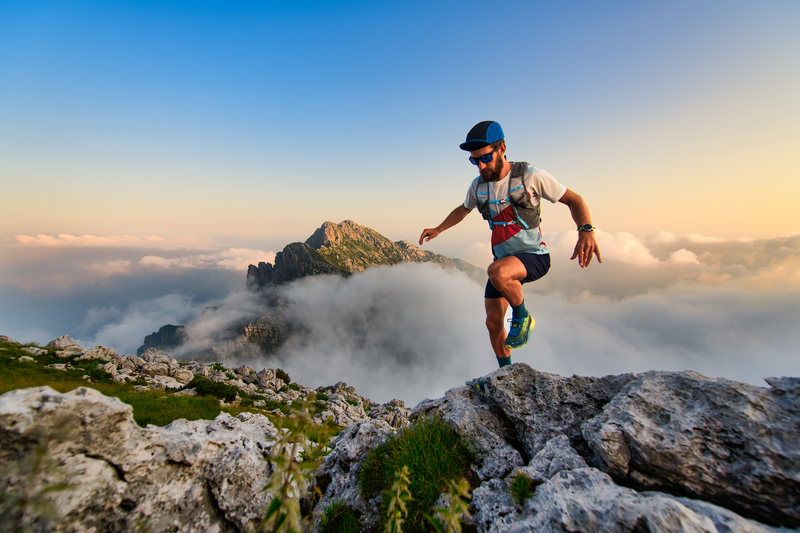
95% of researchers rate our articles as excellent or good
Learn more about the work of our research integrity team to safeguard the quality of each article we publish.
Find out more
ORIGINAL RESEARCH article
Front. Drug Discov. , 29 October 2024
Sec. In silico Methods and Artificial Intelligence for Drug Discovery
Volume 4 - 2024 | https://doi.org/10.3389/fddsv.2024.1460672
This article is part of the Research Topic Pharmacokinetics Modeling in the Artificial Intelligence Era View all 4 articles
Understanding drug–drug interactions (DDIs) plays a vital role in the fields of drug disease treatment, drug development, preventing medical error, and controlling health care-costs. Extracting potential from biomedical corpora is a major complement of existing DDIs. Most existing DDI extraction (DDIE) methods do not consider the graph and structure of drug molecules, which can improve the performance of DDIE. Considering the different advantages of bi-directional gated recurrent units (BiGRU), Transformer, and attention mechanisms in DDIE tasks, a multimodal feature fusion model combining BiGRU and Transformer (BiGGT) is here constructed for DDIE. In BiGGT, the vector embeddings of medical corpora, drug molecule topology graphs, and structure are conducted by Word2vec, Mol2vec, and GCN, respectively. BiGRU and multi-head self-attention (MHSA) are integrated into Transformer to extract the local–global contextual DDIE features, which is important for DDIE. The extensive experiment results on the DDIExtraction 2013 shared task dataset show that the BiGGT-based DDIE method outperforms state-of-the-art DDIE approaches with a precision of 78.22%. BiGGT expands the application of multimodal deep learning in the field of multimodal DDIE.
With increasing numbers of diseases and new drugs, drug combination therapy is growing in popularity. However, taking multiple drugs at the same time often causes undesired drug–drug interactions (DDIs), with adverse drug reactions (ADRs) such as headache, nausea, shock, and even death (Makiani et al., 2017). Understanding DDIs is critical to improving drug safety and efficacy to avoid the risk of ADRs before clinical combination therapy. However, the number of known DDIs is limited because laboratory and manual DDI testing is difficult, expensive, and time-consuming (Hammoud and Shapiro, 2022). Extracting potential DDIs from a biomedical corpus is a good complement to existing DDI datasets. Several DDI extraction (DDIE) methods that use drug characteristics and the biomedical corpus have better results on the known datasets, but they have some limitations (Han K. et al., 2022; Wang et al., 2024). Due to the lack of a uniform language format in the biomedical corpus, such as drug entity combinations and abbreviations, and the unsatisfactory performance of existing DDIE methods in long and complex sentences, DDIE from the biomedical corpus remains challenging (Han K. et al., 2022; Wang et al., 2024; Luo et al., 2024). BiGRU is a typical feature extractor and has been widely applied to DDIE tasks. It can extract local features efficiently, but it is less effective in capturing global features (Zhang et al., 2023). Compared to BiGRU, Transformer is another powerful feature extractor that can extract context global information by self-attention (Zaikis and Vlahavas, 2021).
Inspired by BiGRU, GCN, Transformer, and multimodal deep learning models that have achieved better DDIE (Deng et al., 2020), a hybrid deep learning model called BiGGT is here constructed for DDIE. It makes use of the medical corpus and the graph and structure of drug molecular to improve the performance of DDIE. The main contributions are described as follows.
