- 1Division of Metabolic Diseases, Department of Internal Medicine, Faculty of Medicine, University of Debrecen, Debrecen, Hungary
- 2Aesculab Medical Solutions, Black Horse Group Ltd., Debrecen, Hungary
- 3Department of Laboratory Medicine, Faculty of Medicine, University of Debrecen, Debrecen, Hungary
- 4Department of Dermatology, Roswell Park Comprehensive Cancer Center, Buffalo, NY, United States
- 5Center of Oncoradiology, Bács-Kiskun County Teaching Hospital, Kecskemét, Hungary
- 6Central Medical Department, Synlab Group (Synlab Hungary Ltd.), Budapest, Hungary
- 7Central Laboratory, St. John’s Hospital, Budapest, Hungary
- 8Laboratory of Immunology, Synlab Budapest Diagnostic Center, Budapest, Hungary
- 9Department of Artificial Intelligence and Systems Engineering, Faculty of Electrical Engineering and Informatics, Budapest University of Technology and Economics, Budapest, Hungary
- 10Evidia MVZ Radiologie, Nürnberg, Germany
- 11Department of Cardiology, Faculty of Medicine, University of Debrecen, Debrecen, Hungary
- 12Artificial Intelligence National Laboratory, Institute for Computer Science and Control (SZTAKI), Hungarian Research Network (HUN-REN), Budapest, Hungary
Introduction: The integration of AI into healthcare is widely anticipated to revolutionize medical diagnostics, enabling earlier, more accurate disease detection and personalized care.
Methods: In this study, we developed and validated an AI-assisted diagnostic support tool using only routinely ordered and broadly available blood tests to predict the presence of major chronic and acute diseases as well as rare disorders.
Results: Our model was tested on both retrospective and prospective datasets comprising over one million patients. We evaluated the diagnostic performance by (1) implementing ensemble learning (mean ROC-AUC.9293 and mean DOR 63.96); (2) assessing the model's sensitivity via risk scores to simulate its screening effectiveness; (3) analyzing the potential for early disease detection (30–270 days before clinical diagnosis) through creating historical patient timelines and (4) conducting validation on real-world clinical data in collaboration with Synlab Hungary, to assess the tool's performance in clinical setting.
Discussion: Uniquely, our model not only considers stable blood values but also tracks changes from baseline across 15 years of patient history. Our AI-driven automated diagnostic tool can significantly enhance clinical practice by recognizing patterns in common and rare diseases, including malignancies. The models' ability to detect diseases 1–9 months earlier than traditional clinical diagnosis could contribute to reduced healthcare costs and improved patient outcomes. The automated evaluation also reduces evaluation time of healthcare providers, which accelerates diagnostic processes. By utilizing only routine blood tests and ensemble methods, the tool demonstrates high efficacy across independent laboratories and hospitals, making it an exceptionally valuable screening resource for primary care physicians.
1 Introduction
Chronic workforce shortages, unequal distribution of healthcare professionals, and rising labor costs present significant challenges for most healthcare systems worldwide. In recent years, technological advancements have aimed to mitigate these pressures. The broad adoption of digital databases, modern diagnostic testing and screening, and the expansion of digital communication channels created the opportunities to redistribute the workload of skilled medical professionals. This technological transformation of the available tools of healthcare systems combined with pandemic-induced restrictions, has led to the accelerated transition and acceptance of virtual medicine. Laboratory follow-ups, in particular, offer an ideal environment for virtual healthcare encounters. Routine tests can be performed in remote, controlled environments, convenient for the patient, and communication between healthcare providers (HCPs) and the patient can be performed via digital platforms.
Despite this, the diagnostic process remains largely reliant on the clinical judgment of HCPs, based on the diagnostic test results. While clinical pathology/laboratory medicine physicians perform and report these tests, the direct communication with HCPs is typically limited to critical or emergency situations, leaving most non-emergency cases without in-depth interpretive support. This creates a gap where a more comprehensive and in-depth analysis of laboratory results could greatly aid HCPs in reaching accurate diagnosis.
However, such detailed evaluation support is currently impractical at the clinical-pathologist level due to the sheer volume of patients and tests conducted. Therefore, other means of evaluating laboratory results are sorely needed.
Diagnostic errors and delays, which occur in 15%–20% of all patient-doctor encounters (1, 2) are significant concerns in modern medicine, contributing to delayed diagnoses and misdiagnoses, making them the sixth leading cause of death in the United States (3). Interestingly, while diagnostic errors persist, laboratory error rates are exceptionally low, estimated at just .33% (ranging from .1% to 3.0%) (4); indicating that laboratory test results are reliable and high-quality source of information that could be leveraged to improve diagnostic processes through technology such as artificial intelligence (AI).
Blood tests are among the most common and cost-effective diagnostic tools in medicine. They provide vital information on a wide range of physiological conditions, from routine screenings to complex disease monitoring. However, interpreting the growing number of specialized tests can be challenging due to human limitations, particularly when test results are borderline or inconclusive. Diagnostic errors in medicine are of a complex origin and are mostly classified as no-fault, system-related, or cognitive errors. Cognitive errors are primarily due to the false processing of available information and often account for the most severe misdiagnoses (2). Moreover, many rare diseases are notoriously difficult to diagnose, often resulting in delayed recognition. For instance, a study of late-onset Pompe disease showed that 48% of patients were incorrectly diagnosed in the past, with an increased mean time to diagnosis of 10.5 vs. 2.5 years (from symptom onset) (5).
Blood tests were chosen as the focal point for this AI application due to their ubiquity, low cost, and vital role in diagnostic processes. Furthermore, their high reliability presents an ideal foundation for applying machine learning algorithms to enhance diagnostic accuracy and aim to provide evidence that such tools will transform clinical diagnostic processes, improving diagnostic accuracy and enabling earlier diagnosis. Our objectives were to
1. Train and test our ensemble learning model to diagnose rare and common chronic diseases on widely available, low-cost blood tests.
2. Evaluate the diagnostic performance of the AI and the possibility for earlier diagnosis.
3. Implement our AI-based diagnostic support tool in practice and evaluate in-practice performance vs. performance on retrospective data.
2 Related work
Artificial intelligence (AI) has the potential to revolutionize the interpretation of blood tests and improve diagnostic accuracy. Since the emergence of deep learning technologies in 2012, AI has rapidly advanced within healthcare, with substantial applications in areas such as medical imaging and image-based diagnosis. More recently, additional field genome interpretation and biomarker discovery, and patient monitoring have also gained increased importance (6). However, in laboratory medicine, as reviewed in Santos-Silva et al. (7), AI advancements have been focusing on narrow diagnostic areas, and include only one or few specific laboratory parameters (7). These AI developments included the evaluation of bacterial infection on hospital admission (8), predicting bacteremia in maternity patients (9), classifying outcomes in malaria infection based on hematological parameters (10), or supporting clinical pathologists in flow cytometry evaluations (11, 12), and detection of Alzheimer's disease based on specific data about the state of the brain and patient's metadata (13). The primary disadvantage of these models is the narrow diagnostic focus, which makes them less efficient to implement and utilize on large numbers of patients and conditions. For a detailed description and comparison of the mentioned literature, see Table 1. We argue that the main challenges in the field of AI-based blood test analysis are:
• How to achieve robustness despite limitations in the available data?
• How to identify a general attribute set that can be used across different disease classes that are physiologically connected to the blood work?
• How to define a set of machine learning methods suitable for preprocessing and inference?
In this paper, we primarily focus on these questions with the intention to create a solution to evaluate routine blood tests across a broad spectrum of diseases, thereby decreasing the human professional workload within the interpretation of screening tests and providing a broadly used method for risk mitigation of medical decisions and differential diagnosis.
