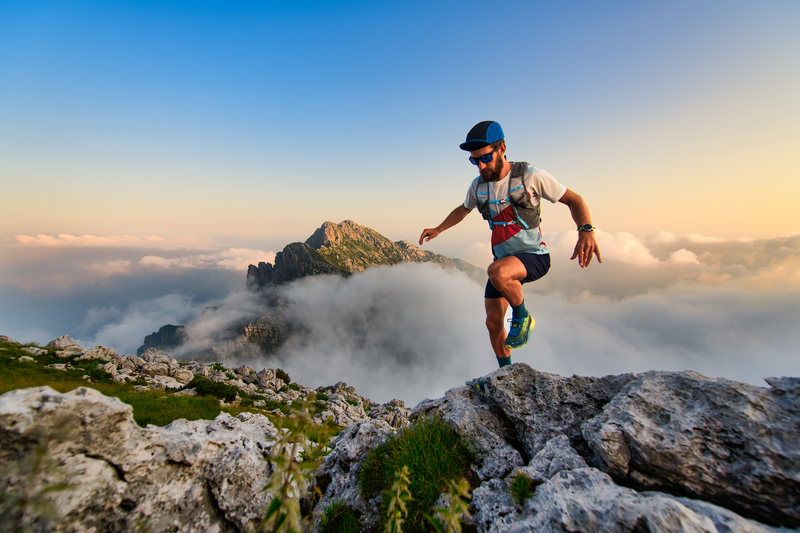
95% of researchers rate our articles as excellent or good
Learn more about the work of our research integrity team to safeguard the quality of each article we publish.
Find out more
ORIGINAL RESEARCH article
Front. Earth Sci. , 10 July 2023
Sec. Solid Earth Geophysics
Volume 11 - 2023 | https://doi.org/10.3389/feart.2023.1144151
This article is part of the Research Topic Earthquake Swarms and Complex Seismic Sequences in Tectonic and Volcanic Areas View all 6 articles
A correction has been applied to this article in:
Corrigendum: The february 2018 seismic swarm in São Miguel, Azores
The island of São Miguel is among the most seismically active areas of the Azores archipelago. This work focuses on the most significant recent swarm, which occurred on February 2018. We set up an automated procedure to process continuous full seismic waveform data from local stations to generate high-quality earthquake information on the volcano unrest episode. First, we applied an automated detector software, next we located the detected events and then classified the earthquakes based on their waveform similarity, identifying three families of seismic events. We then extended the catalog by template matching. Finally, we computed moment tensors to investigate the source mechanisms of the largest earthquakes. Our results image the ∼2-week swarm evolution. The activity started with a precursory phase with low rate and low magnitude (ML < 2.0) seismicity and the activation of a deeper structure (∼10-15 km). After ∼1 week, a new earthquake family emerged at shallower depths (∼8–12 km) reaching magnitudes up to ML 3.4. Finally, a third slightly shallower family was activated. Moment tensors show mostly normal faulting mechanisms, striking ∼NW-SE, compatible with the orientation of the regional stress field. A surface deformation transient was recorded by geodetic stations, starting with the swarm, and continuing over the following ∼17 months, corresponding to either inflation or extension around the swarm region. The prolonged surface deformation implies a process that was initiated during the swarm and subsequently accommodated mostly aseismically. We interpret the seismicity observed at the early stage of deformation as indicating episodic fluid injection through the crust, related to the local hydrothermal or magmatic systems. We conclude that the Fogo-Congro region continues to be seismo-volcanically active, with both seismic and aseismic deformation observed and requiring close multidisciplinary monitoring. The proposed methology based on the automated analysis of continuous waveform data provides high-quality imaging of the spatio-temporal evolution of seismicity, which can be used elsewhere in the operational monitoring of seismo-volcanic crises to gain insight into the ongoing deformation processes, improve hazard assessment and help in the development of effective mitigation strategies.
The Azores archipelago lies in a region of thickened oceanic crust, on the triple junction between the North American, Eurasian, and Nubian plates (Kueppers and Beier, 2016). The region is known as the Azores plateau and extends from the western border of the Mid-Atlantic Ridge (MAR), close to the islands of Corvo and Flores, to Santa Maria Island in the easternmost part of the archipelago (Figure 1A). Seafloor morphology shows a possible boundary between the Nubian and Eurasian plates along a transform fault known as the East Azores Fracture Zone (EAFZ). However, the EAFZ currently does not exhibit seismic activity. Miranda et al. (2015) argued that the EAFZ has not been active since 20 Ma ago. They further argued that the plate boundary deformation is now accommodated in a wide shear zone called the Terceira Rift, located further north of the EAFZ and aligned with the islands of the archipelago. The Terceira Rift is marked by an alignment of basins and volcanic highs that runs from the Western Graciosa Basin to the Formigas Islets, located at the eastern edge of the Gloria Fault. The Terceira Rift currently concentrates most of the seismic activity of the Azores, accommodating slow transtensional deformation at a rate of approximately 5 mm/yr (Miranda et al., 2014; Miranda et al., 2015; Carmo et al., 2015; Madeira et al., 2015). Focal mechanisms of earthquakes along the Terceira Rift show a mix of normal and strike-slip earthquakes, with the extensional T axes consistently oriented NE-SW, perpendicular to the ridge (Ekström et al., 2012, Custódio et al., 2016).
FIGURE 1. (A) Location of the Azores Archipelago, in the triple junction between the North American, Eurasian and Nubian plates. Earthquake locations are shown according to the ISC catalog from 1995 to 2021 (brown dots). The currently inactive East Azores Fracture Zone (EAFZ) is shown by a dotted line and the rectangle identifies the Azores archipelago region, shown in (B). (B) Earthquake epicenters (brown dots) and focal mechanism, sized by magnitude, indicating a dominantly transtensional regime from the Global CMT catalog (Ekström et al., 2012). Colors indicate the type of faulting, from reverse (blue) through strike-slip (red) to normal (green), according to the Kaverina classification (Kaverina et al., 1996), as implemented in FMC (Álvarez-Gómez, 2019). The three events with magnitude Mw > 6 mentioned in the text are identified by their dates. The rectangle identifies the S. Miguel region, shown in (C). (C) Earthquake epicenters in S. Miguel colored according to time of occurrence, circle size indicates magnitude. S. Miguel Digital Elevation Model (DEM) was generated from a 1:5,000 scale digital altimetric database from the Secretaria Regional do Turismo e Transportes of the Azores Government. It is also shown the locations of the volcano-tectonic features identified in Carmo et al. (2015): (SCV) Sete Cidades Volcano; (PFVS) Picos Fissural Volcanic System; (FGV) Fogo Volcano; (CFVS) Congro Fissural Volcanic System; (FNV) Furnas Volcano; (PV) Povoação Volcano; (NVS) Nordeste Volcanic System.
The source of the long-term volcanism of the Azores is still a matter of discussion. Previous studies suggested that the volcanism results from a ridge-hotspot interaction (Silveira et al., 2006; Adam et al., 2013; Saki et al., 2015; O’Neill and Sigloch, 2018). Despite the ambiguity on the nature of a possible underlying mantle plume, there is evidence for such ridge-hotspot interaction, namely, the elevated spreading ridge rate, the basalt geochemistry, and the presence of a gravity anomaly (Silveira et al., 2006; Miranda et al., 2014; Miranda et al., 2015). Silveira et al. (2006) presented a surface wave regional tomography that showed a strong broad negative seismic-velocity anomaly, clearly visible at 100 km depth which vanishes below 250–300 km depth. They interpreted this anomaly as suggestive of the presence of an elongated dying plume associated with the creation of the Azores plateau and the Terceira rift 20 Ma ago. This mantle anomaly has also been associated with both intrusive and extrusive magmatism and with the active hydrothermal activity observed in the region (Neves et al., 2013; Kueppers and Beier, 2016; Mitchell et al., 2018; Schmidt et al., 2020).
The Azores archipelago shows a high seismic rate that results from the ongoing tectonic and volcanic deformation. In recent times, three earthquakes with M > 6 were felt in the archipelago, which focal mechanisms is shown in Figure 1B: the 9 July 1998, Mw 6.2 earthquake in Faial Island, which destroyed several buildings (Dziewonski et al., 1981; Matias et al., 2007; Zonno et al., 2010; Ekström et al., 2012); and the doublet Mw 6.3 and Mw 6.1 earthquakes on 5 April 2007, close to the Formigas Islets (Dziewonski et al., 1981; Ekström et al., 2012). Although the latter main shocks were followed by several moderate magnitude aftershocks and were strongly felt on eastern São Miguel and Santa Maria islands, they only caused low to moderate building damage due their distances to the islands (Silva et al., 2012; Silva et al., 2015; Caldeira et al., 2017; Fontiela et al., 2018; Silva et al., 2021).
Swarm-like activity involving low magnitude earthquakes is common in the Azores (Fontiela et al., 2018; Silva et al., 2021). One of the islands most prone to this type of activity is São Miguel (S. Miguel), the capital and most populated island of the Azores. S. Miguel is located to the south-east of the archipelago, towards the eastern end of the Terceira Rift. Like all islands of the Azores, S. Miguel is also of volcanic origin. Eruptions in S. Miguel have been relatively frequent during the last 5 ka (Chester et al., 2021). Studied eruptions have been associated with the volcanic features of the island, namely, the Sete Cidades, Furnas and Fogo volcanoes and the basaltic fissural complexes of Congro and Picos. The latter marks the location of the most recent eruption in S. Miguel, which occurred in the year of 1652 (Carmo et al., 2015; Gaspar et al., 2015; Kueppers and Beier, 2016).