A hybrid deep-learning model, BiGGT, is constructed to capture the local, global, and contextual features of DDIE and overcome the limitation of single-model based DDIE methods.
The graph and structure of drug molecules is utilized to improve the performance of DDIE from the medical corpus.
Integrating BiGRU into Transformer enhances its ability to aggregate local drug molecule information.
The rest of this paper is organized thus. Section 2 reviews recent advances in DDIE. Section 3 describes BiGGT for DDIE in detail. Experiments and analysis are performed in Section 4. Section 5 makes some conclusions and proposes future research.
DDIE is a fundamental task that has several important applications in clinical and drug decision making. Various DDIE methods have been recently proposed to extract the correct type of DDI between two drugs in the input biomedical corpus (Deng et al., 2020). They generally consist of two steps: drug naming entity identification and relationship extraction. We here focus on DDIE, assuming that the drug entity pair is given according to existing methods and that each drug is represented as a graph and structure of the drug molecule (Niu et al., 2024; Lin et al., 2023). For example, the risk or severity of osteomalacia can be increased when acetazolamide is combined with phenytoin. Moreover, acetazolamide can reduce phenytoin excretion, resulting in increased serum levels of the latter and increased adverse effects, including osteomalacia. Their medical corpus, chemical formula, drug molecule topology graph, and drug molecular structure are shown in Figure 1, where the molecular structure can be encoded by simplified molecular-input line-entry system (SMILES), and the nodes of the molecule topology graph are atoms and the edges are the bonds between the atoms. RDKit is used to generate a drug molecule topology graph by SMILES4, where the details of two drugs are described on Webs: acetazolamide (https://go.drugbank.com/drugs/DB00819) and phenytoin (https://go.drugbank.com/drugs/DB00252).
Figure 1. Example of medical corpus with a topology graph and structure of the drug molecule. (A) Corpus, (B) Chemical Formula, (C) Molecular graph, and (D) Molecular structure.
There have been a number of recent DDIE approaches which can be broadly divided into three categories: traditional machine learning (Han K. et al., 2022; Wang et al., 2024), deep learning (Luo et al., 2024; Zhang et al., 2023), and multimodal hybrid learning (Zaikis and Vlahavas, 2021; Deng et al., 2020).
Traditional machine-learning-based DDIE methods have three main steps: data preprocessing, feature extraction, and classification. Han K. et al. (2022) reviewed machine-learning-based DDIE approaches, including widely used datasets, multiple DDIE methods, their advantages and disadvantages, and challenges and prospects of DDIE methods. These are useful for promoting DDIE research. Wang et al. (2024) systematically reviewed the DDIE problem from three perspectives—classical DDI datasets, commonly used drug features, and popular machine-learning-based DDIE methods—summarized and compared relevant studies, and identified existing problems, potential opportunities, and future challenges and research directions.
Based on the DDIE results of these traditional machine-learning methods, it is apparent that their performance mainly relies on tedious feature engineering, and their results are generally limited because it is difficult to extract robust features from complex irregular medical texts.
Due to the ability of deep learning to learn deep-level features from the corpus, many DDIE approaches based on deep learning have been proposed. Luo et al. (2024) introduced the existing biomedical data and drug-related public databases, discussed existing DDIE methods using deep learning, and examined the knowledge graph (KG), which is divided into three categories: deep learning, KG, and a combination of deep learning and KG. Zhang et al. (2023) reviewed recent deep-learning methods applied to DDIE from the biomedical literature, briefly described each method, systematically compared their performance in the medical corpus, summarized their advantages and disadvantages, and discussed some of the challenges and future research in DDIE. This review provided useful guidance to further advance DDIE algorithms from the literature. Niu et al. (2024) proposed a DDIE method based on substructure fine-representation learning and self-attention mechanism, and designed drug-similarity features to extract potential DDIs which can improve the robustness of substructure features, determine drug properties, and thus improve DDIE performance.
Compared with traditional DDIE methods, the above deep learning-based DDIE methods have achieved remarkable results by using a large number of annotated training samples. However, it is unrealistic to annotate a large amount of training samples because this would likely be costly and time-consuming, and high-level understanding DDI requires domain knowledge on drugs and DDI.
