Figure 8). Despite the higher rainfall in the western region, the relatively gentle terrain resulted in fewer triggered landslides. In contrast, the eastern region, characterized by more rugged terrain, had fewer landslides triggered, possibly due to its greater distance from the rainfall center. The variation in landslide distribution may be influenced by multiple factors, though currently only preliminary speculation can be made. Future research will focus on analyzing the influencing factors of landslides to further elucidate their formation mechanisms.
By Figures 2, 8, landslides are mostly distributed in the Upper Triassic strata. This geological stratigraphy is largely made up of relatively hard quartz sandstone and interbedded shale with poor permeability and lower hardness. The presence of these weak interbeds makes them extremely vulnerable to deformation and failure under external stresses, resulting in slope instability. To further investigate the mechanisms behind rainfall-induced landslides, we selected the western mountainous region as a comparative area. This location is geographically near to the target area, with similar landscape undulations. The geological features of the western mountainous region reveal that the underlying strata are from the Lower Triassic, and are predominantly formed of limestone, marl and other rocks with comparable characteristics. In contrast, these rock layers are more uniform and less prone to significant deformation and damage, thus posing a relatively lower risk of slope instability. By comparing the lithology of these two areas, the distribution of landslides appears to be reasonable. This finding provides an important geological basis for further study of the formation mechanism of rainfall landslides.
In this study, we utilized the GPM IMERG Final Run daily product with a spatial resolution of 0.1°. Pan et al. (2023) assessed the applicability of GPM in the Chinese mainland. Their research revealed that satellite rainfall data exhibited increasing errors compared to national station data under moderate, heavy, and torrential rainfall conditions. Spatially, the GPM IMERG product performed well in the eastern and southern regions but relatively poorly in the western and northern regions. Temporally, the IMERG product could reasonably estimate the seasonal rainfall distribution in China, with the best performance in summer and the worst in winter. Despite the errors in estimating precipitation in the Chinese mainland, these data still hold significant value. The GPM IMERG precipitation product shows good performance in exploring the spatial distribution of three-day cumulative rainfall in Chongqing. However, for our specific area of interest, this spatial resolution is relatively coarse, leading to discrepancies between the rainfall amounts and those recorded by ground-based observation stations. It should be noted that we believe these differences do not hinder the analysis of spatial distribution characteristics and that of rainfall trends.
Satellite technology plays a crucial role in landslide research, among which Planet images, with its 3-meter resolution, provide high accuracy, offering strong support for landslide extraction. However, rainfall-triggered landslides often have small areas, making precise delineation of landslide boundaries a challenging task. Therefore, landslide interpretation personnel are required to have extensive experience. To validate the spatial accuracy of the established landslide database, a series of validation steps were conducted. Firstly, we used sub-meter-level resolution Google Earth images as a reference to carefully inspect the extracted landslide boundaries. The results showed that although there were some errors, they were still within an acceptable range. More importantly, these errors did not significantly affect subsequent analysis and research, ensuring the reliability and usability of the data obtained.
To obtain comprehensive information about the landslide event, we acquired Planet images from one month before and after the event, and introduced Google Earth satellite images on August 26 after the event as auxiliary evidence while considering the time resolution. Despite the at least one-month interval before and after images, we realize that rainfall landslides are usually triggered by extreme rainfall events (Peruccacci et al., 2012; de Oliveira et al., 2016; Zhang et al., 2022). Although the probability of such extreme events is low, it does not rule out the possibility of other rainfall events triggering landslides during this interval. To validate the accuracy of our landslide database, we further utilized the GPM IMERG Final Run daily product from May to August 2017 to investigate the rainfall conditions in the study area (Figure 11). The results show that from May to August, the maximum rainfall occurred on June 8th (approximately 85 mm/day), while the second-highest rainfall (approximately 39.8 mm/day) occurred on August 25th. The rest of the time mainly experienced light rain, with rainfall much lower than the maximum value. In this study, we used Google Earth images to assist in identifying buildings, farmland, and other features. Now, we applied Google Earth images to examine whether landslides were triggered between June 11th and August 25th (the date of the second-highest precipitation). The results indicate that no new landslides were triggered in this period.
