- Digital Humanities Laboratory (DHLAB), Ecole Polytechnique Fédérale de Lausanne, Lausanne, Switzerland
Big Data is not a new phenomenon. History is punctuated by regimes of data acceleration, characterized by feelings of information overload accompanied by periods of social transformation and the invention of new technologies. During these moments, private organizations, administrative powers, and sometimes isolated individuals have produced important datasets, organized following a logic that is often subsequently superseded but was at the time, nevertheless, coherent. To be translated into relevant sources of information about our past, these document series need to be redocumented using contemporary paradigms. The intellectual, methodological, and technological challenges linked to this translation process are the central subject of this article.
Introduction
The future of cultural heritage in the digital era goes beyond the technical questions related to the digitization of objects and documents. Mass digitization of archival documents has begun, and although many challenges remain in these research areas, one of the most important questions from a cultural point of view is how to extract and articulate information structures out of their digital surrogates (Kaplan, 2015). How far can we apply the logic of contemporary datasets to redocument large corpora of information produced several centuries ago? For instance, would it be possible to reconstruct social networks for certain periods of the past with the same information density we experience in social networks of the present? Along that same line of thinking, would it be possible to add a slider to a contemporary geographical information system interface and look at a particular place as if it were 5, 50, or even 500 years ago? Does enough data of the past exist to realize such applications? Or are these just anachronistic questions, a common form of “presentism” (Hull, 1979; Hartog, 2003; Bourne, 2006)?
This article introduces and discusses the concept of Big Data of the Past. It is based on the hypothesis that data bigness is relative and that history is punctuated by several Big Data moments which are characterized by a widespread, shared sense of information overload alongside rapid societal acceleration accompanied by the invention of new intellectual technologies. To be translated into relevant sources of information about our past, datasets produced in these moments of acceleration need to be remodeled and reinterpreted. To progress in our understanding of these redocumentation processes, the article introduces six intermediary concepts: data bigness, data acceleration regimes, regulated representations, inferred patterns, and fictional spaces. The following sections introduce and discuss each of these.
Data bigness in Datafication Processes
The term Big Data is associated with two foundational narratives, both of which present it as an epochal paradigm shift. In the data deluge narrative, Big Data is a reaction to an unexpected abundance of information. In the Big Science narrative, Big Data is a structured effort by the international scientific community to crack very hard problems by joining research forces and creating large-scale infrastructures. Both narratives contribute to structuring a multifaceted definition of the bigness of Big Data.
In the data deluge narrative, Big Data is born out of the possibilities of the Internet and digital communication networks. In the last decade, several companies and research groups realized that fluxes of real-time information that irrigate endlessly growing worldwide information systems have the potential to constitute an original knowledge base for understanding the present and anticipating the future (Boyd and Crawford, 2012). From that perspective, Big Data research essentially tries to convert these fluxes into structured knowledge systems that document the lives of people, companies and institutions, aggregating information about places, topics, or events. The resulting knowledge systems are coded in machine-readable formats, facilitating data mining and exchanges. This general transformative process has been called datafication by Mayer-Schönberger and Cukier (2013) (Chapter 5). In such a perspective, Big Data research is the science behind massive datafication.
Interest in Big Data has another—slightly older—origin that is connected to the constitution and management of very large scientific archives, what has been called Big Science. In this narrative Big Data, methodologies were initially pioneered in some domains of life sciences, climatology, astronomy, and physics. The Human Genome Project, starting with its creation in 1989 to its achieved target in 2001, paved the way for large-scale collaborative research infrastructures and experimented in publishing the resulting data sets and results (Cantor and Smith, 1999; Lander et al., 2001). The massive data produced by CERN required the construction of new software and hardware systems (Armstrong et al., 1994). International attempts to model and simulate the brain based on massive curated experimental data revealed new challenges in the link between measures and simulation (Markram, 2006; Markram et al., 2015). The Big Science narrative insists on tackling the challenge of organizing collaborations on an international level despite academic competition, designing information pipelines to harness the massiveness of the data produced and on the relevant use of algorithmic simulation to test the coherence of the data and extrapolate for making new predictions.
Big Data research is multifaceted possibly because of its dual origins, combining features from both massive datafication and large-scale science. This is why it can be defined in different ways that are not always fully compatible. Below is a list of some complementary perspectives on the bigness of Big Data.
• Technological perspective: Big Data is big in the sense that it “hurts” to compute it using traditional “manual” methods. Its bigness calls for new strategies of processing and interpretation. The “envisioned” data volume needs special storage and computing infrastructure to be managed (Berman et al., 2003). Such data-intensive computing infrastructures include, for instance, large clusters and parallelization algorithms (Dean and Ghemawat, 2008). In turn, such technological progress opens the way for storing and computing even more data.
• Open-endedness perspective: Big Data is big when it is in a state of continuous open-ended expansion (Mayer-Schönberger and Cukier, 2013). Large-scale databases of book scans are in perpetual extension (Jacquesson, 2010), photos uploaded on social networks constitute ever-growing datasets, and the volume of micro-messages sent per day keeps rising. This calls for iterative methodologies, different from the ones adapted to close datasets. From the perspective of Big Data, every dataset tends to become a data stream (i.e., a part of the data deluge).
• Relational perspective: Big Data is big not only because of its size but because of its relationship with other data and its “fundamentally networked” nature (Boyd and Crawford, 2011). The semantic web approach that hypothesizes the existence of a Giant Global Graph, a machine-readable version of the information contained on the World Wide Web, is a typical example of such kind of interlinked datasets (Berners-Lee et al., 2006).
• Paradigmatic perspective: Big Data is big when there is sufficient data to perform new forms of data-driven sciences. It is currently being debated how massive research into patterns or correlations in large databases could replace hypothetic-deductive and model-based approaches (Hey et al., 2009). For instance, by relying on massive amount of examples, translations could potentially be done without any grammatical models, and species could be identified by their genomic signature without knowing much biology.
The central challenge for evaluating the bigness of data in these four dimensions is to invent quantitative measures adapted to these different perspectives. Technological performance can certainly be plotted in some coherent charts, even if technological disruptions at times impose new metrics. Likewise, data streams can be measured, like any fluxes, as number of data units per unit of time. The relational nature of the data can be assessed using measurements from graph theory. It is undoubtedly the paradigmatic perspective which is the most difficult to quantify. As Thomas Kuhn famously argues, paradigms are incommensurable between themselves by definition. However, paradigm shifts can also happen gradually, and therefore, it might be possible to invent distances to measure this fourth kind of bigness. Defining such measures is a crucial step in segmenting acceleration moments in data production both spatially and chronologically.
Data Acceleration Regimes
The four dimensions of data bigness are intrinsically related. Technology (e.g., more computing power, increase in communication speed) enables Big Data by making it possible to produce open-ended streams of data. This new data stream, operating in a newly standardized environment, facilitates the creation of new relations with one another. Out of the opportunities created from these network sets of new data emerges a new relationship to knowledge, leading in some cases to a paradigm shift. This self-reinforcing loop creates what can be called, a data acceleration regime (Figure 1).