To improve DDIE performance, drug information such as detailed drug description, molecular structure, and graph of drugs are employed for DDIE. Several multimodal DDIE methods have been presented using a variety of drug information. Zaikis and Vlahavas (2021) proposed a multimodal deep learning framework for DDIE. This framework based on multi-drug characteristics has high accuracy on the known datasets but also has some limitations. The hypothesis that drugs with similar chemical structures have similar DDI has not been scientifically tested. Therefore, in actual clinical verification, there may be a large deviation in DDIE results. Lin et al. (2023) introduced the widely used molecular representations and described the theoretical framework of graph convolutional networks (GCNs) to represent drug molecular structures, discussed potential challenges, and highlighted future directions for deep graph learning models that accelerate DDIE. Asada et al. (2021) proposed a multimodal DDIE method by effectively utilizing large-scale raw text information, drug description, and drug molecular structure information. Their results verified that this drug-related information can further improve DDIE performance. Zhao et al. (2019) proposed a multi-type feature fusion GCN (MFFGNN) for DDIE, where the intra-drug features and external DDI features are fused by GCN encoder to update drug representation, and multi-layer perceptron (MLP) is used to predict the missing DDIs in the DDI graph. Zhang et al. (2020) constructed a large-scale multimodal DDIE approach, employed four operators to represent drug–drug pairs, and adopted the random forest classifier to train the DDIE model. Huang et al. (2022) proposed a hybrid deep-learning framework for DDIE using the biomedical information of drugs. In this model, multi-drug similarities between drug substructures, targets, and enzymes and two different-level fusion strategies are combined to predict DDI events. Gan et al. (2023) proposed a multimodal feature fusion network for DDIE. They introduced an attention-gated GCN to capture the global features of the molecule topology graph and the local features of each atom and introduced sparse GCN to learn the DDIE topology information.
Transformer is a global feature extractor based on multi-head self-attention (MHSA). It has been applied to DDIE tasks. Jiang et al. (2023) constructed a TranGRU model by integrating BiGRU into Transformer. It can encode the local and global information of molecules and employs a gated mechanism to effectively fuse two molecular features. Gu et al. (2024) proposed a multimodal feature fusion-based deep learning model for DDIE by integrating the multimodal features of drug molecular structure and graphs extracted through GCN. Han X. et al. (2022) constructed a multimodal SmileGNN model for DDIE by integrating drug structural features and drug topological features.
The many approved drugs contain medical corpora and drug molecule topology graph and structure, and these data are closely related to DDI. However, the above DDI methods are rarely fully integrated for DDIE, particularly drug chemical structure. Of the above multimodal DDIE methods, BiGRU is effective at capturing local dependencies in sequences for DDIE tasks when dealing with shorter sequences, but it is not effective in extracting and fusing the multimodal DDIE dependencies. Transformer is good at capturing global information to effectively alleviate the sequence dependencies in BiGRU but weak at capturing local information from medical texts. Considering the different behaviors of BiGRU and Transformer in extracting DDI features, we aim to integrate the BiGRU into the encoder layer of the original Transformer to better capture local and global DDIE features simultaneously. The graph and structure of drug molecules are useful for further improving the performance of DDIE. Due to the complexity, irregularity, and even fuzziness of medical texts, drug descriptions, and drug molecular structure and graphs, some existing models rarely consider semantic translation from entity to relationship, and low-dimensional embeddings learned by relationship do not capture enough DDI information from drugs and the medical corpus, resulting in incorrect multidrug DDIs between drug pairs. A multimodal hybrid deep learning model BiGGT is constructed to explore the potential correlations between the multimodal features and improve DDIE performance. BiGGT focuses on modeling the local–global contextual features for DDIE by integrating BiGRU and MHSA into Transformer and can effectively learn the local and global DDIE feature representation simultaneously.
BiGGT’s framework is shown in Figure 2, consisting of the following main modules: data representation and preprocessing, vector embedding and principal component analysis (PCA) reduction, attention gating multi-modal feature fusion, Transformer and BiGRU (TransBiGRU), and DDI classification, and model training, which are introduced as follows.
Data representation and preprocessing includes negative instance filtering, drug blinding, and tokenization. Suppose n ≥ 2 drug references appear in an input sentence with
Given a sentence of biomedical corpus
In the sentence of the biomedical corpus, two molecule structures and two molecule topology graphs of drug1 and drug2 are encoded into vector embeddings—that is, low-dimensional vectors, respectively, which are extracted by a medical concept embedding algorithm.
Vector embedding of the biomedical corpus is done by Word2vec. The given input sentence
where
The word position embeddings
In the vector embedding of the drug molecular structure, SMILES2Vec is used to convert the drug molecular structure into vector space (Jiang et al., 2023). The drug molecular structure is represented by a SMILES string from the DrugBank dataset,
In the vector embedding of the drug molecule topology graph, Graph2Vec is an algorithm based on the subgraph of the graph embedding (Kim et al., 2021; Narayanan et al., 2017). Graph2Vec is a Python library developed by Benedek Rozemberczki. Its substructure updates its neighborhood information through the substructure of the adjacent nodes. Graph2vec is used to convert each molecule topology graph of drug1 and drug2 into a fixed-length vector
In PCA reduction, PCA is used to reduce the above embedding vectors by retaining 98% energy:
where
The features obtained by Equation 2 are fused by an attention mechanism by assigning learnable weights to automatically determine the importance of the features:
where
In Equation 3,
As defined in Equation 4, where
Using Equation 3, the DDIE feature embedding matrix E is obtained to integrate information from the biomedical corpus, drug molecular structure, and drug molecule topology graph. This learning fusion process enables TransBiGRU to better adapt to the DDIE task and use the information for each feature.
