- Department of Medicine, Hadassah Hebrew University Medical Center, Jerusalem, Israel
Artificial intelligence (AI) digital health systems have drawn much attention over the last decade. However, their implementation into medical practice occurs at a much slower pace than expected. This paper reviews some of the achievements of first-generation AI systems, and the barriers facing their implementation into medical practice. The development of second-generation AI systems is discussed with a focus on overcoming some of these obstacles. Second-generation systems are aimed at focusing on a single subject and on improving patients' clinical outcomes. A personalized closed-loop system designed to improve end-organ function and the patient's response to chronic therapies is presented. The system introduces a platform which implements a personalized therapeutic regimen and introduces quantifiable individualized-variability patterns into its algorithm. The platform is designed to achieve a clinically meaningful endpoint by ensuring that chronic therapies will have sustainable effect while overcoming compensatory mechanisms associated with disease progression and drug resistance. Second-generation systems are expected to assist patients and providers in adopting and implementing of these systems into everyday care.
Introduction
The use of artificial intelligence (AI)-based systems in medicine has drawn much attention over the last decade (1). However, high expectations have not always been met by reality. Various obstacles have arisen, blocking AI platforms' validation and implementation (2). AI-based algorithm applications have been slower to spread in medical practice than anticipated. Obstacles include difficulty in achieving adequate algorithm stability, mainly due to difficulties associated with big data analysis, and also the failure of these methods to contribute to noteworthy clinical effects. The need for improved clinical outcomes, is a barrier to the adoption of AI by patients and healthcare providers (3–7).
The AI “quadruple aim” of improving care, improving overall population health, reducing healthcare costs, and improving the work life of healthcare providers, is still an unreachable objective for the majority of first-generation systems (8). These platforms were designed to promote the 4P model of medicine: Predictive, Preventive, Personalized, and Participatory, providing patient autonomy (9). This paper reviews some achievements of first-generation systems used over the last decade, and discusses difficulties of implementing them in practice. The development of second-generation platforms in the upcoming years may overcome some of these hurdles. Second-generation systems aim at focusing on a single subject and on improving clinically meaningful endpoints and clinical outcomes. This makes their implementation in everyday care more viable. Establishment of personalized closed-loop platforms, which improve organ function and response to chronic therapies, is presented, with the introduction of individualized patterns of variability discussed as an example application for second-generation AI.
Benefits of First-Generation Artificial Intelligence Platforms in Clinical Practice
AI has been proposed to serve in augmented medicine platforms to improve different aspects of clinical practice. However, AI's real world utilization in clinical practice is limited (1, 10–14). Most algorithms do not inevitably result in better outcomes (10). The term “AI chasm” is sometimes used, and this term suggests that the improved accuracy sought by most platforms does not necessarily represent better clinical efficacy (15). Unsurprisingly, a lack of clear beneficial effects is a major obstacle to adoption of AI in clinical medicine.
First-generation AI systems developed over the last decade have largely focused on clinical decision making through big data analysis as a way to generate evidence-based information. These include supervised machine learning, where the algorithm based on a labeled dataset, provides a model that the algorithm can use to determine its accuracy on training data (16); An unsupervised modeling which provides unlabeled data that the algorithm attempts to fit by extracting patterns on its own (17); recommender systems that seeks to predict the preference a user would give to an item (18); expert systems which attempt to match the decision-making ability of a human expert (19); natural language processing which process and analyze large amounts of natural language data (20); computer vision which analyzes data from digital images or videos (21); and expert-guided feature extraction schemes, where derived features are based on an initial set of measured data for facilitating subsequent learning and generalization steps (22, 23).
Machine learning (ML)-based techniques and neural networks can rapidly process numerous inputs. Thus, they can discover complex associations which are not easily analyzed by regular equations, which assists in reaching better clinical conclusions (1, 24–26). Electronic medical records (EMR) and digital databases are used for these purposes (27–29). Precision medicine-based AI is aimed at optimizing diagnostic pathways, therapeutic interventions, and prognoses using large datasets comprising individual genes, other phenotypic parameters, and environmental measures. The algorithms are largely designed to tailor their outputs on an individual basis (30).
A relatively limited number of settings in clinical practice currently benefit from the application of first-generation systems. A review of 450 published AI articles highlighted four areas: risk stratification of populations at risk, clinical decision support, disease screening, and tools for patient self-management (31). First-generation AI can reduce variations in clinical practice, improve efficacy and reduce medical errors (32). It has shown advantages when extracting and analyzing a large volume of data from EMR (33), developing risk scores (34), predicting mortality, readmission risks, prolonged length of stay, and failure to attend appointments (35, 36), and for summarizing doctor-patient consultations (10, 37).
First-generation systems have also been found useful for detection of atrial fibrillation and epilepsy seizures (11). These methods also showed some degree of success when interpreting phenotype and genomic data for genomics-based diagnosis (38), and for assessment of cancer risk (39–42). Benefits were also documented for identifying cancerous skin lesions (43–45), diagnosing cancer in computational histopathology (46), interpreting retinal imaging (47–51), detecting arrhythmias (52, 53), polyp detection from colonoscopy (54), identifying genetic conditions from facial appearance (10, 55), predicting acute kidney injury (56), detecting epilepsy (57), process images from endoscopy (58, 59), and predicting outcomes of gastrointestinal bleeding (58, 59). Some advantages were likewise shown for decision making in sepsis management (60), and in pharmacogenomics (61, 62). Even self-monitoring of blood glucose can be facilitated by AI (63), helping to improve blood glucose control, reduce hypoglycemic episodes, and reduce diabetes-related complications (31). The resulting “do it yourself” automated insulin delivery for diabetics exemplifies democratization of medicine by providing privacy, intelligent computing, and sharing of information (64).
In the field of imaging, AI showed benefits for interpreting chest radiographs (65–67), detecting cancer in mammograms (68), analyzing computer tomography scans (69, 70), identifying brain tumors in magnetic resonance images (71), and predicting the development of Alzheimer's disease from positron emission tomography (72). AI can also assist radiologists by identifying abnormal or negative exams. Centralized interpretation of chest radiographs can compensate for a shortage of radiologists in under-resourced areas (73). Web-based applications can be used to augment psychiatric services (74). Smartphone-based applications are used to improve adherence to medications, and to monitor heart rate, activity levels, sleep levels, and electrocardiogram (ECG) tracings (75, 76). Algorithms outperformed cardiologists in diagnosing heart attacks by ECG (77), and outperformed dermatologists in classifying skin lesions as benign or malignant, due to their rapid learning from multiple cases (43). These algorithms also performed better than experts in analyzing pulmonary tuberculosis in chest radiographs (78).
In addition to these applications, AI can support preventative medicine by determining the risks for complications that would warrant intervention. Patients identified as low risk receive reassurance while high-risk patients are referred for intervention (1). AI is used for precision therapeutics, for repurposing drugs, and for drug development, by analyzing large volumes of data (30). Several algorithms have yielded unanticipated findings, such as predicting breast cancer prognosis using stromal cells (79), predicting cardiovascular risks using fundus photographs (80), identifying atrial fibrillation from ECGs acquired during sinus rhythm (53), and earlier diagnosis of dementia using retinal imaging (10, 81).
Many of the studies described above were retrospective and used historical data for training the algorithms. However, prospective studies are required to prove software's utility in real world. Prospective studies have shown limited benefit in diabetic retinopathy grading (82–84), detection of breast cancer metastases in lymph node biopsies (85), wrist fracture detection (86), colonic polyp detection (87), and detection of atrial fibrillation in Apple watch users (10, 88). Results have been shown when predicting risk of certain cancers (39, 89, 90) and for cardiovascular diseases (80, 91). Lesser results have been noted for epilepsy, and for neurodevelopmental disorders, including intellectual disability and autism spectrum disorder (30, 92, 93).
Only a few AI-based randomized controlled trials (RCTs) have been conducted to date. These include a trial to detect childhood cataracts which showed lower performance compared to senior clinicians (94), a single-blinded RCT that demonstrated reduced blind-spot rate in gastroscopy (95), an open non-blinded trial for polyp detection by colonoscopy which showed improved detection rate of diminutive adenomas and hyperplastic polyps (96), a trial to detect acute neurologic events (97), and an open trial for analyzing cardiotocographs during labor that showed no improvement in clinical outcomes (98).
Overall, the data have shown some benefits of first-generation platforms for certain tasks with a defined input and a binary output (1).
Obstacles Faced by First-Generation AI Systems Associated With Limited Penetration Into Clinical Practice
The data used by the first-generation systems are a major cause of difficulties. Large databases sometimes lack well-structured training sets that are stable over time. Many of the current algorithms lack the ability to make clinically relevant associations when used for analysis of EMR, physicians' notes, natural language processing (NLP), identification of patterns within datasets, or attempts to associate patients' phenotypes and genotypic markers (30, 99). Sources may suffer from the absence of a unifying data format across centers (100). Batch effect technology biases are obstacles in analyzing population scale omics and imaging data (101–103). Inadvertent discriminatory bias, inapplicability outside of training domains, and propagation of unintentional biases in clinical practice all affect outputs. Moreover, a lack of valid interpretability and of reliable measures for model confidence affects accuracy (1, 10). Input data is produced within non-stationary settings with shifting patient populations, and changes can occur in clinical practices. Dataset shifts, which happens when test and training inputs and outputs have different distributions, commonly occur when these realities are disregarded (10, 104).