Data acceleration regimes are not unique to contemporary massive datafication; rather, they echo other moments in history. In Mesopotamia, a large empire developed standardized administrative rules to cope with the new complexity of good circulation and population management. The invention of a new writing and accounting technology enabled the standardization of data streams materially embedded in clay tablets. The resulting information systems gave birth to an early science of planification that surely played a key role in the long-lasting power of these empires (Pettinato, 1981).
Likewise, The Roman Empire’s need to unify the circulation of goods, person, and information, and to exercise societal and military coordination over an extremely vast territory gave birth to additional forms of writing technologies and record-event handling methods. Standardized information started to spread all over the Empire, from the Mediterranean region to the territories now comprising Great Britain and Germany. The resulting paradigm shifts in terms of global governance marked a watershed in information management (Garnsey and Saller, 1987).
Data acceleration regimes generally start with an initial societal stress. This can, for instance, occur when a governing entity needs to cope with an unexpected, intrinsic, and complex evolution of its territory or social structures, or in the case of unexpected encounters with new populations and cultures. Acceleration starts when a new technology enables massive data production that follows regular patterns by defining specific production constraints (e.g., administrative rules, printing industries, scientific experiments).
For Europe, one classically discussed case of “acceleration” is the Renaissance, linked with the rise of the printing industry, the discovery of Asia and the Americas, and the globalization of exchanges all over the world. Not only were new editions of ancient texts starting to be printed and circulated but also a deluge of “how-to books” explaining previously secret arts and methods (Blair, 2003, 2010; Rosenberg, 2003; Gleeson-White, 2013). This sudden increase in knowledge and exposure to new practices created a well-documented feeling of information overload: there was definitely “too much to know” (Blair, 2010; Rosa, 2013). Likewise, the discovery of new species in Asia and in the Americas challenged the capacity of scholars to recognize and classify natural beings (Ogilvie, 2003). Organizing the steady stream of new species was an extremely demanding endeavor that called for new intellectual paradigms. Eventually, the globalization of monetary exchanges and the increased complexity of trade networks challenged the traditional methods for tracking commercial processes and advanced the rise of more mathematically sound, standardized methods (Gleeson-White, 2013).
From a technological perspective, the Renaissance and early modern period were intrinsically linked with the invention of several intellectual technologies for search and retrieval: indexes, bibliographies, accounting tables, and hierarchical collection structuring methods (de Vivo, 2010; Robert, 2010) in addition to chronologies and maps (Rosenberg and Grafton, 2012). From an open-ended perspective, the acceleration of exchanges, the rise of the printing press industry, and the early attempts to conduct experimental science contributed to producing streams of new data. From a relational perspective, both early modern collections, which attempted to create a system for organizing natural and artificial entities (Findlen, 1996), and the double accounting system, which enabled a new tractability of economic exchanges at the global level (Gleeson-White, 2013), helped advance the fundamentally network nature of the new datasets. Eventually, from the paradigmatic perspective, the early modern episteme reframed entire views of the world both past and present into new coherent systems of knowledge, introducing, for instance, tree-based genealogical approaches in early natural history or philology (Foucault, 1966).
Following the Big Data multifaceted criteria we previously introduced, the Renaissance and early modern periods qualify as data acceleration regimes, even if the size of the datasets managed seem small compared to contemporary standards. These epochs have produced datasets structured using the specific intellectual technologies and following the epistemic paradigms of their time. These datasets, if interpreted correctly, could be precious for reconstructing entire systems of knowledge.
From the antique administrative structures to the new information logics (cadaster, census) that accompanied the industrial revolution in the nineteenth century, how many data acceleration regimes can be identified? Can we clearly segment them? Are they more easily identifiable by their enabling technology, by the volume of data they produced, by the new connections they enabled, or by the intellectual shift they introduced? Developing methods for mapping data acceleration regimes in space and time is a crucial challenge for reconstructing Big Data of the past. This global data census could take the form of a digital historical atlas, thereby reconstructing—from a distance—great as well as minor moments in the world’s information history.
Archeological methods, such as studying material traces, can reveal complex spatial structures and symbolic practices that help in formulating hypotheses on the organization of societies. Likewise, microhistorical methods focusing on small-scale investigations develop approaches that can be used even in situations where limited documentation is available. Stressing the importance of exploiting documentation produced during data acceleration regimes does not diminish the relevance of other historical methods. On the contrary, it is clear that both archeological or microhistorical approaches, to offer two examples, would greatly benefit from the systematic spatiotemporal mapping of administrative documentation. More generally, the reconstructed data streams could be used in various forms of historical modeling and are not tied to a particular methodology. However, in order to be fully exploited, data produced during acceleration regimes needs specific conceptual keys and proper interpretative methods.
Regulated Representations
In contemporary Syria, 60 km south of Aleppo, in the now totally destroyed ancient city of Ebla, 17,000 argyle tablets and fragments were discovered (Figure 2). The tablets written in Sumerian and Eblaite constitute an antique administrative archive documenting with precision the life of this city, which was one of the most powerful of this region between 2500 and 2400 BC. This ancient information system provides a valuable example of how massive information about economic, diplomatic, and commercial exchanges were recorded and used several millennia ago (Pettinato, 1981).
In Rome, the Annales Maximi (Figure 3), 80 books from 400 BC to 130 BC, were the products of a very different early recording machine for capturing streams of events. The Pontifex Maximus, chief priest of the Capitoline, systematically maintained a detailed record of key public events, including the names of the involved magistrates and other important events such as famines, battles, extraordinary phenomena, and treaties (Frier, 1999). Contrary to the lightweight and easily erasable argyle tablets—facilitating information management, accumulation and control—the Annales engraved, locally and in a stable manner, information that could resist centuries of wear.
Probably elaborated in reaction to the encounter with other languages (for example, with the invasion of Darius in 520) and the need to define normative rules for religious Vedic texts, the Panini Sanskrit grammar (Figure 4) elaborated in the fourth century BCE contains about 4,000 grammatical rules, providing not only an extremely early historical linguistic account of the shape of this language but also a formal system for describing languages in general using compact logical rules (Missra, 1966; Kiparsky, 1979).
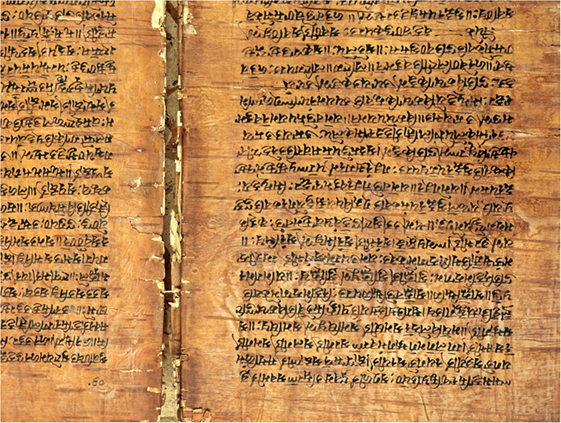
Figure 4. Manuscript on birch bark: the Rupavatra, a grammatical textbook based on the Sanskrit grammar of Panini, Kashmir, 1663, London, Wellcome Library.