TransBiGRU is similar to TranGRU (Jiang et al., 2023). It is a simple, effective module that integrates BiGRU into Transformer to simultaneously learn local and global feature representations. Its structure is shown in Figure 2, having N identical encoder layers. The embedded matrix E is input into TransBiGRU, the output of the (i-1)th layer is the input of the ith layer (i = 1,2, … , N), layer normalization is performed, the output is input into the MHSA and BiGRU modules, and the final output of the top layer is the high-level feature representation for DDIE.
TransBiGRU is introduced in detail as follows. Two output states of MHSA and BiGRU with the same size are fused through the gating mechanism, and then layer normalization is performed, and the fused normalized state is sent to the feedforward layer position to generate the output of the current layer.
For the BiGRU encoder, the output
where
In MHSA, multiple single-head self-attention layers process each input vector simultaneously in parallel. The output of each single-head layer is concatenated and converted into a fixed-length vector generated using affine transformations. Single-head self-attention performs a linear transformation on each input vector using three separate matrices: query, key, and value. MHSA encodes the input E. The output
where MHSA is MHSA processing,
As shown in Equation 7, the gating mechanism is used to fuse the outputs of BiGRU Bi and MHSA Si:
where ‘◦’ is element-wise multiplication and
where
where
Like the multimodal data fusion-based deep learning approach (MMDFDL) (Huang et al., 2022), DMFDDI (Gan et al., 2023), and TranGRU (Jiang et al., 2023), BiGGT uses forward propagation to calculate the model losses, backward propagation is used to iteratively update network hyperparameters along the gradient descent direction, and cross-entropy as the loss function is used to avoid the problem of the decreasing learning rate in the process of gradient descent. Loss function is evaluated by summing the label loss of all training samples, which is defined as the negative likelihood of predicting correct labels of multiple downstream tasks
As defined in Equation 10, where M is the number of training samples and L is the number of DDI types.
The BiGGT-based DDIE-based method is evaluated on the DDIExtraction-2013 shared task (SemEval-2013 Task 9.2) (https://aclanthology.org/S13-2056/) and compared with baselines and state-of-the-art DDIE-based deep learning: DBGRU-SE (Zhang et al., 2023), TP-DDI (Zaikis and Vlahavas, 2021), drug descriptions and molecular structures (DDMS) (Asada et al., 2021), and multi-type feature fusion based on GNN (MFFGNN) (He et al., 2022). BiGRU and Transformer are adopted as the baselines (Zhao et al., 2019; Su and Qian, 2024), Transformer is the standard Transformer model, and the layer depth of Transformer is set to 3, equal to the layer-depth of TransBiGRU. Four comparative methods are briefly introduced as follows.
DBGRU-SE: a DDIE method that combines double BiGRU and SE-attention mechanism.
TP-DDI: a Transformer-based pipeline for DDIE that utilizes the powerful semantic understanding ability of Transformer to effectively extract DDI features from biomedical text.
DDMS: uses CNNs and GNNs to integrate drug description information and molecular structure information for DDI extraction.
MFFGNN: a multi-type feature fusion model that integrates topological information in drug molecular graphs, drug interaction information, and local molecular structure in SMILE strings.
The DDIExtraction-2013 dataset consists of texts annotated with drug mentions, drug molecular structure, drug molecular graphs, and their DDIs from DrugBank and MEDLINE, with 792 annotated documents from DrugBank and 233 abstracts with drug mentions and their relationships from MEDLINE (Isabel et al., 2013; Shanbhag et al., 2021). This dataset contains more than 10,000 drugs, 4,997 positive DDI instances, and 24,893 negative DDI instances, of which all positive DDI instances are divided into five DDI types: Advice, Mechanism, Effect, Int, and Negative. They are explained as follows.
Mechanism: DDI type used to annotate the pharmacokinetic mechanism of two drugs.
Effect: DDI type used to annotate DDIs describing an effect or a pharmacodynamic mechanism.
Advice: DDI type used when a suggestion or advice regarding a drug interaction is given.
Int: DDI type used to annotate DDIs appearing in text without any additional information.
Negative: DDI type used to indicate no DDI between two drugs.
All instances are divided into training sets for model training and test sets for model testing. The detailed statistics of DDI types and the DDI instance distribution are shown in Table 1 and Figure 3, respectively.
Figure 3. DDI instance distribution. (A) Original distribution of positive and negative samples. (B) Filtered distribution of positive and negative samples. (C) Original distribution of positive samples.