The retrospective designs of most studies and their limited sample sizes lead to selection bias and overfitting. Selection bias is introduced by selection of data in a way that proper randomization is not achieved, thereby ensuring that the sample obtained is not representative. Overfitting is a modeling error that occurs when a function is too closely fit to a limited set of data points (10, 58, 105). Small sample sizes also lead to marked variability in predicting risk, such as for cardiovascular disease, decline of glomerular filtration rate in polycystic kidney disease, and risk of progressive IgA nephropathy (106, 107). Using fitting confounders rather than true signals, unintentional fitting of confounders, or “over-fitting” may impact the accuracy of output. Accidentally fitting confounders vs. true signals or simply using whatever signals are available to achieve the best performance in a dataset are ML practices associated with bias (10). AI may give undue importance to spurious correlations within past data, as exemplified by a failure to predict seasonal prevalence of influenza (108).
Data-related obstacles such as differences between sites' EMR, laboratory equipment, coding definitions, and clinical practices undermine the reliability and generalizability of medical AI systems (10). Comparing algorithms across studies is a major difficulty, as they often use different methodologies on diverse populations. Discriminatory bias can lead to inaccuracies when working with minority subgroups, as AI can disproportionate affect groups disadvantaged by factors such as race, gender, and socioeconomics (10, 68, 109–113). Implementing generalizations is challenging across new populations where specificity at a fixed operating point varies across independent datasets (65, 70). For instance, one system would classify a skin lesion as malignant if an image included a ruler, which correlated with an increased likelihood of diagnosing a cancerous lesion (43). Another interpreted surgical skin markings falsely as evidence of increased melanoma probability scores (114). A third system classified images of malignant moles, but was trained on fair skinned patients, and underperformed on images of lesions in dark skin (43, 115, 116).
A lack of validation sets is also a major drawback for some of the platforms used (10, 117, 118). A recent review found that only 6% of 516 eligible published AI studies performed external validation (109). Real-world clinical performance necessitates external validation. By testing the algorithms on properly sized datasets collected from multiple institutions, it can be ensured that variations in patient demographics and disease parameters are represented in the model (113).
AI can examine multidimensional data and identify patterns that capture multiple parameters which determine disease progress and response to therapy. These include genotype, metabolism, drug pharmacokinetics, disease characteristics, comorbidities, and environmental factors (30). Individualizing phenotypic or genotypic patterns requires high-performance computing for processing multidimensional datasets (30, 49, 55, 119–122). Despite this, high phenotype overlap between different disorders, and phenotypic variability between individuals with the same diagnosis, may lead to misdiagnosis (112, 123, 124). Quantifying risks from patterns of genomic variation involves marked heterogeneity, variable penetrance of risk variants, and environmental factors (30, 92, 112, 125–129). Numerous penetrant variants are linked with more than one clinical manifestation, while several diagnoses are described by variable presentations (30, 122, 130). Inability to address gene-gene interactions involving more than few genes (131, 132), and overlap between gene lists and/or protein/co-expression networks for different diseases, reduce diagnostic accuracy (125, 128). A high degree of overlap in common-SNP based genetic etiology and patterns of differentially expressed genes are associated with a low diagnostic accuracy (133, 134). To make matters worse, unknown variants' penetrance can be a hurdle in classifying mutations according to clinical relevance. While low penetrance is common, high penetrance variants are not unheard of. Most variants are non-coding, and defining their pathogenicity requires multidimensional data analysis (3, 105, 121). Many syndromes are diagnosed by a threshold number of symptoms and clinical history. The availability of data varies between subjects. Lack of concordance among experts, and dynamicity of symptoms which change over time, complicate the use of AI to reach accurate clinical results (135).
Many studies comparing the efficiency or accuracy of AI to that of clinicians can be shown to have an unreliable design, to be hard to replicate, or to lack validation (11, 112, 136, 137). A flowchart approach combines history taking and symptoms for diagnosis and requires large amounts of data. A database approach is based on the use of ML or pattern recognition to identify groups of symptoms or certain clinical image patterns (76). But since numerous signs can only be observed by a physician, many of these platforms are inferior to humans. AI-based software has been claimed as improving interpretation of pulmonary function tests, but the study was criticized for the AI scoring lower than average scores provided by clinicians (138). Computer-assisted diagnosis in screening mammography showed suboptimal positive predictive values, false positives, sensitivity, and specificity values (139–141). The study's algorithm, developed by reviewing 640,000 digital mammograms, could only reach a specificity, sensitivity, and area under the receiver operator curve similar to those of the bottom 10% of radiologists (142). When searching for a diagnostic of serious disease, AI can easily identify trivial data relationships which may be of no clinical significance, and therefore worsen over-diagnosis and overtreatment (143).
Many AI systems fail to explain the decision-making algorithm in an understandable way (10). Concerns regarding unacceptable results, the risk of unquantified biases that may prove difficult to identify, and the potential to use inappropriate confounding variables, all make it extremely desirable that the AI be explicable (10). AI trained by high volume data may recognize patterns that are not observable to humans (144), and which may be difficult for humans to deduce by observation. However, the trade-off between performance and explicability implies that the best performing models will often be the least explicable, whereas linear regression or decision tree models, which show poorer performance, are more explicable (10, 73, 113, 119, 145). Furthermore, increased administrative burdens associated with EMR and the lack of a legal framework defining liability when adopting or rejecting algorithm recommendations present additional barriers to the adoption of medical AI (11, 146–149).
Taken together, these examples highlight some of the barriers which underlie medical AI's limited accuracy and inability to achieve significant, reliable clinical impacts. These limitations help to explain the low penetration of first-generation AI into clinical practice.
Second-Generation Systems Provide the “5th P”: Focus on Improving the Clinical Condition of a Single Patient
Healthcare applications of AI research have received extensive investments in recent last year's (76, 150). However, the stereotypical “Silicon Valley mindset” calling for engineers to “move fast and break things” is clearly inappropriate for applying AI to healthcare (143, 151). Currently most tasks performed by these platforms are limited, leaving the primary responsibility of patient management with a human doctor (1). First generation systems were designed to promote the 4P model of medicine: Predictive, Preventive, Personalized, and Participatory, providing patient autonomy (9). Second-generation AI systems are anticipated to add the “5th P:” Progress, an improvement of a clinically meaningful endpoint in a subject-tailored manner. Rather than analyzing data for assisting in diagnosis, prediction, or tailoring therapy, second-generation platforms focus on improving biological processes. Several fundamental changes needed for achievement of the “5th P” are described below.
Aiming at Clinical Outcomes
A focus on clinically meaningful endpoints and clinical outcomes that have a quantifiable benefit, and on improving patient outcomes, is expected to assist in adoption of these new platforms by patients and caregivers (10). These systems, when designed to assist in response to interventions, will need to generate outputs based on quantified clinical responses. If a clinical benefit is not achieved, the likelihood of these algorithms being implemented is low (30).
Improving Organ Function and Response to Therapies
For second- generation AI to improve quantifiable symptomatic or laboratory endpoints, it will have to improve organ function, mental healthcare, and response to drugs. The algorithms ought to improve biological systems, but not by attempting to change them, nor by pushing them in the opposite direction from the one they are tilted toward by a disease. Their goal should be “getting the train back on track,” independently of which direction the system has been skewed (152, 153).
Controlling for the Dynamic Nature of Host and Disease
Biological systems are dynamic by nature. Subjects, disease processes, and responses to therapies continually change. Chronic diseases move along a dynamic trajectory, creating a challenge of unpredictable progression, which is often disregarded by first-generation AI (30). The internal and external parameters that determine the progression of chronic disease and host response vary constantly. These require constant adaptation of therapeutic regimens (154). Furthermore, many therapies do not show efficacy or loss of response until considerable time has passed. Subjects may remain essentially untreated for several months in cases where reaching an effect requires several attempts. For many drugs, secondary loss of response occurs following an initial benefit (155, 156). Second-generation AI systems which are designed to improve response to therapies, must therefore facilitate analyzing inter-subject and intra-subject variabilities in response to therapies over time (157–160). The dynamicity of biological systems requires algorithms to implement continual, periodic system-wide updates and identification of performance deficits over time in an individual subject (10).
Overcoming Big Data Challenges by Implementing an n = 1 Concept
Most first-generation AI systems extract data from large databases, and artificially impose a rigid “one for all” algorithm on all subjects. Attempts to constantly amend treatment regimens based on big data analysis might be irrelevant for an individual patient. Imposing a “close to optimal” fit on all subjects does not resolve difficulties associated with dynamicity and the inherent variability of biological systems. Second-generation AI systems must therefore focus on a single patient as the epicenter of an algorithm and to adapt their output in a timely manner. They are required to continually respond to feedback in an individualized manner while generating an insightful database that can be used to further improve the algorithm for other patients.
