- 1Guizhou Geological Survey, Bureau of Geology and Mineral Exploration and Development Guizhou Province, Guiyang, China
- 2Engineering Technology Innovation Cente of Mineral Resources Explorations in Bedrock Zones Minlsity of Natural Resources, Guiyang, China
- 3Evaluation and Utilization of Strategic Rare Metals and Rare Earth Resource Key Laboratory of Sichuan Province, Sichuan Institute of Comprehensive Geological Survey, Chengdu, China
- 4Faculty of Geography, Yunnan Normal University, Kunming, China
- 5Yunnan Key Laboratory of Plateau Geographic Processes and Environmental Change, Kunming, China
- 6Yunnan Haiju Geographic Information Technology Co., Ltd., Kunming, China
- 7College of Resources and Environmental Engineering, Guizhou University, Guiyang, China
Liupanshui City in Guizhou Province represents a karst mountainous region with a delicate geological environment. The area has a long history of coal mining, and several coal mines have been progressively closed in recent years. However, even after closure of coal mines, the fractured rock mass in the mined-out areas continues to undergo deformation over a specific period. In karst mountainous regions, this deformation is affected by various factors including elevation, slope, precipitation, and vegetation. In this study, we employed SBAS-InSAR technology to construct a time series of surface deformation data from January 2019 to May 2022 within Liupanshui City’s LuJiaZhai-DaPingDi Minefield. Subsequently, this data was comprehensively analyzed in conjunction with time series vegetation cover, monthly precipitation, elevation, and slope data from the identical period. The key findings of this research are as follows: 1) After the closure of the mine, the subsidence area gradually stabilized, yet the volume of subsidence continues to increase. The subsidence area primarily occurs near the MaiZiGou Coal Mine air-mining area, which had a relatively short closure time, as well as in higher elevation areas in the southern and eastern parts of the minefield. Specifically, the maximum cumulative subsidence and the subsidence rate during the study period reached −60.3 mm and −21.83 mm/a, respectively. 2) Surface deformation is closely linked to slope, vegetation type, and rainfall, with subsidence rates and amounts noticeably higher during the rainy season than in the dry season. 3) Surface vegetation cover types display varied effects on deformation, with grassland or shrub areas being more sensitive to precipitation than forests. Forests, especially pine and fir, show a delayed subsidence response to rain, typically 2–3 months post-rainy season onset. This inhibitory effect lessens with increasing slope, particularly beyond a 25° threshold, where responsiveness to precipitation and associated subsidence significantly increase. The findings of this study hold substantial scientific implications for the restoration and management of closed mining areas, as well as the prevention of geological hazards in karst complex mountainous regions.
1 Introduction
Karst landscapes are widespread throughout China. Specifically, soluble rocks of Karst landscapes cover over an expansive area of up to 365 × 104 km2, which accounts for more than one-third of the national territory (Sun et al., 2023). Guizhou Province stands out as the region of Karst landscapes due to its extensive development of carbonate rocks and the thickest exposure, predominantly composed of limestone and dolomite, resulting in the formation of representative karst landforms (Chu et al., 2015). Additionally, Guizhou Province is characterized by significant coal resources, making it one of the southern Chinese regions with abundant coal reserves. The primary coal-producing areas are located within the zone of karst landscape development. The complex terrain and fragile geological environment of this karst mountainous region (Shi and Zhang, 2023), coupled with years of coal mining, have exacerbated surface deformation issues. Furthermore, large-scale landslides and collapses frequently occur, causing severe casualties and property losses (Wang et al., 2020; Chen H. et al., 2022; Zhong et al., 2022; Zhao Q. et al., 2023).
Coal, as a vital energy source (Yu et al., 2021), is extensively utilized in industrial production and electricity generation, remaining a primary source of energy in many countries (Chen et al., 2020). China, with its abundant coal reserves and limited oil resources, heavily relies on coal as a fundamental energy source and essential raw material (Chen B. et al., 2023), accounting for over 60% of primary energy consumption (Deng et al., 2022). However, the exploitation of coal resources has given rise to significant geological environmental challenges, particularly concerning long-term coal mining in the fragile surface environments of karst complex mountainous regions. Prolonged coal mining activities can lead to ground subsidence, damage to surface structures, and the occurrence of geological hazards such as ground fissures, landslides, and surface collapses. These hazards pose severe threats to the productivity and safety of local communities, as well as the construction and operation of critical national infrastructure such as power grids and transportation routes (Zhu Q. et al., 2019; Li et al., 2019; Li et al., 2021). In the 1980s, China experienced a substantial demand for coal resources, resulting in a rapid increase in the number of coal mines in mining cities. Over time, the intensive and large-scale extraction of coal resources has led to resource depletion in certain areas. Furthermore, recent adjustments to China’s energy structure and the implementation of supply-side structural reforms have prompted the consolidation and closure of small, scattered, and disorderly coal mines, significantly increasing the number of closed coal mines in the country. The closure of these mines leaves behind a considerable amount of land resources, leading to various issues related to resources, environment, safety, and society, which have gradually become apparent (Hu and Yan, 2018; Chen Z. et al., 2022; Li et al., 2022). The transformation, redevelopment, and management of the ecological environment of these closed mines have become a critical concern for the sustainable economic and social development of resource-based cities. Once a mine is closed, the rock mass of the coal seam undergoes weathering deterioration and a reduction in strength due to factors such as stress and groundwater. Consequently, the stress and bearing capacity of the rock mass, disrupted by mining activities, change, often resulting in secondary subsidence of the air-mined area and the surrounding ground surface (Chen B. et al., 2022). This deformation, particularly in complex karst mountainous regions, tends to exhibit complex, concealed, sudden, and long-term characteristics, posing potential threats to the mining area and its surrounding engineering construction and mining geological environment. Therefore, there is an urgent need for comprehensive, long-term, and high-precision monitoring of subsidence in closed mines. Furthermore, it is crucial to explore the intricate relationship between surface deformation in closed mining areas and variables such as elevation, slope, precipitation, and vegetation cover. This exploration serves as a vital prerequisite for conducting ecological restoration and management of mining areas.
Despite high precision in subsidence monitoring, traditional methods such as precise leveling measurements (Wang, 2013), GPS measurements (Zhao and Zhu, 2020), and crack gauges (Bai et al., 2020) suffer from limitations such as low point density, providing only discrete subsidence data in well-explored deformation areas. Moreover, these methods are characterized by long observation cycles, high costs, lower efficiency, and struggle to meet the increasing demand for regional subsidence monitoring. In contrast, Interferometric Synthetic Aperture Radar (InSAR) technology has gained widespread adoption for surface deformation monitoring due to its advantages such as all-weather capability, extensive coverage, high precision, and cost-effectiveness (Zhu et al., 2017; Yang et al., 2020). Nevertheless, in mining areas, the presence of dense surface vegetation and significant mining-induced deformations often results in decreased coherence of SAR interferograms (Zhu J. et al., 2019). This presence poses a challenge for conventional differential InSAR (D-InSAR) techniques, making it difficult to achieve optimal results in deformation monitoring in mining areas. To address this challenge, mining area InSAR monitoring has transitioned from D-InSAR to time series interferometric synthetic aperture radar (TS-InSAR). TS-InSAR effectively mitigates the challenges associated with D-InSAR, including spatial-temporal decorrelation, DEM errors, and atmospheric delay disturbances, while conforming to the requirements for prolonged dynamic monitoring in mining areas (Du et al., 2017; Tang et al., 2021; Liu et al., 2022). Noteworthily, existing research employed two primary techniques for mining area deformation monitoring based on TS-InSAR, including the phase stacking method (Zhang et al., 2021) and the small baseline subsets InSAR (SBAS-InSAR) (Berardino et al., 2002). Both of the aforementioned techniques are well-suited for long-term deformation monitoring in mining areas and have gained widespread use in subsidence monitoring. Zhang et al. (2016) utilized the Stacking InSAR method to monitor subsidence in the Pei Bei mining area, such that this method is confirmed to be effective in large-scale deformation monitoring. Based on Sentinel-1 data, Zhang et al. (2018) employed a novel TOPS imaging mode Stacking technique to analyze ground subsidence characteristics in the Huainan mining area, achieving excellent monitoring results. Their work also underscored the advantages of the Sentinel-1 satellite constellation, with its short revisit cycle and wide coverage, making it particularly suitable for subsidence monitoring in mining areas with expansive subsurface regions. Similarly, Xu et al. (2022) applied three InSAR techniques based on Sentinel data to extract mining-induced deformations in the Datong Coalfield from November 2020 to October 2021. The results suggested that both Stacking InSAR and SBAS InSAR outperformed D-InSAR, with SBAS InSAR providing more precise displacement rate results. Notably, Stacking InSAR exhibited superior performance in densely vegetated or low-coherence regions.
The Liupanshui region boasts abundant coal resources and a longstanding mining history. Researchers have successfully utilized InSAR technology to investigate surface deformation in this region. Zhu et al. (2022) employed Stacking InSAR technology, alongside multi-temporal optical remote sensing images, stratigraphy, and geomorphology, to identify 588 active landslides in western Guizhou for the first time. These landslides, primarily mining-induced (91.8%), reservoir bank-induced (4.1%), and reactivated ancient ones (2.4%), underscore the profound impact of coal mining on regional landslides, especially in steep, elevated areas. Wu et al. (2021) applied SBAS InSAR technology using Sentinel-1 radar images for extensive surface deformation monitoring in Liupanshui, Tongren, and Guiyang, Guizhou, identifying 102 hazard areas. Using optical images, they identified 72 deformation areas from mining or construction, along with 16 landslides and 14 suspected landslides. Chen L. et al. (2023) utilized the Intermittent Small Baseline Subset (ISBAS) method with Sentinel-1 images to determine Guizhou’s surface deformation rate, mapping 693 active landslides. They noted a significant correlation between landslide distribution and factors such as altitude, slope, and coal-bearing strata. The aforementioned researchers have effectively utilized InSAR technology for a comprehensive surveillance of Guizhou’s landslides, providing crucial insights into the macroscopic distribution of surface deformation in the area. Furthermore, researchers have examined and analyzed the destabilization mechanisms behind typical mining-induced landslides in the region (He et al., 2022; Zhao C. et al., 2023; Chen H. et al., 2023; Li et al., 2023). Literature review suggests that research primarily concentrates on extensive landslide monitoring and analyzing deformation and destabilization mechanisms of typical mining-induced landslides in Guizhou’s Liupanshui area. Reports on surface deformation of closed mines in this region are scarce. Recent studies on closed mines predominantly focus on long-term monitoring, subsidence trends, and post-closure mechanism analysis in plain areas (Deng et al., 2015; Yu et al., 2021; Liang and Hu, 2022; Qin et al., 2022; Zhang et al., 2023). At present, the majority of researchers have primarily concentrated on long-term monitoring and the analysis of subsidence trends and mechanisms after mine closure. Nevertheless, in the karst mountainous regions of southwestern China, factors (e.g., high altitudes, steep slopes, concentrated precipitation, and diverse vegetation types) complicate the subsidence patterns in closed mines. These mines not only experience the effects of underground goaf areas but are also affected by the mentioned environmental factors. Therefore, investigating the complex relationship between surface deformation within closed mining areas and multiple influencing factors in this region holds significant practical importance.