As seen from Table 1 and Figure 3, there is a serious imbalance between positive and negative instances in the dataset. The number of negative instances far exceed positive instances, the negative proportion is more than 86%, the positive proportion is less than 14%, and the positive instance set is also unbalanced, with the proportion of Int at only 6%—significantly less than that of other types. The unbalanced data distribution often results in a bias in the model training and classification result, making the model learn more features from negative instances while ignoring features from positive instances. Some rules are usually used to filter out possible negative instances (Zhang et al., 2023; Deng et al., 2020; Lin et al., 2023). The distribution of the filtered instances is shown in Table 2 and Figure 3B, where it can be seen that the unbalanced distribution problem may be significantly alleviated. The distribution of the filtered positive instances is the same as that of the original positive instances (Figure 3C).
DDIE is a multi-type classification problem for extracting the DDIs in an input sentence. It consists of named entity recognition and DDIE. This study aims to extract DDIs, assuming drug entities given in accordance with existing methods (Su and Qian, 2024; Liu et al., 2016). BiGGT is evaluated on the filtered dataset in Table 2.
Like other multimodal deep learning models (Zhang et al., 2020; Huang et al., 2022; Han X. et al., 2022), BiGGT has several trainable hyperparameters that are usually set by the Xavier (Han X. et al., 2022). The initial experimental parameters of BiGGT are set as follows.
The embedding dimensions of the word and position embedding vector are 300 and 20, respectively—learning rate to 0.001, batch-size to 200, max. length of sentence to 200, number of iterations to 3,000, and the layer-depth of TransBiGRU is 3. SMILES2Vec is used to preprocess SMILES sequences of drug molecules, where the embedding size is 64 and the number of heads in multi-head attention is 2. The open-source package RDKit is employed to construct the drug molecular graph G based on the SMILES sequence. The Adam optimizer and binary cross-entropy loss function are used to train the model and optimize the DDIE component using the default parameters. In the hidden layers, the batch normalization layer is used to accelerate convergence, and the dropout layer is used to avoid overfitting and improve generalization; the dropout rate is set to 0.1.
NLTK (natural language toolkit) is a natural language processing tool set based on Python. Its functions “nltk.sent_tokenize ()” and “nltk.word_tokenize ()” are used to preprocess the sentences in the dataset, including sentence splitting, sentence tokenizing, converting all words to lowercase, and replacing all digits by a special token “dg” by regular expressions (Gu et al., 2024; Han X. et al., 2022). The pretrained GloVe and Stanford parser tools (https://nlp.stanford.edu/software/parser-faq.html) are used for word vector embedding (Kudo and Richardson, 2018). The experiments are performed on the given train–test distribution and by five-fold cross-validation (5FCV). The experimental environment configuration is shown in Table 3.
The evaluation metrics of average results of precision (P), recall (R), and F1-score (F1) are used to evaluate the model performance, calculated as shown in Equation 11:
where SDDI = {Advice, Mechanism, Effect, Int, Negative} is the DDI label set, and the Pl and Rl of each instance l∈SDDI are evaluated by the calculation formula as shown in Equation 12.
BiGGT is implemented by using different parameter combinations on the given filtered dataset, and the optimized parameters are obtained when the best prediction is achieved. The average results of precision (P), recall (R) and F1-score (F1) are calculated by Equation 11. To estimate the performance of BiGGT-based DDIE, Figure 4 shows its overall training loss of precision versus the iterations on the filtered training set with the given default parameters, compared to using BiGRU-GCN (Zhao et al., 2019) and Transformer (Su and Qian, 2024) as baseline methods.
It is seen from Figure 4 that the losses of three methods decrease rapidly before 1,000 iterations and tend to be stable and close to convergence after about 2,500 iterations. Because there are some fluctuations when increasing the training iteration, their overall performance can be still improved. It is also found that the training performance of BiGGT is better than BiGRU-GCN’s and Transformer’s, and its training curve is relatively smooth with fewer fluctuations during training, indicating that BiGGT has better stability and fast convergence. From Figure 4, the trained model is selected when the number of iterations is 3,000.
To further evaluate the impact of important parameters on prediction performance, different values are assigned to them to evaluate the performance of BiGGT on the filtered dataset. In BiGGT, the word embedding dimension of the medical corpus is an important parameter. To determine the optimal embedding dimension, different embedding dimensions of 50, 100, 200, 300, 350, and 400 are selected for DDIE. The precision and training time on the given train–test distribution set are shown in Table 4.
From Table 4, it is found that by increasing the size of word embeddings, the precision increases while the training cost increases. The precision of the 350 and 400 dimensions are slightly higher than that of the 300 dimensions, but its training time is much larger. According to the trade-off between precision and training time, the embedding dimension is set as 300, retaining the larger precision and less training time.