In addition to volcanic activity, the island of S. Miguel also shows evidence of hydrothermal activity such as fumaroles, boiling mud pools and gas release (predominantly CO2) (Mateus et al., 2015; Rosário Carvalho et al., 2015). The Ribeira Grande geothermal system (CRG in Figure 1C), located near the Fogo volcano, is a site of energy exploration and hot springs facilities. This geothermal site extends towards the northern flank of the Fogo volcano, where evidence of active faulting can be found (Oliveira et al., 1990; Nunes, 1991; Carmo, 2014). Previous studies showed that the composition, temperature, and pH of fluids indicate a deep geothermal flow enriched in volcanic gases (Mateus et al., 2015; Rosário Carvalho et al., 2015).
Instrumental earthquake catalogs show two main regions of seismic activity in S. Miguel (Figure 1C) (Storchak et al., 2013; Storchak et al., 2015; Di Giacomo et al., 2018). The first lies on the westernmost part of the island, near the Sete Cidades Volcano and was mostly active from at least 1995 until approximately the year 2000. The second region has been active more recently and is located at the center of the island, around the Fogo Volcano and the Congro Fissural Volcanic Complex. During the last decade, several seismic swarms were recorded in this central region of S. Miguel. Silva et al. (2012) studied the seismicity in this region using data recorded between 2002 and 2010. This period included 4 notable swarms, including the 2005 swarm, which was the most intense swarm recorded in the instrumental catalog and occurred in the region of the Congro fissural system. Based on earthquake locations, the authors identified two clusters of activity, one around the Fogo volcano, to the east, and the other around Congro, to the west. A stress inversion showed a dominant normal faulting regime in Fogo, consistent with the Azores regional stress field. However, a heterogeneous local stress field was found in Congro, superimposed on the regional stress field. The authors interpreted the compressive stress at depth as causing fluids to rise, and the heterogeneous, dominantly extensive stress field at more shallow levels as brittle failure along fractures with various orientations due to the circulation of fluids rising from depth.
More recently, a new earthquake crisis occurred in S. Miguel in February 2018. The abnormal increase in seismicity rate, affecting the central part of the island between the Fogo Volcano and the Congro fissural volcanic system, was first reported by the Centro de Informação e Vigilância Sismovulcânica dos Açores (CIVISA) on 12 February 2018, (Serviço Regional de Proteção Civil e Bombeiros dos Açores, 2018). Hundreds of small earthquakes were detected within a few hours, most with ML << 3. The strongest event reached a magnitude of 3.4 and intensity V but caused no material damage (Instituto Português do Mar e da Atmosfera (IPMA), 2018).
In this work, we tackle two main objectives: 1) To validate a highly automated strategy based on the analysis of continuous waveform data to quickly characterize seismo-volcanic crises; and 2) To use this strategy to study the spatio-temporal evolution of seismicity during the recent February 2018 S. Miguel crisis. We start by analyzing the local earthquake catalogs to establish the February 2018 S. Miguel crisis as significantly above the background seismicity level. We then apply a mostly automated workflow to characterize the crisis. The automated workflow consists of earthquake detection, location, magnitude estimation, waveform-similarity classification, and augmentation of the catalog by template matching. We complement this analysis by computing focal mechanisms for the events with higher signal-to-noise ratio (SNR), assessing some statistical properties of the seismicity, and investigating the relationship between the seismicity and surface deformation. Finally, we interpret the February 2018 S. Miguel seismic sequence in light of possible driving mechanisms.
Two independent local earthquake catalogs are available for the Azores, generated by the two monitoring agencies that operate in the region, IPMA and CIVISA. Figure 2 shows the two local catalogs for the year of 2018 and for the island of S. Miguel (37.5° ≤ latitude ≤38.0° and −26.0° ≤ longitude ≤ −25.0°). The IPMA catalog contain hypocentral information for 621 earthquakes (620 with magnitude estimation) and the CIVISA catalog for 3,617 earthquakes (3,362 with magnitude estimation) (Figures 2A, D). The higher number of earthquakes in the CIVISA catalog is related to the higher station density of the CIVISA network in S. Miguel. The two local catalogs show similar general features, namely, an active earthquake cluster in the center of the island, in the Fogo-Congro region. A second region of activity is located mostly offshore, along the southern coast of S. Miguel, close to the Povoação Volcano (Figures 2D, E). However, the two catalogs also show some detail differences. In particular, the IPMA catalog shows epicenters distributed with a roughly N-S trend, whereas the CIVISA catalog shows epicenters clustered without a clear orientation.
FIGURE 2. (A,D) Magnitude histogram for the 2018 IPMA and CIVISA earthquakes (white bars), histogram for the February 2018 crisis only (gray bars), cumulative number of events with magnitude for the 2018 reference catalogs (solid black line) and for the February 2018 cluster (dashed black line). (B,E) Map view of S. Miguel showing the epicenters of earthquakes according to the two reference catalogs. Epicenters of all earthquakes occurred in 2018 are shown in black, whereas white circles represent those of the February 2018 cluster. (C,F) Epicenters of the February 2018 cluster with depth (by color) and magnitude (size of circle). Yellow triangles represent seismic stations.
Given that one of our goals is to validate an automated strategy for quickly building an earthquake catalog during seismo-volcanic crises, we started by identifying the most significant earthquake crisis that occurred in S. Miguel during 2018. For this purpose, we applied Seiscloud (Cesca, 2020) to the two local earthquake catalogs (Figure 3). Seiscloud is a clustering algorithm that groups earthquakes based on various measures of proximity. For our purpose, we searched for clusters of earthquakes that occurred close in time, where a cluster was defined by at least 10 events occurring within 3.65 days of each other (Nmin=10, ε=0.01).
FIGURE 3. Temporal evolution of the seismicity in S. Miguel for the year 2018 (left) and for the February 2018 seismic crisis (right). Histogram of the number of earthquakes in bins of (A) 1 week, and (D) six hours. Evolution of earthquake magnitude in time, considering the IPMA (B,E) and CIVISA (C,F) reference catalogs. Colors show different temporal clusters of earthquakes identified using Seiscloud (Cesca, 2020). The February 2018 cluster is identified in both catalogs with MLmax = 3.4.
Figure 3A shows weekly histograms of the number of earthquakes in S. Miguel during 2018 and Figures 3B, C show the temporal evolution of local magnitudes, considering both the IPMA and CIVISA catalogs. Distinct colors indicate the different earthquake clusters identified by Seiscloud. In both cases, the most populated cluster is the first one (pink dots in Figures 3B, C), which corresponds to February 2018 crisis. This cluster reached a maximum magnitude of ML 3.4 in both catalogs. Other clusters are identified later in the year, some consistently identified in the two catalogs (e.g., June 2018) and others whose characteristics depends on the catalog. We selected for further analysis the period of the first and most populated cluster, spanning 2 weeks between the 5th and the 20th of February 2018. This time interval encompasses 342 or 3,107 earthquakes, depending if IPMA or CIVISA catalog is considered (Figures 3D–F). The CIVISA catalog shows some early precursory activity already on February 8th. Both catalogs then show a sharp increase in the seismic rate on February 12th, when the largest earthquake occurred. The seismic rate then progressively decreases and is mostly over by February 20th.
To validate our automated strategy for catalog building, we used continuous waveform data recorded at 10 permanent seismic stations located in the island of S. Miguel. In particular, we used 3 broadband and 7 short-period stations, operated by Instituto Português do Mar e da Atmosfera, IP, 2006, CIVISA Seismo-Volcanic Monitoring Network-Azores Islands and Scripps Institution of Oceanography, 1996. The broadband stations are equally distributed throughout the island, whereas the short-period stations are concentrated close to the crisis (Figures 2B, C, E, F). Table 1 shows the details of the seismic stations.
For nearly automated catalog building, we adopted a workflow that consisted of detection, location, magnitude estimation, earthquake classification and catalog expansion using template matching (Figure 4). We further computed moment tensor solutions for the larger events.
In order to detect earthquakes, we used Lassie to continuously scan waveform data recorded at the selected stations during the chosen time interval. Lassie is an automated full waveform method inside the Pyrocko toolbox that detects and locates earthquakes using a grid search approach (Heimann et al., 2017). Lassie has been successfully applied before in various settings, including near-fault observatories and gas reservoirs (Comino et al., 2017; Adinolfi et al., 2019; Adinolfi et al., 2020; Braun et al., 2021; Meier et al., 2021; Simanjuntak et al., 2022). A recent comparison of detection performance for offshore seismicity (Cesca et al., 2022) showed comparable results among Lassie and PhaseNet (Zhu and Beroza, 2019). Lassie starts by computing characteristic functions from the continuous seismic waveforms recorded at different station channels. The characteristic functions should be tuned depending on the application. In our case, we used characteristic functions suitable for detecting P and S-waves, which results in two distinct Image Function Contributions (IFCs). Lassie then applies a delay-and-stack method to the characteristic functions and performs a grid search to image the likelihood of possible hypocenters based on the coherence of the characteristic functions. Lassie implements a spatial and temporal sampling reduction that improves computational efficiency, but on the other hand leads to high location uncertainties. Thus, we used Lassie only as a detection algorithm and locations were refined subsequently.