3 Methods
The development leveraged 15 years and 1.3 million complete, uncleaned, but anonymous patient medical record data (2000–2015, directly from the HIS - Hospital Information system) in cooperation with the University of Debrecen Clinical Center (UDCC), County Hospitals of Szabolcs-Szatmár-Bereg (CHSSB) and UDCC's contractual software developer partner (Aesculab Medical Solutions - Black Horse Group Ltd.). The sites involved in the research were the following: UDCC, the András Jósa University Hospital, and additional smaller hospitals in neighboring cities, five hospital units, and their outpatient centers (all together referred to as CHSSB). Table 2 shows the diseases, the number of patients included in the retrospective study, and the descriptive statistics for each group.
Due to the cardinality of the patients, and the variability of the contextual factors regarding age, sex, occupation, and availability of the healthcare system, we developed a framework to preprocess the data. As a result, we managed to identify disease groups where we met all the necessary conditions to apply machine learning while achieving a reliable, reproducible, and scalable system. The dataset we used contained data from multiple hospitals and laboratories. One of our biggest challenges was to make the data comparable and to find robust models that are as insensitive to data from different sources as possible.
3.1 Creating the set of disease cases
Identifying valid disease labels before applying machine learning methods was essential for the disease classification software. This labeling procedure had to be primarily machine-driven due to the vast amount - tens of thousands - of medical cases. We used a multilevel structure of mainly machine-led and partly manual labeling validation procedures. The first layer of disease categorization was the ICD-10 coding within each patient visit record, which is mandatory in the Health Information System (HIS) database. Each medical case had at least one applied disease code in the HIS. Therefore, we labeled each case with our defined disease group, disease, or sub-disease labels based on the ICD-10 code(s) of the given case in the HIS. Records from the early 2000s used the ICD-9 framework, but the transition to the ICD-10 already occurred at the institutional level before accessing the datasets; thus, we did not need to address any transitional issues. Both the primary and additional diagnoses were labeled. As a subsequent layer, we leveraged available textual anamnestic data to (1) remove non-similar miscategorized cases and (2) find additional disease cases that missed the proper ICD coding. We used the classic data mining library of regular expressions (regex), the statistical descriptors term frequency-inverse document frequency (TF-IDF) and bag-of-words (14), and mathematical methods such as cosine similarity matrices and a neural network, the bidirectional encoder representations from transformers (BERT) model (15). This is a crucial step, as this procedure can alter the number of labeled disease cases by 10%–50%. Manual case validation was the last step of the labeling process and is usually considered verification (for diseases of massive case occurrence); however, this process is also a significant step in identifying rare diseases (along with similarity calculations due to their heavy underdiagnosis), see Figure 1. Each medical case/patient can have multiple conditions and thus may be categorized into multiple disease categories. Each patient has one binary condition for each disease group — “yes” or “no”— but a single patient can have multiple “yes” calls for the different diseases, see Figure 2.
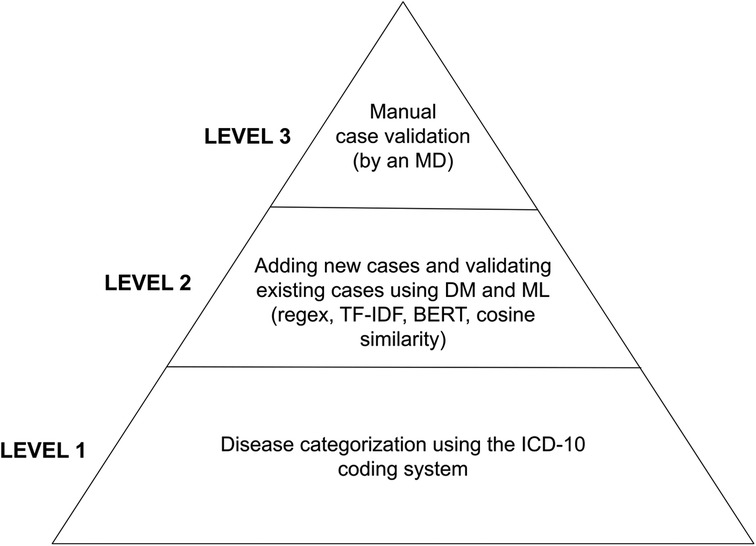
Figure 1. Layers of validation procedures starting from mass categorization based on HIS data and timely assignment (bottom of the pyramid) toward refinement with modern data mining tools and manual validation. BERT, bidirectional encoder representations from transformers; DM, data mining; MD, medical doctor; ML, machine learning; TF-IDF, term frequency-inverse document frequency.
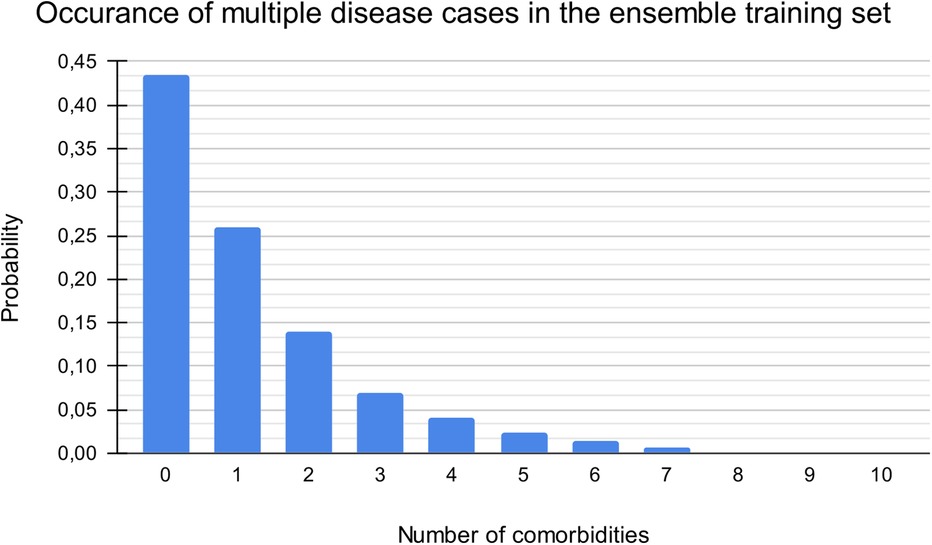
Figure 2. Distribution of patients by number of comorbidities. The maximum number of simultaneous diseases was ten (out of the 14 categories). Zero comorbidity indicates a healthy patient (without suspected or reported diagnosis).
3.2 Machine learning models
The core elements of the system are disease-specific machine learning models. These models were trained on historical medical data originating from two major hospitals. The models were selected by a validation procedure with four distinct steps. The first objective during selecting the final models was to identify a subset of the available laboratory tests we aim to include. First, we defined candidate laboratory tests, then we dismissed disease-specific tests, e.g., TSH, or expensive tests. Due to statistical reasons, we also excluded rare laboratory tests. In the second phase, we selected a subset of diseases as possible candidates for analysis directly from blood tests. In the third phase, we trained machine learning models for all candidate diseases using the full set of laboratory tests. In the last step, we identified a subset of 70 laboratory tests and a set of disease groups where the models show very high performance with low variance. For a detailed list of blood tests see Table 3.
The data shown in the article are based on a random 50–50 split between training and testing for machine learning for software development. The sampling procedure was always repeated 30 times. The data are presented as the average of the different models and train-test data. All the raw data were split for training purposes; the split was made to account for temporal and regional differences in the dataset. For time-based separation, we usually used a five- to eight-year split depending on the participating hospital, partially due to HIS needs (system changes) and to achieve more homogenous ata. The most common time separations were 2000–2005, 2000–2008, 2007–2015, 2006–2010, and 2011–2015. Regional separation was used to separate data from the two leading county hospitals (UDCC and CHSSB), smaller hospitals (CHSSB data), and clinical departments. The training was carried out on the intersections of the separated data, and the resulting performance metrics are the averages of the best-performing models with the restriction that the final ensembles must always contain test- trains on different regional separated data (trained on one hospital/department and tested on another) with different laboratories involved. For more details about the preparation steps for machine learning see Supplementary Appendix A and for a detailed framework structure see Supplementary Appendix B. The disease representation learned by the mathematical models was used to classify patients into one or multiple disease groups. For an extended description of the learned representation and the machine-learning models of disease classification, see Supplementary Appendix C. To visualize the quality of the learned representation, we showed that the patients with different diagnoses align in space using the UMAP (16) framework, see Figure 3A,B.