By fixing other parameters, we evaluate the settings of word position embedding size and drug molecular embedding size on the performance of BiGCN. The precisions versus two parameter settings are shown in Figure 5. As shown in Figure 5A, the word position embedding size and drug molecular structure embedding size are set to 25 and 90, respectively.
Figure 5. Precision versus two parameter settings. (A) Word position embedding size. (B) Drug molecular structure 368 embedding size.
As the dimensions of the drug embedding feature increase, BiGCN can extract useful information. However, dimensions that are too high can increase noise and cause performance degradation. PCA is used to reduce the dimension of the extracted features, and the effect of the dimension reduction in the process of retaining energy from 99% to 90% is shown in Figure 6.
As can be seen from Figure 6, when the features are further reduced, the information of DDIE may be greatly lost, thus affecting the performance of the model. The features are uniformly reduced by retaining 98% of the energy.
In BiGGT, the number of layers of TransBiGRU is another key parameter. Five different layer depths from 1 to 6 are selected to determine the optimal layer depth. The precision and training time of BiGGT versus six layer depths are shown in Table 5. From Table 5, according to the trade-off between precision and training time, the suitable layer-depth is set as 3.
To estimate the influence of data imbalance on the experimental results, two DDIE experiments are conducted on the filtered ddiextracaction2013 dataset, respectively with no negative instances and with negative instances. The experimental results are shown in Table 6.
From Table 6, it is known that a large number of negative instances seriously affect the results of DDIE, and the experimental results with negative instances in the recall rate (36.24%) and F1 (49.25%) of Int are lower than those of other categories. This is because the small number of Int instances results in insufficient model training. On the contrary, the negative class obtains the highest precision, recall, and F1 of the five DDI categories.
The following experiments are carried on the positive instance set. The performance of BiGGT is compared with the baseline methods BiGRU and Transformer and four DDIE methods: DBGRU-SE (Zhang et al., 2023), TP-DDI (Zaikis and Vlahavas, 2021), DDMS (Asada et al., 2021), and MFFGNN (He et al., 2022). Their hyperparameters, including batch size, regularization rate, number of hidden units, dropout rate, and learning rate, are fine-tuned and optimized by a 5FCV experiment—a common machine-learning model evaluation scheme. The average results by 5FCV experiment are given in Table 7, where BiGGT outperforms the other models.
To further test the feasibility and generalization of BiGGT, many variants of it are used for DDIE. Their precisions are shown in Table 8.
From Table 8, it is found that BiGGT is superior to its variants in terms of precision, where BiGRU is better than BiRNN and BiLIST, validating that BiGRU and Softmax can improve precision. Using PCA to reduce feature dimensionality and noise can improve the performance of model training and DDIE. From Table 8, the results show that LSTM is slightly better than RNN, and RNN and LSTM are both very low. This is because RNN cannot remember what it learns in longer sequences, so its memory is short-term. LSTM is a variant of RNN that can overcome gradient disappearance and short-term memory problems. BiRNN (or BiLSTM) can capture the past and future context of input elements by processing forward and backward sequential data using two independent RNN (or LSTM) networks. In BiLSTM, the lower-level LSTM state models the local information inside atomic groups, and the higher-level LSTM state captures the semantic information. Softmax is better than MLP because the Softmax classifier is a special neural network structure with only one hidden layer and uses the Softmax activation function to calculate the probability distribution of the class.
To further test the importance of the embedding features, many ablation experiments are conducted versus different embedding feature combinations. Their precisions and training time are shown in Table 9.
Table 9 shows that word embedding, drug molecular-structure embedding, and drug molecular-graph embedding are useful for DDIE. Word embedding contributes more to DDIE. Drug molecular-structure and graph embedding is better than word position embedding. In drug molecular-structure embedding and drug molecular-graph embedding, it is more appropriate to choose one of the two. It is also found that integrating all embedding features can improve the performance but that the training time and test time are greatly increased. The results verify that a single drug profile is not a comprehensive representation of drug information and will affect prediction results, but the more features that are used, the longer the training and testing time of the model and the corresponding increase in computation time.
From the above experimental results of Figures 4 and 5 and Tables 4–9, it is apparent that BiGGT is effective for DDIE and outperforms other methods. This is because its multi-modal feature fusion layer makes use of the embedding features of the medical corpus, drug molecular topological structure, and graph information to comprehensively represent drug information, and its TransBiGRU layer can extract the contextual semantic relationships of these embedding features, which can improve DDIE results. From Table 8, it is seen that multi-modal feature fusion, MHSA, and BiGRU are three key components of BiGGT which can be used to extract local and global features and their contextual relationship. Table 9 demonstrates that the global features of drug molecular structure and graph are important, and that BiGGT performs better than methods that only use one type feature.