Establishing a comprehensive and accurate database of rainfall-induced landslides is crucial for enhancing the precision of landslide risk assessment and optimizing management strategies. The high accuracy of this database not only deepens our understanding of the processes leading to landslides, but also provides critical data support for the establishment of effective early warning systems. A detailed and precise database of rainfall-induced landslides can be used to train more sophisticated landslide semantic segmentation models (Bragagnolo et al., 2021; Li et al., 2023), which play a vital role in disaster emergency response. These models can quickly identify potential landslide areas, issue timely warnings, and guide evacuations, thereby effectively reducing casualties and property damage. This has a long-term and profound impact on safeguarding people’s lives and property, as well as the sustained development of the social economy.
In the realm of landslide research, the rainfall-triggered landslide threshold has always been a focal point. In earlier studies, Caine (1980) proposed an empirical formula correlating the intensity and duration of rainfall with the incidence of landslides and debris flows, based on an integrated analysis of literature available at that time. Subsequently, an increasing number of scholars have employed statistical methods to investigate rainfall-triggered landslide thresholds. For instance, Guzzetti et al. (2007) exerted rainfall landslide databases of the Central European Adriatic Danubian South-Eastern Space to establish the relationship between rainfall intensity and duration, inferring threshold curves using Bayesian statistical techniques. They further updated Caine’s model by analyzing a database of 2,626 rainfall events worldwide that caused landslides and debris flows (Guzzetti et al., 2008). Rosi et al. (2015) updated 12 rainfall thresholds in Tuscany (Italy) using the MaCumBA software (Segoni et al., 2014). Galanti et al. (2018) derived rainfall thresholds for the Riviera Spezzina region in Italy using least-squares linear fit, quantile regression, and logistic regression, with logistic regression providing the most accurate thresholds. While these studies have made some progress in adjusting the parameters of empirical rainfall threshold formulas, there are still some limitations. Differing from the statistical methods mentioned, Ma et al. (2023b) initially conducted a physics-based spatiotemporal prediction and trigger mechanism analysis of rainfall-induced landslides for four short-duration rainfall events and long-duration intermittent rainfall that occurred from June 19 to 26 July 2013, in the Tianshui area of Gansu Province, China. Building on this, they employed a method based on the TRIGRS physical model, tailored to the specific geological and climatic conditions of the area, to delve into the trigger thresholds for rainfall-induced landslides (Ma et al., 2023c). Additionally, the team conducted an in-depth analysis of the causes of loess landslides triggered by this intense rainfall event (Shao et al., 2023b), which has deepened our understanding of rainfall-induced landslide thresholds. The physics-based modeling approach offers a new perspective for understanding the physical processes of landslides and complements statistical methods, jointly advancing in-depth research in the field. Meanwhile, the advancement of artificial intelligence algorithms has yielded more satisfactory results (Chiang et al., 2022; Distefano et al., 2022).
In exploring the mechanisms behind landslide occurrence, we focuses on the impact of moisture on soil physical properties in this study, particularly the effect of rainfall on soil saturation and shear strength. When rainfall reaches a certain level, the physical properties of the soil undergo significant changes (Moriwaki et al., 2004; Ahmadi-adli et al., 2017), which increases the likelihood of landslides. Based on these observations, the study posits a simplified linear relationship between rainfall volume and the occurrence of landslides. This linear model streamlines the geological processes, making the theoretical framework clearer and easier to operate and validate. At the same time, it provides a foundation for exploring more complex nonlinear relationships, aiding in the understanding of the fundamental conditions for landslide occurrence. The universality of the model makes it applicable across different geological and climatic conditions, facilitating its widespread use and meeting the need for rapid and effective prediction of landslide risk in disaster risk management. Based on the simplified linear model and considering the differences between local observation station data and global satellite precipitation data, it is speculated that the rainfall threshold for the area is much higher than the 39.8 mm/day recorded on August 25th. In fact, there is not a simple linear relationship between precipitation and landslide occurrence. Future research could make use of machine learning models to explore their more complex relationship, aiming to obtain more accurate rainfall thresholds and provide stronger support for landslide hazard assessments and rainfall-induced landslide warning systems.
Through preliminary analysis of a localized heavy storm event near the Huaying Mountain in the parallel ridge-valley area of western Chongqing on 8 June 2017, we established a landslide database triggered by this event using high-resolution satellite images. The study revealed that the rainfall event triggered 487 landslides, affecting an area of approximately 4,85,587 m2, which accounts for 0.083% of the study area. The largest landslide covered an area of about 8,608 m2, while the smallest was 76 m2. The maximum volume of a landslide was approximately 35,574 m3, while the minimum was 38 m3. The average LND was 0.84 num/km2, with the highest LND reaching around 55.6 num/km2, predominantly distributed along the southern foothills of the Huaying Mountain. The maximum LAD was about 6.4%, highlighting the significant impact of extreme climate events on geological disasters. Future research should analyze the factors influencing landslides to reveal their mechanisms. Additionally, more accurate landslide early warning systems could be developed to effectively reduce the occurrence of landslide disasters, thereby ensuring the safety of people’s lives and property.