These platforms may not require a large volume of high-quality data. Second-generation systems are expected to be able to function based on input from a single patient (158–160). Conventional ML systems developed to analyze massive datasets are not analogous to the way brains perform. The brain learns by analyzing data within a certain context. It does not need to watch a thousand airplanes to differentiate an airplane from a bird. This difference in approach is problematic when trying to achieve good outcomes for individual patients. Generalizing from large datasets to a single patient is unsuccessful in many cases, due to a large heterogeneity among subjects, and to ongoing individualized changes in disease triggers and host responses. The n = 1 concept can be implemented into second-generation platforms by focusing on dynamicity of disease and response to intervention in a single patient. The multiple host, disease, and environment-related variables learned from big datasets can be implemented into a single subject-based algorithm that analyzes input from, and generates output to, that subject (158–160).
In addition to the above four requirements for second-generation platforms, several additional parameters must be considered. These platforms should improve patient care, cost-effectiveness, and workflow for everyday clinical practice in terms of decision making and the physicians' workload (1, 161). They must use metrics which are clinically important and intuitive to clinicians (10). Reducing bias, using explainable approaches, and using common statistical methods are also desirable, as they improve transparency and trust, and encourage adoption by clinicians (10, 162–164).
Establishment of a Second-Generation AI System: Implementing Individualized Signatures of Variability for Promoting a Subject-Tailored Improved Response to Therapy
Variability Characterizes Biological Systems
Algorithms that implement natural patterns into biological systems are being evaluated (160, 165–169). Rather than enforcing an artificial change on a biological process, such algorithms are designed to make use of rules that are inherent to the system.
Biological systems are characterized by a high degree of variability and randomness. The rules by which these systems behave are not fixed and continually change over time. Their dynamicity, and the multiple variables that continually affect these biological laws, stand in contrast to the laws found in physics. In physics, laws are regulated by more constant, more predictable rules (170–173). In contrast to randomness in physics, where only a fixed range of possibilities exists, in biology this range is itself variable, and sometimes even randomly constituted (174–177). Some may argue that biological systems may be less random and appear random when represented by linear or additive models. While these theories are more easily explainable when represented as complex systems, they do not rule out the option of some degree of intrinsic variability (178).
The term “randomness” in biological systems is thus used with several meanings. In some studies, it describes disorder in a thermodynamic sense; others use it when referring to a high degree of complexity of a process (153, 160, 165, 179, 180). Randomness is used to describe noisy or stochastic behavior of a system, or when referring to unpredictability of its structure and behavior (166, 174, 180–183). Randomness has evolutionary associations, wherein variation and probability are linked to the adaptability of a system (184, 185). Darwinian evolutionary processes are partially ascribed to an ability to adapt to unpredictable environmental changes. Neo-Darwinism suggests “blind chance” as an origin of variation. Spontaneous and induced mechanisms of phenotypic adaptation indicate a role for chance in compensatory processes (185–190). Chaos theory describes the process of disorder arising in deterministic systems that are sensitive to initial conditions, increasing the unpredictability of the systems (191).
The noisy or stochastic behavior of biological systems characterizes their dynamic and adaptable behavior in response to internal and external triggers (169, 192). Intrinsic stochasticity has been described for numerous intracellular pathways (181, 193). Organisms are dissimilar to each other, and undergo unpredictable changes affected by multiple irregular variables (158–160, 194, 195). Intra-subject and inter-subject biological variability is observed at every level from cellular organelles to whole organs (160, 196–201).
The Crick and Watson interpretation of molecular biology, influenced by quantum mechanics, holds that functional order is a result of order at the molecular level, where information passes from DNA to proteins (202, 203). However, DNA-dependent processes do not assure functionality at molecular levels. Proper function involves multiple correlations between processes at a higher level. As Schrödinger once argued for the physics of subatomic particles, it has been proposed that molecules in an organism cannot avoid stochasticity (203–206). Variability has been described in nucleotide substitutions in DNA sequence (207), and when referring to evolving ancestral DNA sequences, as they begin to take different forms in populations of the same species (208). Random genetic drift is an example of fluctuating gene variants in a population (209). Variability has been shown for cell proliferation and death decisions, evolving from heterogeneity in founder cells (210, 211). Phenotype variability has been described for biomarkers on lymphocyte subpopulations. Antibody response to pathogens comprises expansion of antigen-specific cells and involves stochastic competition among competing cell fates or deterministic cell fate decisions (212). A high rate of variability in generating regulatory T cells has been observed in immune disease responses (213). Ex vivo cytokine release tests have manifested high inter-individual and intra-group variability (214).
Variability Contributes to Proper Function of Biological Systems
Variability should be viewed as a property of causal processes that contribute to proper functions of systems (158, 159, 196). Keeping “steadiness” is not a mandatory objective of physiologic control under all conditions. It can prohibit normal adaptation to ongoing changes (158–160, 194, 195). Non-linear systems can function far from equilibrium, and their dynamicity is part of the plasticity required for optimizing their function (203, 215–217). Biological variability is desirable for the evolutionary dynamics that contribute to stability through adaptation (153, 160, 180, 218, 219). It should be regarded as a method used for generating “new order,” assisting in overcoming errors in assembly and functions (166, 220). For stochastic cellular processes such as single-cell responses, randomness-based modeling improves deterministic models (221–223). Additional examples include gatherings of cells within heterogeneous spaces and random control of associations of biomolecules, which lead to an array of synchronized functions, including transcription, translation, ribosome biogenesis, chromosome replication, and metabolism (160, 196, 224–226). The dynamic instability of microtubules likewise demonstrates that variability is required for their normal structure and function (196, 227).
An Advanced Model for Disease Involving Loss of Variability
Since variability characterizes the normal function of systems, health can be perceived as a continual adaptation which requires constant adjustments. Appearance of “order” in a system that is inherently disordered is associated with the occurrence of a disease, representing rigid dysfunction of a system (228–231). Loss of complexity in diseased states is related to a reduced ability to adapt to changes, and may underlie chronic illnesses and aging (232–234).
Irregularity in Response to Disease-Inducing Triggers and to Therapies
Variability occurs between subjects and within the same subject in the response triggers that induce a disease. An example of personalized response to disease-inducing triggers has been shown in a concanavalin A (ConA)-induced immune-hepatitis model. Marked variability in individualized responses to a disease-trigger was documented under similar conditions (180).
Similarly, marked inter-subject variability in the response to immunomodulatory therapies has been shown by marked differences in degree of alleviation of the immune-mediated liver injury. A personalized response has been documented for the effect of therapies on cytokine levels and on expression of cell epitopes on lymphocyte subsets (180). A physiological lattice model for liver metabolism of drugs has shown that variability of sinusoidal structure underlies variances in drug response in individual hepatic veins at different times, suggesting that individual ports react differently in response to drugs (235). In humans, unpredictability has been described when discussing the response to chronic medications with high rate of loss of response over time, and in some cases paradoxical exacerbation of diseases. Inter and intra-patient variability in drug serum levels between days has been shown (236, 237).
Variability in response to medications among subjects is commonly attributed to pharmacogenomics and pharmacodynamics-based drug metabolism (237–240). However, heterogeneity cannot be solely attributed to these mechanisms (241). The unpredictability of responses to drugs is partly due to the changing dynamic rules by which biological systems function. Complex intracellular drug-target interactions which are not defined solely by simple diffusion and intrinsic chemical reactions, and that are beyond “simple” pharmacodynamics, have been demonstrated. Non-specific interactions can slow the incorporation kinetics of DNA-binding drugs, and can be responsible for irregular drug diffusion in cells (241).
Loss of Response to Chronic Therapies
Individual resistance to drugs is highly variable and results from multiple genetic and environmental factors. This implies that deterministic equations are unsuitable for examining dose-response relations (242). For instance, a secondary loss of response to anti-tumor necrosis alpha (TNF) occurs in 25–61% of patients with rheumatoid arthritis following an initial effect (243–245). More generally, cancer drug resistance associated with patients' genetic background or acquired by tumors is common in patients with malignancies (246, 247). Resistance to anti-depressant medications occurs in a third of patients (248). Similarly, about a third of epileptics develop resistance to anti-epileptic agents (249).
Implementing Personalized Variability Patterns for Improving Function of Biological Systems and Response to Drugs
Regular fixed therapeutic regimens may not be compatible with physiological variabilities in biology, and can underlie loss of response to chronic drugs (158–160, 250, 251). Fixed regimens are incompatible with the variable trajectories which underlie the pathogenesis of diseases and compensatory responses to therapies (215). Introducing variability into therapeutic regimens can improve response to drugs. Dose escalations, reductions, and intermittent dosing with drug holidays can exert clinical benefits while minimizing adverse effects (252–256). For instance, in a prospective trial of patients with inflammatory bowel disease treated with anti-TNFs, loss of clinical response was observed in 36% of patients on fixed dosing compared with only 13% in subjects treated using a (de-) escalation dashboard (257).