We carried out automatic detection using two different 1D velocity models: the regional model used both by IPMA and CIVISA, which we refer to as azoresipma (Steinmetz et al., 1976; Senos et al., 1980; Escuer, 2006), and a model based on P and S receiver functions that we refer to as rfcmlaa (Silveira et al., 2010). We band pass filtered the continuous waveform data from 2 to 9 Hz. We then searched for earthquakes within a 3D grid with a total length of 20 km along latitude and 25 km along longitude, centered on latitude = 37.77°, longitude = −25.40°. In depth, the grid had a total length of 30 km, starting at 1.0 km depth. The spacing between grid nodes was 1.0 km in all directions. This selected search volume includes only the closest seismic stations, leaving out the stations further away from the cluster, namely, BART and PSET. After several tests, we set a coherence threshold of 3.5 for the earthquake detection. This deliberately low threshold avoids missing the detection of low amplitude earthquakes but has the downside of increasing the number of false events (false positives), which must be removed later in the workflow. In our case, we removed the false events using both visual inspection and cross-checking against the reference catalogs.
In order to locate earthquakes, we used the Loki software (Grigoli et al., 2014; Grigoli et al., 2016). Loki has been successfully used before, both independently and coupled with Lassie, in observational setups comparable to ours (Grigoli et al., 2013; Matos et al., 2018; Rossi et al., 2020). Loki has an implementation similar to Lassie but is tuned to produce more accurate hypocentral locations from selected waveform segments. In particular, Loki uses the vertical energy function to determine the P-wave characteristic function and a principal component analysis technique sensitive to signal polarization to estimate the S-wave characteristic function. The hypocenter is found by an iterative search of the considered volume and origin times, considering the stacking of the short/long-term-average (STA/LTA) of the P and s characteristic functions. Loki uses 3D slowness grids that we computed using Non-LinLoc (Lomax et al., 2000). We computed these grids for the two velocity models previously mentioned, azoresipma and rfcmlaa. The 3D grids had the same dimensions as the grid used for detection with Lassie. Waveforms were bandpass filtered from 2 to 9 Hz, and a 15 s time window was selected around the Lassie detection time, starting 2 s before Lassie’s origin time.
We estimated the earthquake magnitudes using the waveform maximum amplitude approach (Båth, 1966). We used the traces recorded at the broadband stations CMLA and PCALD which are stations with fully documented and open-access instrumental response. The peak amplitude method consists of estimating the linear relationship between the maximum displacement recorded in the waveform and the local magnitude of an earthquake:
In this equation,
Our next step was to classify the events based on waveform similarity. For this purpose, we used vertical velocity recordings at the single station component MESC-EHZ. We chose this component because this station is very close to the focal region, its waveforms have a good signal-to-noise ratio, and continuous data are available for the entire study period. We extracted 15 s long waveforms for each earthquake starting 2 s prior to the origin time and applied a bandpass filter in the frequency range 2–9 Hz. We computed the cross-correlation coefficient (CC) between pairs of waveforms of different events using Obspy (Deichmann and Garcia-Fernandez, 1992; Krischer et al., 2015). Based on the CC, we then calculated a normalized distance d = 1 - CC. Hence, the distance between two events is equal to zero (d = 0) when the events are identical (CC = 1). Finally, we applied Seiscloud to identify subclusters of events using the resulting distance matrix. We used clustering parameters Nmin = 10 and ε = 0.2, meaning that a cluster was formed whenever for one event there were at least 10 others with a CC equal to or larger than 0.8.
Finally, we applied a matching phase algorithm PyMPA (Vuan et al., 2018) to complete the seismic catalog that we obtained after the location step with Loki. The template matching aims to detect new earthquakes hidden below the noise level, which rupture the same or nearby fault patch as the templates, thus generating identical waveforms. We resampled waveforms to 50 Hz and applied a 3–15 Hz bandpass filter; this frequency band is appropriate considering the seismic network configuration, the events size, and the studied region’s extent. Templates were trimmed using a 6 s window that started 3.5 s before the theoretical s wave arrival, estimated using the ObsPy TauP toolkit routines (Crotwell and Owens, 1998) and the 1D-velocity models mentioned above. We adopted Kurtosis-based tests to evaluate the signal-to-noise ratio of templates (Baillard et al., 2014), thus avoiding unwanted signals in the matching procedure (e.g., Vuan et al., 2018; Vuan et al., 2020).
In our analysis we considered the events with Loki’s highest waveform coherence (coherence ≥0.5), amounting to 402 or 409 templates, depending on the velocity model used. To declare a new detection, we used the following criteria: 1) the average of the normalized cross-correlation coefficient (ncc) between template and detection must be greater or equal to 0.6; 2) the coherent waveform signal of the detections must be observed in at least 7 channels; and 3) the threshold, defined as N times the daily median absolute deviation (MAD) of the network stack function (NSF) must also be greater than or equal to 15.
The location of the small events in the augmented catalog strictly depends on the quality of the input catalog locations and associated errors. Ross et al. (2019) and Simon et al. (2021) used different relocation tools and demonstrated that only a small portion of events from template matching could be relocated (on average less than 20%). Due to the high resolution of the starting catalog, we decided to keep the new detections co-located with the templates. The magnitude of the new events was estimated by amplitude comparison with the templates. A tenfold increase in the amplitude ratio corresponds to a one-unit increase in magnitude at each recording channel (Peng and Zhao, 2009). The final catalog is then formed by the template events plus the associated new detections with correlation equal to or higher than 0.6 with respect to the templates, which we refer to as “slaves.”
Finally, we performed a moment tensor (MT) inversion for the highest magnitude earthquakes, which are representative of the families identified by waveform similarity. The MT inversion was done using a probabilistic earthquake source inversion approach implemented in the software Grond (Heimann et al., 2018). The moment tensor inversion is challenging, given the weak magnitudes of these earthquakes, which rarely exceed ML 2.0 (Figure 3). Therefore, we inverted only for the deviatoric part of the MT. We fit 3-component velocity traces at all stations by cross-correlation and additionally fit full time-domain waveforms at stations CMLA and BART. This choice, already tested for the joint inversion of onshore and offshore data (Cabieces et al., 2020), is justified by the unverified sensitivity of the short-period seismic stations. For the cross-correlation we used a time window starting 1.5 s before the P-phase and ending 1.5 s after the S-phase, which were manually picked. For the time domain we fit body wave data, using 2 s long windows for P phases in the vertical component and for S-phase in the horizontal components. Green’s functions were computed using the velocity model azoresipma.
Figure 5 shows examples of a true (coherence = 8.9) and a false (coherence = 3.8) event detected with Lassie. In this case, the false detection is a result of noisy data and/or the low threshold that we considered for the detections. A visual inspection of the Lassie detections showed that the false detections also often resulted from instrumental issues (e.g., spikes) and events that overlap in time. Consequently, we systematically revised the catalog to remove false detections. The false positives could alternatively be reduced by either increasing the detection threshold, at the cost of missing the smaller events, or in the subsequent location step with Loki, as false positives should show poor locations. The original detection catalogs produced by Lassie contained 5,192 or 4,312 events for velocity models azoresipma or rfcmlaa, respectively. However, after removal of false events only 1,002 or 1,047 corresponded to true events.
FIGURE 5. Example of the detection of a true (origin time 2018-02-12 03:27:50.5) and a false (2018-02-12 05:02:25.6) event with Lassie. (A,E) Waveform traces at each seismic station used. (B,F) Characteristic functions for the normalized amplitude envelopes indicate the corrected travel time for the P-wave (solid red line) and S-wave (solid blue line) for each station. The best fit of the synthetic arrival time of the respective phases at each station is marked with a small red/blue vertical bar. (C,G) Map view of the station locations and probability density function distribution marking the most likely epicenter location with a white star. (D,H) Global detector function (solid red line) where the red shadowed area represents the time frame shown in the coherence detection map. The horizontal black line marks the threshold chosen for the detection.
The earthquakes detected in the previous step were next located using Loki. We retained only events with the best locations, imposing a Loki coherence value of 0.5 or larger, which diminished our catalogs from to 402 or 409 earthquakes with high-quality locations. Independently of the velocity model used, most events are located on the central part of S. Miguel, between the Fogo and Congro volcanic systems (Figure 6; Figure 1C), in agreement with the preliminary analysis of the local earthquake catalogs. The average location of all located earthquakes, shown by the blue cross in Figure 6, is close to the Congro fissural system. This main earthquake cluster has an elongated shape with a NW-SE orientation, previously unimaged. Cross-sections through the location volume (Figures 6B, C) show that earthquakes are located mostly at 10–15 km depth, becoming shallower both towards the NW and SW. The few earthquakes at the bottom of the grid likely have poorly constrained locations.
FIGURE 6. Hypocentral 2D histograms of the earthquakes located with Loki, using the same grid used for location. (A) Map and (B) longitude and (C) latitude cross-sections of the 402 events with coherence higher or equal to 0.5, using velocity model azoresipma. The blue cross represents the average location of the selected events. The cluster is more populated between the Fogo volcano and the Congro fissural complex at depths of 7–15 km (see Supplementary Figure S1 for rfcmlaa velocity model result).