The raw data supporting the conclusions of this article will be made available by the authors, without undue reservation.
JL: Conceptualization, Investigation, Visualization, Writing–original draft. CX: Conceptualization, Funding acquisition, Resources, Writing–review and editing.
The author(s) declare that financial support was received for the research, authorship, and/or publication of this article. This study was supported by the National Institute of Natural Hazards, Ministry of Emergency Management of China (2023-JBKY-57) and the National Natural Science Foundation of China (42077259).
We would like to express my sincere gratitude to the anonymous reviewers for their professional advice and valuable feedback. Their review not only helped to make the content of this paper more accurate and rigorous but also provided important guidance for its quality improvement. I sincerely appreciate their time and effort devoted to the enhancement of this paper.
The authors declare that the research was conducted in the absence of any commercial or financial relationships that could be construed as a potential conflict of interest.
The author(s) declared that they were an editorial board member of Frontiers, at the time of submission. This had no impact on the peer review process and the final decision.
All claims expressed in this article are solely those of the authors and do not necessarily represent those of their affiliated organizations, or those of the publisher, the editors and the reviewers. Any product that may be evaluated in this article, or claim that may be made by its manufacturer, is not guaranteed or endorsed by the publisher.
Ahmadi-adli, M., Huvaj, N., and Toker, N. K. (2017). Rainfall-triggered landslides in an unsaturated soil: a laboratory flume study. Environ. Earth Sci. 76, 735. doi:10.1007/s12665-017-7049-z
Bragagnolo, L., Rezende, L. R., da Silva, R. V., and Grzybowski, J. M. V. (2021). Convolutional neural networks applied to semantic segmentation of landslide scars. Catena (Amst) 201, 105189. doi:10.1016/j.catena.2021.105189
Caine, N. (1980). The rainfall intensity-duration control of shallow landslides and debris flows. Geogr. Ann. 62, 23–27. doi:10.1080/04353676.1980.11879996
Calvello, M., and Piciullo, L. (2016). Assessing the performance of regional landslide early warning models: the EDuMaP method. Nat. Hazards Earth Syst. Sci. 16, 103–122. doi:10.5194/nhess-16-103-2016
CCTV (2017). Heavy rain strikes Hechuan,: residents trapped, firefighters rescue 149 overnight. Available at: https://tv.cctv.com/2017/06/10/VIDEN5pXhZI8v0DPriFkLLD3170610.shtml (Accessed March 28, 2024).
Chen, Z., Huang, Y., He, X., Shao, X., Li, L., Xu, C., et al. (2023). Landslides triggered by the 10 June 2022 Maerkang earthquake swarm, Sichuan, China: spatial distribution and tectonic significance. Landslides 20, 2155–2169. doi:10.1007/s10346-023-02080-0
Chiang, J.-L., Kuo, C.-M., and Fazeldehkordi, L. (2022). Using deep learning to formulate the landslide rainfall threshold of the potential large-scale landslide. Water (Basel) 14, 3320. doi:10.3390/w14203320
Ciurleo, M., Ferlisi, S., Foresta, V., Mandaglio, M. C., and Moraci, N. (2021). Landslide susceptibility analysis by applying TRIGRS to a reliable geotechnical slope model. Geosci. (Basel) 12, 18. doi:10.3390/geosciences12010018
Cui, Y., Yang, L., Xu, C., and Zheng, J. (2024). Spatial distribution of shallow landslides caused by Typhoon Lekima in 2019 in Zhejiang Province, China. J. Mt. Sci. 21, 1564–1580. doi:10.1007/s11629-023-8377-y
de Oliveira, N. S., Rotunno Filho, O. C., Marton, E., and Silva, C. (2016). Correlation between rainfall and landslides in Nova Friburgo, Rio de Janeiro—Brazil: a case study. Environ. Earth Sci. 75, 1358. doi:10.1007/s12665-016-6171-7
Distefano, P., Peres, D. J., Scandura, P., and Cancelliere, A. (2022). Brief communication: Introducing rainfall thresholds for landslide triggering based on artificial neural networks. Nat. Hazards Earth Syst. Sci. 22, 1151–1157. doi:10.5194/nhess-22-1151-2022
Franceschini, R., Rosi, A., Catani, F., and Casagli, N. (2022). Exploring a landslide inventory created by automated web data mining: the case of Italy. Landslides 19, 841–853. doi:10.1007/s10346-021-01799-y