The variability which characterizes properly functioning biological systems, their response to disease-inducing triggers, and their response to therapies, along with real-world data showing beneficial effects of drug holidays and dose escalation/reduction, supports implementing variability-based regimens. This holds promise for improvement of organ function and response to therapies (158, 159, 165, 180, 258–265). Introducing variability into systems increases their degree of complexity and improves their function (160, 266). The resulting variability pattern tracks a similar trajectory to that used by the body while responding to disease-triggers and to therapies. This is expected to improve response to interventions under unpredictable conditions (158–160, 165, 166).
It has been proposed that the type and magnitude of variability in a biological system should ideally be personalized (158, 159). It has been suggested that continuously quantifying individualized variability patterns and implementing them into algorithms enables personalized and dynamic tailoring of therapeutic regimens. Patterns of variability can be quantified from genetic profiling, immune testing, chronotherapy measures, heart rate variability, and additional host and disease-related variability signatures (162, 163, 170, 171). This type of improved precision medicine is compatible with the principles according to which diseases and hosts behave. Rather than imposing a rigid artificial regimen, algorithms continuously adapt to individual changes in disease and the patient's responses to interventions (158–160, 166). Therefore, a patient-tailored approach based on individualized variability patterns implemented into AI systems is expected to improve the efficiency and sustainability of the beneficial effects of chronic therapies.
A Continuously Adapting Individualized-Variability Based Closed-Feedback Loops for Ensuring Plasticity and Improved Function of a Biological System
The establishment of the second-generation AI systems described herein is performed in steps (267). In the first step, the effect of introducing variability into therapeutic regimens of patients who have partially or completely lost their response to chronic medications should be evaluated. These algorithms have embedded pseudo-random number generators which introduce variability in times of administration and dosages within an approved range. These pseudo-random number generators function within regulatory-approved therapeutic dosing and pharmacodynamics windows and can be implemented instantly. From a regulatory authorities' perspective, the use of these systems may be viewed as reminders for improve of patient's adherence (268).
Ongoing clinical trials (NCT03843697; NCT03747705) are evaluating the effects of these regimens in patients with inflammatory bowel disease who have lost their response to anti-TNFs, and in patients with epilepsy who have lost response to anti-epileptics. Data from these studies is expected to find improved response to medications when applying variability-based therapeutic regimens. While this type of variability may not always fit into an underlying personalized variability pattern, it is likely to provide improvement over the fixed regimens which underlie drug resistance.
In the second step, a closed-loop algorithm should be implemented, where inputs are based on clinical outcomes chosen to generate therapeutic regimens. This step requires regulatory approval, as it involves changes of dosing regimens outside approved ranges, based on subject's clinical response, while keeping dosages within pre-determined safety boundaries. The algorithm may prevent certain unwanted side effects, as it enables skipping doses, but should not provide dosages which might potentially induce drug complications (267).
In the third step, host and disease-related patterns of variability would be quantified in a personalized manner, and then implemented in a true-random number generator. This step is expected to further improve the response rate and sustainability of beneficial effects of therapies in patients with chronic diseases. It involves a process of continuous adaptation of algorithm output to inputs from quantifiable variability parameters. These will be parameters associated with disease pathogenesis, host response, and the mechanism of action of the drug. For example, heart rate variability parameters can be used an input for generating therapeutic regimens for patients with heart failure; and measuring variabilities in cytokine response will help treat patients with immune disorders (267).
For all steps, genotypic and phenotypic parameters, which may impact algorithm output, are “ignored” by the platform. The algorithm continuously adapts itself to a clinically meaningful output as its sole endpoint. The sums of effects of all potential factors on a clinical endpoint are thus being considered without splitting them into individual variables. This contrasts with attempts made by most first-generation AIs to dissect and quantify only some of these parameters. Thus, the second-generation medical AI will not consider many unquantifiable measures, or use fixed rules which are based on artificial parameters, or on fitting of data from large datasets. Instead, the proposed platform adapts itself to a sum of all parameters via their effects on a sole measured endpoint in a single subject. The continually individualized dynamic and unpredictable changes in disease, host response, and environmental triggers will be accounted for via their effects on clinical outcomes, generating a dynamic output by the algorithm.
In the fourth step, an insightful database would be generated by the algorithm. Outputs from this database and from conventional datasets would then be used to introduce additional variables into the algorithm. The effects of these interventions would be continuously measured by evaluating their effect on a clinical endpoint in an individual subject (267).
Figure 1 shows a schematic presentation of a closed-loop algorithm where a single subject with a chronic disease is at the focus. A feedback loop is responsive to the effect of therapy on a clinically meaningful endpoint. The algorithm aims at this endpoint and adapts itself in a dynamic manner. The AI platform then quantifies personalized variability patterns relevant to the disease, host response, and environment-related variables. It combines these variables into a dynamic therapeutic regimen. In parallel, the algorithm generates its own insightful database which evolves from analyzing its outputs on organ function (160, 166).
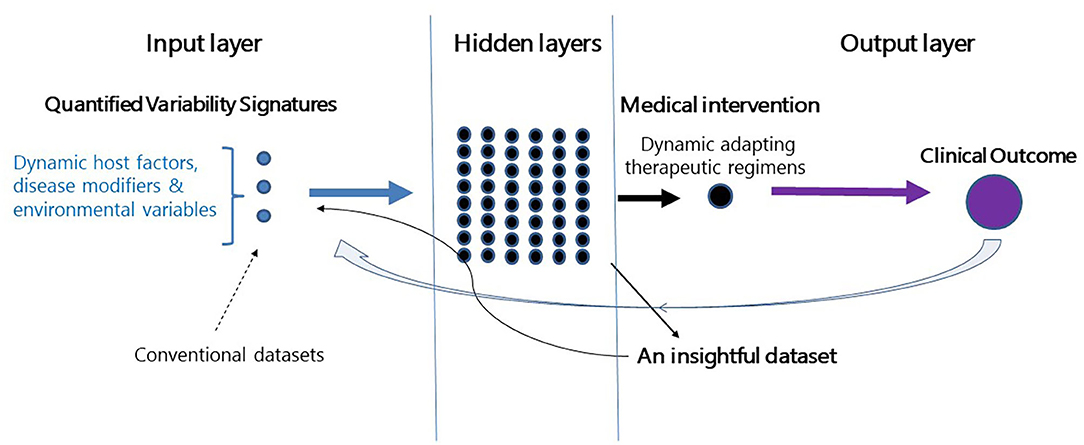
Figure 1. Establishment of a second-generation AI platform. Depiction of a closed-loop platform where a patient with a chronic disease is placed at the center. The closed feedback loop is responsive to the effect of therapy on clinical outcomes. The algorithm adapts itself dynamically to the effects of therapy on the endpoint. The algorithm quantifies personalized variability patterns and implements them into therapeutic regimens. In parallel, the algorithm generates an insightful database which evolves from analyzing outputs on the endpoint. The dataset collects the relevant variability-based quantifiable parameters which are associated with improved clinical outcomes. The dataset is continuously being updated based on the clinical response of each of the treated subjects.
All of this notwithstanding, doctors will most likely not be entirely replaced by AI systems. The platforms discussed here are expected to support physicians for improving patients' management (149, 269). While first-generation systems were designed to assist medical decisions, their penetration into clinical workflow has been limited. Second-generation systems are and will be designed to overcome some of the obstacles experienced over recent years. These new platforms should support better interactions between clinicians and algorithms, showing that a combination of human and AI outperforms either alone, and aiming at improvements to clinical outcomes. The dataset collects the variability-based quantifiable parameters which are associated with improved clinical outcomes. It continuously updated in accordance with the clinical response of each of the treated subjects (10, 270).
The platform described herein introduces a novel method wherein the focus of an algorithm is on improving clinical outcomes of individual subjects. It implements an n = 1 concept into a personalized therapeutic regimen by introducing individualized variability signatures into an algorithm. The system is based on improving the beneficial effects of therapeutic interventions. This makes it more likely to gain adherence from both patients and healthcare providers. The platform is designed to overcome compensatory mechanisms associated with drug resistance and disease progression, to ensure sustainable beneficial effects from medications. Results of ongoing clinical trials and future prospective studies are expected to enable further development of these platforms.
Author Contributions
The author confirms being the sole contributor of this work and has approved it for publication.
Conflict of Interest
YI is the founder of Oberon Sciences and is a consultant for Teva, ENZO, Protalix, Betalin Therapeutics, Immuron, SciM, Natural Shield, and Tiziana.
Abbreviations
AI, artificial intelligence; EMR, electronic medical records; ML, machine learning; RCT, randomized controlled trials; NLP, natural language processing; TNF, tumor necrosis alpha; ConA, concanavalin A.