The common trait of all these ancient recording technologies—beyond their differing physical materialities—is their capacity to deal with an open-ended stream of information and reorganize it to fit a given information paradigm, creating new relations between them. We can call them regulated representations (Kaplan, 2012). A representation is a man-made material document that stands for something else, typically a complex, highly dimensional event or phenomenon. For instance, a photographic picture of a scene, a sculpture of a Greek hero, a theatrical play, or a novel is man-made representations. A regulated representation is a particular case of representation governed by a set of production and usage rules. These rules can be intrinsically embedded in the production process of the representation or the result of cultural conventions. Examples of regulated representation include indexes of names, accounting tables, family trees, flow-chart diagrams, formal processes, and maps of a region. On the contrary, the production of a sculpture, a painting, or a theatrical play is generally too weakly regulated by conventional rules to be considered an example of a regulated representation. This notion is inspired by the concept of intellectual technology developed by Pascal Robert in Mnémotechnologies (Robert, 2010).
There are obviously different qualitative levels of regulation rules. Maps are good examples of how regulation and production rules progressively structure themselves over time. Modern conventions when creating a map, such as the indication of scale and the direction of North, were progressively introduced over time; the associated reading skills (how to handle a map, how to interpret its convention) developed in parallel.
Regulated representations become more regular over time (Kaplan, 2012). The general process of this regulating tendency involves the transformation of conventions into mechanisms. The regulation usually proceeds in two consecutive steps, mechanizing first the representation’s production rules and then its conventional usages. Ultimately, through this process, regulated representations tend to become machine readable.
In the case of maps, the mechanization process is begun by a progressive formalization of the recording, gathering, storage, and unification of geographic information. Early measurement methods using instruments such as the tabula praetoriana opened the way to automated triangulation processes (Figure 5). This corresponds to the mechanization of conventional production rules. Paper maps were still produced, sharing similarities with those of the previous generation, but they were made in a completely different manner.
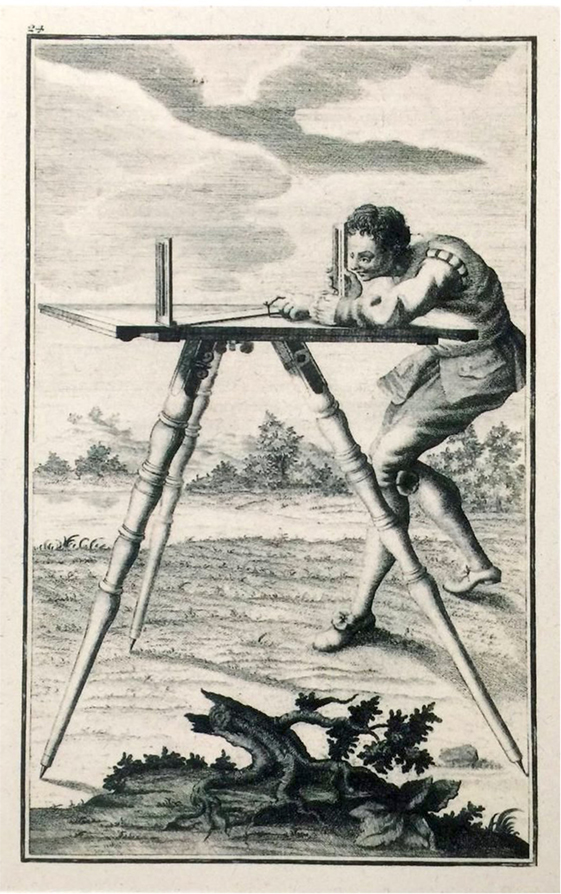
Figure 5. The Tabubla Praetoriana participated to the regularization of regulated representation for producing maps. Giovanni Giacomo Marinoni, De re ichnographica, plate 24, 1751.
The next stage was the mechanization of usage conventions, transforming the regulated representation into machines in which all the possible usages are explicitly treated. The digital maps we use nowadays permit a large set of operations (scaling, rotation, etc.) and offer ways to handle multiple information layers. As machines, they offer many more possibilities than traditional ones. However, these various new modes of usage are explicitly programmed. A paper map can be used freely for purposes other than its original function. In this sense, it is still a tool. A digital map can only be accessed through specific input and output commands; it has internalized its own usage rules. It is therefore a machine.
The mechanization process may not immediately produce changes in usage, but the changing nature of the representation results in technological synergies and aggregation effects. As maps became machines, they progressively merged into a global mechanic system in which a multitude of maps became aggregated into a single one. These dynamics have been well described by Gilbert Simondon as concretization processes (Simondon, 1958). Translated in the vocabulary of regulated representations, this principle can be formulated: As regulated representations become more regular, they tend to aggregate into unified systems.
Of course, there have been various attempts to build theories and models on the evolution of technological objects (Basalla, 1989). Along the same lines, the evolution and progressive unification of regulated representations can be studied in parallel with the succession of data acceleration regimes. Given the unification tendency of regulated representations, their evolution can be likened to a converging tree system in which various branches progressively merged to create larger standardized sets of production and usage rules. The great challenge at this stage is to come up with formalisms capable of modeling different families of regulated representations—and therefore to consider their evolution and transformation through time.
Pascal Robert introduced the idea of dimension to intellectual technology (Robert, 2010). Texts are one dimensional. Maps, tables, and trees are bi-dimensional. They can organize one-dimensional regulated representations in a bi-dimensional space. Books and libraries are volumes or containers and can therefore organize bi-dimensional representations in a 3D space. Videos and computer programs live in higher dimensional spaces that can accommodate 3D representations. Transformations between different forms of regulated representations can be understood as projections in lower or higher spaces. These initial attempts can be considered interesting directions to further understanding of the transformation of regulated representations on a longer timescale; they are necessary steps toward understanding, in a unified framework, the evolution of datafication processes throughout history.
Inferred Patterns
Regulated representations make it possible to infer patterns. In most cases, gathering data about the present is motivated by the desire to produce not only records but also a model. Indeed, a model enables new means of organizing observations, the discovery of principles and reoccurring structures and, hopefully, the prediction of future events. Prediction takes different forms depending on the domain considered. We can identify at least three kinds of dominions that each follow a slightly different logic in their datafication processes.
– The temporal dominion deals with predicting periodic rhythms and chronological patterns. Intellectual technologies associated with this dominion were calendars, chronologies, and causal tables. Astronomy and astrological tables aimed at making sense of long-term phenomena using dedicated measuring and recording methods. Today’s large-scale models from climatology, meteorology, and geology are the continuation of these datasets.
– The natural dominion addresses understanding and classifying living beings. Intellectual technologies associated with this dominion were typically lists, trees and other classification schemes, and indexes. Contemporary Big Science datafication projects for modeling genomes, brains, and particle physics are the continuation of these efforts.
– The cultural dominion encompasses human exchanges, production of artifacts, consumption of goods, migration and urbanization phenomena, etc. Intellectual technologies associated with this dominion were meant to track and monitor fluxes of exchanges through maps and tables and thereby predict cultural phenomena at different time scales. Google, Facebook, Amazon, and Apple are central actors today of the Big Data in this dominion.