Galanti, Y., Barsanti, M., Cevasco, A., D’Amato Avanzi, G., and Giannecchini, R. (2018). Comparison of statistical methods and multi-time validation for the determination of the shallow landslide rainfall thresholds. Landslides 15, 937–952. doi:10.1007/s10346-017-0919-3
Gao, H., Xu, C., Xie, C., Ma, J., and Xiao, Z. (2024). Landslides and debris flows triggered by the “July 2023” extreme rainstorm in the Haihe river basin, China. Landslides. doi:10.1007/s10346-024-02322-9
Gariano, S. L., and Guzzetti, F. (2016). Landslides in a changing climate. Earth Sci. Rev. 162, 227–252. doi:10.1016/j.earscirev.2016.08.011
Guo, Z., Chen, L., Yin, K., Shrestha, D. P., and Zhang, L. (2020). Quantitative risk assessment of slow-moving landslides from the viewpoint of decision-making: a case study of the Three Gorges Reservoir in China. Eng. Geol. 273, 105667. doi:10.1016/j.enggeo.2020.105667
Guzzetti, F., Ardizzone, F., Cardinali, M., Rossi, M., and Valigi, D. (2009). Landslide volumes and landslide mobilization rates in Umbria, central Italy. Earth Planet Sci. Lett. 279, 222–229. doi:10.1016/j.epsl.2009.01.005
Guzzetti, F., Peruccacci, S., Rossi, M., and Stark, C. P. (2007). Rainfall thresholds for the initiation of landslides in central and southern Europe. Meteorology Atmos. Phys. 98, 239–267. doi:10.1007/s00703-007-0262-7
Guzzetti, F., Peruccacci, S., Rossi, M., and Stark, C. P. (2008). The rainfall intensity–duration control of shallow landslides and debris flows: an update. Landslides 5, 3–17. doi:10.1007/s10346-007-0112-1
Handwerger, A. L., Fielding, E. J., Sangha, S. S., and Bekaert, D. P. S. (2022). Landslide sensitivity and response to precipitation changes in wet and dry climates. Geophys Res. Lett. 49, e2022GL099499. doi:10.1029/2022GL099499
He, X., Xu, C., Qi, W., Huang, Y., Cheng, J., Xu, X., et al. (2021). Landslides triggered by the 2020 Qiaojia Mw5. 1 earthquake, Yunnan, China: distribution, influence factors and tectonic significance. J. Earth Sci. 32, 1056–1068. doi:10.1007/s12583-021-1492-1
Huang, Y., Xu, C., Zhang, X., and Li, L. (2022). Bibliometric analysis of landslide research based on the WOS database. Nat. Hazards Res. 2, 49–61. doi:10.1016/j.nhres.2022.02.001
Huang, Y., Xu, C., Zhang, X., Li, L., and Xu, X. (2023). Research in the field of natural hazards based on Bibliometric analysis. Nat. Hazards Rev. 24, 04023012. doi:10.1061/NHREFO.NHENG-1739
Huang, Y., Xu, C., Zhang, X., Xue, C., and Wang, S. (2021). An updated database and spatial distribution of landslides triggered by the Milin, Tibet Mw6. 4 Earthquake of 18 November 2017. J. Earth Sci. 32, 1069–1078. doi:10.1007/s12583-021-1433-z
Huffman, G. J., Stocker, E. F., Bolvin, D. T., Nelkin, E. J., and Tan, J. (2023). Final IMERG 1-Month precipitation estimate on global 0.1 x 0.1 degree grid stored in the GeoTIFF format V07. doi:10.25966/3szv-p092
Kirschbaum, D., Kapnick, S. B., Stanley, T., and Pascale, S. (2020). Changes in extreme precipitation and landslides over high mountain Asia. Geophys Res. Lett. 47, e2019GL085347. doi:10.1029/2019GL085347
Lagomarsino, D., Segoni, S., Fanti, R., and Catani, F. (2013). Updating and tuning a regional-scale landslide early warning system. Landslides 10, 91–97. doi:10.1007/s10346-012-0376-y
Li, C., Long, J., Liu, Y., Li, Q., Liu, W., Feng, P., et al. (2021). Mechanism analysis and partition characteristics of a recent highway landslide in Southwest China based on a 3D multi-point deformation monitoring system. Landslides 18, 2895–2906. doi:10.1007/s10346-021-01698-2
Li, H., He, Y., Xu, Q., Deng, J., Li, W., Wei, Y., et al. (2023). Sematic segmentation of loess landslides with STAPLE mask and fully connected conditional random field. Landslides 20, 367–380. doi:10.1007/s10346-022-01983-8
Lin, W., Yin, K., Wang, N., Xu, Y., Guo, Z., and Li, Y. (2021). Landslide hazard assessment of rainfall-induced landslide based on the CF-SINMAP model: a case study from Wuling Mountain in Hunan Province, China. Nat. Hazards 106, 679–700. doi:10.1007/s11069-020-04483-x
Lin, X., and Wu, Q. (2017). Hechuan hit hard by June’s worst rainfall: flooding, landslides, bridge damage. Available at: http://cq.weather.com.cn/gqtj/2720001.shtml (Accessed March 28, 2024).