References
1. Buch VH, Ahmed I, and Maruthappu M. Artificial intelligence in medicine: current trends and future possibilities. Br J Gen Pract. (2018) 68:143–4. doi: 10.3399/bjgp18X695213
2. Chen JH, and Asch SM. Machine learning and prediction in medicine - beyond the peak of inflated expectations. N Engl J Med. (2017) 376:2507–9. doi: 10.1056/NEJMp1702071
3. Singh RP, Hom GL, Abramoff MD, Campbell JP, Chiang MF, and on behalf of the AAO Task Force on Artificial Intelligence. Current challenges and barriers to real-world artificial intelligence adoption for the healthcare system, provider, the patient. Transl Vis Sci Technol. (2020) 9:45. doi: 10.1167/tvst.9.2.45
4. Inkster B, Sarda S, and Subramanian V. An empathy-driven, conversational artificial intelligence agent (Wysa) for digital mental well-being: real-world data evaluation mixed-methods study. JMIR Mhealth Uhealth. (2018) 6:e12106. doi: 10.2196/12106
5. Jill Hopkins J, Keane PA, and Balaskas K. Delivering personalized medicine in retinal care: from artificial intelligence algorithms to clinical application. Curr Opin Ophthalmol. (2020) 31:329–36. doi: 10.1097/ICU.0000000000000677
6. Chan KS, and Zary N. Applications and challenges of implementing artificial intelligence in medical education: integrative review. JMIR Med Educ. (2019) 5:e13930. doi: 10.2196/13930
7. Paton C, and Kobayashi S. An open science approach to artificial intelligence in healthcare. Yearb Med Inform. (2019) 28:47–51. doi: 10.1055/s-0039-1677898
8. Bodenheimer T, and Sinsky C. From triple to quadruple aim: care of the patient requires care of the provider. Ann Fam Med. (2014) 12:573–6. doi: 10.1370/afm.1713
9. Orth M, Averina M, Chatzipanagiotou S, Faure G, Haushofer A, Kusec V, et al. Opinion: redefining the role of the physician in laboratory medicine in the context of emerging technologies, personalised medicine and patient autonomy ('4P medicine'). J Clin Pathol. (2019) 72:191–7. doi: 10.1136/jclinpath-2017-204734
10. Kelly CJ, Karthikesalingam A, Suleyman M, Corrado G, and King D. Key challenges for delivering clinical impact with artificial intelligence. BMC Med. (2019) 17:195. doi: 10.1186/s12916-019-1426-2
11. Briganti G, and Le Moine O. Artificial intelligence in medicine: today and tomorrow. Front Med. (2020) 7:27. doi: 10.3389/fmed.2020.00027
12. Overley SC, Cho SK, Mehta AI, and Arnold PM. Navigation and robotics in spinal surgery: where are we now? Neurosurgery. (2017) 80:S86–99. doi: 10.1093/neuros/nyw077
13. Tepper OM, Rudy HL, Lefkowitz A, Weimer KA, Marks SM, Stern CS, et al. Mixed reality with hololens: where virtual reality meets augmented reality in the operating room. Plast Reconstr Surg. (2017) 140:1066–70. doi: 10.1097/PRS.0000000000003802
14. Malloy KM, and Milling LS. The effectiveness of virtual reality distraction for pain reduction: a systematic review. Clin Psychol Rev. (2010) 30:1011–8. doi: 10.1016/j.cpr.2010.07.001
15. Keane PA, and Topol EJ. With an eye to AI and autonomous diagnosis. NPJ Digit Med. (2018) 1:40. doi: 10.1038/s41746-018-0048-y
16. Schrider DR, Ayroles J, Matute DR, and Kern AD. Supervised machine learning reveals introgressed loci in the genomes of Drosophila simulans and D.sechellia. PLoS Genet. (2018) 14:e1007341. doi: 10.1371/journal.pgen.1007341
17. Roohi A, Faust K, Djuric U, and Diamandis P. Unsupervised machine learning in pathology: the next frontier. Surg Pathol Clin. (2020) 13:349–58. doi: 10.1016/j.path.2020.01.002
18. La Cava W, Williams H, Fu W, Vitale S, Srivatsan D, and Moore JH. Evaluating recommender systems for AI-driven biomedical informatics. Bioinformatics. (2020) 1:17. doi: 10.1093/bioinformatics/btaa698
19. Sheikhtaheri A, Sadoughi F, and Hashemi Dehaghi Z. Developing and using expert systems and neural networks in medicine: a review on benefits and challenges. J Med Syst. (2014) 38:110. doi: 10.1007/s10916-014-0110-5
20. Wang J, Deng H, Liu B, Hu A, Liang J, Fan L, et al. Systematic evaluation of research progress on natural language processing in medicine over the past 20 years: bibliometric study on pubmed. J Med Internet Res. (2020) 22:e16816. doi: 10.2196/16816
21. Jin L, Tan F, and Jiang S. Generative adversarial network technologies and applications in computer vision. Comput Intell Neurosci. (2020) 2020:1459107. doi: 10.1155/2020/1459107
22. George K, Faziludeen S, Sankaran P, and Joseph KP. Breast cancer detection from biopsy images using nucleus guided transfer learning and belief based fusion. Comput Biol Med. (2020) 124:103954. doi: 10.1016/j.compbiomed.2020.103954
23. Chen EC, McLeod AJ, Baxter JS, and Peters TM. Registration of 3D shapes under anisotropic scaling: anisotropic-scaled iterative closest point algorithm. Int J Comput Assist Radiol Surg. (2015) 10:867–78. doi: 10.1007/s11548-015-1199-9
24. Hamet P, and Tremblay J. Artificial intelligence in medicine. Metabolism. (2017) 69S:S36–40. doi: 10.1016/j.metabol.2017.01.011
25. Rizzi R, Cairo M, Makinen V, Tomescu AI, and Valenzuela D. Hardness of covering alignment: phase transition in post-sequence genomics. IEEE/ACM Trans Comput Biol Bioinform. (2019) 16:23–30. doi: 10.1109/TCBB.2018.2831691
26. Kapun E, and Tsarev F. De bruijn superwalk with multiplicities problem is NP-hard. BMC Bioinformatics. (2013) 14:S7. doi: 10.1186/1471-2105-14-S5-S7
27. Sankar PL, and Parker LS. The precision medicine initiative's all of Us research program: an agenda for research on its ethical, legal, social issues. Genet Med. (2017) 19:743–50. doi: 10.1038/gim.2016.183
28. Gagnon MP, Ghandour el K, Talla PK, Simonyan D, Godin G, Labrecque M, et al. Electronic health record acceptance by physicians: testing an integrated theoretical model. J Biomed Inform. (2014) 48:17–27. doi: 10.1016/j.jbi.2013.10.010
29. Sheikh A, Cornford T, Barber N, Avery A, Takian A, Lichtner V, et al. Implementation and adoption of nationwide electronic health records in secondary care in England: final qualitative results from prospective national evaluation in “early adopter” hospitals. BMJ. (2011) 343:d6054. doi: 10.1136/bmj.d6054
30. Uddin M, Wang Y, and Woodbury-Smith M. Artificial intelligence for precision medicine in neurodevelopmental disorders. NPJ Digit Med. (2019) 2:112. doi: 10.1038/s41746-019-0191-0
31. Dankwa-Mullan I, Rivo M, Sepulveda M, Park Y, Snowdon J, and Rhee K. Transforming diabetes care through artificial intelligence: the future is here. Popul Health Manag. (2019) 22:229–42. doi: 10.1089/pop.2018.0129
32. McGlynn EA, McDonald KM, and Cassel CK. Measurement is essential for improving diagnosis and reducing diagnostic error: a report from the institute of medicine. JAMA. (2015) 314:2501–2. doi: 10.1001/jama.2015.13453
33. Liang H, Tsui BY, Ni H, Valentim CCS, Baxter SL, Liu G, et al. Evaluation and accurate diagnoses of pediatric diseases using artificial intelligence. Nat Med. (2019) 25:433–38.