LiuPanshui City, renowned as the primary “Coal Capital of South China,” possesses abundant coal reserves and occupies a significant position as a major coal-producing city in Guizhou Province and even China. The coal mines in this region are predominantly located in high-altitude areas characterized by complex geological formations, including karst mountains. Extensive coal mining activities over the years have exacerbated surface deformations, resulting in the emergence of geological hazards such as ground fissures, landslides, and subsidence (Fang et al., 2016; Wu et al., 2021). In recent times, LiuPanshui City has closed several coal mines, prompting this study to focus on the LuJiaZhai-DaPingDi Minefield as a representative case. Leveraging 100 scenes of Sentinel-1 ascending orbit data spanning from January 2019 to May 2022, the study employs the Small Baseline Subset (SBAS) InSAR technique to continuously monitor surface deformations in four closed mines within the region. Subsequently, the research investigates the intricate relationships among elevation, slope, vegetation coverage, monthly precipitation, and surface deformations. The outcomes of this investigation can serve as a valuable foundation for decision-making pertaining to ecological restoration, judicious land reuse, and the prevention of geological hazards in closed mining areas.
2 Study area and data sources
2.1 Study area overview
The study area encompasses the LuJiaZhai-DaPingDi Minefield (Figure 1), located in the western region of Liupanshui City, Guizhou Province. The mining area’s central coordinates are 104°31′37.2″E, 25°59′45.6″N. It falls into the transitional slope zone from the Yunnan Plateau to the central Guizhou mountain area, and is characterized as a typical southwestern karst mountain region. The terrain in this area is intricate, featuring expansive canyons, towering peaks, steep slopes, and deep valleys. It exhibits poor stability and limited resistance to interference. The elevation within the region ranges from 1,501 to 2048 m, with a relative height difference of 547 m. The slopes are notably steep, of which the steepest slope reach 88.27 degrees. The mining area comprises MaiZiGou Coal Mine, LongTouShan Coal Mine, FuGuiZhuang Coal Mine and BaiPing Coal Mine, which have a long history of mining but are currently closed, with the time of mine closure depicted in Figure 1D.
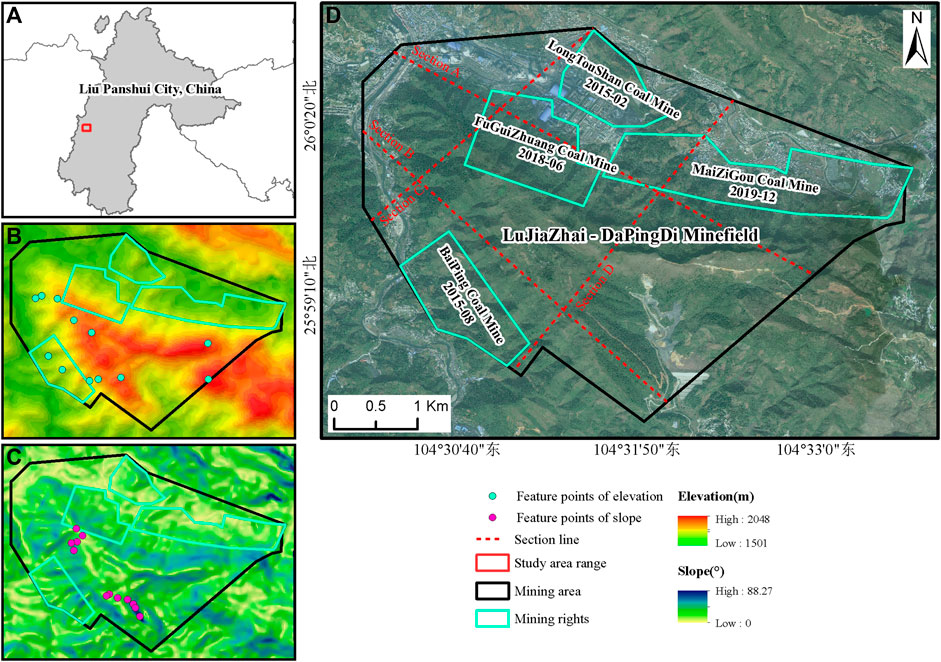
FIGURE 1. Overview of the Study Area and Mine Closure Time. ((A) illustrates the study area's schematic location, (B) its altitude, (C) its slope, and (D) the coal mining rights and mine closure times).
The strata present in the mining area demonstrate a characteristic geological pattern known as “hard on top, soft below.” These formations can be classified into three distinct rock types: hard rock, interbedded hard and soft rock, and soft rock. The hard rock category encompasses the Guanling Formation from the Triassic period and the Emeishan Basalt Formation from the Permian period. These formations consist of limestone, dolomite, and dolomitic limestone, which are characterized by their hardness, brittleness, and high mechanical strength. However, unfavorable slope conditions can lead to deformation and collapse in these rocks. The interbedded hard and soft rock category includes the Yungningzhen Formation from the Triassic period. This formation comprises thin to medium-thickness layers of limestone, dolomite, shale, sandstone, and mudstone. These rocks exhibit variable lithological properties and complex combinations. The presence of karst development, active groundwater flow, high mechanical strength of carbonate rocks, and resistance to weathering contribute to their stability. Nevertheless, the existence of weaker interlayers can result in landslides and other geological hazards under unfavorable slope conditions. The soft rock category consists of the Feixianguan Formation from the Triassic period and the Longtan Formation from the Permian period. These formations consist of thin to medium-thickness layers of mudstone, sandy mudstone, siltstone, sandstone, shale, and coal seams. These rock formations are highly susceptible to disturbances caused by mining activities and exhibit weak resistance to weathering. They tend to soften when exposed to water, leading to poor stability. Under unfavorable slope conditions, these rock formations are prone to landslides, collapses, and other geological hazards.
2.2 Data sources
The study employed Sentinel-1 ascending orbit satellite data to cover the study area. Sentinel-1 is a radar satellite equipped with a C-band radar sensor and is part of the European Space Agency’s Copernicus program. The Interferometric Wide (IW) imagery mode and Single Look Complex (SLC) data format were utilized. The data was collected with HH polarization and had a spatial resolution of 5 m × 20 m. The dataset spanned from January 2019 to May 2022, comprising a total of 100 scenes. This dataset was utilized to construct a time-series of deformation information within the study area. To enhance data accuracy, AW3D30 Digital Surface Model (DSM) data was used for terrain phase correction. Geocoding of the data was performed using SAR imagery, following the methodology described by Yang et al. (2021). Systematic errors resulting from orbital inaccuracies were mitigated using Precise Orbit Ephemerides (POD) data. Landsat8 OLI remote sensing images, provided by the USGS, were used to extract Fraction of Vegetation Coverage (FVC) information for the same time period. The extraction of FVC information was conducted on the Google Earth Engine platform. This involved using Landsat OLI remote sensing images from January 2019 to 2022 and calculating the Normalized Difference Vegetation Index (NDVI) based on the image element dichotomous model. The Landsat OLI remote sensing images from January 2019 to May 2022 were utilized to calculate the monthly NDVI for the study area, and subsequently, the time-series FVC of vegetation cover was calculated based on the image element dichotomous model (Adams et al., 1986). Furthermore, ASTER Global Digital Elevation Model (GDEM) data from NASA was utilized to obtain elevation and slope information for the study area. Monthly average precipitation data for the study area was obtained from the China Meteorological Data Network. Further details regarding the data sources can be found in Table 1. To synchronize the InSAR cumulative deformation, FVC, and monthly rainfall data for time series analysis, we computed both the monthly cumulative deformation and FVC data, with the month serving as the measurement unit.
3 Research methods and technical principles
3.1 SBAS-InSAR technology principlesw
The small baseline subsets InSAR (SBAS InSAR) technique, initially introduced by Berardino et al. (2002) in 2002.
The fundamental principle of this technique assumes the collection of
In general, vertical baseline thresholds and time baseline thresholds are established based on actual conditions to limit the range of
Where
Where
Where
In this study, the processing of Sentinel-1 data involved multi-view processing with spatial resolutions of 23.8 m × 28.0 m in the range and azimuth directions, respectively, using a 10 × 2 multi-view ratio. The differential interferometric combinations employed a maximum time interval of 48 days and a maximum vertical baseline of ±250 m. This resulted in a total of 416 interferometric pairs acquired from ascending orbit data. The differential interferometric processing was performed on these combinations, leading to the generation of differential interferograms and coherence coefficient maps. To mitigate phase noise, an adaptive filtering method was applied. Subsequently, phase unwrapping was carried out on the filtered interferograms, utilizing the Minimum Cost Flow (MCF) method for this purpose. After error removal, the phase unwrapping results underwent Stacking and Small Baseline Subset (SBAS) processing to obtain deformation rates and time-series results.