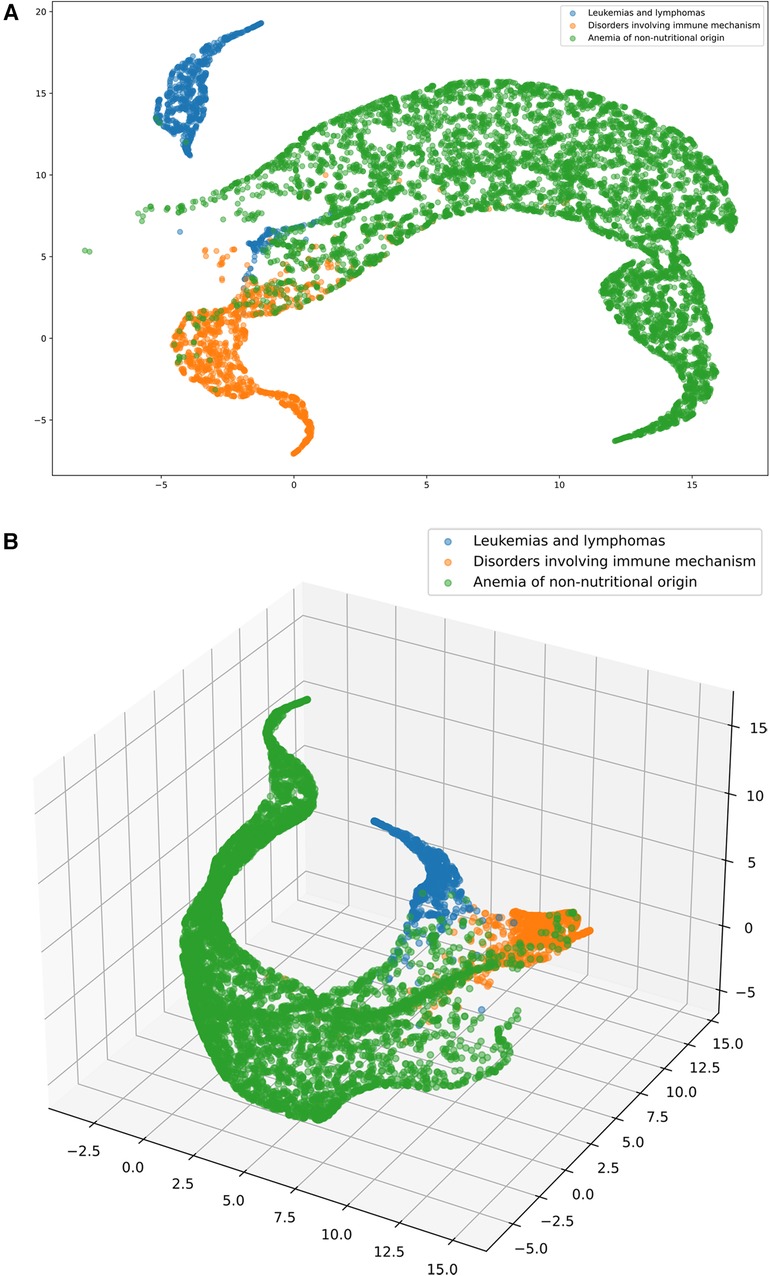
Figure 3. (A and B) The software transforms the blood test results (dots) into a multidimensional feature space to separate major hematological conditions (leukemia and lymphoma - blue, immune disease - yellow, anemia of non-nutritional origin - green). 2D (A, on the left) and 3D (B, on the right) visualization via UMAP. The colors indicate the above mentioned medical conditions. We note that we only selected patients with a single confirmed condition (no comorbidities present) for visualization purposes to clearly show where a patient was assigned.
Our evaluation procedure included various performance metrics, namely, the receiver operating characteristic (ROC) area under the curve (AUC) (14, 17), diagnostic odds ratio (DOR) (18), accuracy, and sensitivity (14); for their definitions and additional details about the evaluation process, see Supplementary Appendix D. After identifying the best hyperparameters per method, we combined the outputs of the best methods for each classifier with an ensemble layer based on adaptive boosting. The final model for a single disease was a linear combination of the six base machine learning methods of all the disease models, see Figure 4.
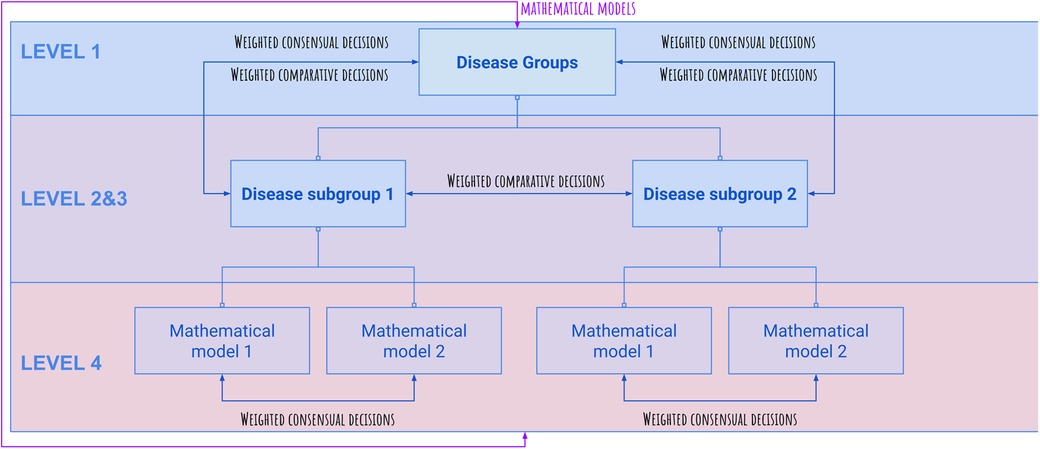
Figure 4. Overview of the framework's tree structure. Comparative (competitive) and consensual decisions are being built up for the final diagnosis of the selected disease group.
3.3 Validating in real practice
Validating in a different clinical environment has been carried out with the aid of Synlab Hungary Ltd. and Medisave Ltd. across Synlab's selected private health facilities in nationwide Hungary to test how a routine blood test interpretation and diagnostic support tool may perform in a real environment involving patients and laboratories of various regions. The test was performed in 2023 and included anonymous data of 8,278 patients in an outpatient setting, who agreed to participate at the time of their independent phlebotomy. Every AI-generated medical report has been thoroughly evaluated by at least two medical professionals before the patient received it and in case of any discrepancy between the AI and MD interpretation, the patient acquired written information about the discrepancy and recommendation by the MD. MDs had the opportunity to stop any AI interpretation being sent out in case they considered the discrepancy significant. MDs first evaluated all patients' blood test results for potential diseases, choosing from three possible states for each disease group: “disease is present”, “disease not present”, or “cannot be decided”. Then they reviewed each AI-generated interpretation at both individual disease levels, with “agree”, “disagree” or “cannot be decided” options to choose from, and rated the AI's performance on the report in general on a 1–5 Likert scale. When the AI interpretation showed the risk for a present rare disease, all concerned patients were offered a medical consultation and follow-up testing, out of which 103 ones were carried out (70 for potential rare disease patients and 33 other patients). Significance was always calculated with 95% C. For data protection reasons and retaining anonymity of data processing the AI considered only the actual blood test result of the patient for the prediction in this testing setup, even if previous test results were available. During the training (model build) of the AI, multiple blood tests related to the same person were used for consideration.