34. Escobar GJ, Turk BJ, Ragins A, Ha J, Hoberman B, LeVine SM, et al. Piloting electronic medical record-based early detection of inpatient deterioration in community hospitals. J Hosp Med. (2016) 11(Suppl.):S18–24. doi: 10.1002/jhm.2652
35. Rajkomar A, Oren E, Chen K, Dai AM, Hajaj N, Hardt M, et al. Scalable and accurate deep learning with electronic health records. NPJ Digit Med. (2018) 1:18. doi: 10.1038/s41746-018-0029-1
36. Nelson A, Herron D, Rees G, and Nachev P. Predicting scheduled hospital attendance with artificial intelligence. NPJ Digit Med. (2019) 2:26. doi: 10.1038/s41746-019-0103-3
37. Rajkomar A, Kannan A, Chen K, Vardoulakis L, Chou K, Cui C, et al. Automatically charting symptoms from patient-physician conversations using machine learning. JAMA Intern Med. (2019) 179:836–8. doi: 10.1001/jamainternmed.2018.8558
38. Xu J, Yang P, Xue S, Sharma B, Sanchez-Martin M, Wang F, et al. Translating cancer genomics into precision medicine with artificial intelligence: applications, challenges and future perspectives. Hum Genet. (2019) 138:109–24. doi: 10.1007/s00439-019-01970-5
39. Ehteshami Bejnordi B, Veta M, van Diest PJ, van Ginneken B, Karssemeijer N, Litjens G, et al. Diagnostic assessment of deep learning algorithms for detection of lymph node metastases in women with breast cancer. JAMA. (2017) 318:2199–210. doi: 10.1001/jama.2017.14580
40. Chang P, Grinband J, Weinberg BD, Bardis M, Khy M, Cadena G, et al. Deep-learning convolutional neural networks accurately classify genetic mutations in gliomas. AJNR Am J Neuroradiol. (2018) 39:1201–7. doi: 10.3174/ajnr.A5667
41. Shu C, Wang Q, Yan X, and Wang J. Whole-genome expression microarray combined with machine learning to identify prognostic biomarkers for high-grade glioma. J Mol Neurosci. (2018) 64:491–500. doi: 10.1007/s12031-018-1049-7
42. Wood DE, White JR, Georgiadis A, van Emburgh B, Parpart-Li S, Mitchell J, et al. A machine learning approach for somatic mutation discovery. Sci Transl Med. (2018) 10:eaar7939. doi: 10.1126/scitranslmed.aar7939
43. Esteva A, Kuprel B, Novoa RA, Ko J, Swetter SM, Blau HM, et al. Dermatologist-level classification of skin cancer with deep neural networks. Nature. (2017) 542:115–8. doi: 10.1038/nature21056
44. Han SS, Kim MS, Lim W, Park GH, Park I, and Chang SE. Classification of the clinical images for benign and malignant cutaneous tumors using a deep learning algorithm. J Invest Dermatol. (2018) 138:1529–38. doi: 10.1016/j.jid.2018.01.028
45. Brinker TJ, Hekler A, Enk AH, Klode J, Hauschild A, Berking C, et al. Deep learning outperformed 136 of 157 dermatologists in a head-to-head dermoscopic melanoma image classification task. Eur J Cancer. (2019) 113:47–54. doi: 10.1016/j.ejca.2019.04.001
46. Campanella G, Hanna MG, Geneslaw L, Miraflor A, Werneck Krauss Silva V, Busam KJ, et al. Clinical-grade computational pathology using weakly supervised deep learning on whole slide images. Nat Med. (2019) 25:1301–9. doi: 10.1038/s41591-019-0508-1
47. Gulshan V, Peng L, Coram M, Stumpe MC, Wu D, Narayanaswamy A, et al. Development and validation of a deep learning algorithm for detection of diabetic retinopathy in retinal fundus photographs. JAMA. (2016) 316:2402–10. doi: 10.1001/jama.2016.17216
48. de Fauw J, Ledsam JR, Romera-Paredes B, Nikolov S, Tomasev N, Blackwell S, et al. Clinically applicable deep learning for diagnosis and referral in retinal disease. Nat Med. (2018) 24:1342–50. doi: 10.1038/s41591-018-0107-6
49. Kermany DS, Goldbaum M, Cai W, Valentim CCS, Liang H, Baxter SL, et al. Identifying medical diagnoses and treatable diseases by image-based deep learning. Cell. (2018) 172:1122–31.e9. doi: 10.1016/j.cell.2018.02.010
50. Ting DSW, Pasquale LR, Peng L, Campbell JP, Lee AY, Raman R, et al. Artificial intelligence and deep learning in ophthalmology. Br J Ophthalmol. (2019) 103:167–75. doi: 10.1136/bjophthalmol-2018-313173
51. Raumviboonsuk P, Krause J, Chotcomwongse P, Sayres R, Raman R, Widner K, et al. Deep learning versus human graders for classifying diabetic retinopathy severity in a nationwide screening program. NPJ Digit Med. (2019) 2:25. doi: 10.1038/s41746-019-0146-5
52. Hannun AY, Rajpurkar P, Haghpanahi M, Tison GH, Bourn C, Turakhia MP, et al. Cardiologist-level arrhythmia detection and classification in ambulatory electrocardiograms using a deep neural network. Nat Med. (2019) 25:65–9. doi: 10.1038/s41591-018-0268-3
53. Attia ZI, Noseworthy PA, Lopez-Jimenez F, Asirvatham SJ, Deshmukh AJ, Gersh BJ, et al. An artificial intelligence-enabled ECG algorithm for the identification of patients with atrial fibrillation during sinus rhythm: a retrospective analysis of outcome prediction. Lancet. (2019) 394:861–7. doi: 10.1016/S0140-6736(19)31721-0
54. Wang P, Xiao X, Glissen Brown JR, Berzin TM, Tu M, Xiong F, et al. Development and validation of a deep-learning algorithm for the detection of polyps during colonoscopy. Nat Biomed Eng. (2018) 2:741–8. doi: 10.1038/s41551-018-0301-3
55. Gurovich Y, Hanani Y, Bar O, Nadav G, Fleischer N, Gelbman D, et al. Identifying facial phenotypes of genetic disorders using deep learning. Nat Med. (2019) 25:60–4. doi: 10.1038/s41591-018-0279-0
56. Tomasev N, Glorot X, Rae JW, Zielinski M, Askham H, Saraiva A, et al. A clinically applicable approach to continuous prediction of future acute kidney injury. Nature. (2019) 572:116–9. doi: 10.1038/s41586-019-1390-1
57. Bruno E, Simblett S, Lang A, Biondi A, Odoi C, Schulze-Bonhage A, et al. Wearable technology in epilepsy: the views of patients, caregivers, healthcare professionals. Epilepsy Behav. (2018) 85:141–9. doi: 10.1016/j.yebeh.2018.05.044
58. Yang YJ, and Bang CS. Application of artificial intelligence in gastroenterology. World J Gastroenterol. (2019) 25:1666–83. doi: 10.3748/wjg.v25.i14.1666
59. Das A, Ben-Menachem T, Cooper GS, Chak A, Sivak MV Jr, et al. Prediction of outcome in acute lower-gastrointestinal haemorrhage based on an artificial neural network: internal and external validation of a predictive model. Lancet. (2003) 362:1261–6. doi: 10.1016/S0140-6736(03)14568-0
60. Komorowski M, Raghu A, Ahmed I, Celi L, Szolovits P, and Ghassemi M. Deep reinforcement learning for sepsis treatment. arXiv. Available online at: https://arxiv.org/abs/1711.09602
61. Athreya AP, Neavin D, Carrillo-Roa T, Skime M, Biernacka J, Frye MA, et al. Pharmacogenomics-driven prediction of antidepressant treatment outcomes: a machine-learning approach with multi-trial replication. Clin Pharmacol Ther. (2019) 106:855–65. doi: 10.1002/cpt.1482
62. Preuer K, Lewis RPI, Hochreiter S, Bender A, Bulusu KC, and Klambauer G. DeepSynergy: predicting anti-cancer drug synergy with deep learning. Bioinformatics. (2018) 34:1538–46. doi: 10.1093/bioinformatics/btx806
63. Derozier V, Arnavielhe S, Renard E, Dray G, and Martin S. How knowledge emerges from artificial intelligence algorithm and data visualization for diabetes management. J Diabetes Sci Technol. (2019) 13:698–707. doi: 10.1177/1932296819847739
64. Burnside M, Crocket H, Mayo M, Pickering J, Tappe A, de Bock M, et al. Do it yourself automated insulin delivery: a leading example of the democratization of medicine. J Diabetes Sci Technol. (2019) 14:878–82. doi: 10.1177/1932296819890623