3.2 Stacking technology principles
Stacking refers to a technique (Sandwell and Price, 1998) used to perform a weighted average solution on multiple differential interferograms acquired through the Differential Interferometric Synthetic Aperture Radar (D-InSAR) method. This process is aimed at obtaining linear phase deformation rates to mitigate the influence of spatially uncorrelated noise. The solution format can be expressed as follows (Eq. 3):
Where
4 Results and analysis
4.1 Subsidence rate results and accuracy analysis
Using SBAS-InSAR technology, we obtained radar line of sight (LOS) deformation rates (Figure 2) and cumulative time-series deformation results (Figure 4) for four closed mines in the LuJiaZhai-DaPingDi Minefield in Liupanshui City from January 2019 to May 2022. In these figures, positive values indicate vertical surface uplift (i.e., along the LOS), while negative values represent vertical subsidence (i.e., along the LOS). As depicted in Figure 2, during the study period, significant deformation was observed in the northeastern and southwestern parts of the mining area. The maximum annual average subsidence rate and the maximum uplift rate within the mining area, located in the western and southern regions of the MaiZiGou Coal Mine, were determined as −21.83 mm/year and 8.13 mm/year, respectively. In the western part of the MaiZiGou Coal Mine, a prominent subsidence center was observed, with subsidence rates irregularly spreading out in an elliptical pattern from the center. This subsidence was notably higher than the other three mines. The overall subsidence within the mine was oriented in an “east-west” direction, primarily affected by the closure time of the mine and the distribution of old goaf areas. The MaiZiGou Coal Mine was closed in December 2019 but was still operational in 2019, resulting in higher and concentrated subsidence rates in the western part of the mine. Furthermore, multiple subsidence centers were observed in the southwestern part of the mining area, which were distributed in a “northwest-southeast” direction, of which the maximum subsidence rate was determined as −15.13 mm/year.
It is imperative to assess the reliability of the monitoring results before the analysis of the results of ground subsidence monitoring. Currently, there are two commonly used accuracy validation methods: internal consistency accuracy validation and external consistency accuracy validation. Due to the lack of concurrent ground-based monitoring data, this study employs the internal consistency accuracy validation method. This method involves cross-validating the subsidence rate results extracted by two different algorithms to investigate the reliability of the surface subsidence monitoring results. Using the SBAS technique to extract Line of Sight (LOS) deformation rates in the study area as the X-axis and the LOS deformation rates extracted by the Stacking technique as the Y-axis, a scatter density plot was created, as presented in Figure 3. The correlation coefficient (R2) between the LOS deformation rates for corresponding points obtained by the two methods was 0.7017, indicating a high degree of correlation between the deformation rates obtained by the two InSAR techniques in the study area, thus validating the reliability of the subsidence monitoring results in the study area.
To evaluate the extent of ground subsidence development in the study area, this research classifies the ground subsidence rates into four distinct categories, as outlined in Table 2. During the period spanning from January 2019 to May 2022, the LuJiaZhai-DaPingDi Minefield exhibits the following tiers of ground subsidence: Areas experiencing subsidence rates below −15 mm/year are classified as regions with a high degree of ground subsidence development, constituting a mere 0.29% of the overall mining area. Subsidence rates ranging from −15 mm/year to −5 mm/year indicate regions with a moderate level of ground subsidence development, encompassing approximately 19.34% of the total mining area. Regions with subsidence rates falling between −5 mm/year and 5 mm/year denote areas with relatively stable ground subsidence development, representing the largest portion, accounting for 79.55% of the entire mining area. Areas exhibiting subsidence rates exceeding 5 mm/year are categorized as uplifted regions, comprising a mere 0.82% of the total mining area. This classification system serves as a valuable tool for evaluating and characterizing the extent of ground subsidence development in the study area, enabling a comprehensive understanding of the spatial distribution of subsidence and uplift patterns within the mining region.
The results indicate that, following the closure of the mines, the overall proportion of surface deformation areas within the LuJiaZhai-DaPingDi Minefield is relatively high. Except for the relatively stable areas, the combined area of other subsidence development levels accounts for 20.45% of the total mining area.
4.2 Spatial and temporal distribution analysis of surface deformation in the mining area
This study aimed to analyze the spatiotemporal distribution patterns of surface deformation in various closed mines and the mining area. To accomplish this, the study utilized acquired subsidence rates and performed temporal integration to assess the cumulative subsidence over time in the study area (Figure 4). Nine equally spaced time intervals were selected to represent the cumulative subsidence for each period.
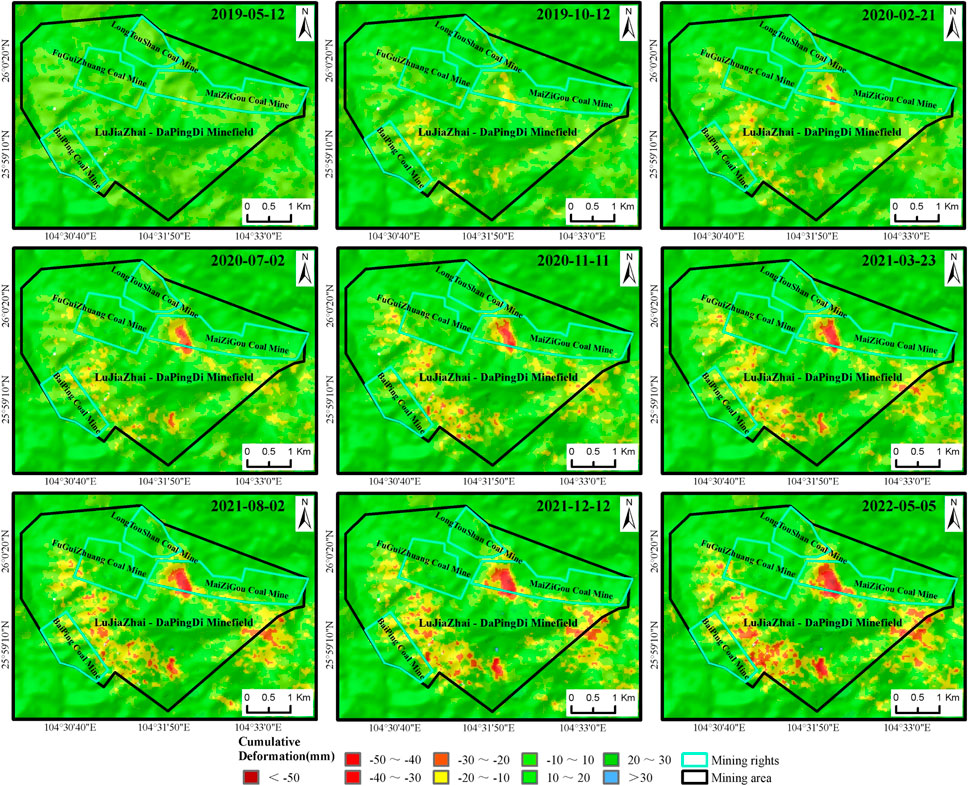
FIGURE 4. Temporal Cumulative Subsidence in the Lu LuJiaZhai-DaPingDi Minefield for Closed Mines (reference time: 2019-01-09).
Figure 4 demonstrates that the distribution of cumulative subsidence aligned with the subsidence rates. Overall, the region’s different mines underwent varying degrees of deformation. The highest cumulative subsidence was observed in the MaiZiGou Coal Mine, measuring −60.3 mm, while the maximum uplift was reported in the southern part of the study area, with a magnitude of 34.17 mm. During the study period, the MaiZiGou Coal Mine was operational in 2019 but closed by the year’s end. However, deformation persisted even after closure, expanding from the goaf area to the surrounding regions. The maximum cumulative subsidence and uplift reached −60.3 mm and 21.45 mm, respectively. The FuGuiZhuang Coal Mine ceased operations in June 2018. Within the study period, this mine experienced a maximum cumulative subsidence of −23.48 mm and an uplift of 22.82 mm. Subsidence primarily affected the higher-altitude western area of the mine. Although cumulative subsidence increased over time, deformation in this mine stabilized, with limited diffusion. The LongTouShan Coal Mine closed in February 2015. Its western part remained relatively stable without significant deformation, while the eastern part experienced cumulative subsidence of −24.18 mm and uplift of 19.71 mm, influenced by the MaiZiGou Coal Mine. The BaiPing Coal Mine closed in August 2015. Within its boundaries, the maximum cumulative subsidence and uplift were −21.86 mm and 22.44 mm, respectively. Deformation in this area remained relatively stable, primarily concentrated in the higher-altitude northeastern region of the mine, with ongoing deformation.
Notably, two distinct subsidence clusters were observed near the closed mines. One cluster was located in the southwestern part of the mining area, while the other was found in the eastern region, characterized by higher altitudes and steeper slopes. These clusters experienced cumulative subsidence and uplift of −49.93 mm and 27.95 mm, respectively. Figure 4 indicates that the extent and magnitude of deformation within these areas continued to expand throughout the study period. Although the range of deformation stabilized by May 2022, the deformation values were still increasing, indicating ongoing subsidence beneath the surface with future persistence.
The study also included two profile lines for the LuJiaZhai-DaPingDi Minefield, one in the longitudinal direction and the other in the transverse direction (Figure 1D). These profile lines were strategically positioned to intersect areas with significant subsidence. Subsidence rates and elevation values were then extracted from these profiles. Figure 5 illustrates an overall negative correlation between subsidence rates and elevation. This suggests that as elevation increases, surface deformation becomes more frequent and exhibits a fluctuating decreasing trend. In contrast, as elevation decreases, subsidence rates decrease and tend to stabilize. In the northern section of Profile Line D, influenced by the closure time of the MaiZiGou Coal Mine, a “V”-shaped curve became evident around the profile line points near 100.
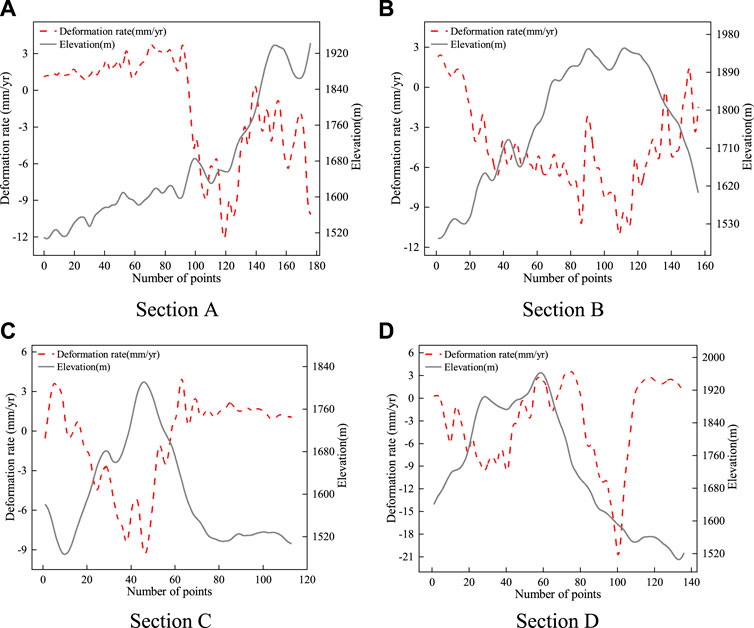
FIGURE 5. Profile of Subsidence Rate and Elevation. [(A–D) depict the subsidence rates and elevations for cross-section lines (A) through (D), respectively].