Considering the different advantages of BiGRU and Transformer in extracting DDIE features, the multimodal feature fusion model BiGGT is constructed for DDIE using the medical corpus, drug molecule topology structure, and graph information. It integrates BiGRU into Transformer, where BiGRU is used to extract the local features while MHSA is used to capture global features. Combining both local and global features is effective for DDIE. The results of the DDIExtraction 2013 shared task dataset validate that BiGGT can effectively integrate information from the medical corpus, drug molecular structure, and topology graph to improve the DDIE performance of the model.
The original contributions presented in the study are included in the article/Supplementary Material; further inquiries can be directed to the corresponding author.
CY: writing–original draft and writing–review and editing. SZ: writing–original draft and writing–review and editing. XW: writing–original draft and writing–review and editing. TS: writing–original draft and writing–review and editing. CJ: writing–original draft and writing–review and editing. SL: writing–original draft and writing–review and editing. GM: writing–original draft and writing–review and editing.
The authors declare that financial support was received for the research, authorship, and/or publication of this article. This work is partially supported by the National Natural Science Foundation of China (Nos 62172338 and 62072378).
The authors would like to thank all editors and reviewers for their constructive advice.
The authors declare that the research was conducted in the absence of any commercial or financial relationships that could be construed as a potential conflict of interest.
All claims expressed in this article are solely those of the authors and do not necessarily represent those of their affiliated organizations, or those of the publisher, the editors, and the reviewers. Any product that may be evaluated in this article, or claim that may be made by its manufacturer, is not guaranteed or endorsed by the publisher.
Asada, M., Miwa, M., and Sasaki, Y. (2021). Using drug descriptions and molecular structures for drug–drug interaction extraction from literature. Bioinformatics 37 (12), 1739–1746. doi:10.1093/bioinformatics/btaa907
Deng, Y., Xu, X., Qiu, Y., Xia, J., Zhang, W., and Liu, S. (2020). A multimodal deep learning framework for predicting drug-drug interaction events. Bioinformatics 36 (15), 4316–4322. doi:10.1093/bioinformatics/btaa501
Gan, Y., Liu, W., Xu, G., Yan, C., and Zou, G. (2023). DMFDDI: deep multimodal fusion for drug-drug interaction prediction. Brief. Bioinform 24 (6), bbad397. doi:10.1093/bib/bbad397
Gu, X., Liu, J., Yu, Y., Xiao, P., and Ding, Y. (2024). MFD–GDrug: multimodal feature fusion-based deep learning for GPCR–drug interaction prediction. Methods 223, 75–82. doi:10.1016/j.ymeth.2024.01.017
Hammoud, A., and Shapiro, M. D. (2022). Drug interactions: what are important drug interactions for the most commonly used medications in preventive cardiology? Med. Clin. North Am. 106 (2), 389–399. doi:10.1016/j.mcna.2021.11.013
Han, K., Cao, P., Wang, Y., Xie, F., Ma, J., Yu, M., et al. (2022a). A review of approaches for predicting drug-drug interactions based on machine learning. Front. Pharmacol. 12, 814858. doi:10.3389/fphar.2021.814858
Han, X., Xie, R., Li, X., and Li, J. (2022b). SmileGNN: drug-drug interaction prediction based on the SMILES and graph neural network. Life 12 (2), 319. doi:10.3390/life12020319
He, C., Liu, Y., Li, H., Zhang, H., Mao, Y., Qin, X., et al. (2022). Multi-type feature fusion based on graph neural network for drug-drug interaction prediction. He al. BMC Bioinforma. 23, 224. doi:10.1186/s12859-022-04763-2
Huang, A., Xie, X., Wang, X., and Peng, S. (2022). A multimodal data fusion-based deep learning approach for drug-drug interaction prediction. Lect. Notes Comput. Sci. 13760, 275–285. doi:10.1007/978-3-031-23198-8_25
Isabel, S., Paloma, M., and Mar´ıa, H. (2013). “SemEval-2013 task 9: extraction of drug-drug interactions from biomedical texts (DDIExtraction 2013),” in Second joint conference on lexical and computational semantics (*SEM), seventh international workshop on semantic evaluation (SemEval 2013), 341–350.