The estimated magnitudes show that most located events have ML between 0.0 and 3.0 (Figure 7). Our maximum estimated magnitude ML = 3.44, for the event that occurred at on 2018-02-12 at 04:54:29, corresponding to the earthquake with ML = 3.4 in both local reference catalogs. Our estimated magnitude values show a good agreement with the earthquake magnitudes of the CIVISA and IPMA reference catalogs (Figures 7A, B).
FIGURE 7. (A) Estimated magnitude of the 402 relocated events vs. magnitude in each reference catalog. Orange circles show the comparison between our estimates and IPMA’s catalog and the purple circles show the comparison with the CIVISA catalog. The solid black line represents the y=x curve, for reference. (B) Comparison between IPMA (orange), CIVISA (purple) and our catalog (light green). (C,D) Same as (A,B) but using the catalog obtained after template matching, i.e., adding the slave events. Our estimated magnitude values generally show a good agreement with the reference catalogs.
The waveform similarity analysis allowed us to identify three different earthquake families. Independently of the catalog used (402 earthquakes - azoresipma or 409 earthquakes - rfcmlaa), the same three families of earthquakes were equally identified. Figure 8 shows and example of the waveforms in each of the three families based on the azoresipma catalog (results with the alternative catalog are similar). The waveforms of the three families all show clear P and S-phases but with different detail characteristics. Observing Figure 8 we note that the body waves of family C1 are very impulsive and high-frequency, followed by a longer-period coda. The events of family C2 show a low amplitude P-phase when compared to the other two families and a notable coda. Family C3 seems to have the longest duration P-phase, and both P and s phases are quite harmonic. We also note that S-P times are not significantly dissimilar among the families, with values of approximately 0.5 s–6.0 s for C1, 1.0 s – 7.0 s for C2 and 2.0 s – 6.0 s for C3.
FIGURE 8. Identification of different earthquake families based on the similarity of waveforms recorded at station MESC, vertical component (EHZ). Family C1 (blue), C2 (red) and C3 (green) are placed from (A–C). Colored traces correspond to the stacked waveforms of each family. Selected traces are plotted downwards by increasing dissimilarity to the top example waveform, where the dissimilarity d = 1- CC.
Finally, after applying the template matching technique to the continuous data and using the relocated 402 or 409 events as templates, we detected additional low amplitude earthquakes, augmenting the previous catalogs to 2,553 and 2,613 events, respectively. Figure 9 shows an example of a template event and its slaves for the three components of station MESC.
FIGURE 9. Example of the template matching results, showing one template event (black line) and associated detections (gray). We show as an example the template with origin time 2018-02-12T03:51:48.80. Each rectangle shows different components: (A) vertical (EHZ), (B) north (EHN), and (C) east (EHE) at station MESC. Each detection is attributed a ncc value, in which an event with ncc = 1 corresponds to the detection of the template itself.
Figures 7B, D show the number of events in the CIVISA and IPMA catalogs compared to those in our catalog both with and without the slaves events found by template matching. The figure also shows the catalog’s completeness magnitude (Mc) computed using ZMAP software (Weimer, 2001). The Mc of the IPMA and CIVISA catalogs are 1.7 and 0.5, respectively, for the two-week period under study. The Mc of our catalog is 1.1 before the template matching and 0.6 after adding the slaves (Figures 7C, D). Although the completeness magnitude is close to CIVISA’s, we detect slightly less earthquakes than CIVISA around Mc and slightly more earthquakes below Mc. On one hand, this indicates that by only using well-located events as templates, we still miss some very low magnitude (ML ∼ 0.6) events. On the other hand, using template matching we were able to detect some very small magnitude events that are not detected by CIVISA (ML ≲ 0). Note that our magnitudes slightly overestimate the magnitudes of the CIVISA catalog, whereas they are in good agreement with IPMA’s (Figures 7A, C). The difference in magnitudes is related to the different stations used to compute magnitudes in the different catalogs. The CIVISA catalog has a higher total number of events due to a higher number of low magnitude events recorded with ML ∼ 0.5 withal this catalog is based on a denser network in the hypocentral region.
Using our final catalog (2,553 events) we analyzed the temporal evolution of the February 2018 sequence (Figure 10). The activity started with a subdued precursory phase, corresponding to earthquake family C1 (blue dots in Figure 10), which lasted from February 5th to February 12th. This phase consisted of low rate, low magnitude (maximum ML < 2.0) seismicity and the activation of a structure at depths between ∼10 and 15 km. At the eighth day, on February 12th, the day with the highest seismic rate, after a slight decrease of the magnitude of the events (maximum ML ≤ ∼1.0), the seismic rate of C1 increased drastically during approximately 1 h, with events reaching ML close to 2.7. During this time, the last earthquakes of family C1 were recorded. The subsidence of activity related to family C1 was accompanied by the onset of earthquake family C2 (red dots in Figure 10). Family C2 is the most populated one (N = 2,263 events) and its events reach the peak magnitude of ML 3.44. It is located at shallower depths of ∼8–12 km and slightly to the SE of C1. After reaching the peak magnitude, the C2 magnitudes start to decrease until they stabilize ∼10 h later at ML ≤ ∼2.0. In the last hours of February 12th, a new earthquake family arises (C3, green dots in Figure 10), and the seismic rate of cluster C2 starts to decrease. C3 is located at slightly shallower levels and further to the SE than C2. Most of the earthquakes in our catalog (∼86%) occur on February 12th. At the end of February 13th, the rate and magnitude of the seismicity becomes similar to the early stages of the sequence. The seismic sequence activity continued for another 7 days, with a decreasing seismic rate.
FIGURE 10. Temporal evolution of the earthquake’s magnitude: (A) two-week period corresponding to the February 2018 swarm. (B) Zoom for February 12th, day with the highest seismic rate. Hypocentral location of the earthquake families: (C) map view and (D,E) cross-sections with contour line surrounding 70% of the events from the final catalog. The events are colored according to the families identified by waveform similarity: C1 (blue), C2 (red) and C3 (green).
The moment tensor inversion of selected earthquakes, including those with the highest magnitude and good signal-to-noise ratio at various stations, led to the determination of 9 deviatoric solutions (Table 2; Figure 11). Most of the focal mechanisms inferred correspond to earthquakes of family C2, since they have higher magnitude. We were also able to obtain one solution each for earthquakes of families C1 and C3. Although we find some variability in the MT solutions, normal faulting focal mechanisms with strike-slip components are dominant. Most Non-Double Couple components show negative compensated linear vector dipoles (CLVDs), ranging from 1% to 58%. The tension axes are, in general, oriented NW-SE. Centroid depths range between 4 and 10 km, slightly shallower than the hypocentral depths estimated with Loki (6–15 km). Moment magnitudes values (Mw) are similar to the ML estimates.
FIGURE11. (A) Map view and (B) cross-section of the final automated earthquake catalog, comprising templates plus slave events, and the location of the focal mechanisms obtained by moment tensor (Table 2). The catalog epicenters are represented by white circles that mark the corresponding location grid cells, and the events for which we estimated the focal mechanisms are marked in colored circles. The colors of the focal mechanisms indicate their families: C1 (blue), C2 (red) and C3 (green). Seismic stations are shown by yellow triangles. Observed displacements at GNSS stations VFDC and FRNS, located in S. Miguel, with respect to station AZSM, in the island of Santa Maria, are shown by purple arrows.
Using a nearly automated workflow based on the analysis of continuous waveform data, we were able to build an earthquake catalogue for the February 2018 S. Miguel sequence. The evolution of the number of cumulative events in our catalog at each stage of the automatic procedure is shown in Figure 12. Note that a direct comparison between our catalogue and the local earthquake catalogs is not warranted because we used a different station network from both IPMA and CIVISA. In particular, we used some IPMA’s and a few CIVISA’s stations. Still, for reference we show our catalog versus the CIVISA catalog, which is more complete than IPMA’s. At the detection step, when using Lassie, we deliberately opted to use a low threshold, which allowed us not to miss true small amplitude events. Figure 12B shows that the number of Lassie’s automated detections deviates from CIVISA’s on February 6th and 18 February 2018. These deviations are due mostly to instrumental problems and spikes. The false positives must be filtered out, which in our case was done by visual inspection and cross-validation with the local earthquake catalogs. Alternatives to decrease the number of false positives include increasing the detection threshold, manually revising the events if manpower is available, or filtering at the next step during location, as false events should not be successfully located. After removal of the spurious detections, our cumulative number of detected events follows the same trend as CIVISA’s (Figure 12C). After earthquake location and retention of well-located earthquakes (coherence ≥0.5), we further reduced the number of total events to approximately half of those detected (Figure 12D). However, with template matching, using the high-quality catalog of well-located events as templates, we were able to efficiently augment the catalog again, finally reaching ∼82% of the number of events in the CIVISA catalog. We thus successfully achieved a very complete catalogue, that includes information on different sub-clusters identified by waveform similarity (Figure 12E).
FIGURE 12. Temporal evolution of the cumulative number of earthquakes after each step of our workflow, using the azoresipma velocity model: (A) IPMA (solid green line) and CIVISA (solid gray) reference catalogs, (B) Lassie detections, (C) Lassie revised detections, (D) Loki results with coherence ≥0.5 and (E) Final earthquake catalog obtained with the template matching. Results after each step are marked with solid red lines, whereas the CIVISA catalog is shown with a solid gray line for comparison. The automatic process could reproduce ∼82% of the manually picked CIVISA catalog (see Supplementary Figure S1 for rfcmlaa velocity model result).