4 Results
We evaluated the performance of the ensemble system on retrospective data via three main approaches. Details of all the approaches can be found in Table 4 and Table 5. The generated performance statistics describe the models' effectiveness in making aggregated predictive decisions tested on retrospective non-training data. We mapped the continuous zero-one logistic score to a one-to-five scale risk score. Sensitivity shows a monotonic increase in this risk score with respect to the probability of the disease being present. This confirms that our model estimates the risk and that is coherent with the real risk. Finally, early detection scores assess the possibility of evaluating the risk of the analyzed disease groups earlier than the clinically validated diagnosis.
4.1 Detection quality
On a diagnostic time scale, the disease appears at an unknown time point, while diagnostic tests lead to an established clinical diagnosis only at a later time point. The tool generally considered only medical cases with a valid diagnosis at the model training stage (only blood tests related to a given diagnosis, either based on HIS data or timely, were used for training). However, we observed that if a broad history of multiple blood tests is available to a person and used for model training, the prediction (ROC-AUC) performance increases significantly, on average +0.0272 ± 0.0213.
Table 5 shows that the sensitivity increases monotonously and proportionately with increasing prediction score (projected to one-to-five), which reinforces the hypothesis that the binary classification works well; the higher the prediction score is, the greater the actual chance of disease presence in a single medical case. As the classification system uses “relevant” medical cases (a set of medical cases that results in a specific diagnosis) during clinical disease progression, by using only routine blood tests, see Table 6 for availability of the blood tests in the data, the software can categorize the clinical manifestations of the examined diseases during this period in time (we also carried out detailed testing outside of our time window for early detection). The classification works well for straightforward diagnoses (e.g., kidney, thyroid, or lipid metabolism disorders), hematology (traditionally performed mainly from a complete blood count panel), and complex diseases. We observed a clear presentation pattern for these pathologies during disease progression, where prediction is possible without disease-specific testing. For many disease groups (primarily for more complex ones, i.e., inflammatory bowel diseases and rare diseases), the classification performance may also vary due to the higher rate of underdiagnosis and misdiagnosis. The ensemble method outperformed the individual methods. For the performance of the individual methods see Table 7 and Table 8. Additionally, we report the Precision-Recall curve for all diseases, see Figure 5.
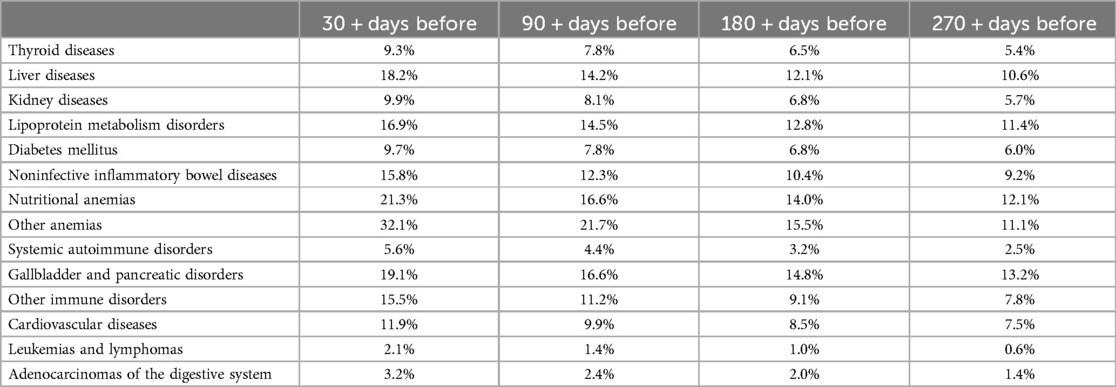
Table 6. Percentage of patients with available blood test results on specific days before the establishment of the diagnosis.
4.2 Early detection
When evaluating the possibility of early detection, we used only “non-relevant” medical cases (lab tests), which cannot be directly associated with the studied disease manifestation. Approximately 10% of patients had “non-relevant” cases 30–360 days before the studied diagnosis was established. This number is generally greater for patients with widespread chronic diseases, complications of other (chronic) diseases, or diseases for which diagnostic procedures are less straightforward. This low number could be due to the low participation rate in screening programs and the fact that our data source healthcare institutes mainly provide more advanced care and fewer screening visits. Despite these limitations, thanks to the large sample size, we still gained useful insight into early screening analysis.
4.3 Rare disease detection
Among the seven rare disease categories, all had reasonably high ROC-AUC values. Diseases with direct or semidirect markers within routine blood work are of exceptionally high value (dyslipidaemias, hepatic and metabolic diseases, hematologic disorders), which is mainly in line with the findings of common disease groups. Even diseases that require special blood testing (e.g., Wilson disease, Cushing's syndrome, or Addison's disease) show a clear pathologic pattern on routine bloodwork that can be used for educated prediction. Table 9 shows the aggregated average performance of the software for each rare disease.
4.4 Importance of different blood tests
Furthermore, with reverse engineering logic, we determined the importance of each laboratory parameter in our AI's diagnosis of each disease group. The data suggest that our AI considers those parameters (laboratory tests) important in confirming or excluding the diagnoses that are also part of the clinical diagnostic definitions in the medical literature, and human medical professionals also consider them in their clinical reasoning. These findings indicate that the AI has revealed similar pathophysiological changes in diseases that have been identified through decades of medical research.
We calculated the importance of individual blood tests in the decision-making of AI in a multistep process in which models were tested multiple times with one or more tests missing from the training. Based on the decrease in the average model performance (ROC-AUC), we created an importance ranking for all the blood tests used: tests whose absence created a greater loss in performance received a higher importance rank. The rank is an average based on the multiple training runs of multiple models. The ranking is described with the score of relative importance, where 100 is the value for the most important test (calculated separately for each examined disease group), and the rest of the tests are represented with a value as a percentage compared to the most important one. High relative importance of a test means that in case that test is missing (e.g., the test was not ordered) then the AI's performance to recognize the disease drops significantly.
The average relative importance, Table 10, of the individual blood test results was measured for all disease groups. The categories show how important the individual test is, on average, for deciding the presence or absence of the disease group.
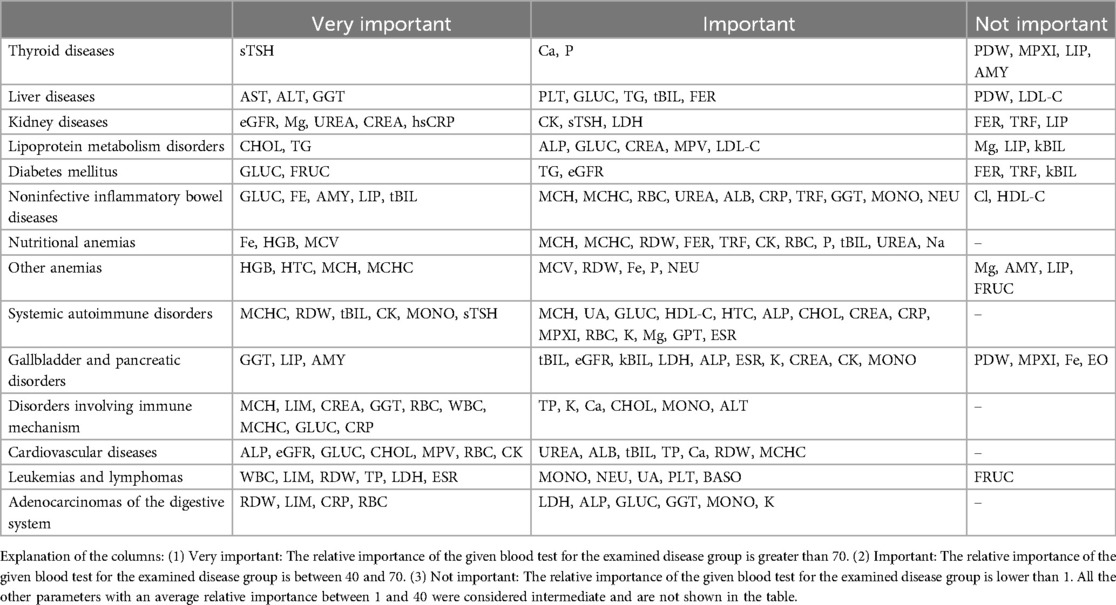
Table 10. The average relative importance of individual blood tests in diagnosing each disease group.