A common characteristic of modeling in these three dominions is the transformation of descriptive systems into not only predictive systems but also prescriptive models, and in turn transforming data acquisition strategies. In 1567, following the wish of Ferdinando II de Medici and under the initiative of two pupils from Galileo, the Accademia Fiorentine del Cimento started a systematic measuring campaign across Europe. A network of correspondents performed local meteorological measurements following a standardized protocol and then sent their measurements to Florence, which acted as a central data repository. This sixteenth century distributed measurement system quickly led to a new language for describing meteorological phenomena, giving existence to previously invisible data and consequently shaping other information systems in return. In 1666, 100 years later, a compendium of their methods was published under the title: Saggi di naturali esperienze. Translated in Latin, it became the referential work for the next century. Ten years later, standards and new measurement methods lead to the creation of an annual publication, La Connaissance des temps (Figure 6) in France. The journal created in 1678 and thereafter continued, published every year both the scientific state of the art dealing with astronomy, time scales, referential systems, coordinate transformation, and a collection of data measurements for the current year documenting the position of the planets and other temporal and astronomical phenomena.
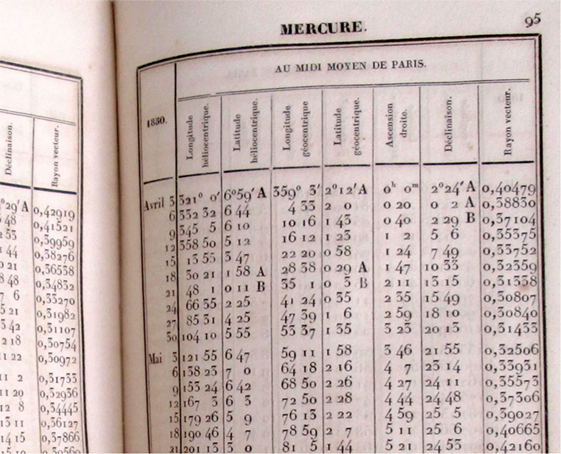
Figure 6. Regulated representation based on structured observation strategies facilitate the inference of patterns. Example of page from La Connaissance des temps, Paris, 1850.
Likewise, finding efficient and adaptable nomenclature to describe the seemingly infinite diversity of living beings was, for a long time, the cornerstone of the Big Data approach in the natural dominion. The binomial nomenclature formalized by Linné in Systema Naturae was progressively upgraded and adapted at each subsequent edition from 1735 (1st edition) to 1758 (10th edition). The binomial formalization was not entirely new as a classification and naming system. Gaspard and Johan Bauhin had developed a similar system nearly 200 years earlier. However, Linné used it in a consistent and systematic manner, offering an organizing paradigm for new observations. The 1758 edition featured an organization of 4,400 animals and 7,700 plants and established Linné’s nomenclature as the new paradigm for describing the multitude of species discovered or to be discovered.
Obviously, it is in the cultural dominion that the prescriptive effect is the strongest. In the nineteenth century, Ildefonso Cerdà, considered to be the inventor of modern urbanism, was the first to transform a data measurement approach about key urban statistics into a science of the city. His approach identified and measured not only transportation and energy flux but also global characteristics about the general quality of life in a city (sunlight, ventilation), and thereby fostered the design of spatial configurations that optimized these reified concepts. Once again, the descriptive system became a prescriptive one. For his project of expanding the area of Barcelona in 1859 (Figure 7), Cerdà took a network-oriented approach. The city was organized into a grid optimized for pedestrian movements, (horse-drawn) trams, sewer networks, and gas supplies. This pattern-based design made the city into a logical system, optimal from a statistical point of view but also partly adjustable depending on actual measurements of its performance. This Big Data-informed design was capable of producing a predictive and adaptive computational urban model.
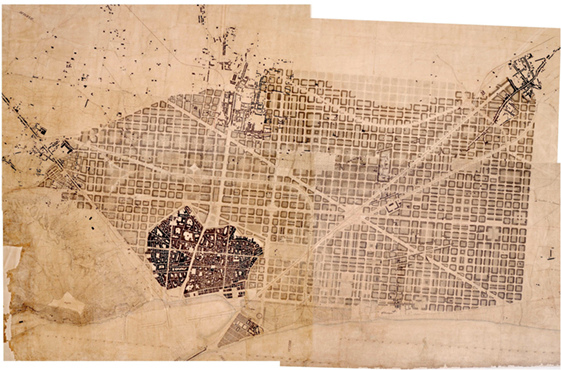
Figure 7. The pattern-based design of Ildefons Cerdà i Sunyer’s expansion of Barcelona in 1859, Barcelona, Museu d’Historia de la Ciutat.
As these examples illustrate, regulated representations are not just formal conventional systems for encoding data. They form the basis of prescriptive systems, projecting their inferred pattern-based models to shape particular interpretations and observations. When a regulated representation evolves, the associated observation grid changes in turn.
From the perspective of Big Data of the past, the challenge is to infer prescriptive models and use them, one the one hand, to test the coherence of the previously modeled dataset and, on the other hand, to procedurally reconstruct new data for which direct sources are lacking.
Redocumentation Processes
In general terms, massive datafication and data acceleration regimes tend to lead to some new forms of disequilibrium: There is too much information about the present and only “incompatible” information about the past. This can lead to a reduction of “temporal horizons,” a shift of “temporal regime,” making long-term thinking difficult (Hartog, 2003; Rappaport, 2011; Rushkoff, 2013). In these moments, the present becomes “bigger” and prediction about the future more difficult (Brand, 2000). To be capable of longer term predictions, longer datasets are necessary. Pressure increases for “redocumenting” the past using the regulated representations of the present (Pédauque, 2007).
Redocumentation processes were frequent in the nineteenth century, when data paradigms and standard of measurement were mature. For instance, Matthew Maury created a standard form for logging ship data and produced an entirely new form of navigational chart. His masterwork The Physical Geography of the Sea (Figure 8) contained about 1.2 million data points (Maury, 1857) and was based, to a large extent, on the additional “massive” extraction of data from old logbooks—a systematic reinterpretation of older data using a new grid.
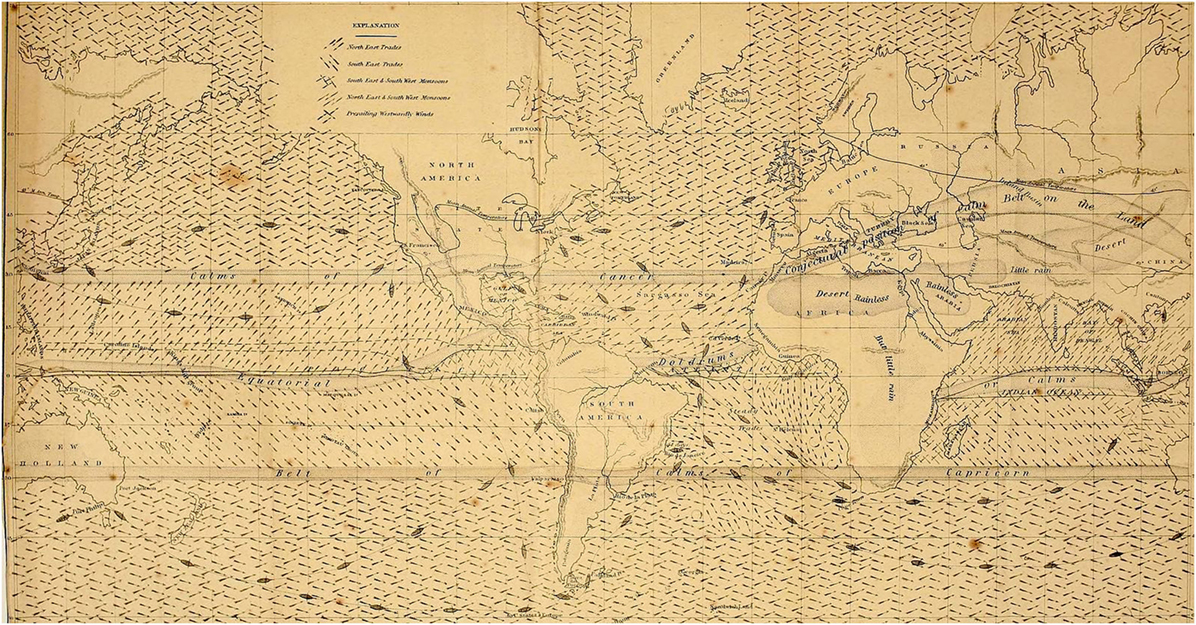
Figure 8. Matthew Maury’s “Explanations and Sailing Directions” in The Physical Geography of the Sea, 1855, London and New York. Maury introduces a standard for logging ship data and redocumenting data from old logbooks.