Ma, S., Shao, X., and Xu, C. (2022). Characterizing the distribution pattern and a physically based susceptibility assessment of shallow landslides triggered by the 2019 heavy rainfall event in Longchuan County, Guangdong Province, China. Remote Sens. (Basel) 14, 4257. doi:10.3390/rs14174257
Ma, S., Shao, X., and Xu, C. (2023a). Landslides triggered by the 2016 heavy rainfall event in Sanming, Fujian Province: distribution pattern analysis and spatio-temporal susceptibility assessment. Remote Sens. (Basel) 15, 2738. doi:10.3390/rs15112738
Ma, S., Shao, X., Xu, C., and Xu, Y. (2023b). Insight from a physical-based model for the triggering mechanism of loess landslides induced by the 2013 Tianshui heavy rainfall event. Water (Basel) 15, 443. doi:10.3390/w15030443
Ma, S., Shao, X., and Xu, C. (2023c). Physically-based rainfall-induced landslide thresholds for the Tianshui area of Loess Plateau, China by TRIGRS model. Catena (Amst) 233, 107499. doi:10.1016/j.catena.2023.107499
Magrì, S., Solimano, M., Delogu, F., Del Giudice, T., Quagliati, M., Cicoria, M., et al. (2024). Modelling rainfall-induced landslides at a regional scale, a machine learning based approach. Landslides 21, 573–582. doi:10.1007/s10346-023-02173-w
Martha, T. R., Roy, P., Govindharaj, K. B., Kumar, K. V., Diwakar, P. G., and Dadhwal, V. K. (2015). Landslides triggered by the June 2013 extreme rainfall event in parts of Uttarakhand state, India. Landslides 12, 135–146. doi:10.1007/s10346-014-0540-7
Moriwaki, H., Inokuchi, T., Hattanji, T., Sassa, K., Ochiai, H., and Wang, G. (2004). Failure processes in a full-scale landslide experiment using a rainfall simulator. Landslides 1, 277–288. doi:10.1007/s10346-004-0034-0
Pan, X., Wu, H., Chen, S., Nanding, N., Huang, Z., Chen, W., et al. (2023). Evaluation and applicability analysis of GPM satellite precipitation over mainland China. Remote Sens. (Basel) 15, 2866. doi:10.3390/rs15112866
Patton, A. I., Rathburn, S. L., and Capps, D. M. (2019). Landslide response to climate change in permafrost regions. Geomorphology 340, 116–128. doi:10.1016/j.geomorph.2019.04.029
Peruccacci, S., Brunetti, M. T., Luciani, S., Vennari, C., and Guzzetti, F. (2012). Lithological and seasonal control on rainfall thresholds for the possible initiation of landslides in central Italy. Geomorphology 139–140, 79–90. doi:10.1016/j.geomorph.2011.10.005
Petley, D. (2012). Global patterns of loss of life from landslides. Geology 40, 927–930. doi:10.1130/G33217.1
Razavi-Termeh, S. V., Shirani, K., and Pasandi, M. (2021). Mapping of landslide susceptibility using the combination of neuro-fuzzy inference system (ANFIS), ant colony (ANFIS-ACOR), and differential evolution (ANFIS-DE) models. Bull. Eng. Geol. Environ. 80, 2045–2067. doi:10.1007/s10064-020-02048-7