4.5 Prevalence
When comparing the test results on the data of model build to the validation in a different clinical environment, we see that the results not only deliver the same performance measures but sometimes even outperform them, see Table 11. The disease prevalences were similar to the train population. Anomalies can be found for lipid disorders, cardiovascular diseases, nutritional anemias, and immune diseases. Except for non-inflammatory bowel diseases and cardiovascular diseases, medical doctors fully agreed with well above 95% of the diagnostic proposals of the AI on the individual disease groups. The average report score (on a 1–5 Likert scale) was 4.82. The average scores received by laboratory and clinical practitioners are 4.86 and 4.76, respectively, which means a slight, but significant difference in favor of clinical pathologists when adjudging the performance of the AI.
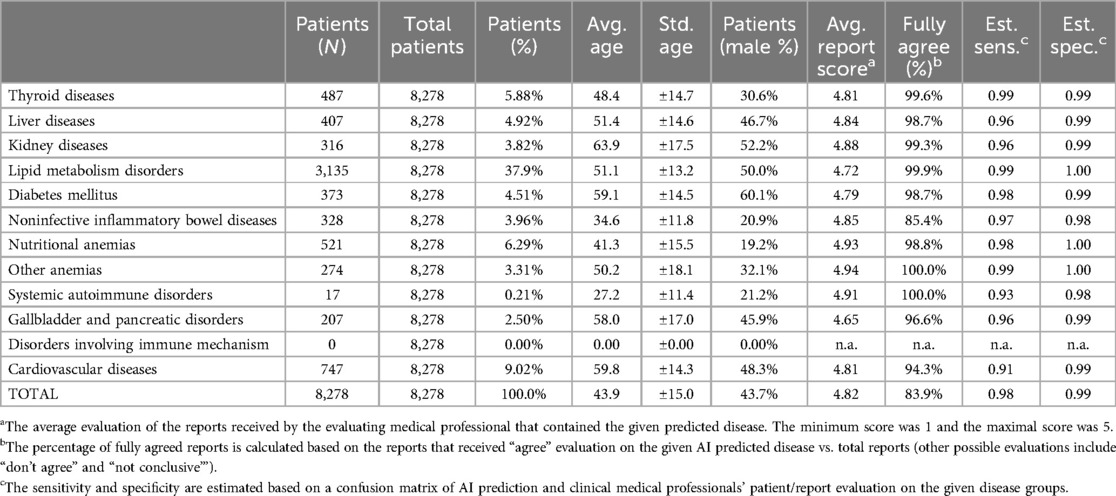
Table 11. General patient statistics and common disease results of the pilot software testing carried out in synlab blood testing facilities across Hungary (all regions) in 2023 with 8,278 outpatients. Every AI-interpreted and evaluated report was cross-evaluated by at least one clinical pathologist and one clinical internist.
Interestingly, though, when assessing the decisions on individual disease levels, we see that clinical internists agree significantly more with the AI decisions (98,4% vs. 96,0%). We also measured the time needed for a detailed medical evaluation of all medical reports without (not considering AI-generated reports) and with AI-aided generation reports. We found that with the help of the AI, the report validation time decreased significantly by 50.7% (from 645s to 318s on average).
When assessing potential performance with rare diseases/conditions, Table 12, we checked the estimated prevalence of a certain disease and how it is related to the prevalence in the literature while also appraising the precision based on the medical consultations of real patients. FH and Gilbert cases showed very high estimated precision (95%+), while hemochromatosis also showed an acceptable hit ratio (76%). Other diseases had few or no potential patients, so deeper evaluation was not possible.
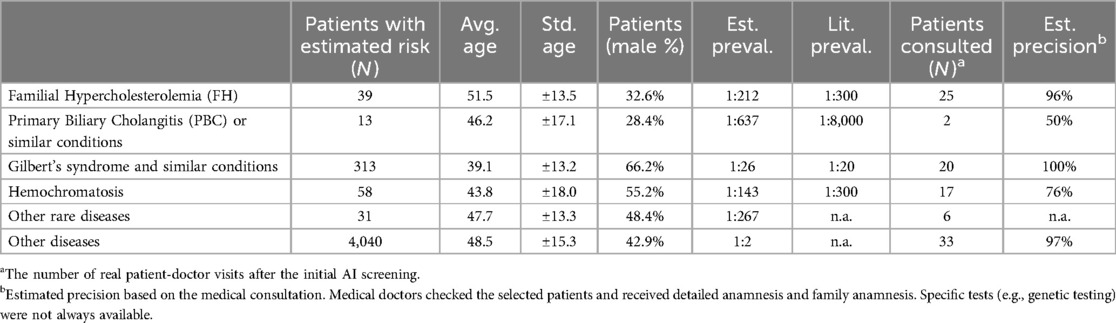
Table 12. Rare disease predictions during the pilot testing and patient statistics where the software predicted at least 3 out of 5 risk scores. 103 patients participated in medical consultations and checkups where screening precision was estimated.
5 Discussion
The importance of laboratory tests in medical diagnostic processes is undisputed. Recent surveys show that laboratory test results influence 60%–70% of clinical decisions (19), and are embedded in at least 80% of guidelines, which are aimed at establishing a diagnosis or managing a disease (20). The low error rate of laboratory tests makes them a solid base for AI-supported medical diagnostic processes. Numerous studies have shown that laboratory tests can be used to effectively identify chronic diseases or infections (21–25). Similar to these results, our findings demonstrate that machine learning can extend diagnostic capabilities to a broad range of conditions, including rare diseases.
Our findings indicate that AI-aided screening can detect diseases up to six to nine months earlier than current clinical diagnoses, consistent with emerging trends in AI applications for early diagnosis. However, some state-of-the-art methods focus on radiological or imaging-based data, whereas our approach leverages only routine blood test results, which are more readily accessible.
We previously showed that machine learning is ideal for diagnosing rare dyslipidemias (26, 27), but analyzing a wider set of rare diseases shows that many rare diseases may also be ideal candidates for computer-aided screening efforts, even those that usually require (multiple) specific diagnostic tests for confirming the diagnosis. Our initial models were promising to further evaluate the diagnostic opportunities of AI models on laboratory tests, see Table 11. Both prevalence estimation and the estimated precision align with the original calculations and expectations.
We tested the tool's general capability for early screening of supported diseases by evaluating the blood test results of patients who presented regularly at hospitals for screening or other purposes but had not yet received an official diagnosis. As shown in Table 3 in the Results section, routine test-based AI-aided diagnostics can boost early screening efforts, save time, and may improve patient outcomes. The model's performance statistics did not, or only slightly, decrease within six months before medical diagnosis. Our AI-aided blood test interpretation can diagnose most of the analyzed disease groups at least one-to-six or even one-to-nine months before the current traditional clinical diagnosis. A significant decrease in early diagnostic performance was detected only in more acute disease groups, such as liver disease and some cardiovascular pathologies.
Regional and hospital-specific changes and patient behavior (e.g., frequent hospital goers) can impact the time point of possible early diagnosis. The earliest point at which a disease can be diagnosed is also a question of debate, as most of the time, exact thresholds of parameters are to be met for definitive diagnosis. However, we can achieve an earlier diagnosis with more frequent follow-ups for patients in the “predefinitive disease stage”. Furthermore, guideline updates may later include these “predefinitive disease stages” with early treatment options to improve outcomes. Our data suggest that many common diseases may be diagnosed 30–90 days before clinical diagnosis via AI-aided evaluation based on routine phlebotomy results, see Table 4. Our results also indicate that diagnosis earlier than 180 days will be possible in only a few medical cases without the use of other specific blood markers. On the national level, significant savings may come from using AI-aided inexpensive and nonspecific blood test evaluation for early diagnosis; thus, involving AI in laboratory result evaluation may facilitate the much-needed paradigm shift in healthcare toward early diagnosis, treatment, and prevention.