Translating historical datasets into the structured information of a new paradigm can be challenging when it implies processes of recollection and remapping. Recollection, like any archival practice, implies choosing some data and rejecting others and therefore amplifying certain sources while neglecting others. Remapping implies the bending of data in some ways to fit the new regulated representation, with the risk of introducing artifacts. In most cases, it requires finding homologous points in space and time in order to realign models.
In antiquity, methodological wars were already being staged over how linguistic redocumentation processes should be conducted. Librarians at Alexandria and Librarians at Pergamon are the classical representative of the opposing view of the so-called analogists and anomalists. The collation methodology was practiced by the Alexandrians to reconstruct ideal authoritative texts and a kind of ideal virtual reconstruction out of multiple copies (Greetham, 2013, p. 385). In some cases, Alexandrian librarians and their followers went as far as deciding to reintroduce “ideal” linguistic forms that did not exist in the document, following of Neoplatonistic philosophy in their textual transmission strategy. On the other side of the debate, Pergaminians insisted on describing linguistic elements based on the preserved documentary forms, possibly following the Stoician philosophical stance that all material traces are inevitably flawed. This debate from antiquity is still at the heart of the creation of linguistic formal systems based on redocumentation processes.
Grammatical systems themselves need to remodeled to adapt to a new formal model. In the nineteenth century, the German linguist Franz Bopp rediscovered the Panini grammar and pioneered early comparative theories about Indo-European languages. Later, several founders of modern linguistics such as de Saussure, Bloomfield, and Jakobson reinterpreted the ancient grammar into the formal systems they invented from describing languages. In such a context more than in any other, data are not given but rather constructed through a long chain of recollection and remapping (Gitelman, 2013).
The so-called Bamboo Annals, written on bamboo slips (Figure 9), a classical writing medium before the Chinese invention of paper, is one of the most ancient chronicles of China, covering the period from the legendary times to the third century BC. Discovered in a tomb in 298 Anno Domini (AD), they survived the burning of books (and burying of scholars) that is thought to have occurred in China around 210 BC, which resulted a vast collective memory loss in Chinese History. After their discovery and throughout their translation and reinterpretation, the contents were significantly modified, adapting to the new ways of describing events and chronology. Due to this redocumentation process, they feature a unique regularly paced reconstructed chronology of China’s antiquity. As such these annals also invented an original way of organizing historical events following specific geographical and temporal conventions, a kind of ancient information system for the past.
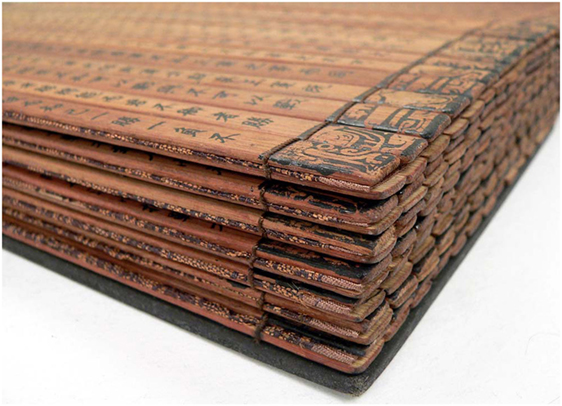
Figure 9. Example of bamboo slips. The Bamboo Annals led to a reconstructed chronology of China’s ancient history. Vlasta2.
In the historical and geographical domains, redocumentation implies the existence of fixed points, common references that enables the alignment of data produced under different paradigms. The Venerable Bede (673–735), English monk, had to address the problem of aligning different chronological systems to organize the profusion of unverified historical facts in a common system. To address this challenge, Bede defines the AD temporal origin using homological events present in various chronicles (Figure 10). The dissemination of this new dating system played a crucial role for shaping a common chronological framework and aligning different historical sources into a coherent dating system (Wallis, 1999).
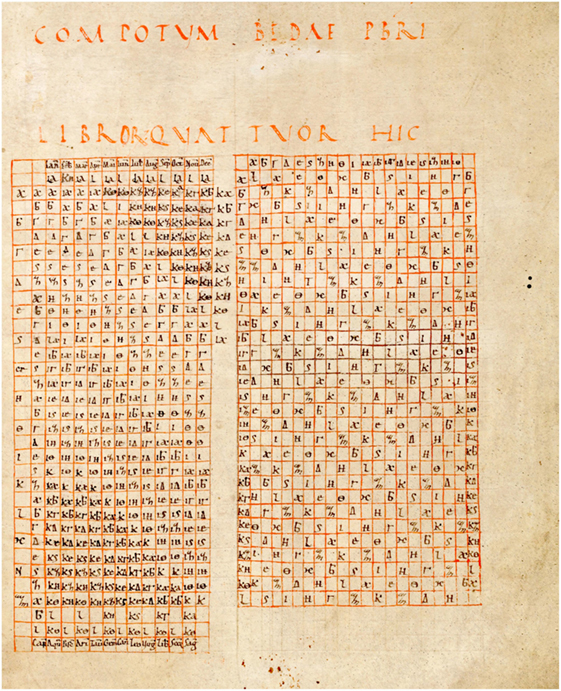
Figure 10. Bede, De natura rerum, De temporibus, Cod. Sang. 248, f. 59 St. Gallen, Stiftsbiothek. Examples of tables reconstructed by Venerable Bede to align different chronological systems. e-codices.
Likewise, the Polychronicon from Anglo-Saxon Benedictine monk Ranulf Hidgen (1280–1363) is a compilation of several chronicles combining many different traditions that aimed to be an encyclopedic world history (Figure 11). To perform this universal recompilation of knowledge, Hidgen developed a systematic framework to redocument the records of other chronicles. To align events in a coherent temporal framework, Hidgen use eight calendar systems: three Hebraic (one starting in January, one starting in February, one starting in March), three Greek (Troy, Olympiads, Alexander), one Roman (Ad Urbe condita, starting in 753 BC), and a Christian one. Events reported were then tagged in the margin of the text using several chronological systems. This multicolumn system was pivotal to the organization of this universal chronicle.