Rosi, A., Lagomarsino, D., Rossi, G., Segoni, S., Battistini, A., and Casagli, N. (2015). Updating EWS rainfall thresholds for the triggering of landslides. Nat. Hazards 78, 297–308. doi:10.1007/s11069-015-1717-7
Santangelo, M., Althuwaynee, O., Alvioli, M., Ardizzone, F., Bianchi, C., Bornaetxea, T., et al. (2023). Inventory of landslides triggered by an extreme rainfall event in Marche-Umbria, Italy, on 15 September 2022. Sci. Data 10, 427. doi:10.1038/s41597-023-02336-3
Segoni, S., Rossi, G., Rosi, A., and Catani, F. (2014). Landslides triggered by rainfall: a semi-automated procedure to define consistent intensity–duration thresholds. Comput. Geosci. 63, 123–131. doi:10.1016/j.cageo.2013.10.009
Shao, X., Ma, S., and Xu, C. (2023a). Distribution and characteristics of shallow landslides triggered by the 2018 Mw 7.5 Palu earthquake, Indonesia. Landslides 20, 157–175. doi:10.1007/s10346-022-01972-x
Shao, X., Ma, S., Xu, C., Xie, C., Li, T., Huang, Y., et al. (2024). Landslides triggered by the 2022 Ms. 6.8 Luding strike-slip earthquake: an update. Eng. Geol. 335, 107536. doi:10.1016/j.enggeo.2024.107536
Shao, X., Ma, S., Xu, C., and Xu, Y. (2023b). Insight into the characteristics and triggers of loess landslides during the 2013 heavy rainfall event in the Tianshui area, China. Remote Sens. (Basel) 15, 4304. doi:10.3390/rs15174304
Sun, D., Wen, H., Wang, D., and Xu, J. (2020). A random forest model of landslide susceptibility mapping based on hyperparameter optimization using Bayes algorithm. Geomorphology 362, 107201. doi:10.1016/j.geomorph.2020.107201
Sun, J., Shao, X., Feng, L., Xu, C., Huang, Y., and Yang, W. (2024a). An essential update on the inventory of landslides triggered by the Jiuzhaigou Mw6.5 earthquake in China on 8 August 2017, with their spatial distribution analyses. Heliyon 10, e24787. doi:10.1016/j.heliyon.2024.e24787
Sun, J., Xu, C., Feng, L., Li, L., Zhang, X., and Yang, W. (2024b). The Yinshan Mountains record over 10,000 landslides. Data (Basel) 9, 31. doi:10.3390/data9020031
Tatard, L., Grasso, J. R., Helmstetter, A., and Garambois, S. (2010). Characterization and comparison of landslide triggering in different tectonic and climatic settings. J. Geophys Res. Earth Surf. 115, F04040. doi:10.1029/2009JF001624
Thapa, P. S., Daimaru, H., Ichion, E., and Yanai, S. (2024). Impacts of sediment transported downstream from the 2015 deep-seated landslide in Mt. Hakusan, Japan. Earth Surf. Process Landf. 49, 1273–1288. doi:10.1002/esp.5764
Thiery, Y., Terrier, M., Colas, B., Fressard, M., Maquaire, O., Grandjean, G., et al. (2020). Improvement of landslide hazard assessments for regulatory zoning in France: STATE–OF–THE-ART perspectives and considerations. Int. J. Disaster Risk Reduct. 47, 101562. doi:10.1016/j.ijdrr.2020.101562
Tianqi Network (2017). 2017 Chongqing rainstorm: Hechuan’precipitation broke the record, and 30 people were trapped. Available at: https://www.tianqi.com/news/189235.html (Accessed March 27, 2024).