The findings of this study indicate that surface deformation within the closed mining area is influenced not only by the timing of mine closures but also by the elevation of the region. This correlation can be attributed to the study area’s characteristics as a typical karst mountainous region, where high elevations and significant topographic variations are prevalent. These high-elevation areas are often characterized by steep slopes and deep valleys. Due to external factors such as precipitation, these regions are more susceptible to complex and concealed surface deformation. Additionally, elevation plays a role in determining the types of vegetation covering the surface. Different vegetation types exert diverse effects on slope stability (Asada and Minagawa, 2023). Consequently, this study places particular emphasis on investigating the interconnected relationship between surface deformation, elevation variations, slope characteristics, vegetation distribution, and precipitation patterns following mine closures within karst regions.
4.3 Analysis of surface deformation at different elevation gradients and slope levels
To conduct a comprehensive analysis of surface deformation within a mining area in a complex karst region following mine closures at various elevations and slopes, and to investigate the interplay between surface deformation, precipitation patterns, and vegetation coverage, this study employed two sets of deformation feature points for cross-validation purposes within the designated study area. The selection of these feature points aimed to minimize the influence of anthropogenic activities, such as urban areas and croplands, on surface deformation. Figure 1B illustrates the deformation feature points at different elevations (points a to l), while Figure 1C displays the feature points at various slopes (points m to x). The primary objective of this research is to examine the relationship between surface deformation within a mining area in a typical karst region after mine closures, considering different elevations and slopes, as well as its interaction with precipitation and vegetation coverage.
Figure 6 presents time series curves depicting the deformation patterns at different elevations, ranging from 1566 m to 2022 m. The subsidence trends for these points consistently exhibit fluctuating downward movement. Among these points, the maximum cumulative subsidence is −31 mm (point c), while the minimum is −16 mm (point f), with the remaining points falling within the −20 mm to −30 mm range. Precipitation in the study area is concentrated between May and October, while vegetation coverage is particularly high from June to September. Generally, the subsidence values at these points display a systematic response to precipitation. During the rainy season, subsidence rates and magnitudes increase significantly, whereas during the dry season, the points tend to experience sliding or uplift. Notably, points a, c, d, and e exhibit a more rapid response to precipitation, showcasing significant subsidence early in the rainy season as precipitation accumulates. In contrast, points b and f demonstrate a delayed response, with noticeable subsidence occurring two to 3 months after the onset of the rainy season.
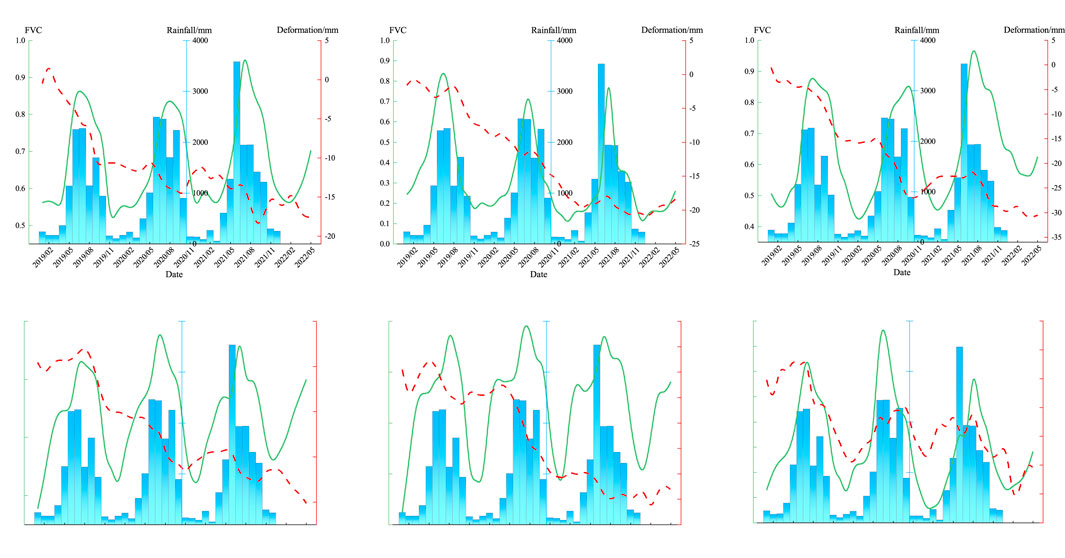
FIGURE 6. Time Series Curves of Deformation Points at Different Elevations. [(A–F) have elevations of 1566 m, 1672 m, 1729 m, 1829 m, 1930 m, and 2022 m, respectively].
Precipitation is a significant contributing factor to surface deformation and geological hazards, such as landslides and collapses, particularly when precipitation is prolonged and heavy (Zhang et al., 2020; Wang et al., 2022; Ma et al., 2023; Pei et al., 2023). In the designated study area, precipitation is concentrated within specific periods, with substantial amounts recorded. Monthly average precipitation at the deformation points from May to October can reach as high as 1887 mm, and certain months exhibit maximum monthly precipitation of up to 3,586 mm. The unique geological conditions in this area, characterized by a layered structure of hard rock atop soft rock and the presence of coal seams in the Longtan Formation (P3l) underlying the mountains, have been disrupted by past coal mining activities, resulting in the formation of fractures. Consequently, precipitation plays a significant role in surface deformation and the development of geological hazards in this region. Figure 6 demonstrates that subsidence is more pronounced during the rainy season. This can be attributed to two main factors. Firstly, heavy precipitation leads to surface soil erosion and extensive infiltration through rock fractures, resulting in the softening of rocks and soils, increased water content, and added weight to the slopes. Prolonged precipitation further saturates the soft rock layers, significantly reducing their shear strength and resistance to sliding along contact surfaces. The combination of increased material weight and slope gradient accelerates surface subsidence. Secondly, the study area exhibits typical characteristics of a complex karst mountainous region, with underlying fractured rock bodies and well-developed joint and fracture networks influenced by tectonic movement. Previous coal mining activities have created pathways for atmospheric precipitation to penetrate the rock mass. Prolonged precipitation intensifies dissolution processes, leading to the formation of numerous karst fissures and conduits. These geological features reduce the stability of the mountain mass and trigger surface deformation.
In general, a scarcity of vegetation coverage leads to a relatively exposed surface with limited capacity for retaining soil, rendering it more vulnerable to subsidence during prolonged and heavy precipitation (Jacquemart and Tiampo, 2021). Conversely, high vegetation coverage indicates the presence of well-established surface vegetation, which enhances the soil’s ability to retain its structure. However, despite the substantial vegetation coverage during the rainy season, significant surface subsidence persists in the study area, indicating a robust response of surface deformation to precipitation. Additionally, it is important to note that vegetation coverage solely reflects the extent of surface vegetation and does not consider the specific types of vegetation present. Different vegetation types have varying impacts on soil retention capacity (Zhang et al., 2020). Among the deformation points presented in Figures 6A–F, with the exception of deformation point d, which has a slope of 33°, the other deformation points exhibit similar slopes ranging from 11° to 19°. Deformation points b and f demonstrate distinct deformation patterns compared to other feature points. These points are predominantly covered by dense coniferous trees, including tall species such as fir and pine. The presence of these tree species, characterized by expansive canopies and deep-rooted systems, plays a crucial role in stabilizing the soil structure, thereby reducing soil loosening and collapse. Furthermore, the dense canopies of fir and pine trees act as effective buffers during precipitation, mitigating the impact of precipitation on the soil. Moreover, the accumulation of fallen branches and leaves on the ground resulting from these trees significantly decelerates water flow and erosion, contributing positively to soil and water conservation efforts.
Although deformation points a, c, d, and e have relatively high vegetation coverage, they exhibit a more rapid response to precipitation. Typically, with the increase in precipitation during the early part of the rainy season, these deformation points experience a significant increase in subsidence. The primary reason for this behavior is that the surface coverage in these deformation points mainly consists of shrubs and grassland. Compared to large trees (such as fir and pine trees), the surfaces with shrubs and grassland are more vulnerable to the impact and erosion of heavy rain, leading to soil loosening and collapse, and consequently accelerating surface deformation.
To establish the correlation between surface deformation at different elevations and precipitation and vegetation coverage, an additional set of deformation points was selected for validation. The selection criteria for these points remained consistent, with efforts made to avoid areas influenced by human activities, such as farmland and urban regions. In Figure 7, deformation points g-l were chosen, exhibiting elevations that incrementally increased by approximately 100 m, ranging from 1591 m to 2011 m. With the exception of deformation points I and j, which possessed a slope of 28°, the slope of the remaining deformation points exhibited minimal variation, falling within the range of 12°–22°. Figure 7 illustrates a comparable pattern among deformation points g-l and deformation points a-f in Figure 6. Overall, all deformation points displayed a fluctuating downward trend, with the subsidence values systematically influenced by precipitation. Notably, the subsidence rate and magnitude of deformation points notably escalated during the rainy season. The diverse types of vegetation coverage observed at the deformation points led to distinct deformation patterns during this period. Deformation points g, k, and l were predominantly characterized by the presence of large trees, such as fir and pine species. These points exhibited a noticeable delay in subsidence, typically occurring 2–3 months after the onset of the rainy season. Conversely, deformation points h and j were primarily covered by shrubs and grasslands, rendering them highly responsive to precipitation. These points often displayed significant subsidence during the initial month of the rainy season. Additionally, deformation point I, despite having tree vegetation, possessed a steep slope of 28.63°. It demonstrated a sensitive response to the rainy season, exhibiting significant subsidence during the early stages of precipitation. This suggests that the terrain’s slope is a contributing factor to surface deformation, with the steepness of the slope influencing the extent to which precipitation impacts deformation points.