The results obtained throughout our workflow using the two local velocity models, azoresipma and rfcmlaa, are generally similar. This may be because the two models are not significantly different at the depths where most events originate (5–15 km). The similar results also indicate that our solutions are robust and stable to minor velocity model variations, which is important given that the procedure is mostly automated.
The temporal evolution of the seismicity in our catalog shows that although, at first, the earthquake sequence may look like a mainshock-aftershock sequence (Figure 10A). When we look in detail, we find that the highest magnitude earthquake occurred after the onset of the period of most intense activity (Figure 10B). We assess the clustering and swarm-like behavior of seismicity by computing different indicators, which are typically used to discriminate among seismic swarms and seismic sequences. The coefficient of variation (CV), which reflects the statistics of inter-event times (Kagan and Jackson, 1991; Zöller et al., 2006; Passarelli et al., 2015), is a measure of the temporal clustering, where CV >> 1 is expected for a clustered behavior. The skewness (μ) (Roland and McGuire, 2009; Chen and Shearer, 2011) and the normalized time of the mainshock (tm) (Zhang and Shearer, 2016) account for the temporal evolution of moment release. Seismic sequences are expected to release most of the moment in the early phase, while in seismic swarm large magnitude earthquakes can occur in later phases. Skewness values below 6 and tm values above 0.5 are generally suggested for swarm-like activity. The classification conditions based on CV (Kagan and Jackson, 1991; Zöller et al., 2006) is accomplished for all cases, either considering the whole catalog or single clusters (Table 3). Classification based on μ and tm (Zhang and Shearer, 2016) is more heterogeneous, often showing mixed seismicity types. In detail, cluster C1 is clearly resolved as swarm. Cluster C3 and the whole sequence are identified as mixed swarm/mainshock-aftershock types. The modulations of these indicators among different sub-clusters indicates a complex behavior, with an evident swarm activity in the initial phase and some combination of swarm and mainshock-aftershock sequences during the latter part of the sequence, that includes the most significant events.
TABLE 3. Summary of the statistical analysis and corresponding classification among swarm, mixed and mainshock-aftershocks (MA) seismicity.
Our final catalog shows that earthquakes are located in the central part of S. Miguel, in the region of the Congro fissural complex, at 5–15 km depth. The inferred location of the February 2018 swarm is quite close to the May/September 2005 swarm (Silva et al., 2012), suggesting a reactivation of the same system. The swarm starts with a precursory phase that includes a small number of low magnitude events, that we identified as the beginning of C1 activity. This is the deepest sub-cluster, suggesting that the swarm initiated with fracture at depth, eventually resulting from the intrusion of volcanic fluids. The high-frequency character of C1 waveforms confirms the predominance of brittle failure. As the activity of C1 subsides and disappears, family C2 emerges with a high seismic rate. This family contains the highest magnitude earthquakes observed during the entire swarm and is located slightly shallower and to the SE of C1, suggesting the migration of fracturing towards the surface and the activation of shallower faults. The last family of earthquakes (C3) emerged closely after C2 and very briefly after the earthquake with highest magnitude. Family C3 is slightly shallower and to the SE than C2, suggesting the continued migration of earthquakes and the activation of a third shallower fault system. The events of family C3 have a very harmonic character, which we interpret as indicating faulting in a fluid-enriched system. We hypothesize that the swarm started with the activation of family C1, whose earthquakes opened a pathway for fluids to rise to the surface, with the seismicity migrating to shallower levels, activating families C2 and finally C3, which occurs already in a fluid enriched environment (Figure 10).
Previous works show that seismicity in volcanic environments is often used to track the propagation of fluids within the plumbing system, with recent outstanding cases involving the migration over tens of kilometers and even across the whole crust (Cesca et al., 2020; Cesca et al., 2022). The spatial distribution of volcano seismicity with different characteristics, such as a different frequency content of their signals, is often interpreted to understand the plumbing system and different processes along complex paths for fluid migration (del Fresno et al., 2023; Gaete et al., 2019; Galluzzo et al., 2023). A very visible difference between the earthquake families resides in the frequency content of their waveforms. We speculate that the higher-frequency waveform family, C1, indicates brittle failures. In contrast, the more harmonic and lower frequency waveforms of C2 and C3 may correspond to earthquakes occurring in the presence of fluids. Thus, similar to the 2005 swarm, the February 2018 swarm appears to have been triggered at depth, possibly due to the uprising of fluids. The brittle failure at depth seems to have opened a pathway for fluids to migrate upwards from depth. Once activated, faulting responds dominantly to the regional stress regime.
Moment tensor inversion shows normal faulting with strikes-orientated NW-SW. This pattern is consistent with the regional stress regime (Figure 1B) and with reference MT solutions computed for earthquakes to the east and the west of S. Miguel (e.g., Custódio et al., 2016). Silva et al. (2012) obtained a similar result when analyzing the focal mechanisms of the 2005 swarm, indicating that the Fogo-Congro stress field is dominantly extensional and coincident with the regional tectonics. The most robust solutions, i.e., solutions with a high consistency between the best and the mean result, have a small non-DC component percentage and a common T axis. The presence of non-DC components close but not equal to zero, despite not being very reliable due to the moment tensor estimation uncertainties, indicates the possibility of tensile and compressive movements associated with the opening and/or closing of cracks that we hypothesize to be caused by infiltration of volcanic and/or thermal fluids. We hypothesize that fluids induced and facilitated fracturing, generating earthquakes whose kinematics is governed by the regional stress field.
In order to further complement our analysis, we investigated whether surface deformation was associated with the swarm. To this end, we processed data from GNSS stations of the REPRAA network (Geo-Referencing Permanent Stations Network from Azores Government - REPRAA) along with multiple IGS (Johnston et al., 2017) and EUREF (Bruyninx et al., 2019) stations using the GAMIT/GLOBK software (Herring et al., 2018). For this processing, we adopted the conventions, models, and recommendations by IGS according to the 3rd IGS Data Reprocessing Campaign held in 2019 (Johnston et al., 2017), resulting in a consistent set of daily station position time series, expressed in the ITRF2014 reference frame (Altamimi et al., 2019). These were subsequently transformed into a local reference frame with three components: North, East, and Up. We analyzed the time series for 3 stations in S. Miguel (PDEL, VFDC, and FRNS) and 1 station in Santa Maria Island (AZSM), starting in April 2017 until September 2022. We considered AZSM as fixed station because it is located outside the area of deformation. The time series of the differences between the position of GNSS stations in S. Miguel and AZSM show different pattens (Figure 13). PDEL, the GNSS station in S. Miguel further away from the swarm, shows no significative motion with respect to the fixed station AZSM. However, the February 2018 seismic swarm marks the beginning of a period of ground deformation observed at the 2 closest stations, VFDC and FRNS, which lasts over a long-time interval of about 17 months. Station VFDC, to the southwest of the swarm, moves mostly to the south with a total displacement of 29.0 mm, whereas station FRNS, located to the east of the swarm, shows movement mostly to the east with a total displacement of 9.8 mm, both with respect to fixed AZSM (Figure 13A).
FIGURE 13. Evolution of the position of GNSS stations in São Miguel Island with respect to station AZSM, located at Santa Maria Island. Displacement of (A) the Fogo Volcano station, VFDC, towards the north, and (B) the Furnas station, FRNS, towards the east. During an approximately 17-month long period starting with the 2018 swarm, station VFDV moves 29.0 mm to the south and station FRNS moves 9.8 mm towards the east, deviating from their long-term trends. We did a linear fit (red line) of the differential positions between February 2018 and July 2019 in order to obtain the displacement estimates.
The normal faulting with strike-oriented NW-SE inferred from moment tensor inversion together with the observed surface deformation showing VFDC moving to the south and FRNS to the east are compatible with the observed regional extensional pattern of S. Miguel Island, associated with the regional opening of the Terceira Rift. Alternatively, the surface deformation could have a radial pattern around the swarm’s center, similar to what was observed during the 2005 swarm (Silva et al., 2012), more indicative of an intrusion. In either case, the swarm marks the beginning of a ∼1.5 years long period of surface deformation, which may indicate either aseismic slip of tectonic faults or an aseismic intrusion.
We studied the spatio-temporal evolution of the seismic swarm that occurred in February 2018 in S. Miguel. We successfully set up a semiautomatic procedure capable of generating a high-quality earthquake catalog that reproduces the main features of the local reference catalogs. The automatization of the seismic monitoring workflow presented here has the advantage of reducing the time and human power needed for earthquake catalog generation, namely, for the determination of basic earthquake parameters such as origin time, location, and magnitude. In addition, the full waveform analysis allowed us to identify different families of similar earthquakes, which in turn provided a clearer image of the spatio-temporal migration of seismicity and of the underlying processes. We deduce that earthquakes with higher frequency content are associated to brittle failure and lower frequencies are associated to the presence of fluids. The template matching allowed us to augment the catalog, and further provided information on the sites where seismicity occurred persistently. We conclude that the automated analysis of full waveform data is key to provide information on the evolution of unrest episodes and their underlying processes, representing a critical complement to traditional earthquake catalogs and helping to better assess the hazard of seismo-volcanic crises.