The list of laboratory tests included in our AI analysis covers screening laboratory tests that are commonly used worldwide: basic and complete metabolic panels (ALP, ALT, AST, bilirubin, BUN, creatinine, sodium, potassium, chloride, albumin, total protein, glucose, and calcium); complete blood counts with differential; and screening tests for lipid, iron, hepatic and thyroid metabolism. These tests are among the most frequently ordered tests (28), allowing easy translation of our findings to help efficiently screen a wide range of diseases.
The common laboratory tests used as input are part of routine phlebotomy due to their importance in diagnosing many acute and chronic diseases. For example, TSH tests are widely recommended for first-line thyroid dysfunction screening (29, 30). Moreover, AST, ALP, GGT, and bilirubin levels alone may indicate the correct type and etiology of liver disease, allowing for a targeted investigative approach during clinical examination and further diagnostic testing (31). Although these tests are part of general medical education and common medical knowledge, the clinical interpretations of these tests are far from trivial (31, 32). In addition to diagnosing acute liver pathologies, proper evaluation via liver tests also helps diagnose Wilson's disease; thus, liver tests can distinguish rare pathologies from common medical conditions (31). A comparison of certain blood analysis methods and other factors may also raise issues that are difficult to handle and may lead to misinterpretation or misdiagnosis. For example, sTSH test reference ranges and values are highly sensitive to measurement technology, circadian patterns, analytical platforms, and geographic regions (33). Therefore, following AI-aided analysis, including interpretative comments in laboratory reports, could decrease error rates, thus improving the quality of laboratory information and patient safety (34). Although the major driver for including interpretative comments is new and complex tests in laboratory reports (35), interpretation of common laboratory tests is also welcome. In a survey in the UK, 88% of primary care doctors and nurse practitioners found interpretative comments on the thyroid, gonadotropin, and glucose tolerance test reports helpful (36). AI-supported decision-making may also decrease the rate of missed or late diagnoses originating from HCPs' incorrect interpretation of even basic laboratory tests. Self-reported testing practices for anemia include the overuse of screening laboratory tests, the underuse of bidirectional endoscopy to evaluate new-onset iron deficiency anemia, and the misinterpretation of iron studies, which were also included in our analysis (37). As elaborated in (4, 19–25, 28–32), laboratory testing is a major part of the current SOTA diagnostics for all major common and rare diseases. AI is anticipated to enhance diagnostic accuracy while simultaneously reducing the workload of medical professionals in the interpretation of laboratory results. Fitting AI to the current SOTA should be a focal point of further research and development of tailored protocols for each individual disease.
The data validation in a private health clinical environment confirmed the hypotheses and findings on the retrospective model-build data (both with common and rare diseases). Patient age histograms, see Figure 6, and disease prevalences show that the clinical test population and the training population differ significantly. However, the ensemble was robust enough to perform well on the different populations. The differences in disease prevalences can be explained by considering that the training data originated from tertiary and quaternary care hospital data, while the clinical test was carried out in private outpatient healthcare (less complicated cases and generally healthier patients). We did not find a satisfactory explanation for the major discrepancy regarding immune diseases. This has to be further evaluated in the future from both medical and informatic aspects. All participating medical doctors regarded very highly the diagnostic evaluations of the AI. Though the clinical pathologists gave higher scores to the system, the clinical internists agreed more with the individual disease decisions. The differences were significant but not large. This might show differences in approach toward technology among the medical professions, but this has to be further evaluated.
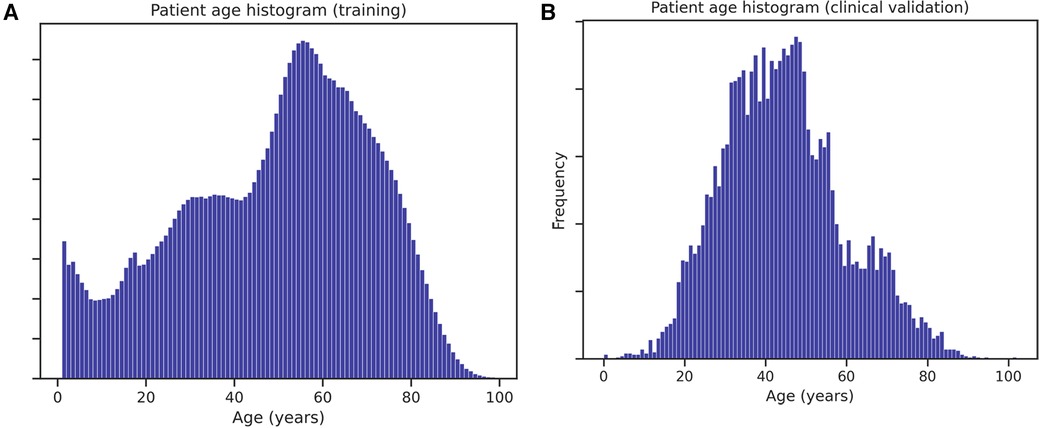
Figure 6. Age histogram of patients in the validation set (A) and in the historical data used for training the models (B).
5.1 Limitations
Initial results are promising, several limitations must be considered. First, our study's dataset, while comprehensive, was limited to a couple of regional hospital sites. Larger and more diverse datasets from different regions and healthcare settings would provide a better understanding of AI's potential across various demographics and testing platforms.
It is also important to note that we obtained the lowest amount of early diagnostic data (i.e., people's participation in screening programs) for malignancies, a category where early diagnosis is especially critical for effective therapy and where our findings show the possibility of very effective AI-assisted early screening.
Despite the promising results, discrepancies between AI-aided blood test interpretations and current medical diagnoses must be acknowledged. Traditional diagnostic approaches integrate multi-modal data sources (e.g., medical history, physical examination and imaging), whereas our model is limited to laboratory data.
6 Conclusion
This study demonstrates the potential of AI-aided decision support systems in disease classification using only routine blood tests, offering valuable contributions to early diagnosis and healthcare efficiency.
Our model is ab ovo different from related works (7–13) as our model does not focus on a selected disease but is meant to detect a large number of diseases and conditions. We demonstrated that robust mathematical models and ensemble methods can yield reliable disease classifications across diverse patient populations and laboratory settings. We found that machine learning is strongly capable of recognizing pathological blood test result patterns and integrating AI into laboratory analysis could lead to earlier diagnoses, improved patient outcomes, and a more cost-efficient healthcare system. The novelty of our approach lies in (1) using only routine laboratory tests for AI-based early screening (2) allowing the models to train through the entire patient history timeline (not just on diagnostic “snapshots”) (3) focusing on broad disease categorization to provide medical personnel a generic instead of a specific diagnostic aid, and (4) combining multiple models to create a robust ensemble which proven to work across multiple laboratories and regions. This is a valuable advancement over existing developments, which often focus on more specific diagnostic tests for disease confirmation. However, when comparing existing and recent studies in this field with our method, see Related work, we also see many similarities. For instance, in (12) the authors also used routine blood tests for differentiating acute leukemia subtypes (except for prothrombin time and fibrinogen, which we did not include in most common ones, the blood tests are similar to our initial feature set), and found that the best models were gradient boosting with the similar performance which we reported. We both found MCV, LDH, and MONO# as features of key importance. However, we rigorously focused on not using any other features but blood tests (e.g., age). In (9, 10, 13) the authors used similar machine learning models for classification of bacterial infections, Malaria, Alzheimer's disease and reported similarly high AUC values however these studies were either limited in the size of dataset (10, 13) or their attributes included specific blood tests (9, 10, 13).