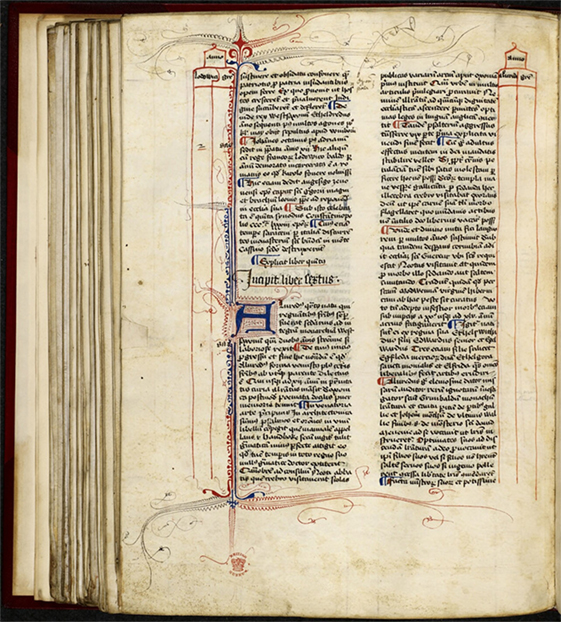
Figure 11. Ranulf Higden, Polychronicon, England, ab. 1445, book VI, f. 118v, London, Harleian Manuscripts, British Museum. The beginning of Book VI, with two marginal columns prepared for recording the year, dating both from the birth of Jesus and within the reigns of King Louis II (left) and Alfred the Great (right). British Library.
Finding homological events, as Bede and Hidgen did, are key to remapping chronologies in standardized temporal projections. Likewise, a particular landmark, such as a church’s campanile, could serve as a fixed point to align maps of different centuries, and several common landmarks could constitute the basis of a mapping function to realign data from the past. In other cases, structural mapping can be envisioned, for instance, for aligning early modern documentary structure with contemporary information systems (de Vivo, 2010).
Data from the past systematically undergo a form a regularization to match a new paradigm. During this regularization process, data are reinterpreted, patterns are induced, and new data are inferred. In that sense, redocumenting data from the past are like trying to simulate the past and filling the gap left by the datasets using the inference methods of new paradigms.
The core challenges of redocumentation go beyond the mastering of recollection and remapping techniques. Precisely because redocumentation is not a one-step process but a series of recursive reconstructions, redocumentation processes must be carefully modeled. Ideally, recollection and mapping operations should be described along with the persons performing them. In many contemporary cases, some of these operations include algorithmic steps which should be equally described. The standard for such form of metahistorical modeling or paradata (Bentkowska-Kafel et al., 2012) must still be invented and agreed upon.
Fictional Spaces
Information extracted from historical documents can be wrong in many ways. Historical reconstruction is highly uncertain not only because of the long series of redocumentation processes but also for many other potential reasons. The document contents could be false or imprecise. This affects all kinds of primary and secondary sources from ancient manuscripts, administrative records, and cadastral maps to contemporary academic books, news articles, or virtual reconstructions. In addition, the regulated representations encountered in two documents could be partly incompatible, like the diverse chronology that Bede and Hidgen needed to align. Eventually, any procedural method and particularly contemporary algorithmic processes can add noise, artifacts, and other errors in both the processing of single documents and in the alignments of large sets of extracted information.
This provides motivation to consider that information extracted from historical documents could be organized in potentially disjointed fictional spaces. Each fictional space contains only coherent information extracted from an identified set of sources. It constitutes a virtual historic reconstruction, a possible historic reality.
Coherence is calculated by the non-violation of a series of defined constraints. For instance, it could be assumed that a dead person may not interact anymore after the date of his death, or that someone cannot be at two different places at the same time. If one document indicates that Dürer is in Venice in early June 1502, and it can be deduced from another one that he is in Nuremberg at this date, two fictional spaces must be created. One difficulty is that such common sense constraints may vary depending on beliefs (e.g., the possibility of supernatural forces impacting the world!) and actual knowledge about a given culture (e.g., depending on means of transportation, the subsequent presence of the same person in two different places may or may not be possible). Finding flexible ways of representing such constraints is a core challenge of this kind of modeling.
Several fictional sets could be joined to create bigger ones. If the coherence constraints match, they could be merged into a larger set. As in any redocumentation process, this implies mapping the sets on a common framework of regulated representations and aligning the two sets by identifying common stable elements (identifying a person, a particular place or a concept as the same). These mapping operations should obviously be fully documented in a standard form as they inform the nature of the reconstruction.
Imposing only a fully compatible union between fictional spaces maybe unrealistic, given the uncertain and incoherent nature of extracted historical information. This could result in large sets of disjointed fictional spaces. For this reason, it may be relevant to consider partial matching and to evaluate based on a cost function proportional to the amount of incoherent information provoked by the matching. Fictional spaces with marginal cost, in terms of incoherence, may be accepted as offering a potentially coherent reconstruction. Metaphorically, constraints acting over the entire reconstructed system may be compared to a large system of springs that generally pushes the system toward a stable solution but allows for a certain degree of freedom. The cost of reconstruction would correspond, in this case, to how much the system must be stressed to fit the desired configuration (Figure 12).
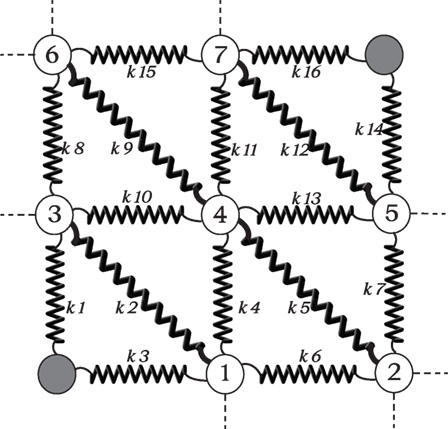
Figure 12. The cost of a given reconstruction could be compared to the action of a system of springs. Reconstructed statements are nodes and each constraint ensuring the coherence of the statements corresponds to the tension operated by a spring.
The action of algorithms will be crucial to performing the optimized union of fictional spaces. Beyond a given volume of extracted information, human tests of coherence are out of reach. Given that coherence constraints need to be written using formal operators, and since certain historical hypotheses are likely to be expressed using procedural methods, the historical debate is likely to be increasingly mediated by algorithms (Roux and Aussenac-Gilles, 2010; Kaplan et al., 2014).
The design of large-scale unification engines capable of exploring the various combinations of fictional spaces is one of the greatest challenges to creating a large set of coherent Big Data of the past. Such an effort can be traced back to early expert systems, now rebranded as cognitive computing (Kelly and Hamm, 2013). This problem of solving constraints necessitates finding good exploration strategies, and it is likely that in many cases only suboptimal unions can be found. In addition, the arrival of new data from digitization and extraction could create new fictional spaces that are incompatible with the present unification. In such cases, backtracking may be needed to separate previously joined spaces.
One can consider the landscape of all the possible pasts and see the reconstruction as its progressive exploration. The cost of each reconstructed past can be evaluated based on the number of statements it does not consider and the number of constraints it breaks. In such terms, the unification of fictional spaces can be seen as an optimization problem, analogous, for instance, to simulated annealing (Aarts and Korst, 1988). To avoid being trapped in local “valleys” due to suboptimal unions of fiction spaces, it could make sense to create disorder, to temporarily increase the level of the reconstruction “temperature,” disjoining some of the fictional spaces and letting other, potentially more fruitful unifications emerge. The methodology and technology to perform such global optimizations has yet to be developed and constitutes a cornerstone of the successful exploitation of Big Data of the past.