The February 2018 swarm occurred in the region of the Congro fissural complex, similar to the previous May/September 2005 swarm (Silva et al., 2012) and likely reactivating the same fault system. The epicenters form an elongated shape with a roughly NW-SE orientation. The seismogenic region extends through depths of 5–15 km, becoming shallower to the northwest and to the southwest. The sequence displays mostly a swarm-like behavior, although some complexity is found. Most of the seismicity occurs over the first day of the second week of activity (February 12th), with the highest magnitude earthquake occurring approximately one hour after the onset of the phase of most intense activity. Earthquake family C1 occurs at the deepest level and is identified as a precursory phase of the swarm. It is followed by C2, the most active family, and finally C3 at the shallowest levels. The onset of family C2 marks a clear change in the swarm. Our moment tensor results are consistent with previous studies of seismic swarms that occurred in the same region, including the 2005 swarm, indicating that the Fogo-Congro stress field is predominantly tensional, with the main extension axes oriented NW-SE. Thus, the seismicity of this region seems to be a local response to the regional forcing triggered by the uprising of fluids from depth. Through the detailed analysis of the February 2018 swarm, we conclude that this seismic activity is due to episodic fluid transfer through the crust associated with hydrothermal and/or magmatic activity.
We conclude that the Fogo-Congro system continues to be seismo-volcanically active and that the 2005 crisis was not an isolated unrest episode but rather a part of long-lived and ongoing deformation processes. The region should be closely monitored using multidisciplinary instrumentation, namely, comprising seismic and geodetic capability, among others. As we have shown here, both seismic and aseismic deformation are observed, and the two datasets should be used analyzed together for better monitoring of the region and improved hazard assessment and risk mitigation.
The datasets presented in this article are not readily available because a part of the raw data supporting the conclusions of this article will be only made available by the authors or by CIVISA (Centro de Informação e Vigilância Sismovulcânica dos Açores) if a signed authorization is provided by the CIVISA institute. Requests to access the datasets should be directed to CIVISA (Y2l2aXNhQGF6b3Jlcy5nb3YucHQ=) or RS (Uml0YS5BTS5TaWx2YUBhem9yZXMuZ292LnB0).
AS, analysed the detailed spatio-temporal evolution of the February 2018 swarm applying and tuning the automatic waveform-based detection and location algorithms to the continuous seismic data, and compiling all the results. SCu, contribution, as my PhD main supervisor, was based on supervision, guidance and revision of all the seismic data analysis. SCe, performed the moment tensor (MT) inversion for the highest magnitude earthquakes using a probabilistic earthquake source inversion approach implemented in the software Grond (Heimann et al., 2018). RS, contributed by sharing the São Miguel Island earthquake catalog of 2018 and waveform data of 5 CIVISA seismic stations, with station codes: LFA, MESC, PCNG, PRCH, VIF in São Miguel. AV, shared the software and contributed to the template matching [PyMPA (Vuan et al., 2018)] application to complete the seismic catalog by detecting new earthquakes with identical waveforms hidden below the noise level. VM, computed ground deformation through the analysis of the time series of GNSS station position for 3 closest stations to the swarm epicentral area located in S. Miguel (station codes: PDEL, VFDC, and FRNS) and 1 station in Santa Maria Island (station code: AZSM). All authors contributed to the article and approved the submitted version.
We thank the Fundação para a Ciência e Tecnologia (FCT) for funding this work through grant SFRH/BD/147385/2019, project UIDB/50019/2020 – IDL and the RESTLESS project PTDC/CTAGEF/6674/2020.
We thank Fernando Carrilho (IPMA) for providing the earthquake catalog of the Azores archipelago. We thank CIVISA for sharing the earthquake catalog of 2018 and the waveform data of 5 seismic stations in São Miguel Island. We also recognize AV contribution which research is supported by Project PE0000005 - RETURN under the National Recovery and Resilience Plan (NRRP), Mission 4 Component 2 Investment 1.3 - Call for tender No. 341 of 15 March 2022 of Italian Ministry of University and Research funded by the European Union – NextGenerationEU.
The authors declare that the research was conducted in the absence of any commercial or financial relationships that could be construed as a potential conflict of interest.
All claims expressed in this article are solely those of the authors and do not necessarily represent those of their affiliated organizations, or those of the publisher, the editors and the reviewers. Any product that may be evaluated in this article, or claim that may be made by its manufacturer, is not guaranteed or endorsed by the publisher.
The Supplementary Material for this article can be found online at: https://www.frontiersin.org/articles/10.3389/feart.2023.1144151/full#supplementary-material
Adam, C., Madureira, P., Miranda, J. M., Lourenço, N., Yoshida, M., and Fitzenz, D. (2013). Mantle dynamics and characteristics of the Azores plateau. Earth Planet. Sci. Lett. 362, 258–271. doi:10.1016/j.epsl.2012.11.014
Adinolfi, G. M., Cesca, S., Picozzi, M., Heimann, S., and Zollo, A. (2019). Detection of weak seismic sequences based on arrival time coherence and empiric network detectability: An application at a near fault observatory. Geophys. J. Int. 218 (3), 2054–2065. doi:10.1093/gji/ggz248
Adinolfi, G. M., Picozzi, M., Cesca, S., Heimann, S., and Zollo, A. (2020). An application of coherence-based method for earthquake detection and microseismic monitoring (Irpinia fault system, Southern Italy). J. Seismol. 24 (5), 979–989. doi:10.1007/s10950-020-09914-7
Altamimi, Z., Rebischung, P., Collilieux, X., Métivier, L., and Chanard, K. (2019). “Review of reference frame representations for a deformable Earth,” in IX hotine-marussi symposium on mathematical geodesy (Cham: Springer).
Álvarez-Gómez, J. A. (2019). FMC-Earthquake focal mechanisms data management, cluster and classification. SoftwareX 9, 299–307. doi:10.1016/j.softx.2019.03.008
Baillard, C., Crawford, W. C., Ballu, V., Hibert, C., and Mangeney, A. (2014). An automatic kurtosis-based P-and S-phase picker designed for local seismic networks. Bull. Seismol. Soc. Am. 104 (1), 394–409. doi:10.1785/0120120347
Båth, M. (1996). Earthquake energy and magnitude. Physics and Chemistry of the Earth 7, 115–165. doi:10.1016/0079-1946(66)90003-6
Braun, T., Famiani, D., Caciagli, M., Cesca, S., Heimann, S., and Dahm, T. (2021). On the origin of micro-earthquakes in geothermal areas (Omega): First results from a seismic experiment at Mt. Amiata (Italy). Bull. Geophys. Oceanogr. 62 (2), 231–242. doi:10.4430/bgta0316
Bruyninx, C., Legrand, J., Fabian, A., and Pottiaux, E. (2019). GNSS metadata and data validation in the EUREF Permanent Network. GPS Solutions 23 (4), 106. doi:10.1007/s10291-019-0880-9
Cabieces, R., Buforn, E., Cesca, S., and Pazos, A. (2020). Focal parameters of earthquakes offshore cape st. Vincent using an amphibious network. Pure Appl. Geophys. 177 (4), 1761–1780. doi:10.1007/s00024-020-02475-3
Caldeira, B., Fontiela, J., Borges, J. F., and Bezzeghoud, M. (2017). Grandes terremotos en Azores. Física La Tierra 29, 57601. doi:10.5209/fite.57601
Carmo, R. L. (2014). Estudos de neotectónica na ilha de São Miguel: uma contribuição para o estudo do risco sísmico no arquipélago dos Açores.
Carmo, R., Madeira, J., Ferreira, T., Queiroz, G., and Hipólito, A. (2015). Chapter 6 volcano-tectonic structures of são Miguel island, azores. Geol. Soc. Mem. 44 (1), 65–86. doi:10.1144/M44.6
Cesca, S., Letort, J., Razafindrakoto, H. N. T., Heimann, S., Rivalta, E., Isken, M. P., et al. (2020). Drainage of a deep magma reservoir near Mayotte inferred from seismicity and deformation. Nat. Geosci. 13 (1), 87–93. doi:10.1038/s41561-019-0505-5
Cesca, S. (2020). Seiscloud, a tool for density-based seismicity clustering and visualization. J. Seismol. 24 (3), 443–457. doi:10.1007/s10950-020-09921-8
Cesca, S., Sugan, M., Rudzinski, Ł., Vajedian, S., Niemz, P., Plank, S., et al. (2022). Massive earthquake swarm driven by magmatic intrusion at the Bransfield Strait, Antarctica. Commun. Earth Environ. 3, 89. doi:10.1038/s43247-022-00418-5
Chen, X., and Shearer, P. M. (2011). Comprehensive analysis of earthquake source spectra and swarms in the Salton Trough, California. J. Geophys. Res. Solid Earth 116 (9), B09309. doi:10.1029/2011JB008263
Chester, D., Duncan, A., Coutinho, R., and Wallenstein, N. (2021). “Earthquakes and volcanic activity on islands,” in History and contemporary perspectives from the azores (Oxfordshire, UK: Routledge).
Comino, J. Á. L., Heimann, S., Cesca, S., Milkereit, C., Dahm, T., and Zang, A. (2017). Automated full waveform detection and location algorithm of acoustic emissions from hydraulic fracturing experiment. Procedia Eng. 191, 697–702. doi:10.1016/j.proeng.2017.05.234
Crotwell, H. P., and Owens, T. J. (1998). The TauP toolkit: Flexible seismic travel-time and raypath utilities. Available at: http://www.seis.sc.edu.