Jiang, J., Zhang, R., Ma, J., Liu, Y., Yang, E., Du, S., et al. (2023). TranGRU: focusing on both the local and global information of molecules for molecular property prediction. Appl. Intell. 53, 15246–15260. doi:10.1007/s10489-022-04280-y
Kim, H., Lee, J., Ahn, S., and Lee, J. R. (2021). A merged molecular representation learning for molecular properties prediction with a web-based service. Sci. Rep. 11, 11028. doi:10.1038/s41598-021-90259-7
Kudo, T., and Richardson, J. (2018). “SentencePiece: a simple and language independent subword tokenizer and detokenizer for neural text processing,” in Conference on empirical methods in natural language processing: System demonstrations, 66–71. doi:10.18653/v1/D18-2012
Lin, X., Dai, L., Zhou, Y., Yu, Z. G., Zhang, W., Shi, J. Y., et al. (2023). Comprehensive evaluation of deep and graph learning on drug-drug interactions prediction. Brief. Bioinform 24 (4), bbad235. doi:10.1093/bib/bbad235
Liu, S., Tang, B., Chen, Q., and Wang, X. (2016). Drug-drug interaction extraction via convolutional neural networks. Comput. Math. Methods Med. 2016, 6918381–6918388. doi:10.1155/2016/6918381
Luo, H., Yin, W., Wang, J., Zhang, G., Liang, W., Luo, J., et al. (2024). Drug-drug interactions prediction based on deep learning and knowledge graph: a review. iScience 27 (3), 109148. doi:10.1016/j.isci.2024.109148
Makiani, M., Nasiripour, S., Hosseini, M., and Mahbubi, A. (2017). Drug-drug interactions: the importance of medication reconciliation. J. Res. Pharm. Pract. 6 (1), 61–62. doi:10.4103/2279-042X.200992
Narayanan, A., Chandramohan, M., Venkatesan, R., Chen, L., Liu, Y., and Jaiswal, S. (2017). Graph2vec: learning distributed representations of graphs. arXiv:1707.05005. doi:10.48550/arXiv.1707.05005
Niu, D., Xu, L., Pan, S., Xia, L., and Li, Z. (2024). SRR-DDI: a drug–drug interaction prediction model with substructure refined representation learning based on self-attention mechanism. Knowledge-Based Syst. 285 (15), 111337. doi:10.1016/j.knosys.2023.111337
Shanbhag, S. V., Karmakar, P., Prajwala, P., and Patil, N. (2021). “Drug-drug interaction extraction based on deep learning models,” in Advances in intelligent systems and computing (Singapore: Springer), 1392. doi:10.1007/978-981-16-2709-5_53
Su, J., and Qian, Y. (2024). DDI-Transform: a neural network for predicting drug-drug interaction events. Quant. Biol. 12 (2), 155–163. doi:10.1002/qub2.44
Wang, N., Zhu, B., Li, X., Liu, S., Shi, J. Y., and Cao, D. S. (2024). Comprehensive review of drug-drug interaction prediction based on machine learning: current status, challenges, and opportunities. J. Chem. Inf. Model 64 (1), 96–109. doi:10.1021/acs.jcim.3c01304
Zaikis, D., and Vlahavas, I. (2021). TP-DDI: transformer-based pipeline for the extraction of drug-drug interactions. Artif. Intell. Med. 119, 102153. doi:10.1016/j.artmed.2021.102153
Zhang, M., Gao, H., Liao, X., Ning, B., Gu, H., and Yu, B. (2023). DBGRU-SE: predicting drug-drug interactions based on double BiGRU and squeeze-and-excitation attention mechanism. Brief. Bioinform 24 (4), bbad184. doi:10.1093/bib/bbad184
Zhang, Y., Qiu, Y., Cui, Y., Liu, S., and Zhang, W. (2020). Predicting drug-drug interactions using multi-modal deep auto-encoders based network embedding and positive-unlabeled learning. Methods 179, 37–46. doi:10.1016/j.ymeth.2020.05.007
Keywords: drug–drug interaction, DDI extraction, graph convolutional networks, transformer, multimodal feature fusion
Citation: Yu C, Zhang S, Wang X, Shi T, Jiang C, Liang S and Ma G (2024) Drug–drug interaction extraction based on multimodal feature fusion by Transformer and BiGRU. Front. Drug Discov. 4:1460672. doi: 10.3389/fddsv.2024.1460672
Received: 06 July 2024; Accepted: 08 October 2024;
Published: 29 October 2024.
Edited by:
Patric Schyman, Eikon Therapeutics, Inc., United StatesReviewed by:
Vishal Siramshetty, Genentech Inc., United StatesCopyright © 2024 Yu, Zhang, Wang, Shi, Jiang, Liang and Ma. This is an open-access article distributed under the terms of the Creative Commons Attribution License (CC BY). The use, distribution or reproduction in other forums is permitted, provided the original author(s) and the copyright owner(s) are credited and that the original publication in this journal is cited, in accordance with accepted academic practice. No use, distribution or reproduction is permitted which does not comply with these terms.
*Correspondence: Changqing Yu, eGF5Y3FAMTYzLmNvbQ==
Disclaimer: All claims expressed in this article are solely those of the authors and do not necessarily represent those of their affiliated organizations, or those of the publisher, the editors and the reviewers. Any product that may be evaluated in this article or claim that may be made by its manufacturer is not guaranteed or endorsed by the publisher.
Research integrity at Frontiers
Learn more about the work of our research integrity team to safeguard the quality of each article we publish.