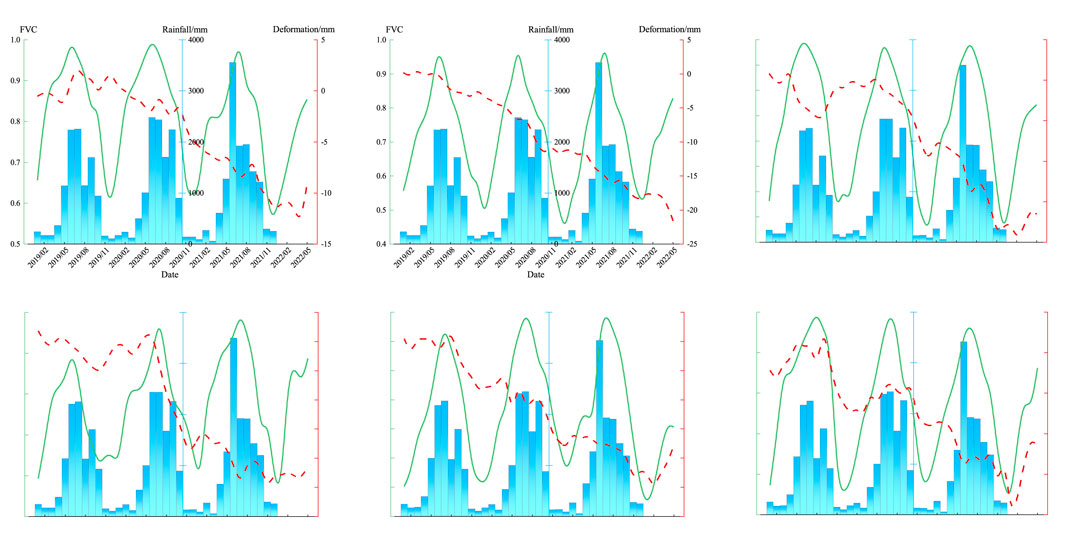
FIGURE 7. Time Series Curves for Deformation Points at Different Elevations. [(G–L) have elevations of 1591 m, 1634 m, 1750 m, 1855 m, 1938 m, and 2011 m, respectively].
Topography and geomorphology play a significant role in governing slope stability, with slope gradient serving as a crucial parameter for characterizing the terrain (Guo et al., 2008). To examine the deformation patterns of deformation points at different slopes and their response to precipitation, we extracted time series cumulative subsidence data, vegetation cover data, and monthly cumulative precipitation data from two distinct sets of deformation feature points. To reduce the effect of different vegetation types on precipitation data, we selected deformation feature points that all exhibited woody vegetation cover.
As depicted in Figure 8, time series curves were generated for deformation points at different slope levels (<5°, 5°–15°, 15°–25°, 25°–35°, 35°–45°, >45°). The slope angles for points in Figures 8M–R increased progressively, ranging from 4.38° to 57.76°. In general, all deformation points exhibited a fluctuating subsidence trend, demonstrating a distinct response to variations in precipitation. During the rainy season, deformation points are subjected to significant subsidence, while they will remain relatively stable during the dry season. With the increase of the slope gradient, subsidence rates will accelerate. Notably, deformation points in Figures 8M, N, O do not show significant subsidence at the early stages of the rainy season, even under intense precipitation in June 2021. Instead, they exhibit delayed subsidence, typically occurring 2–3 months after the onset of the rainy season. In contrast, deformation points in Figures 8P, Q, R responded rapidly to increased precipitation during the early stages of the rainy season, displaying noticeable subsidence. These results suggested that with the increase of slope gradient, deformation points will become more sensitive to precipitation. Specifically, when the slope gradient was less than 25°, deformation points were less affected by precipitation, and their response time may be delayed, with significant subsidence occurring 2–3 months after the rainy season starts. Nevertheless, when the slope gradient was greater than 25°, deformation points responded more quickly to precipitation, typically showing substantial subsidence at the beginning of the rainy season.
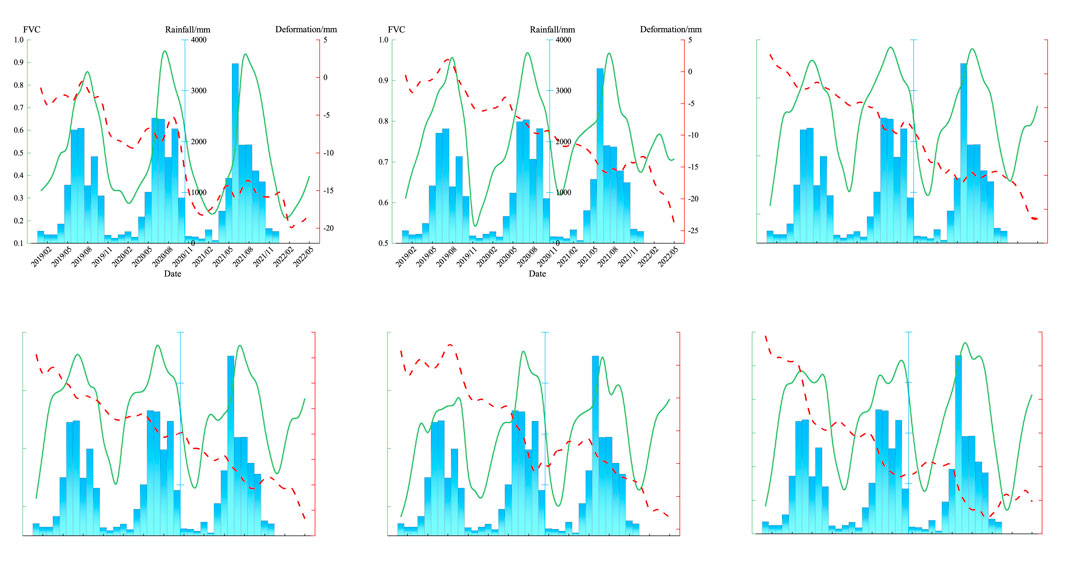
FIGURE 8. Time Series Curves of Deformation Points at Different. [(M–R) have slopes of 4.38°, 10.54°, 21.53°, 29.69°, 37.68°, and 57.76°, respectively].
The topographic characteristics of the study area, characterized by elevated mountains and steep slopes, exert a profound influence on slope stability. The gradient of the slope not only impacts the distribution of stress within the slope but also plays a pivotal role in determining crucial factors such as surface water runoff, the distribution of loose deposits across the slope, and the thickness of such deposits. Steeper slopes experience more significant stress distribution across their surfaces and upper sections, rendering them more susceptible to deformation and failure. Furthermore, during the rainy season, steeper slopes tend to accumulate surface runoff, and the steep gradient amplifies the velocity of the runoff. This phenomenon intensifies erosion and scouring of the slope surface, exacerbating soil erosion and facilitating soil loosening and collapse. In such circumstances, surface deformation becomes more probable, thereby increasing the occurrence of geological hazards.
To verify the deformation patterns and their response to precipitation at different slopes, we selected another set of deformation points with varying slopes. The slopes of these points, as presented in Figure 9, were 3.58°, 12.31°, 21.05°, 32.58°, 35.93°, and 49.33°, and all of them had woody vegetation cover. As depicted in Figures 9S–X, these points displayed a similar pattern to those in Figure 8. Overall, with an increase in slope, the deformation points showed an accelerating sinking trend. The response of the deformation points to precipitation was quite apparent, with significant sinking occurring during the rainy season. Among these points, a slope of 25° appeared to be a threshold for the speed of response to precipitation. Specifically, when the slope was less than 25°, the deformation points exhibited a delayed response to precipitation, typically showing significant sinking 2–3 months after the rainy season begins. On the other hand, when the slope exceeded 25°, the deformation points responded very rapidly, with significant sinking occurring at the start of the rainy season.
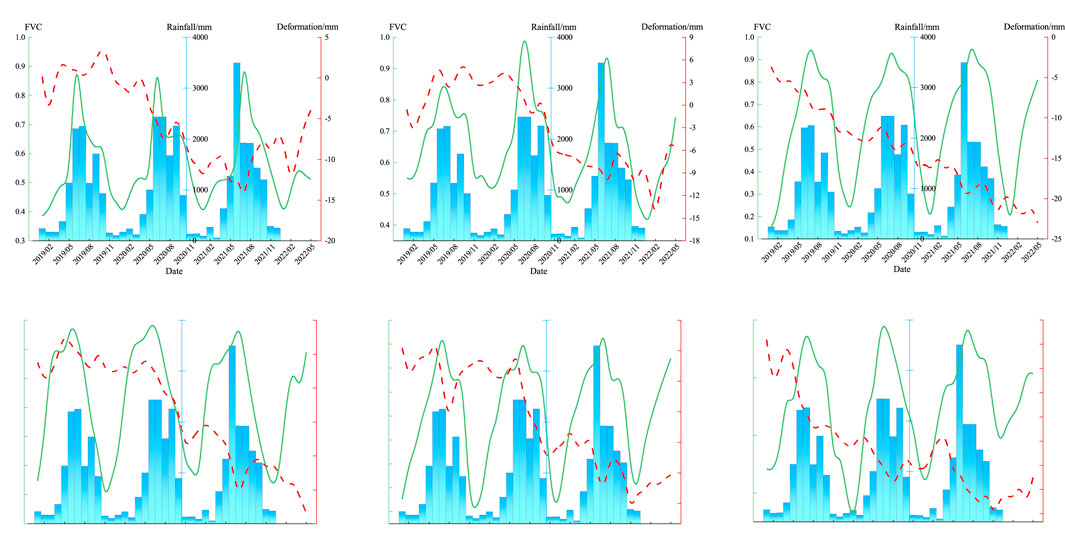
FIGURE 9. Time Series Curves for Deformation Points at Different Slopes. [(S–X) have slopes of 3.58°, 12.31°, 21.05°, 32.58°, 35.93°, and 49.33°, respectively].
5 Discussion
5.1 Analysis of surface deformation in karstic mountainous regions as affected by subsidence of closed mines
Following the cessation of mining activities, the persistence of ground subsidence, characterized by collapsed rock masses within goaf regions, remains a prominent contributor to land subsidence in the area. Within the study area, which represents a typical karst complex mountainous region, comprehending the implications of subsidence induced by mine closures on surface deformation is of utmost importance. Currently, there exists a dearth of research concerning the distribution patterns and impact models of surface deformation resulting from mine closure within this specific study area. Hence, our discourse is primarily centered around this representative study area.