Implementation of prescreening with AI can reduce screening costs and improve patient selection for personalized treatments. Moreover, the ability to utilize retrospective data enhances the immediate applicability, offering substantial benefits to both patients and healthcare providers. At the national or payee level, healthcare may become more economical by using AI-aided inexpensive and nonspecific blood test evaluations for early diagnosis. Future work should focus on expanding the dataset to include a broader and more diverse patient population, integrating multi-modal data sources.
Data availability statement
The data analyzed in this study is subject to the following licenses/restrictions: Both source code and source medical data are proprietary technology and can not be shared publicly. However, for clinical and research use, the developed software is accessible free of charge to non-profit medical organizations (using standard HL7 communication of blood test data and outputting standard textual JSON/HL7 with disease/disease group predictions both qualitatively - one and zero - and quantitatively with estimated risk percentages). Therefore, the software may be made fully available based on a request to the corresponding authors or the UDCC Department of Internal Medicine (https://belklinika.unideb.hu/en). Requests to access these datasets should be directed toZGFyb2N6eWJAaWxhYi5zenRha2kuaHU=.
Ethics statement
The study was carried out as a part of University of Debrecen Clinical Center's data mining and machine learning initiative under ethical permission TUKEB 44549-2/2018/EKU and DE RKEB/IKEB 6697-2024. The studies were conducted in accordance with the local legislation and institutional requirements. The human samples used in this study were acquired from a by- product of routine care or industry. Written informed consent for participation was not required from the participants or the participants' legal guardians/next of kin in accordance with the national legislation and institutional requirements.
Author contributions
ÁN: Conceptualization, Data curation, Formal Analysis, Investigation, Methodology, Software, Supervision, Validation, Visualization, Writing – original draft, Writing – review & editing. GT: Formal Analysis, Writing – original draft. PF: Validation, Writing – review & editing. GP: Writing – review & editing, Supervision. BN: Writing – review & editing, Validation. ZK: Writing – review & editing, Methodology. GP: Writing – review & editing, Supervision. ZH: Writing – review & editing, Formal Analysis. ZC: Formal Analysis, Writing – review & editing. EP: Formal Analysis, Writing – review & editing. DS: Methodology, Writing – review & editing. GB: Formal Analysis, Writing – review & editing. IÉ: Supervision, Writing – review & editing. JK: Supervision, Writing – review & editing. MH: Supervision, Writing – review & editing. BD: Conceptualization, Data curation, Formal Analysis, Methodology, Software, Supervision, Validation, Visualization, Writing – original draft, Writing – review & editing.
Funding
The author(s) declare financial support was received for the research, authorship, and/or publication of this article. This research was funded by public channels. G. Toth received funding through the Society to Improve Diagnosis in Medicine's fellowship program, which is financially supported by the Gordon and Betty Moore Foundation. B. Daróczy received funding from the European Union project RRF-2.3.1-21-2022-00004 within the framework of the Artificial Intelligence National Laboratory and from the C.N.R.S. E.A.I. project “Stabilité des algorithmes d’apprentissage pour les réseaux de neurones profonds et récurrents en utilisant la géométrie et la théorie du contrôle via la compréhension du rôle de la surparamétrisation”. The study was supported by the National Research, Development, and Innovation Office (NKFIH, grant number: K142273). The funders played no role in the study design, data collection, analysis and interpretation of data, or the writing of this article. Synlab Hungary Ltd. was involved in the clinical testing as a testing partner and facilitator but it neither funded the project nor owns any IP related to it. Synlab Hungary provided validating clinical pathologists in their employment for the testing.
Conflict of interest
ÁN, DS, BD were employed by Black Horse Group Ltd. ZC, EP were employed by Synlab Group (Synlab Hungary Ltd.).
The remaining authors declare that the research was conducted in the absence of any commercial or financial relationships that could be construed as a potential conflict of interest.
Publisher's note
All claims expressed in this article are solely those of the authors and do not necessarily represent those of their affiliated organizations, or those of the publisher, the editors and the reviewers. Any product that may be evaluated in this article, or claim that may be made by its manufacturer, is not guaranteed or endorsed by the publisher.
Supplementary material
The Supplementary Material for this article can be found online at: https://www.frontiersin.org/articles/10.3389/fdgth.2024.1505483/full#supplementary-material
Abbreviations
ALP, alkaline phosphatase; ALT, alanine transaminase; AST, aspartate transaminase; AUC, area under curve; BUN, blood urea nitrogen; DOR, diagnostic odds ratio; ROC, receiver-operating characteristic; TSH, thyroid-stimulating hormone; UDCC, University of Debrecen Clinical Center; UMAP, Uniform Manifold Approximation and Projection; SOTA, state-of-the-art.
References
1. Barwise A, Leppin A, Dong Y, Huang C, Pinevich Y, Herasevich S, et al. What contributes to diagnostic error or delay? A qualitative exploration across diverse acute care settings in the US. J Patient Saf. (2021) 17(4):239–48. doi: 10.1097/PTS.0000000000000817
2. Graber ML, Franklin N, Gordon R. Diagnostic error in internal medicine. Arch Intern Med. (2005) 165(13):1493–9. doi: 10.1001/archinte.165.13.1493
3. Car LT, Papachristou N, Bull A, Majeed A, Gallagher J, El-Khatib M, et al. Clinician-identified problems and solutions for delayed diagnosis in primary care: a PRIORITIZE study. BMC Fam Pract. (2016) 17(1):131. doi: 10.1186/s12875-016-0530-z
4. Carraro P, Plebani M. Errors in a stat laboratory: types and frequencies 10 years later. Clin Chem. (2007) 53(7):1338–42. doi: 10.1373/clinchem.2007.088344
5. Hobson-Webb LD, Kishnani PS. How common is misdiagnosis in late-onset Pompe disease? Muscle Nerve. (2012) 45(2):301–2. doi: 10.1002/mus.22296
6. Yu KH, Beam AL, Kohane IS. Artificial intelligence in healthcare. Nat Biomed Eng. (2018) 2(10):719–31. doi: 10.1038/s41551-018-0305-z
7. Santos-Silva MA, Sousa N, Sousa JC. Artificial intelligence in routine blood tests. Front Med Eng. (2024) 2:1369265. doi: 10.3389/fmede.2024.1369265
8. Rawson TM, Hernandez B, Moore LS, Blandy O, Herrero P, Gilchrist M, et al. Supervised machine learning for the prediction of infection on admission to hospital: a prospective observational cohort study. J Antimicrob Chemother. (2019) 74(4):1108–15. doi: 10.1093/jac/dky514
9. Mooney C, Eogan M, Ní Áinle F, Cleary B, Gallagher JJ, O'Loughlin J, et al. Predicting bacteraemia in maternity patients using full blood count parameters: a supervised machine learning algorithm approach. Int J Lab Hematol. (2021) 43(4):609–15. doi: 10.1111/ijlh.13434
10. Morang’a CM, Amenga–Etego L, Bah SY, Appiah V, Amuzu DS, Amoako N, et al. Machine learning approaches classify clinical malaria outcomes based on haematological parameters. BMC Med. (2020) 18:1–6. doi: 10.1186/s12916-019-1443-1
11. Lu Z, Morita M, Yeager TS, Lyu Y, Wang SY, Wang Z, et al. Validation of artificial intelligence (AI)-assisted flow cytometry analysis for immunological disorders. Diagnostics. (2024) 14(4):420. doi: 10.3390/diagnostics14040420
12. Alcazer V, Le Meur G, Roccon M, Barriere S, Le Calvez B, Badaoui B, et al. Evaluation of a machine-learning model based on laboratory parameters for the prediction of acute leukaemia subtypes: a multicentre model development and validation study in France. The Lancet Digital Health. (2024) 6(5):e323–33. doi: 10.1016/S2589-7500(24)00044-X
13. Loveleen G, Mohan B, Shikhar BS, Nz J, Shorfuzzaman M, Masud M. Explanation-driven HCI model to examine the mini-mental state for Alzheimer’s disease. ACM Trans Multimed Comput Commun Appl. (2023) 20(2):1–6. doi: 10.1145/3527174
14. Baeza-Yates R, Ribeiro-Neto B, Ribeiro BAN. Modern Information Retrieval. New York: ACM Press (1999). p. 548.
15. Devlin J, Chang MW, Lee K, Toutanova K. BERT: pre-training of deep bidirectional transformers for language understanding. (2019). p. 4171–86. Available online at: https://aclanthology.org/N19-1423 (cited August 28, 2023).