Vranken, L., Van Turnhout, P., Van Den Eeckhaut, M., Vandekerckhove, L., and Poesen, J. (2013). Economic valuation of landslide damage in hilly regions: a case study from Flanders, Belgium. Sci. Total Environ. 447, 323–336. doi:10.1016/j.scitotenv.2013.01.025
Wang, H., Zhang, L., Yin, K., Luo, H., and Li, J. (2021). Landslide identification using machine learning. Geosci. Front. 12, 351–364. doi:10.1016/j.gsf.2020.02.012
Wang, L., Yin, Y., Zhang, Z., Huang, B., Wei, Y., Zhao, P., et al. (2019a). Stability analysis of the Xinlu Village landslide (Chongqing, China) and the influence of rainfall. Landslides 16, 1993–2004. doi:10.1007/s10346-019-01240-5
Wang, Z., Hu, C., Wu, S., and Mu, D. (2019b). Diagnostic analysis of a heavy rain process on June 8th in Chongqing. Mid-low Latit. Mt. Meteorol. 43, 8–16. (in Chinese). doi:10.3969/j.issn.1003-6598.2019.03.002
Wei, X., Zhang, L., Luo, J., and Liu, D. (2021). A hybrid framework integrating physical model and convolutional neural network for regional landslide susceptibility mapping. Nat. Hazards 109, 471–497. doi:10.1007/s11069-021-04844-0
World Bank, and United Nations (2010). Natural hazards, unnatural disasters: the economics of effective prevention. Washington, DC: The World Bank. doi:10.1596/978-0-8213-8050-5
Wu, R., Li, Z., Zhang, W., Hu, T., Xiao, S., Xiao, Y., et al. (2023). Stability analysis of rock slope under Sujiaba overpass in Chongqing City based on kinematic and numeric methods. Front. Earth Sci. (Lausanne) 11, 181949. doi:10.3389/feart.2023.1181949
Xie, C., Huang, Y., Li, L., Li, T., and Xu, C. (2023). Detailed inventory and spatial distribution analysis of rainfall-induced landslides in Jiexi County, Guangdong Province, China in August 2018. Sustainability 15, 13930. doi:10.3390/su151813930
Xu, C., Xu, X., and Shyu, J. B. H. (2015). Database and spatial distribution of landslides triggered by the Lushan, China Mw 6.6 earthquake of 20 April 2013. Geomorphology 248, 77–92. doi:10.1016/j.geomorph.2015.07.002
Yang, S., Wang, Y., Wang, P., Mu, J., Jiao, S., Zhao, X., et al. (2022). Automatic identification of landslides based on deep learning. Appl. Sci. 12, 8153. doi:10.3390/app12168153
Zhang, Q. X., Wu, Y. P., Ou, G. Z., Fan, X. G., and Zhou, J. H. (2014). Displacement prediction of Liangshuijing landslide based on time series additive model. Comput. Model. and New Technol. 18, 215–223. Available at: http://www.cmnt.lv/upload-files/ns_39art37_CMNT1803-16_Zhang.pdf.
Zhang, S., Jiang, Q., Wu, D., Xu, X., Tan, Y., and Shi, P. (2022). Improved method of defining rainfall intensity and duration thresholds for shallow landslides based on TRIGRS. Water (Basel) 14, 524. doi:10.3390/w14040524
Zhang, X., Chen, L., and Zhou, C. (2023). Deformation monitoring and trend analysis of reservoir bank landslides by combining time-series InSAR and Hurst Index. Remote Sens. (Basel) 15, 619. doi:10.3390/rs15030619
Keywords: rainfall-triggered landslides, database, parallel range-valley area, satellite imagery, western Chongqing
Citation: Liu J and Xu C (2024) Construction and preliminary analysis of landslide database triggered by heavy storm in the parallel range-valley area of western Chongqing, China, on 8 June 2017. Front. Earth Sci. 12:1420425. doi: 10.3389/feart.2024.1420425
Received: 20 April 2024; Accepted: 30 July 2024;
Published: 08 August 2024.
Edited by:
Yanfang Sang, Institute of Geographic Sciences and Natural Resources (CAS), ChinaReviewed by:
Huajin Li, Chengdu University, ChinaCopyright © 2024 Liu and Xu. This is an open-access article distributed under the terms of the Creative Commons Attribution License (CC BY). The use, distribution or reproduction in other forums is permitted, provided the original author(s) and the copyright owner(s) are credited and that the original publication in this journal is cited, in accordance with accepted academic practice. No use, distribution or reproduction is permitted which does not comply with these terms.
*Correspondence: Chong Xu, eGMxMTExMTExMUAxMjYuY29t
Disclaimer: All claims expressed in this article are solely those of the authors and do not necessarily represent those of their affiliated organizations, or those of the publisher, the editors and the reviewers. Any product that may be evaluated in this article or claim that may be made by its manufacturer is not guaranteed or endorsed by the publisher.
Research integrity at Frontiers
Learn more about the work of our research integrity team to safeguard the quality of each article we publish.