In their investigation on surface deformation resulting from mine closures, Chen et al. (2020) explored the characteristics of surface deformation in several abandoned goaf areas at Ying’an Coal Mine and Baoshan Coal Mine, situated in the alluvial plains of Jilin, China. Their findings revealed that the subsidence process subsequent to coal mining can be divided into two distinct stages: the initial stage primarily entails subsidence in the central region of the goaf, while the subsequent stage witnesses subsidence predominantly concentrated in the peripheral areas of the goaf. The distribution patterns of surface deformation arising from mine closures in this study exhibit consistency with the observations made by Chen et al. However, it is crucial to recognize that the study area under consideration represents a typical karst complex mountainous region characterized by robust karst processes, thereby rendering the impact of mine closure on ground subsidence more intricate than in plain areas.
Surface deformation in the LuJiaZhai-DaPingDi Minefield extends beyond the confines of the closed mining areas, predominantly impacting high-altitude regions adjacent to the closed mining areas. This phenomenon can be attributed to multiple factors. Coal mining operations typically disrupt the initial stress state of overlying rock layers, resulting in their redistribution and consequent movements and deformations. This process generates various voids, encompassing fractures between rocks, cracks within overlying rock layers, and fissures within loose sedimentary materials (Wang et al., 2016). The study area exhibits significant elevation variation, characterized by a “hard on top and soft underneath” pattern in the rock layers. Coal seams are situated within the Longtan Formation (P3l) at the base of the mountains, while the mining goaf is located at the foothills. During the initial phases of coal mining, the central portion of the goaf, providing the weakest support to the overlying rock layers, experiences substantial early movements and deformations concentrated in its central region. As the overlying rock layers subside, the progressive deformation gradually extends upward, resulting in surface subsidence and the formation of numerous fractures within the mountains. With time, the central part of the mining goaf undergoes compaction first. At this stage, the central portion of the goaf reaches a relatively stable state, leading to a decline in surface deformation. However, in the boundary regions of the goaf, there remains space that requires compaction. Consequently, during the later stages following mine closure, subsidence primarily concentrates in the border areas of the goaf.
Preceding the coal mining activities in the karst mountain regions, the area was already influenced by tectonic movements, resulting in fractured rock formations with well-developed joint fissures. Under the influence of precipitation and weathering, the existing cracks had already manifested in the mountainous terrain. As coal mining operations progressed, these initial cracks further widened, and new cracks emerged (Sun et al., 2023). These mountain cracks not only compromised the stability of the terrain but also provided pathways for rainwater infiltration into the rock formations, thereby accelerating the karstification process. Consequently, this gave rise to karst subsidence occurrences within the area.
5.2 Analysis of the coupled relationship between surface deformation of closed mines in karst mountainous areas and factors such as slope, precipitation, and vegetation types
Our experimental results indicate that besides the goaf, slope is the primary factor causing surface deformation in closed mines in karst mountainous areas, with precipitation acting as a triggering factor leading to periodic changes in surface deformation in the study area. When the slope is less than 25°, arboreal coverage (such as pine and fir trees) significantly mitigates surface deformation induced by rainfall. However, this mitigating effect rapidly diminishes when the slope exceeds 25°. To further analyze the impact of slope on surface subsidence in the Karst mountain areas of Liupanshui and its response to precipitation and vegetation coverage, we have compared our findings with previously published similar studies, outlining the complementary aspects of our research and identifying any existing limitations.
Contemporary investigations concerning the impact of slope, precipitation, and vegetation coverage on surface deformation primarily concentrate on landslide causation and susceptibility assessments. For example, Zhang et al. (Zhang et al., 2020) examined the spatial distribution characteristics of landslide-prone regions in Xiangxi Autonomous Prefecture, Hunan Province, China, based on diverse geographical factors. Their study underscored the significance of slope and vegetation coverage in landslide occurrence. Among the 21 geographical factors examined, slope emerged as the most influential contributor to landslides. Furthermore, the distribution of landslide-prone areas exhibited a close association with vegetation coverage. Specifically, regions with higher Normalized Difference Vegetation Index (NDVI) values displayed fewer landslides, indicating that areas with lower NDVI values possessed weaker vegetation stability and were more susceptible to landslides. Bao and You (2010), through field surveys and an analysis of geological hazard-prone locations in the Zhongshan area of Liupanshui, investigated the characteristics and causes of geological disasters. Their findings revealed that landslides predominantly transpired in areas with slopes ranging from 10° to 65°, with the majority falling within the 25°–35° range. Additionally, the peak period for geological disasters was from May to September, corresponding to heightened precipitation and heavy precipitation. Similarly, Qian et al. (2022) conducted a susceptibility analysis of landslides in Liupanshui, employing various geographical factors and a Logistic fuzzy comprehensive coupling model on a Geographic Information System (GIS) platform. Their results indicated that areas at extremely high and high risk of landslides were considerably influenced by slope and surface undulation. These researchers generally evaluated the contribution of influencing factors to landslide occurrence at a general level, typically relying on known geological hazard-prone locations and employing statistical or modeling approaches. Our study aligns with these trends in a general sense. However, our research distinguishes itself by utilizing SBAS technique to obtain time series deformation monitoring results, enabling a more detailed assessment of the contributions of different slope levels, vegetation types, and precipitation to surface subsidence in closed mining areas. In recent studies, Asada et al. (2020) emphasized the importance of precipitation as a significant factor compared to vegetation, attributing approximately 40% of the likelihood of shallow landslides to it. Their research also highlighted the effective mitigation of shallow landslides by vegetation under specific conditions. In their more recent study (Asada and Minagawa, 2023), they constructed generalized linear models (GLM) and random forest models (RF) using statistical methods to quantitatively assess the impact of different vegetation conditions on shallow landslide occurrence. The models were developed considering slope and hourly precipitation as critical parameters for evaluating slope stability. The study found that forest-covered slopes exhibited greater stability compared to grass-covered slopes, and secondary grasslands and shrubs were more prone to landslides than coniferous forests. However, it was observed that the slope stability of forested areas had its limitations. Our study exhibits a similar trend to Asada’s research. Specifically, under comparable slope conditions, areas with grassland and shrub coverage display heightened sensitivity to precipitation, while areas with forest coverage exhibit a certain degree of delayed response. Moreover, when vegetation primarily consists of trees, slope becomes a pivotal factor influencing the response to precipitation. Specifically, when the slope is less than 25°, tree-covered surfaces exhibit a noticeable delay in response to precipitation. Conversely, when the slope exceeds 25°, the response to precipitation is rapid. Our research provides an analysis of the contributions of slope, vegetation type, and precipitation to surface subsidence in closed mining areas based on time series deformation results. However, numerous factors influence surface deformation in karst mountain areas with closed mines. Consequently, undertaking a quantitative exploration of the contributions of multiple factors to surface deformation in closed mining areas using Interferometric Synthetic Aperture Radar (InSAR) technology represents a crucial focus of our future research.
In addition, in order to further verify the reliability of the deformation extraction results in this study, we went to the LuJiaZhai-DaPingDi Minefield to conduct a field investigation, and the results of the field investigation are shown in Figure 10, from which it can be clearly seen that the slopes with steeper slopes under the cover of shrubs have been sliding obviously (Figures 10D, F) and the sliding surfaces are fresh, and meanwhile, a number of highways in the wellfield have been damaged due to the ground settlement (Figures 10A–C, E), which suggests that surface subsidence has continued to take place in the area even though all the mines in the wellfield are closed to the public so far.
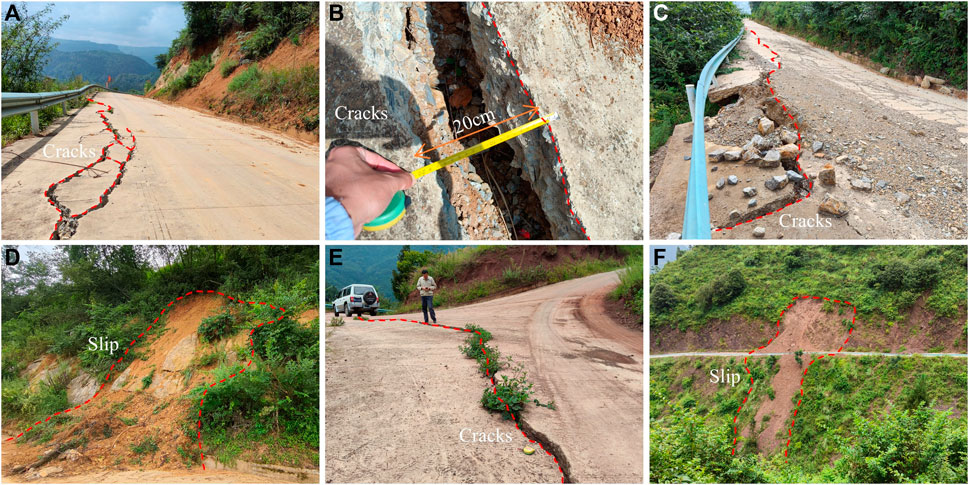
FIGURE 10. Photographs of LuJiaZhai-DaPingDi Minefield survey: (D) and (F) depict pronounced sliding on steep slopes under bush cover; (A), (B), (C) and (E) illustrate road damage caused by subsidence.
6 Conclusion
In the context of the intricate interplay between surface deformation, precipitation, and vegetation cover in karst mountain regions, this study focuses on the LuJiaZhai-DaPingDi Minefield situated in Liupanshui City. Leveraging remote sensing technology, we investigate the influence of various factors, including altitude, slope, vegetation cover, and precipitation, on surface deformation within closed mining areas. An extensive analysis was performed utilizing time series data of surface deformation, vegetation cover, precipitation, altitude, and slope. The key findings are as follows:
The areas exhibiting surface deformation within the study region are primarily concentrated near the recently closed MaiZiGou Coal Mine and the higher-altitude eastern and southern regions of the mining field. These deformation areas comprise 20.45% of the total mining area. Over the study period, the maximum cumulative subsidence reaches −60.3 mm, while the maximum uplift is 34.17 mm. The maximum annual average subsidence rate and uplift rate are −21.83 mm/yr and 8.13 mm/yr, respectively. Overall, surface deformation in the closed mining area displays systematic variations with precipitation. During the rainy season, both the subsidence rate and subsidence values in the mining area are significantly higher compared to the dry season. Furthermore, distinct types of vegetation cover exert varying degrees of influence on surface deformation. Surfaces covered by grassland and shrubs exhibit a more rapid response to precipitation compared to areas adorned with deciduous trees such as fir and pine. Typically, substantial subsidence occurs promptly during the initial stages of the rainy season or periods of heavy precipitation on grassland and shrub-covered surfaces. However, when the surface is enveloped by deciduous trees, even in the presence of the rainy season or heavy precipitation, surface subsidence does not manifest immediately. Instead, a delayed response ensues, typically lagging behind precipitation by 2–3 months. In regions characterized by deciduous tree vegetation cover, the response of surface deformation to precipitation is primarily influenced by the terrain slope. When the slope is below 25°, surfaces covered by deciduous trees do not display significant subsidence in the early stages of the rainy season or heavy precipitation, with the response typically lagging behind the rainy season by 2–3 months. Conversely, when the slope exceeds 25°, substantial subsidence occurs early in the rainy season or during heavy precipitation, even if the surface is covered by deciduous trees. This indicates that a slope of 25° represents a critical threshold for surface deformation in response to precipitation within this region. Moreover, deciduous trees such as fir and pine exhibit a certain degree of restraining effect on surface subsidence triggered by precipitation. However, this restraining effect is limited and diminishes as the slope increases.