Conclusion
Big Data of the past can be seen as a construct, articulated on the basis of six intermediary concepts, briefly discussed here. The purpose of this first overview was mainly to discuss their mutual articulation.
(1) The bigness of data can be measured in a least four dimensions. Big Data is big because of the technological difficulty to process it, because of its open-ended nature, because of its networked structure, and because it fosters the structuration of new knowledge paradigms. Defining quantitative measures for these four dimensions, despite their extremely diverse qualitative nature, is one of the challenges to grounding this concept.
(2) The four dimensions of data bigness are obviously not independent. During what can be defined as data acceleration regimes, they mutually reinforce themselves in circular dynamics. Data acceleration regimes can be spotted not only during the European Renaissance and the transformations of the nineteenth century but also much further in the past with the administrative inventions of Mesopotamian city states or the Roman Empire. Finding rigorous methods to identify and segment these “Big Data moments” spatially and chronologically is one of the challenges to studying the global impact of these information transformations.
(3) At finer granularity, what links technology with a continuous production of standardized data streams are regulated representations, governed by sets of production and usage rules. Indexes of names, accounting tables, family trees, flow-chart diagrams, maps, or graphs are examples of such regulated representations. Mapping the genealogical evolution of regulated representations in space and time and understanding how to translate and align their contents are two necessary preparatory steps to reconstructing the transformation of data structures in time.
(4) Patterns are the results of the structuration imposed by regulated representations. During each data acceleration regime, regulated representations structures data streams, making it possible to accumulate organized information and to infer subsequent data patterns. Descriptive models turn into prescriptive ones, guiding data acquisition strategies and impacting design and societal choices. For this reason, regulated representations should always be considered in association with the prescriptive patterns they induce. The core challenge is to find forms that express these underlying models and exploit their prescriptive dimensions to compensate for a lack of information about the past.
(5) Redocumentation processes have always been needed to translate information stored in obsolete regulated representations into updated information paradigms. Redocumentation implies re-collecting (i.e., choosing what data to keep and what data to ignore) and remapping (i.e., searching for homologous points in space, time, and other more complex cultural dimensions). As redocumentation is a central characteristic of the nature of the dataset from the past, it is crucial to not only understand how to redocument old datasets using the paradigms of the present but how successive translation processes previously occurred in the past, explaining the particular nature of the data considered. As Big Data of the past is the result of a series of rewriting processes, the core challenge is not only to perform these redocumentations but to model them as recursive operations.
(6) Information about the past that results from different datafication operations and consecutive redocumentation processes is always potentially unreliable and often inconsistent. Coherent sets of information extracted from historical documents can be organized as disjointed but locally consistent fictional spaces. The core challenge is to unify these fictional spaces into larger spaces, sometimes accepting partial inconsistencies. Through this formalization, historical reconstruction becomes a problem of optimization.
At the end of this progressive conceptual construction, the question of a collectively negotiated common past needs to be articulated. In practice, the convergence of digital, regulated representations capable of describing large varieties of data in standardized forms and sharing dynamics that unify previously disconnected datasets could result in a shared repository of reconstructed data. Each historical archive contains information that could be complementary to others located in other repositories. Frozen datasets can be interconnected to be put in motion, reenacting via simulation the open-ended nature of the Big Data of the present. During redocumentation processes, this interdependency fosters the transformation of privately owned datasets to commonly accessible goods. The logic of freeing and interconnecting datasets from the past is much stronger than for datasets of the present, and this force can potentially break through some of the data silos.
The process of transforming of privately held archives or collections to common public repositories is historically well documented. Perceived as both a sustainable way of allowing these collections to survive for centuries and morally justified, donation of art collections or private libraries to public institutions has been consistently on the rise. Exchanges and aggregations of public collection to greater repositories have constituted a reinforcing movement. Since the eighteenth century, shared knowledge systems are recognized as political, economic, and philosophical assets that are crucial to societal progress. The rise of standardization reinforced the idea that the fate of every dataset is to become, sooner or later, a shared resource by which new predictions and pattern findings can be established.
With the digital turn, the establishment of exchange standards and open data/open source politics continues to facilitate the establishment of large datasets for the common good. Indeed, sharing large datasets about the past as open contents facilitates crowdsourcing and distributed curating. This is of crucial importance for dealing with different scales of history, from worldwide phenomena to local histories and involving different kinds of audiences to actively participate to the reconstruction of the past. Moreover, this opens the way for something previously impossible to imagine: a multiscale, collectively negotiated common history.
Needless to say the construction of the past is highly political territory, and agreeing to common reconstructions is always difficult. In addition, the abundance of diverse fictional spaces could also be seen as a fundamental richness that fosters a multiplicity of interpretations. Nevertheless, if we match all the challenges articulated in this article, the project of a collective negotiation toward a potentially unique common reconstruction becomes possible. By optimizing the unification of fictional spaces, one can create shared historical reconstructions that are continuously under negotiation but nevertheless moved by convergent dynamics. There will be historical investigators seduced by what we could call, the Common History Stance, the goal to reach a shared reconstruction of the past, and others who will view this position as a new form of intellectual imperialism, a dangerous reductionism or a rigid view of the complex processes of historical reconstruction. This debate has not really started yet. In the meantime, we must seize the opportunity to build today the science that will allow us tomorrow to exploit the vast amounts of heretofore under-examined historical data and the tools to organize the negotiation of common pasts.
Author Contributions
Both authors contributed equally to the article.
Conflict of Interest Statement
The authors declare that the research was conducted in the absence of any commercial or financial relationships that could be construed as a potential conflict of interest.
References
Aarts, Emile, and Korst, Jan. (1988). Simulated Annealing and Boltzmann Machines: A Stochastic Approach to Combinatorial Optimization and Neural Computing. Hoboken, NJ: Wiley.
Armstrong, W.W., Burris, W., Gingrich, D.M., Green, P., Greeniaus, L.G., Hewlett, J.C., et al. (1994). ATLAS: Technical Proposal for a General-Purpose p p Experiment at the Large Hadron Collider at CERN. Available at: http://hal.in2p3.fr/in2p3-00011404
Basalla, George. (1989). The Evolution of Technology. Reprint Edition. Cambridge, England; New York: Cambridge University Press.
Bentkowska-Kafel, A., Denard, H., and Baker, D. (2012). Paradata and Transparency in Virtual Heritage. Farnham: Ashgate Publishing, Ltd.
Berman, Fran, Geoffrey, Fox, and Hey, Anthony J.G. (2003). Grid Computing: Making the Global Infrastructure a Reality. Hoboken, NJ: John Wiley and Sons.
Berners-Lee, Tim, Wendy, Hall, James, Hendler, Nigel, Shadbolt, and Weitzner, Daniel J. (2006). Creating a science of the web. Science 313:769–71. doi: 10.1126/science.1126902
Blair, Ann. (2003). Reading strategies for coping with information overload ca. 1550–1700. Journal of the History of Ideas. Philadelphia, PA, USA: University of Pennsylvania Press 64:11–28. doi:10.2307/3654293
Blair, Ann. (2010). Too Much to Know: Managing Scholarly Information Before the Modern Age. New Haven.