65. Hwang EJ, Park S, Jin KN, Kim JI, Choi SY, Lee JH, et al. Development and validation of a deep learning-based automated detection algorithm for major thoracic diseases on chest radiographs. JAMA Netw Open. (2019) 2:e191095. doi: 10.1001/jamanetworkopen.2019.1095
66. Singh R, Kalra MK, Nitiwarangkul C, Patti JA, Homayounieh F, Padole A, et al. Deep learning in chest radiography: detection of findings and presence of change. PLoS ONE. (2018) 13:e0204155. doi: 10.1371/journal.pone.0204155
67. Nam JG, Park S, Hwang EJ, Lee JH, Jin KN, Lim KY, et al. Development and validation of deep learning-based automatic detection algorithm for malignant pulmonary nodules on chest radiographs. Radiology. (2019) 290:218–28. doi: 10.1148/radiol.2018180237
68. Wu N, Phang J, Park J, Shen Y, Huang Z, Zorin M, et al. Deep neural networks improve radiologists' performance in breast cancer screening. IEEE Trans Med Imaging. (2019) 39:1184–94. doi: 10.1109/TMI.2019.2945514
69. Yasaka K, Akai H, Abe O, and Kiryu S. Deep learning with convolutional neural network for differentiation of liver masses at dynamic contrast-enhanced CT: a preliminary study. Radiology. (2018) 286:887–96. doi: 10.1148/radiol.2017170706
70. Chilamkurthy S, Ghosh R, Tanamala S, Biviji M, Campeau NG, Venugopal VK, et al. Deep learning algorithms for detection of critical findings in head CT scans: a retrospective study. Lancet. (2018) 392:2388–96. doi: 10.1016/S0140-6736(18)31645-3
71. Parisot S, Darlix A, Baumann C, Zouaoui S, Yordanova Y, Blonski M, et al. A probabilistic atlas of diffuse WHO grade II glioma locations in the brain. PLoS ONE. (2016) 11:e0144200. doi: 10.1371/journal.pone.0144200
72. Ding Y, Sohn JH, Kawczynski MG, Trivedi H, Harnish R, Jenkins NW, et al. A deep learning model to predict a diagnosis of Alzheimer disease by using (18)F-FDG PET of the brain. Radiology. (2019) 290:456–64. doi: 10.1148/radiol.2018180958
73. Hoog AH, Meme HK, van Deutekom H, Mithika AM, Olunga C, Onyino F, et al. High sensitivity of chest radiograph reading by clinical officers in a tuberculosis prevalence survey. Int J Tuberc Lung Dis. (2011) 15:1308–14. doi: 10.5588/ijtld.11.0004
74. McCall HC, Richardson CG, Helgadottir FD, and Chen FS. Evaluating a web-based social anxiety intervention among university students: randomized controlled trial. J Med Internet Res. (2018) 20:e91. doi: 10.2196/jmir.8630
75. Labovitz DL, Shafner L, Reyes Gil M, Virmani D, and Hanina A. Using artificial intelligence to reduce the risk of nonadherence in patients on anticoagulation therapy. Stroke. (2017) 48:1416–9. doi: 10.1161/STROKEAHA.116.016281
76. Amisha Malik P, Pathania M, and Rathaur VK. Overview of artificial intelligence in medicine. J Family Med Prim Care. (2019) 8:2328–31. doi: 10.4103/jfmpc.jfmpc_440_19
77. Strodthoff N, and Strodthoff C. Detecting and interpreting myocardial infarction using fully convolutional neural networks. Physiol Meas. (2019) 40:015001. doi: 10.1088/1361-6579/aaf34d
78. Lakhani P, and Sundaram B. Deep learning at chest radiography: automated classification of pulmonary tuberculosis by using convolutional neural networks. Radiology. (2017) 284:574–82. doi: 10.1148/radiol.2017162326
79. Beck AH, Sangoi AR, Leung S, Marinelli RJ, Nielsen TO, van de Vijver MJ, et al. Systematic analysis of breast cancer morphology uncovers stromal features associated with survival. Sci Transl Med. (2011) 3:108ra113. doi: 10.1126/scitranslmed.3002564
80. Poplin R, Varadarajan AV, Blumer K, Liu Y, McConnell MV, Corrado GS, et al. Prediction of cardiovascular risk factors from retinal fundus photographs via deep learning. Nat Biomed Eng. (2018) 2:158–64. doi: 10.1038/s41551-018-0195-0
81. Mutlu U, Colijn JM, Ikram MA, Bonnemaijer PWM, Licher S, Wolters FJ, et al. Association of retinal neurodegeneration on optical coherence tomography with dementia: a population-based study. JAMA Neurol. (2018) 75:1256–63. doi: 10.1001/jamaneurol.2018.1563
82. Abramoff MD, Lavin PT, Birch M, Shah N, and Folk JC. Pivotal trial of an autonomous AI-based diagnostic system for detection of diabetic retinopathy in primary care offices. NPJ Digit Med. (2018) 1:39. doi: 10.1038/s41746-018-0040-6
83. Kanagasingam Y, Xiao D, Vignarajan J, Preetham A, Tay-Kearney ML, and Mehrotra A. Evaluation of artificial intelligence-based grading of diabetic retinopathy in primary care. JAMA Netw Open. (2018) 1:e182665. doi: 10.1001/jamanetworkopen.2018.2665
84. Grzybowski A, Brona P, Lim G, Ruamviboonsuk P, Tan GSW, Abramoff M, et al. Artificial intelligence for diabetic retinopathy screening: a review. Eye. (2020) 34:451–60. doi: 10.1038/s41433-019-0566-0
85. Liu Y, Kohlberger T, Norouzi M, Dahl GE, Smith JL, Mohtashamian A, et al. Artificial intelligence-based breast cancer nodal metastasis detection: insights into the black box for pathologists. Arch Pathol Lab Med. (2019) 143:859–68. doi: 10.5858/arpa.2018-0147-OA
86. Lindsey R, Daluiski A, Chopra S, Lachapelle A, Mozer M, Sicular S, et al. Deep neural network improves fracture detection by clinicians. Proc Natl Acad Sci USA. (2018) 115:11591–96. doi: 10.1073/pnas.1806905115
87. Mori Y, Kudo SE, Misawa M, Saito Y, Ikematsu H, Hotta K, et al. Real-time use of artificial intelligence in identification of diminutive polyps during colonoscopy: a prospective study. Ann Intern Med. (2018) 169:357–66. doi: 10.7326/M18-0249
88. Turakhia MP, Desai M, Hedlin H, Rajmane A, Talati N, Ferris T, et al. Rationale and design of a large-scale, app-based study to identify cardiac arrhythmias using a smartwatch: the apple heart study. Am Heart J. (2019) 207:66–75. doi: 10.1016/j.ahj.2018.09.002
89. Ainscough BJ, Barnell EK, Ronning P, Campbell KM, Wagner AH, Fehniger TA, et al. A deep learning approach to automate refinement of somatic variant calling from cancer sequencing data. Nat Genet. (2018) 50:1735–43. doi: 10.1038/s41588-018-0257-y
90. Bowden SG, Gill BJA, Englander ZK, Horenstein CI, Zanazzi G, Chang PD, et al. Local glioma cells are associated with vascular dysregulation. AJNR Am J Neuroradiol. (2018) 39:507–14. doi: 10.3174/ajnr.A5526
91. Bello GA, Dawes TJW, Duan J, Biffi C, de Marvao A, Howard L, et al. Deep learning cardiac motion analysis for human survival prediction. Nat Mach Intell. (2019) 1:95–104. doi: 10.1038/s42256-019-0019-2
92. Uddin M, Tammimies K, Pellecchia G, Alipanahi B, Hu P, Wang Z, et al. Brain-expressed exons under purifying selection are enriched for de novo mutations in autism spectrum disorder. Nat Genet. (2014) 46:742–7. doi: 10.1038/ng.2980
93. Bone D, Goodwin MS, Black MP, Lee CC, Audhkhasi K, and Narayanan S. Applying machine learning to facilitate autism diagnostics: pitfalls and promises. J Autism Dev Disord. (2015) 45:1121–36. doi: 10.1007/s10803-014-2268-6
94. Lin H, Li R, Liu Z, Chen J, Yang Y, Chen H, et al. Diagnostic efficacy and therapeutic decision-making capacity of an artificial intelligence platform for childhood cataracts in eye clinics: a multicentre randomized controlled trial. EClinicalMedicine. (2019) 9:52–9. doi: 10.1016/j.eclinm.2019.03.001
95. Wu L, Zhang J, Zhou W, An P, Shen L, Liu J, et al. Randomised controlled trial of WISENSE, a real-time quality improving system for monitoring blind spots during esophagogastroduodenoscopy. Gut. (2019) 68:2161–69.