Data availability statement
The original contributions presented in the study are included in the article/Supplementary Material, further inquiries can be directed to the corresponding authors.
Author contributions
GH: Conceptualization, Methodology, Project administration, Writing–original draft, Writing–review and editing. JD: Software, Writing–review and editing. WX: Conceptualization, Validation, Writing–review and editing. ZZ: Conceptualization, Writing–review and editing. SL: Validation, Writing–review and editing. ZK: Investigation, Validation, Writing–review and editing. QA: Formal Analysis, Writing–review and editing. JW: Investigation, Writing–review and editing. YZ: Software, Writing–review and editing.
Funding
The author(s) declare financial support was received for the research, authorship, and/or publication of this article. This research was funded by Guizhou Scientific and Technology Fund [Grant No. QKHJ-ZK (2023) YB 193], Major scientific and technological projects of Yunnan Province: Research on Key Technologies of ecological environment monitoring and intelligent management of natural resources in Yunnan (Grant No. 202202AD080010), Geological Research Project of Bureau of Geology and Mineral Exploration and Development Guizhou Province [Grant No. QDKKH-(2021)33] and High-resolution Comprehensive Application Demonstration for the Central Yunnan Water Diversion Project (in the Mid-Yunnan Plateau Area) (89-Y50-G31-9001-22/23-05).
Acknowledgments
The authors would like to thank the reviewers for their constructive comments that improved this research.
Conflict of interest
Author ZZ was employed by Yunnan Haiju Geographic Information Technology Co., Ltd.
The remaining authors declare that the research was conducted in the absence of any commercial or financial relationships that could be construed as a potential conflict of interest.
Publisher’s note
All claims expressed in this article are solely those of the authors and do not necessarily represent those of their affiliated organizations, or those of the publisher, the editors and the reviewers. Any product that may be evaluated in this article, or claim that may be made by its manufacturer, is not guaranteed or endorsed by the publisher.
References
Adams, J. B., Smith, M. O., and Johnson, P. E. (1986). Spectral mixture modeling: a new analysis of rock and soil types at the Viking Lander 1 Site. J. Geophys. Res. Solid Earth 91 (B8), 8098–8112. doi:10.1029/JB091iB08p08098
Asada, H., and Minagawa, T. (2023). Impact of vegetation differences on shallow landslides: a case study in Aso, Japan. Water 15 (18), 3193. doi:10.3390/w15183193
Asada, H., Minagawa, T., Koyama, A., and Ichiyanagi, H. (2020). Factor analysis of surface collapse on slopes caused by the july 2017 northern kyushu heavy rain. Ecol. Civ. Eng. 23 (1), 185–196. doi:10.3825/ece.23.185
Bai, J., Ju, N., Zhang, C., and He, Z. (2020). Characteristics and successful early warning case of Xingyi landslide in Guizhou province. J. Eng. Geol. 28 (6), 1246–1258. doi:10.13544/j.cnki.jeg.2019-360
Bao, D., and You, G. (2010). The characteristics of geological hazards and analysis on genesis in zhongshan district of Liupanshui City,Guizhou province. Mineral. Explor. 1 (4), 400–406. doi:10.3969/j.issn.1674-7801.2010.04.016
Berardino, P., Fornaro, G., Lanari, R., and Member, S. (2002). A new algorithm for surface deformation monitoring based on small baseline differential SAR interferograms. IEEE Trans. Geoscience Remote Sens. 40 (11), 2375–2383. doi:10.1109/TGRS.2002.803792
Chen, B., Liu, H., Li, Z., and Zheng, M. (2023a). Research progress and prospect of secondary subsidence monitoring, prediction and stability evaluation in closed underground mines. J. China Coal Soc. 48 (2), 943–958. doi:10.13225/j.cnki.jccs.2022.1385
Chen, B., Yu, H., Zhang, X., Li, Z., Kang, J., Yu, Y., et al. (2022a). Time-varying surface deformation retrieval and prediction in closed mines through integration of SBAS InSAR measurements and LSTM algorithm. Remote Sens. 14(3), 788. doi:10.3390/rs14030788
Chen, D., Chen, H., Zhang, W., Cao, C., Zhu, K., Yuan, X., et al. (2020). Characteristics of the residual surface deformation of multiple abandoned mined-out areas based on a field investigation and SBAS-InSAR: a case study in Jilin, China. Remote Sens. 12(22), 3752. doi:10.3390/rs12223752
Chen, H., Zhao, C., Li, B., Gao, Y., Chen, L., and Liu, D. (2023b). Monitoring spatiotemporal evolution of Kaiyang landslides induced by phosphate mining using distributed scatterers InSAR technique. Landslides 20 (3), 695–706. doi:10.1007/s10346-022-01986-5
Chen, H., Zhao, C., Sun, R., Chen, L., Wang, B., and Li, B. (2022b). Two-dimensional deformation monitoring of karst landslides in Zongling, China, with multi-platform distributed scatterer InSAR technique. Landslides 19 (7), 1767–1777. doi:10.1007/s10346-022-01860-4
Chen, L., Zhao, C., Chen, H., Kang, Y., Li, B., and Liu, X. (2023c). The detection and control factor analysis of active landslides in Guizhou province, China, using sentinel-1 SAR imagery. Remote Sens. 15 (23), 5468. doi:10.3390/rs15235468
Chen, Z., Yang, Y., Zhou, L., Hou, H., Zhou, W., Liu, S., et al. (2022c). Ecological restoration of a loess open-cast mining area in China: perspective from an ecological security pattern. Forests 13(2), 269. doi:10.3390/f13020269
Chu, X., Dang, S., and Ding, J. (2015). Analysis of karst collapse distribution in Guizhou Province and influential factors. Yangtze River 46 (12), 42–44. doi:10.16232/j.cnki.1001-4179.2015.12.010
Deng, K., Wang, L., and Fan, H. (2015). InSAR-based monitoring and analysis of ground subsidence in old goaf. J. Min. Saf. Eng. 32 (6), 918–922. doi:10.13545/j.cnki.jmse.2015.06.008
Deng, K., Zheng, M., Zhang, H., Fan, H., and Tan, Z. (2022). Research status and prospect of secondary subsidence in closed mine. Coal Sci. Technol. 50 (5), 10–20. doi:10.13199/J.CNKI.CST.2021-1403
Dong, J., Ma, Z., Liang, J., and Liu, B. (2022). A comparative study of the identification of hidden landslide hazards based on time series InSAR techniques. Remote Sens. Nat. Resour. 34 (3), 73–81. doi:10.6046/zrzyyg.2021333
Du, Y., Zhang, L., Feng, G., Lu, Z., and Sun, Q. (2017). On the accuracy of topographic residuals retrieved by MTInSAR. IEEE Trans. Geoscience Remote Sens. 55 (2), 1053–1065. doi:10.1109/TGRS.2016.2618942
Fang, J., Xie, X., and Liu, J. (2016). Study on causes and countermeasures of geological disasters in panxian Guizhou province. Coal Technol. 35 (12), 188–189. doi:10.13301/j.cnki.ct.2016.12.070
Guo, F., Yang, N., Meng, H., and Zhang, Y. (2008). Application of the relief amplitude and slope analysis to regional landslide hazard assessments. Geol. China 35 (1), 131–143. doi:10.3969/j.issn.1000-3657.2008.01.014
He, K., Li, J., Li, B., Zhao, Z., Zhao, C., Gao, Y., et al. (2022). The Pingdi landslide in Shuicheng, Guizhou, China: instability process and initiation mechanism. Bull. Eng. Geol. Environ. 81 (4), 131. doi:10.1007/s10064-022-02596-0
Hu, B., and Yan, B. (2018). Study on potential geological disasters and prevention technology and resources utility of abandon mine. Coal Min. Technol. 23 (3), 1–5. doi:10.13532/j.cnki.cn11-3677/td.2018.03.001
Jacquemart, M., and Tiampo, K. F. (2021). Leveraging time series analysis of radar coherence and normalized difference vegetation index ratios to characterize pre-failure activity of the Mud Creek landslide, California. Nat. Hazards Earth Syst. Sci. 21 (2), 629–642. doi:10.5194/nhess-21-629-2021
Li, B., Zhao, C., Li, J., Chen, H., Gao, Y., Cui, F., et al. (2023). Mechanism of mining-induced landslides in the karst mountains of Southwestern China: a case study of the Baiyan landslide in Guizhou. Landslides 20 (7), 1481–1495. doi:10.1007/s10346-023-02047-1
Li, J., Gao, F., Lu, J., and Fang, R. (2019). Deformation monitoring and predicton of residential areas based on SBAS-InSAR and GM-SVR. J. Geodesy Geodyn. 38 (08), 837–842. doi:10.14075/j.jgg.2019.08.014
Li, T., Wu, M., Duan, C., Li, S., and Liu, C. (2022). The effect of different restoration approaches on vegetation development in metal mines. Sci. total Environ. 806 (2), 150626. doi:10.1016/j.scitotenv.2021.150626
Li, X., Zhuo, L., Su, F., and Wu, W. (2021). Application of InSAR technology in landslide hazard: progress and prospects. Natl. Remote Sens. Bull. 25 (2), 614–629. 1007-4619(2021) 02-0614-16. doi:10.11834/jrs.20209297
Li, Y., Zhang, J., Li, z., and Luo, Y. (2013). Land subsidence in Beijing city from InSAR time series analysis with small baseline subset. Geomatics Inf. Sci. Wuhan Univ. 38 (11), 1374–1377. doi:10.13203/j.whugis2013.11.011