Custódio, S., Lima, V., Vales, D., Cesca, S., and Carrilho, F. (2016). Imaging active faulting in a region of distributed deformation from the joint clustering of focal mechanisms and hypocentres: Application to the Azores-western Mediterranean region. Tectonophysics 676, 70–89. doi:10.1016/j.tecto.2016.03.013
Deichmann, N., and Garcia-Fernandez, M. (1992). Rupture geometry from high-precision relative hypocentre locations of microearthquake clusters. Geophys. J. Int. 110 (3), 501–517. doi:10.1111/j.1365-246X.1992.tb02088.x
del Fresno, C., Cesca, S., Klügel, A., Domínguez Cerdeña, I., Díaz-Suárez, E. A., Dahm, T., et al. (2023). Magmatic plumbing and dynamic evolution of the 2021 La Palma eruption. Nat. Commun. 14 (1), 358. doi:10.1038/s41467-023-35953-y
Di Giacomo, D., Robert Engdahl, E., and Storchak, D. A. (2018). The ISC-gem earthquake catalogue (1904-2014): Status after the extension project. Earth Syst. Sci. Data 10 (4), 1877–1899. doi:10.5194/essd-10-1877-2018
DziewonskiChou, A. M. T. A., Wondhouse, J. H., and Woodhouse, J. H. (1981). Determination of earthquake source parameters from waveform data for studies of global and regional seismicity. J. Geophys Res. 86 (2), 2825–2852. doi:10.1029/jb086ib04p02825
Ekström, G., Nettles, M., and Dziewoński, A. M. (2012). The global CMT project 2004-2010: Centroid-moment tensors for 13,017 earthquakes. Phys. Earth Planet. Interiors 200-201, 1–9. doi:10.1016/j.pepi.2012.04.002
Escuer, M. (2006). “Improving seismic vp and vs models of the azores archipelago,”. M. Sc. thesis (Ponta Delgada, Portugal: University of Azores).
Fontiela, J., Sousa Oliveira, C., and Rosset, P. (2018). “Characterisation of seismicity of the azores archipelago: An overview of historical events and a detailed analysis for the period 2000–2012,” in Active volcanoes of the world (Cham: Springer), 127–153.
Gaete, A., Cesca, S., Franco, L., San Martin, J., Cartes, C., and Walter, T. R. (2019). Seismic activity during the 2013-2015 intereruptive phase at Lascar volcano, Chile. Geophys. J. Int. 219 (1), 449–463. doi:10.1093/gji/ggz297
Galluzzo, D., Manzo, R., La Rocca, M., Nardone, L., and Di Maio, R. (2023). Detection of low frequency seismicity at Mt. Vesuvius based on coherence and statistical moments of seismic signals. Appl. Sci. Switz. 13 (1), 194. doi:10.3390/app13010194
Gaspar, J., Guest, J. E., Duncan, A., Chester, D., and Barriga, F. (2015). Chapter 1 volcanic geology of são Miguel island (azores archipelago): Introduction. Geol. Soc. Mem. 44 (1), 1–3. doi:10.1144/M44.1
Geo-referencing permanent stations network from Azores government - REPRAA Geo-referencing permanent stations network from Azores government - REPRAA. Available at: http://repraa.azores.gov.pt.
Grigoli, F., Cesca, S., Amoroso, O., Emolo, A., Zollo, A., and Dahm, T. (2014). Automated seismic event location by waveform coherence analysis. Geophys. J. Int. 196 (3), 1742–1753. doi:10.1093/gji/ggt477
Grigoli, F., Cesca, S., Krieger, L., Kriegerowski, M., Gammaldi, S., Horalek, J., et al. (2016). Automated microseismic event location using Master-Event Waveform Stacking. Sci. Rep. 6, 25744. doi:10.1038/srep25744
Grigoli, F., Cesca, S., Vassallo, M., and Dahm, T. (2013). Automated seismic event location by travel-time stacking: An application to mining induced seismicity. Seismol. Res. Lett. 84 (4), 666–677. doi:10.1785/0220120191
Heimann, S., Isken, M., Kühn, D., Sudhaus, H., Steinberg, A., Daout, S., et al. (2018). Grond: A probabilistic earthquake source inversion framework. Potsdam, Germany: GFZ Data Services.
Heimann, S., Kriegerowski, M., Isken, M., Cesca, S., Daout, S., Grigoli, F., et al. (2017). Pyrocko-An open-source seismology toolbox and library. Potsdam, Germany: GFZ Data Services.
Herring, T. A., King, R., Floyd, M. A., and McClusky, S. C. (2018). “GAMIT reference manual: GPS analysis at MIT, release 10.7; department of Earth,” in Atmospheric and planetary sciences (Cambridge, MA: Massachusetts Institute of Technology).
Instituto Português do Mar e da Atmosfera, IP (2006). “Portuguese national seismic network [data set],” in International federation of digital seismograph networks.
Instituto Português do Mar e da Atmosfera (IPMA) (2018). Boletim sismológico preliminar dos Açores. Available at: http://www.ipma.pt.
Johnston, G., Riddell, A., and Hausler, G. (2017). The internati 33. Int. GNSS Serv. 33 (1). doi:10.1007/978-3-319-42928-1_33
Kagan, Y. Y., and Jackson, D. D. (1991). Long-term earthquake clustering. Geophys. J. In. 104, 117–134. doi:10.1111/j.1365-246x.1991.tb02498.x
Kaverina, A. N., Lander, A. V., and Prozorov, A. G. (1996). Global creepex distribution and its relation to earthquake-source geometry and tectonic origin. Geophys. J. Int. 125 (1), 249–265. doi:10.1111/j.1365-246X.1996.tb06549.x
Krischer, L., Megies, T., Barsch, R., Beyreuther, M., Lecocq, T., Caudron, C., et al. (2015). ObsPy: A bridge for seismology into the scientific Python ecosystem. Comput. Sci. Discov. 8 (1), 014003. doi:10.1088/1749-4699/8/1/014003
Kueppers, U., and Beier, C. (2016). Active volcanoes of the world volcanoes of the azores revealing the geological secrets of the central northern atlantic islands. Available at:http://www.springer.com/series/10081.
Lomax, A., Virieux, J., Volant, P., and Berge-Thierry, C. (2000). Imaging active faulting in a region of distributed deformation from the joint clustering of focal mechanisms and hypocentres: Application to the Azores-western Mediterranean region. Tectonophysics 676, 70–89. doi:10.1007/978-94-015-9536-0_5
Madeira, J., Brum da Silveira, A., Hipólito, A., and Carmo, R. (2015). Chapter 3 active tectonics in the central and eastern azores islands along the eurasia–nubia boundary: A review. Geol. Soc. Mem. 44 (1), 15–32. doi:10.1144/M44.3
Mateus, A., Carvalho, M. R., Nunes, J. C., and Carvalho, J. M. (2015). Influence of wall-rock alteration and fluid mixing mechanisms in the chemistry of thermal fluids and mud-pool sediments at Caldeiras da Ribeira Grande (S. Miguel Island, Azores). Environ. Earth Sci. 73 (6), 2809–2831. doi:10.1007/s12665-014-3439-7
Matias, L., Dias, N. A., Morais, I., Vales, D., Carrilho, F., Madeira, J., et al. (2007). The 9th of july 1998 faial island (azores, north atlantic) seismic sequence. J. Seismol. 11 (3), 275–298. doi:10.1007/s10950-007-9052-4
Matos, C., Custódio, S., Batló, J., Zahradník, J., Arroucau, P., Silveira, G., et al. (2018). An active seismic zone in intraplate west iberia inferred from high-resolution geophysical data. J. Geophys. Res. Solid Earth 123 (4), 2885–2907. doi:10.1002/2017JB015114
Meier, M., Schlindwein, V., Scholz, J. R., Geils, J., Schmidt-Aursch, M. C., Krüger, F., et al. (2021). Segment-scale seismicity of the ultraslow spreading knipovich ridge. Geochem. Geophys. Geosystems 22 (2). doi:10.1029/2020GC009375
Miranda, J. M., Luis, J. F., Lourenço, N., and Goslin, J. (2014). Distributed deformation close to the azores triple “point”. Mar. Geol. 355, 27–35. doi:10.1016/j.margeo.2014.05.006
Miranda, J. M., Luis, J. F., Lourenço, N., and Fernandes, R. M. S. (2015). Chapter 2 the structure of the azores triple junction: Implications for são Miguel island. Geol. Soc. Mem. 44 (1), 5–13. doi:10.1144/M44.2
Mitchell, N. C., Stretch, R., Tempera, F., and Ligi, M. (2018). “Volcanism in the azores: A marine geophysical perspective,” in Volcanoes of the azores. Active volcanoes of the world. Editors U. Kueppers, and C. Beier (Berlin, Heidelberg: Springer), 101–126.
Neves, M. C., Miranda, J. M., and Luis, J. F. (2013). The role of lithospheric processes on the development of linear volcanic ridges in the Azores. Tectonophysics 608, 376–388. doi:10.1016/j.tecto.2013.09.016
Nunes, J. C. (1991). Microssismos e neotectónica–Contribuição para o seu estudo nos Açores. Provas de Aptidão Pedagógica e Capacidade Cient\’\ifica. Ponta Delgada: Monografia. Departamento de Geociências. Universidade Dos Açores, 245.