16. McInnes L, Healy J, Saul N, Großberger L. UMAP: uniform manifold approximation and projection. J Open Source Softw. (2018) 3(29). Available online at: https://par.nsf.gov/biblio/10104557-umap-uniform-manifold-approximation-projection (cited September 20, 2023). doi: 10.21105/joss.00861
17. Tan PN, Steinbach M, Karpatne A, Kumar V. Introduction to Data Mining. London: Pearson (2019). p. 839.
18. Glas AS, Lijmer JG, Prins MH, Bonsel GJ, Bossuyt PMM. The diagnostic odds ratio: a single indicator of test performance. J Clin Epidemiol. (2003) 56(11):1129–35. doi: 10.1016/S0895-4356(03)00177-X
19. Rohr UP, Binder C, Dieterle T, Giusti F, Messina CGM, Toerien E, et al. The value of in vitro diagnostic testing in medical practice: a Status report. PLoS One. (2016) 11(3):e0149856. doi: 10.1371/journal.pone.0154008
20. The Lewin Group. The value of diagnostic innovation, adoption and diffusion into health care (2005). Available online at: https://www.lewin.com/content/dam/Lewin/Resources/Site_Sections/Publications/ValueofDiagnostics.pdf (accessed September 17, 2017).
21. Brinati D, Campagner A, Ferrari D, Locatelli M, Banfi G, Cabitza F. Detection of COVID-19 infection from routine blood exams with machine learning: a feasibility study. J Med Syst. (2020) 44(8):135. doi: 10.1007/s10916-020-01597-4
22. Gunčar G, Kukar M, Notar M, Brvar M, Černelč P, Notar M, et al. An application of machine learning to haematological diagnosis. Sci Rep. (2018) 8:411. doi: 10.1038/s41598-017-18564-8
23. Alsheref FK, Gomaa WH. Blood diseases detection using classical machine learning algorithms. Int J Adv Comput Sci Appl IJACSA. (2019) 10(7):77–81. Available online at: https://thesai.org/Publications/ViewPaper?Volume=10&Issue=7&Code=IJACSA&SerialNo=12 (cited September 20, 2023). doi: 10.14569/IJACSA.2019.0100712
24. AlJame M, Ahmad I, Imtiaz A, Mohammed A. Ensemble learning model for diagnosing COVID-19 from routine blood tests. Inform Med Unlocked. (2020) 21:100449. doi: 10.1016/j.imu.2020.100449
25. Aslan MF, Celik Y, Sabanci K, Durdu A. Breast cancer diagnosis by different machine learning methods using blood analysis data. Int J Intell Syst Appl Eng. (2018) 6(4):289–93. doi: 10.18201/ijisae.2018648455
26. Paragh G, Harangi M, Karányi Z, Daróczy B, Németh Á, Fülöp P. Identifying patients with familial hypercholesterolemia using data mining methods in the northern great plain region of Hungary. Atherosclerosis. (2018) 277:262–6. doi: 10.1016/j.atherosclerosis.2018.05.039
27. Németh Á, Harangi M, Daróczy B, Juhász L, Paragh G, Fülöp P. Identifying patients with familial chylomicronemia syndrome using FCS score-based data mining methods. J Clin Med. (2022) 11(15):4311. doi: 10.3390/jcm11154311
28. Horton S, Fleming KA, Kuti M, Looi LM, Pai SA, Sayed S, et al. The top 25 laboratory tests by volume and revenue in five different countries. Am J Clin Pathol. (2019) 151(5):446–51. doi: 10.1093/ajcp/aqy165
29. Garber JR, Cobin RH, Gharib H, Hennessey JV, Klein I, Mechanick JI, et al. Clinical practice guidelines for hypothyroidism in adults: cosponsored by the American Association of Clinical Endocrinologists and the American Thyroid Association. Endocr Pract. (2012) 18(6):988–1028. doi: 10.4158/EP12280.GL
30. Esfandiari NH, Papaleontiou M. Biochemical testing in thyroid disorders. Endocrinol Metab Clin North Am. (2017) 46(3):631–48. doi: 10.1016/j.ecl.2017.04.002
31. Agrawal S, Dhiman RK, Limdi JK. Evaluation of abnormal liver function tests. Postgrad Med J. (2016) 92(1086):223–34. doi: 10.1136/postgradmedj-2015-133715
32. Metra BM, Guglielmo FF, Halegoua-DeMarzio DL, Civan JM, Mitchell DG. Beyond the liver function tests: a radiologist’s guide to the liver blood tests. RadioGraphics. (2022) 42(1):125–42. doi: 10.1148/rg.210137
33. Soh SB, Aw TC. Laboratory testing in thyroid conditions - pitfalls and clinical utility. Ann Lab Med. (2019) 39(1):3–14. doi: 10.3343/alm.2019.39.1.3
34. Sciacovelli L, Aita A, Padoan A, Pelloso M, Antonelli G, Piva E, et al. Performance criteria and quality indicators for the post-analytical phase. Clin Chem Lab Med. (2016) 54(7):1169–76. doi: 10.1515/cclm-2015-0897
35. Vasikaran S, Sikaris K, Kilpatrick E, French J, Badrick T, Osypiw J, et al. IFCC WG harmonization of quality assessment of interpretative comments. Assuring the quality of interpretative comments in clinical chemistry. Clin Chem Lab Med. (2016) 54(12):1901–11. doi: 10.1515/cclm-2016-0709
36. Barlow IM. Are biochemistry interpretative comments helpful? Results of a general practitioner and nurse practitioner survey. Ann Clin Biochem. (2008) 45(Pt 1):88–90. doi: 10.1258/acb.2007.007134
Keywords: blood test analysis, chronic diseases, rare diseases, machine learning, prevention and control, classification
Citation: Németh Á, Tóth G, Fülöp P, Paragh G, Nádró B, Karányi Z, Paragh G Jr., Horváth Z, Csernák Z, Pintér E, Sándor D, Bagyó G, Édes I, Kappelmayer J, Harangi M and Daróczy B (2024) Smart medical report: efficient detection of common and rare diseases on common blood tests. Front. Digit. Health 6:1505483. doi: 10.3389/fdgth.2024.1505483
Received: 2 October 2024; Accepted: 18 November 2024;
Published: 5 December 2024.
Edited by:
Xiaofeng Li, Heilongjiang International University, ChinaReviewed by:
Lambert Zixin Li, Stanford University, United StatesMohan Bhandari, Samridhhi College, Nepal
Copyright: © 2024 Németh, Tóth, Fülöp, Paragh, Nádró, Karányi, Paragh, Horváth, Csernák, Pintér, Sándor, Bagyó, Édes, Kappelmayer, Harangi and Daróczy. This is an open-access article distributed under the terms of the Creative Commons Attribution License (CC BY). The use, distribution or reproduction in other forums is permitted, provided the original author(s) and the copyright owner(s) are credited and that the original publication in this journal is cited, in accordance with accepted academic practice. No use, distribution or reproduction is permitted which does not comply with these terms.
*Correspondence: Bálint Daróczy, ZGFyb2N6eWJAaWxhYi5zenRha2kuaHU=