Boyd, Danah, and Crawford, K. (2011). “Six Provocations for Big Data.” A Decade in Internet Time: Symposium on the Dynamics of the Internet and Society. Available at: http://ssrn.com/abstract=1926431
Boyd, Danah, and Crawford, K. (2012). Critical questions for big data. Information, Communication & Society 15:662–79. doi:10.1080/1369118X.2012.678878
Brand, Stewart. (2000). Clock of the Long Now: Time and Responsibility: The Ideas Behind the World’s Slowest Computer. New York, NY: Basic Books.
Cantor, Charles, and Smith, C. (1999). Genomics: The Science and Technology behind the Human Genome Project. 1st ed. New York: Wiley.
de Vivo, Filippo. (2010). Ordering the archive in early modern Venice (1400–1650). Archival Science 10:231–48. doi:10.1007/s10502-010-9122-1
Dean, Jeffrey, and Ghemawat, Sanjay. (2008). MapReduce: simplified data processing on large clusters. Communications of the ACM 51:107–13. doi:10.1145/1327452.1327492
Findlen, Paula. (1996). Possessing Nature – Museums, Collecting & Scientific Culture in Early Modern Italy. Reprint. Berkeley: University of California Press.
Foucault, Michel. (1966). Les Mots Et Les Choses: Une Archéologie Des Sciences Humaines. Paris: Gallimard.
Frier, Bruce W. (1999). Libri Annales Pontificum Maximorum: The Origins of the Annalistic Tradition. Ann Arbor: University of Michigan Press.
Garnsey, Peter, and Saller, R.P. (1987). The Roman Empire: Economy, Society, and Culture. Berkeley: University of California Press.
Gleeson-White, Jane. (2013). Double Entry: How the Merchants of Venice Created Modern Finance. 1 ed. New York, NY: W. W. Norton & Company.
Greetham, David. (2013). Textual Transgressions: Essays toward the Construction of a Biobibliography. Abingdon-on-Thames, UK: Routledge.
Hartog, François. (2003). Régimes d’historicité: Présentisme et expériences du temps. Paris: Éditions du Seuil.
Hey, Tony, Tansley, S., and Tolle, K. (2009). The Fourth Paradigm: Data-Intensive Scientific Discovery. 1st ed. Redmond, WA: Microsoft Research.
Hull, David L. (1979). In Defense of Presentism, History and Theory. Vol. 18. Hoboken, NJ: Wiley. 1–15.
Jacquesson, Alain. (2010). Google Livres et le futur des bibliothèques numériques. Paris: Editions du Cercle de La Librairie.
Kaplan, Frédéric. (2012). How books will become machines. In Lire Demain. Des Manuscrits Antiques à L’ère Digitale, Edited by C. Claire, M. Jérome, V. François, and V. Joseph, 25–41. Lausanne: PPUR.
Kaplan, Frédéric. (2015). A map for big data research in digital humanities. Frontiers in Digital Humanities 1:7. doi:10.3389/fdigh.2015.00001
Kaplan, Frédéric, Fournier, Mélanie, and Nüssli, Marc-Antoine. (2014). L’historien et l’algorithme. In Le Temps des Humanités Digitales, Edited by O. Le Deuff, 49–63. Limoges: Fyp Editions.
Kelly, John, and Hamm, Steve. (2013). Smart Machines: IBM’s Watson and the Era of Cognitive Computing. New York, NY: Columbia University Press.
Lander, E.S., Linton, L.M., Birren, B., Nusbaum, C., Zody, M.C., Baldwin, J., et al. (2001). Initial sequencing and analysis of the human genome. Nature 409:860–921. doi:10.1038/35057062
Markram, Henry. (2006). The blue brain project. Nature Reviews Neuroscience 7:153–60. doi:10.1038/nrn1848
Markram, Henry, Muller, Eilif, Ramaswamy, Srikanth, Reimann, Michael W., Abdellah, Marwan, Sanchez, Carlos Aguado, et al. (2015). Reconstruction and simulation of neocortical microcircuitry. Cell 163:456–92. doi:10.1016/j.cell.2015.09.029
Maury, Matthew Fontaine. (1857). The Physical Geography of the Sea. New York, NY: Harper & Brothers. Available at: https://archive.org/details/physicalgeograph01maur
Mayer-Schönberger, Viktor, and Cukier, Kenneth. (2013). Big Data: A Revolution That Will Transform How We Live, Work, and Think. Boston, MA: Houghton Mifflin Harcourt.
Missra, Vidyaniwa. (1966). The Descriptive Technique of Panini: An Introduction, Mouton. Paris: Walter de Gruyter GmbH & Company KG.
Ogilvie, Brian. (2003). The many books of nature: renaissance naturalists and information overload. Journal of the History of Ideas. Philadelphia, PA, USA: University of Pennsylvania Press 64:29–40. doi:10.1353/jhi.2003.0015
Pettinato, Giovanni. (1981). The Archives of Ebla: An Empire Inscribed in Clay. New York, NY: Doubleday.
Rappaport, Alfred. (2011). Saving Capitalism from Short-termism: How to Build Long-term Value and Take Back our Financial Future. New York: McGraw Hill Professional.
Robert, Pascal. (2010). Mnémotechnologies: Une Théorie Générale Critique Des Technologies Intellectuelles. Paris: Hermes Science Publications.
Rosa, Hartmut. (2013). Social Acceleration: A New Theory of Modernity. 1 ed. New York: Columbia University Press.
Rosenberg, Daniel. (2003). Early modern information overload. Journal of the History of Ideas 64:1–9. doi:10.1353/jhi.2003.0017
Rosenberg, Daniel, and Grafton, Anthony. (2012). Cartographies of Time: A History of the Timeline. New York, NY: Princeton Architectural Press.
Roux, Valentine, and Aussenac-Gilles, N. (2010). Knowledge bases and query tools for a better cumulativity in the field of archaeology: the Arkeotek project. In Proceedings of the 38th Annual Conference on Computer Applications and Quantitative Methods in Archaeology, CAA2010, Edited by F. Contreras, M. Farjas, and F.J. Melero. Granada, Spain.
Keywords: digital humanities, big data, digital history, datafication, fictional spaces
Citation: Kaplan F and di Lenardo I (2017) Big Data of the Past. Front. Digit. Humanit. 4:12. doi: 10.3389/fdigh.2017.00012
Received: 30 September 2016; Accepted: 10 May 2017;
Published: 29 May 2017
Edited by:
Josep Lladós, Universitat Autònoma de Barcelona, SpainReviewed by:
Xavier Rodier, Environnement et Sociétés (CITERES), FranceFrancesca Bortoletti, University of Minnesota, United States
Copyright: © 2017 Kaplan and di Lenardo. This is an open-access article distributed under the terms of the Creative Commons Attribution License (CC BY). The use, distribution or reproduction in other forums is permitted, provided the original author(s) or licensor are credited and that the original publication in this journal is cited, in accordance with accepted academic practice. No use, distribution or reproduction is permitted which does not comply with these terms.
*Correspondence: Frédéric Kaplan, ZnJlZGVyaWMua2FwbGFuQGVwZmwuY2g=