Liang, S., and Hu, H. (2022). Analysis of residual deformation in goaf based on SBAS InSAR technology. China Min. Mag. 31 (12), 70–78. doi:10.12075/j.issn.1004-4051.2022.12.002
Liu, Z., Qiu, H., Zhu, Y., Liu, Y., Yang, D., Ma, S., et al. (2022). Efficient identification and monitoring of landslides by time-series InSAR combining Single- and multi-look phases. Remote Sens. 14 (4), 1026. doi:10.3390/rs14041026
Ma, S., Qiu, H., Zhu, Y., Yang, D., Tang, B., Wang, D., et al. (2023). Topographic changes, surface deformation and movement process before, during and after a rotational landslide. Remote Sens. 15 (3), 662. doi:10.3390/rs15030662
Pei, Y., Qiu, H., Yang, D., Liu, Z., Ma, S., Li, J., et al. (2023). Increasing landslide activity in the Taxkorgan River Basin (eastern Pamirs Plateau, China) driven by climate change. CATENA 223, 106911. doi:10.1016/j.catena.2023.106911
Qian, C., Wang, J., and Wu, W. (2022). Analysis of landslide geological hazard susceptibility based on GIS: a case study of Liupanshui City. J. Tianjin Chengjian Univ. 28 (5), 336–341. doi:10.19479/j.2095-719x.2205336
Qin, X., Deng, K., Zheng, M., and Wang, L. (2022). Monitoring and analysis of surface secondary subsidence of closed mines in Western Xuzhou. Sci. Surv. Mapp. 47 (8). doi:10.16251/j.cnki.1009-2307.2022.08.028
Sandwell, D. T., and Price, E. J. (1998). Phase gradient approach to stacking interferograms. J. Geophys. Res. Solid Earth 103 (B12), 30183–30204. doi:10.1029/1998JB900008
Shi, X., and Zhang, W. (2023). Characteristics of an underground stope channel supplied by atmospheric precipitation and its water disaster prevention in the karst mining areas of Guizhou. Sci. Rep. 13 (1), 15892. doi:10.1038/s41598-023-43209-4
Sun, R., Zhao, C., Li, B., Chen, H., and Chen, L. (2023). Karst landslides detection and monitoring with multiple SAR data and multi-dimensional SBAS technique in Shuicheng, Guizhou, China. Front. Earth Sci. 11, 1121868. doi:10.3389/feart.2023.1121868
Tang, L., Hou, H., Zhang, S., and Guo, S. (2021). Monitoring of short revisited SAR data in rapid deformation field of mining area. Sci. Surv. Mapp. 46 (5), 95–103. doi:10.16251/j.cnki.1009-2307.2021.05.014
Wang, J., Wang, C., Xie, C., Zhang, H., Tang, Y., Zhang, Z., et al. (2020). Monitoring of large-scale landslides in Zongling, Guizhou, China, with improved distributed scatterer interferometric SAR time series methods. Landslides 17 (8), 1777–1795. doi:10.1007/s10346-020-01407-5
Wang, L. (2013). Application of precision leveling in land subsidence monitoring. Huabei Land Resour. (2), 113–116. doi:10.3969/j.issn.1672-7487.2013.02.036
Wang, L., Qiu, H., Zhou, W., Zhu, Y., Liu, Z., Ma, S., et al. (2022). The post-failure spatiotemporal deformation of certain translational landslides may follow the pre-failure pattern. Remote Sens. 14 (10), 2333. doi:10.3390/rs14102333
Wang, S., Li, X., and Wang, D. (2016). Void fraction distribution in overburden disturbed by longwall mining of coal. Environ. Earth Sci. 75, 151. doi:10.1007/s12665-015-4958-6
Wu, L., Wang, J., and Fu, Y. (2021). Early identifying and monitoring landslides in Guizhou province with InSAR and optical remote sensing. Bull. Surv. Mapp. 7, 98–102. doi:10.13474/j.cnki.11-2246.2021.0216
Xiao, L., He, Y., Xin, X., Wen, D., Tong, C., Chen, L., et al. (2019). Time series subsidence analysis of drilling solution miningrock salt mines based on Sentinel-1 data and SBAS-InSAR technique. J. Remote Sens. 23 (3), 501–513. doi:10.11834/jrs.20198292
Xu, Y., Li, T., Tang, X., Zhang, X., Fan, H., and Wang, Y. (2022). Research on the applicability of DInSAR, stacking-InSAR and SBAS-InSAR for mining region subsidence detection in the Datong Coalfield. Remote Sens. 14(14), 3314. doi:10.3390/rs14143314
Yang, C., Dong, J., Zhu, S., and Xiong, G. (2021). Detection, identification and deformation characteristics of landslide groups by InSAR in Batang section of Jinsha River Convergence Zone, China. J. Earth Sci. Environ. 43 (02), 398–408. doi:10.19814/j.jese.2020.12034
Yang, Z., Li, Z., Zhu, J., Wang, Y., and Wu, L. (2020). Use of SAR/InSAR in mining deformation monitoring, parameter inversion, and forward predictions: a review. IEEE Geoscience Remote Sens. Mag. 8 (1), 71–90. doi:10.1109/MGRS.2019.2954824
Yu, H., Chen, B., Kang, J., and Han, M. (2021). Research on surface deformation law of closed mines based on SBAS-InSAR. Industry Mine Automation 47 (2), 45–51. doi:10.13272/j.issn.1671-251x.2020110044
Zhang, F., Zhu, Y., Zhao, X., and Zhang, Y. (2020). Spatial distribution and identification of hidden danger points of landslides based on geographical factors. Geomatics Inf. Sci. Wuhan Univ. 45 (8), 1233–1244. doi:10.13203/j.whugis20200126
Zhang, L., Dai, K., Deng, J., Ge, D., Liang, R., Li, W., et al. (2021). Identifying potential landslides by stacking-InSAR in southwestern China and its performance comparison with SBAS-InSAR. Remote Sens. 13 (18), 3662. doi:10.3390/rs13183662
Zhang, L., Liang, L., Chen, B., Hu, J., and Yu, Y. (2023). Spatio-temporal monitoring and analysis method of MIulti-dimensional surface deformation of closed mines based on SBAS-InSAR technique. Metal. Mine 1, 83–94. doi:10.13272/j.issn.1671-251x.2020110044
Zhang, X., Zhao, X., Ge, D., and Liu, B. (2018). Subsidence monitoring of Huainan coal mine from Sentinel TOPS images based on Stacking technique. Remote Sens. Land Resour. 30 (4), 200–205. doi:10.6046/gtzyyg.2018.04.30
Zhang, Y., Wang, Y., and Yan, S. (2016). Ground subsidence detection of Peibei mining area based on Stacking InSAR technology. Coal Technol. 35 (07), 102–105. doi:10.13301/j.cnki.ct.2016.07.042
Zhao, C., Chen, L., Yin, Y., Liu, X., Li, B., Ren, C., et al. (2023a). Failure process and three-dimensional motions of mining-induced Jianshanying landslide in China observed by optical, LiDAR and SAR datasets. GIScience Remote Sens. 60 (1), 2268367. doi:10.1080/15481603.2023.2268367
Zhao, Q., Yang, Z., Jiang, Y., Liu, X., Cui, F., and Li, B. (2023b). Discrete element analysis of deformation features of slope controlled by karst fissures under the mining effect: a case study of Pusa landslide, China. Geomatics, Nat. Hazards Risk 14 (1), 1–35. doi:10.1080/19475705.2022.2158376
Zhao, X., and Zhu, Q. (2020). Analysis of the surface subsidence induced by sublevel caving based on GPS monitoring and numerical simulation. Nat. Hazards 103 (3), 3063–3083. doi:10.1007/s11069-020-04119-0
Zhong, Z., Xu, Y., Wang, N., Liu, X., and Gao, G. (2022). Environmental characteristics and unified failure mode classification system for mining landslides in the karst mountainous areas of southwestern China. Carbonates Evaporites 38 (1), 2. doi:10.1007/s13146-022-00818-w
Zhu, J., Li, Z., and Hu, J. (2017). Research progress and methods of InSAR for deformation monitoring. Acta Geod. Cartogr. Sinica 46 (10), 1717–1733. doi:10.11947/j.AGCS.2017.20170350
Zhu, J., Yang, Z., and Li, Z. (2019a). Recent progress in retrieving and predicting mining-induced 3D displacements using InSAR. Acta Geod. Cartogr. Sinica 48 (2), 135–144. doi:10.11947/j.AGCS.2019.20180188
Zhu, Q., Zeng, H., Ding, Y., and Xie, X. (2019b). A review of major potential landslide hazards analysis. Acta Geod. Cartogr. Sinica 48 (12), 1551–1561. doi:10.11947/j.AGCS.2019.20190452
Keywords: SBAS-InSAR, mine closure, karst collapse, deformation monitoring, slope, precipitation, vegetation cover type
Citation: Huang G, Dong J, Xi W, Zhao Z, Li S, Kuang Z, An Q, Wei J and Zhu Y (2024) Study on surface deformation pattern in mine closure area of complex karst mountainous region based on SBAS-InSAR technology. Front. Earth Sci. 11:1353593. doi: 10.3389/feart.2023.1353593
Received: 11 December 2023; Accepted: 29 December 2023;
Published: 11 January 2024.
Edited by:
Haijun Qiu, Northwest University, ChinaReviewed by:
Jie Liu, Henan Polytechnic University, ChinaPan Cao, University of Hertfordshire, United Kingdom
Copyright © 2024 Huang, Dong, Xi, Zhao, Li, Kuang, An, Wei and Zhu. This is an open-access article distributed under the terms of the Creative Commons Attribution License (CC BY). The use, distribution or reproduction in other forums is permitted, provided the original author(s) and the copyright owner(s) are credited and that the original publication in this journal is cited, in accordance with accepted academic practice. No use, distribution or reproduction is permitted which does not comply with these terms.
*Correspondence: Wenfei Xi, d2VuZmVpeGlAeW5udS5lZHUuY24=; Zilong Zhao, MzY0NDI0MDM1QHFxLmNvbQ==