Oliveira, C. S., Costa, A. C., Forjaz, V. H., and Nunes, J. C. (1990). Seismic hazard analysis in zones of time and space interdependence: An application to sao Miguel island, azores*. Nat. Hazards 3, 15–29. doi:10.1007/bf00144971
O’Neill, C., and Sigloch, K. (2018). Crust and mantle structure beneath the azores hotspot-evidence from geophysics. Act. Volcanoes World, Febr. 87, 71–87. doi:10.1007/978-3-642-32226-6_5
Passarelli, L., Hainzl, S., Cesca, S., Maccaferri, F., Mucciarelli, M., Roessler, D., et al. (2015). Aseismic transient driving the swarm-like seismic sequence in the Pollino range, Southern Italy. Geophys. J. Int. 201 (3), 1553–1567. doi:10.1093/gji/ggv111
Peng, Z., and Zhao, P. (2009). Migration of early aftershocks following the 2004 Parkfield earthquake. Nat. Geosci. 2 (12), 877–881. doi:10.1038/ngeo697
Roland, E., and McGuire, J. J. (2009). Earthquake swarms on transform faults. Geophys. J. Int. 178 (3), 1677–1690. doi:10.1111/j.1365-246X.2009.04214.x
Rosário Carvalho, M., Mateus, A., Nunes, J. C., and Carvalho, J. M. (2015). Origin and chemical nature of the thermal fluids at Caldeiras da Ribeira Grande (Fogo Volcano, S. Miguel Island, Azores). Environ. Earth Sci. 73 (6), 2793–2808. doi:10.1007/s12665-014-3585-y
Ross, Z. E., Trugman, D. T., Hauksson, E., and Shearer, P. M. (2019). Searching for hidden earthquakes in Southern California. Science 364, 767–771. doi:10.1126/science.aaw6888
Rossi, C., Grigoli, F., Cesca, S., Heimann, S., Gasperini, P., Hjörleifsdóttir, V., et al. (2020). Full-waveform based methods for microseismic monitoring operations: An application to natural and induced seismicity in the hengill geothermal area, Iceland. Adv. Geosciences 54, 129–136. doi:10.5194/adgeo-54-129-2020
Saki, M., Thomas, C., Nippress, S. E. J., and Lessing, S. (2015). Topography of upper mantle seismic discontinuities beneath the North Atlantic: The Azores, Canary and Cape Verde plumes. Earth Planet. Sci. Lett. 409, 193–202. doi:10.1016/j.epsl.2014.10.052
Schmidt, C., Hensen, C., Hübscher, C., Wallmann, K., Liebetrau, V., Schmidt, M., et al. (2020). Geochemical characterization of deep-sea sediments on the Azores Plateau – from diagenesis to hydrothermal activity. Mar. Geol. 429, 106291. doi:10.1016/j.margeo.2020.106291
Scripps Institution of Oceanography (1996). Global seismograph network - IRIS/IDA [data set]. Int. Fed. Digital Seismogr. Netw. doi:10.7914/SN/II
Senos, M. L., Nunes, J. C., and Moreira, V. S. (1980). Estudos da estrutura da crosta e manto superior nos Açores. Lisboa: INMG.
Serviço Regional de Proteção Civil e Bombeiros dos Açores (SRPCBA) (2018). Comunicado sismológico 2/2018. Available at: https://www.prociv.azores.gov.pt/alertas/ver.php?id=1012 (Accessed February 12, 2018).
Silva, R., Carmo, R., and Marques, R. (2021). Characterization of the tectonic origins of historical and modern seismic events and their societal impact on the azores archipelago, Portugal. Geol. Soc. Spec. Publ. 501 (1), 245–267. doi:10.1144/SP501-2019-106
Silva, R., Ferreira, T., Medeiros, A., Carmo, R., Luis, R., Wallenstein, N., et al. (2015). Chapter 17 seismic activity on são Miguel island volcano-tectonic structures (azores archipelago). Geol. Soc. Mem. 44 (1), 227–238. doi:10.1144/M44.17
Silva, R., Havskov, J., Bean, C., and Wallenstein, N. (2012). Seismic swarms, fault plane solutions, and stress tensors for são Miguel Island central region (Azores). J. Seismol. 16 (3), 389–407. doi:10.1007/s10950-012-9275-x
Silveira, G., Stutzmann, E., Davaille, A., Montagner, J. P., Mendes-Victor, L., and Sebai, A. (2006). Azores hotspot signature in the upper mantle. J. Volcanol. Geotherm. Res. 156 (1–2), 23–34. doi:10.1016/j.jvolgeores.2006.03.022
Silveira, G., Vinnik, L., Stutzmann, E., Farra, V., Kiselev, S., and Morais, I. (2010). Stratification of the Earth beneath the azores from P and S receiver functions. Earth Planet. Sci. Lett. 299 (1–2), 91–103. doi:10.1016/j.epsl.2010.08.021
Simanjuntak, A. V. H., Saragih, N. F., Anshori, M., Sinambela, M., Razi, A., Muksin, U., et al. (2022). A preliminary earthquake detection from the seismic temporary network in the opak fault. IOP Conf. Ser. Earth Environ. Sci. 1083 (1), 012072. doi:10.1088/1755-1315/1083/1/012072
Simon, V., Kraft, T., Diehl, T., and Tormann, T. (2021). Possible precursory slow-slip to two ML∼3 mainevents of the diemtigen microearthquake sequence, Switzerland. Geophys. Res. Lett. 48 (19). doi:10.1029/2021GL093783
Steinmetz, L., Hirn, A., Sapin, M., Whitmarsh, R., and Moreira, V. (1976). Zones of P wave attenuation beneath the crest of Mid-Atlantic Ridge. Bull. La Société Géologique Fr. 7 (4), 931–936. doi:10.2113/gssgfbull.s7-xviii.4.931
Storchak, D. A., Di Giacomo, D., Engdahl, E. R., Harris, J., Bondár, I., Lee, W. H. K., et al. (2015). The ISC-gem global instrumental earthquake catalogue (1900-2009): Introduction. Phys. Earth Planet. Interiors 239, 48–63. doi:10.1016/j.pepi.2014.06.009
Storchak, D. A., Giacomo, D. D., Bondár, I., Engdahl, E. R., Harris, J., Lee, W. H. K., et al. (2013). Public release of the ISC-GEM global instrumental earthquake catalogue (1900-2009). Seismol. Res. Lett. 84 (5), 810–815. doi:10.1785/0220130034
Vuan, A., Brondi, P., Sugan, M., Chiaraluce, L., Di Stefano, R., and Michele, M. (2020). Intermittent slip along the alto tiberina low-angle normal fault in central Italy. Geophys. Res. Lett. 47 (17). doi:10.1029/2020GL089039
Vuan, A., Sugan, M., Amati, G., and Kato, A. (2018). Improving the detection of low-magnitude seismicity preceding the Mw 6.3 L’Aquila earthquake: Development of a scalable code based on the cross correlation of template earthquakes. Bull. Seismol. Soc. Am. 108 (1), 471–480. doi:10.1785/0120170106
Weimer, S. (2001). A software package to analyze seismicity: Zmap. Seism. Res. Lett. 72 (2), 374. doi:10.1785/gssrl.72.3.373
Zhang, Q., and Shearer, P. M. (2016). A new method to identify earthquake swarms applied to seismicity near the San Jacinto Fault, California. Geophys. J. Int. 205 (2), 995–1005. doi:10.1093/gji/ggw073
Zhu, W., and Beroza, G. C. (2019). PhaseNet: A deep-neural-network-based seismic arrival-time picking method. Geophys. J. Int. 216 (1), 261–273. doi:10.1093/gji/ggy423
Zöller, G., Hainzl, S., Ben-Zion, Y., and Holschneider, M. (2006). Earthquake activity related to seismic cycles in a model for a heterogeneous strike-slip fault. Tectonophysics 423 (1–4), 137–145. doi:10.1016/j.tecto.2006.03.007
Keywords: Spatio-temporal earthquake evolution, Earthquake detection-location, waveform analysis, seismic swarm, São Miguel Island, Azores triple junction
Citation: Soares A, Custódio S, Cesca S, Silva R, Vuan A and Mendes VB (2023) The February 2018 seismic swarm in São Miguel, Azores. Front. Earth Sci. 11:1144151. doi: 10.3389/feart.2023.1144151
Received: 13 January 2023; Accepted: 19 June 2023;
Published: 10 July 2023.
Edited by:
Eleftheria Papadimitriou, Aristotle University of Thessaloniki, GreeceReviewed by:
Salvatore Gambino, National Institute of Geophysics and Volcanology (INGV), ItalyCopyright © 2023 Soares, Custódio, Cesca, Silva, Vuan and Mendes. This is an open-access article distributed under the terms of the Creative Commons Attribution License (CC BY). The use, distribution or reproduction in other forums is permitted, provided the original author(s) and the copyright owner(s) are credited and that the original publication in this journal is cited, in accordance with accepted academic practice. No use, distribution or reproduction is permitted which does not comply with these terms.
*Correspondence: A. Soares, YW5hbGR5bmUuc29hcmVzQGVkdS51bGlzYm9hLnB0
Disclaimer: All claims expressed in this article are solely those of the authors and do not necessarily represent those of their affiliated organizations, or those of the publisher, the editors and the reviewers. Any product that may be evaluated in this article or claim that may be made by its manufacturer is not guaranteed or endorsed by the publisher.
Research integrity at Frontiers
Learn more about the work of our research integrity team to safeguard the quality of each article we publish.