96. Wang P, Berzin TM, Glissen Brown JR, Bharadwaj S, Becq A, Xiao X, et al. Real-time automatic detection system increases colonoscopic polyp and adenoma detection rates: a prospective randomised controlled study. Gut. (2019) 68:1813–9. doi: 10.1136/gutjnl-2018-317500
97. Titano JJ, Badgeley M, Schefflein J, Pain M, Su A, Cai M, et al. Automated deep-neural-network surveillance of cranial images for acute neurologic events. Nat Med. (2018) 24:1337–41. doi: 10.1038/s41591-018-0147-y
98. Brocklehurst P, Field DJ, Juszczak E, Kenyon S, Linsell L, Newburn M, et al. The infant trial. Lancet. (2017) 390:28. doi: 10.1016/S0140-6736(17)31594-5
99. Sheikhalishahi S, Miotto R, Dudley JT, Lavelli A, Rinaldi F, and Osmani V. Natural language processing of clinical notes on chronic diseases: systematic review. JMIR Med Inform. (2019) 7:e12239. doi: 10.2196/12239
100. Hersh WR, Weiner MG, Embi PJ, Logan JR, Payne PR, Bernstam EV, et al. Caveats for the use of operational electronic health record data in comparative effectiveness research. Med Care. (2013) 51:S30–7. doi: 10.1097/MLR.0b013e31829b1dbd
101. Poldrack RA, Baker CI, Durnez J, Gorgolewski KJ, Matthews PM, Munafo MR, et al. Scanning the horizon: towards transparent and reproducible neuroimaging research. Nat Rev Neurosci. (2017) 18:115–26. doi: 10.1038/nrn.2016.167
102. t Hoen PA, Friedlander MR, Almlof J, Sammeth M, Pulyakhina I, Anvar SY, et al. Reproducibility of high-throughput mRNA and small RNA sequencing across laboratories. Nat Biotechnol. (2013) 31:1015–22. doi: 10.1038/nbt.2702
103. Leek JT, Scharpf RB, Bravo HC, Simcha D, Langmead B, Johnson WE, et al. Tackling the widespread and critical impact of batch effects in high-throughput data. Nat Rev Genet. (2010) 11:733–9. doi: 10.1038/nrg2825
104. Davis SE, Greevy RA, Fonnesbeck C, Lasko TA, Walsh CG, and Matheny ME. A nonparametric updating method to correct clinical prediction model drift. J Am Med Inform Assoc. (2019) 26:1448–57. doi: 10.1093/jamia/ocz127
105. Panch T, Mattie H, and Celi LA. The “inconvenient truth” about AI in healthcare. NPJ Digit Med. (2019) 2:77. doi: 10.1038/s41746-019-0155-4
106. Dorado-Diaz PI, Sampedro-Gomez J, Vicente-Palacios V, and Sanchez PL. Applications of artificial intelligence in cardiology. The future is already here. Rev Esp Cardiol. (2019) 72:1065–75. doi: 10.1016/j.rec.2019.05.014
107. Niel O, and Bastard P. Artificial intelligence in nephrology: core concepts, clinical applications, and perspectives. Am J Kidney Dis. (2019) 74:803–10. doi: 10.1053/j.ajkd.2019.05.020
108. Lazer D, Kennedy R, King G, and Vespignani A. Big data. The parable of Google Flu: traps in big data analysis. Science. (2014) 343:1203–5. doi: 10.1126/science.1248506
109. Kim DW, Jang HY, Kim KW, Shin Y, and Park SH. Design characteristics of studies reporting the performance of artificial intelligence algorithms for diagnostic analysis of medical images: results from recently published papers. Korean J Radiol. (2019) 20:405–10. doi: 10.3348/kjr.2019.0025
110. Crawford K, and Calo R. There is a blind spot in AI research. Nature. (2016) 538:311–3. doi: 10.1038/538311a
111. Zook M, Barocas S, Boyd D, Crawford K, Keller E, Gangadharan SP, et al. Ten simple rules for responsible big data research. PLoS Comput Biol. (2017) 13:e1005399. doi: 10.1371/journal.pcbi.1005399
112. Coe BP, Girirajan S, and Eichler EE. The genetic variability and commonality of neurodevelopmental disease. Am J Med Genet C Semin Med Genet. (2012) 160C:118–29. doi: 10.1002/ajmg.c.31327
113. Debray TP, Vergouwe Y, Koffijberg H, Nieboer D, Steyerberg EW, and Moons KG. A new framework to enhance the interpretation of external validation studies of clinical prediction models. J Clin Epidemiol. (2015) 68:279–89. doi: 10.1016/j.jclinepi.2014.06.018
114. Winkler JK, Fink C, Toberer F, Enk A, Deinlein T, Hofmann-Wellenhof R, et al. Association between surgical skin markings in dermoscopic images and diagnostic performance of a deep learning convolutional neural network for melanoma recognition. JAMA Dermatol. (2019) 155:1135–41. doi: 10.1001/jamadermatol.2019.1735
115. Fink C, Blum A, Buhl T, Mitteldorf C, Hofmann-Wellenhof R, Deinlein T, et al. Diagnostic performance of a deep learning convolutional neural network in the differentiation of combined naevi and melanomas. J Eur Acad Dermatol Venereol. (2019) 34:1355–61. doi: 10.1111/jdv.16165
116. Ward-Peterson M, Acuna JM, Alkhalifah MK, Nasiri AM, Al-Akeel ES, Alkhaldi TM, et al. Association between race/ethnicity and survival of melanoma patients in the United States over 3 decades: a secondary analysis of SEER data. Medicine. (2016) 95:e3315. doi: 10.1097/MD.0000000000003315
117. Shah NH, Milstein A, and Bagley DS. Making machine learning models clinically useful. JAMA. (2019) 322:1351–2. doi: 10.1001/jama.2019.10306
118. Saito T, and Rehmsmeier M. The precision-recall plot is more informative than the ROC plot when evaluating binary classifiers on imbalanced datasets. PLoS ONE. (2015) 10:e0118432. doi: 10.1371/journal.pone.0118432
119. He D, Choi A, Pipatsrisawat K, Darwiche A, and Eskin E. Optimal algorithms for haplotype assembly from whole-genome sequence data. Bioinformatics. (2010) 26:i183–90. doi: 10.1093/bioinformatics/btq215
120. Alyass A, Turcotte M, and Meyre D. From big data analysis to personalized medicine for all: challenges and opportunities. BMC Med Genom. (2015) 8:33. doi: 10.1186/s12920-015-0108-y
121. Alipanahi B, Delong A, Weirauch MT, and Frey BJ. Predicting the sequence specificities of DNA- and RNA-binding proteins by deep learning. Nat Biotechnol. (2015) 33:831–8. doi: 10.1038/nbt.3300
122. Xiong HY, Alipanahi B, Lee LJ, Bretschneider H, Merico D, Yuen RK, et al. RNA splicing. The human splicing code reveals new insights into the genetic determinants of disease. Science. (2015) 347:1254806. doi: 10.1126/science.1254806
123. Kosmicki JA, Sochat V, Duda M, and Wall DP. Searching for a minimal set of behaviors for autism detection through feature selection-based machine learning. Transl Psychiatry. (2015) 5:e514. doi: 10.1038/tp.2015.7
124. Wall DP, Dally R, Luyster R, Jung JY, and Deluca TF. Use of artificial intelligence to shorten the behavioral diagnosis of autism. PLoS ONE. (2012) 7:e43855. doi: 10.1371/journal.pone.0043855
125. Poduri A, Evrony GD, Cai X, and Walsh CA. Somatic mutation, genomic variation, neurological disease. Science. (2013) 341:1237758. doi: 10.1126/science.1237758
126. van Loo KM, and Martens GJ. Genetic and environmental factors in complex neurodevelopmental disorders. Curr Genom. (2007) 8:429–44. doi: 10.2174/138920207783591717
127. Tran NQV, and Miyake K. Neurodevelopmental disorders and environmental toxicants: epigenetics as an underlying mechanism. Int J Genom. (2017) 2017:7526592. doi: 10.1155/2017/7526592
128. Lim ET, Uddin M, De Rubeis S, Chan Y, Kamumbu AS, Zhang X, et al. Rates, distribution and implications of postzygotic mosaic mutations in autism spectrum disorder. Nat Neurosci. (2017) 20:1217–24. doi: 10.1038/nn.4598
129. McConnell MV, Turakhia MP, Harrington RA, King AC, and Ashley EA. Mobile health advances in physical activity, fitness, and atrial fibrillation: moving hearts. J Am Coll Cardiol. (2018) 71:2691–701. doi: 10.1016/j.jacc.2018.04.030
130. Zhou J, and Troyanskaya OG. Predicting effects of noncoding variants with deep learning-based sequence model. Nat Methods. (2015) 12:931–4. doi: 10.1038/nmeth.3547
131. Motsinger AA, and Ritchie MD. Multifactor dimensionality reduction: an analysis strategy for modelling and detecting gene-gene interactions in human genetics and pharmacogenomics studies. Hum Genom. (2006) 2:318–28. doi: 10.1186/1479-7364-2-5-318
132. Yosef N, Yakhini Z, Tsalenko A, Kristensen V, Borresen-Dale AL, Ruppin E, et al. A supervised approach for identifying discriminating genotype patterns and its application to breast cancer data. Bioinformatics. (2007) 23:e91–8. doi: 10.1093/bioinformatics/btl298
133. Grove J, Ripke S, Als TD, Mattheisen M, Walters RK, Won H, et al. Identification of common genetic risk variants for autism spectrum disorder. Nat Genet. (2019) 51:431–44. doi: 10.1038/s41588-019-0344-8
134. Wright CF, McRae JF, Clayton S, Gallone G, Aitken S, FitzGerald TW, et al. Making new genetic diagnoses with old data: iterative reanalysis and reporting from genome-wide data in 1,133 families with developmental disorders. Genet Med. (2018) 20:1216–23. doi: 10.1038/gim.2017.246
135. Krol A, and Feng G. Windows of opportunity: timing in neurodevelopmental disorders. Curr Opin Neurobiol. (2018) 48:59–63. doi: 10.1016/j.conb.2017.10.014
136. Liu X, Faes L, Kale AU, Wagner SK, Fu DJ, Bruynseels A, et al. A comparison of deep learning performance against health-care professionals in detecting diseases from medical imaging: a systematic review and meta-analysis. Lancet Digit Health. (2019) 1:e271–97. doi: 10.1016/S2589-7500(19)30123-2
137. Topol EJ. A decade of digital medicine innovation. Sci Transl Med. (2019) 11:eaaw7610. doi: 10.1126/scitranslmed.aaw7610
138. Delclaux C. No need for pulmonologists to interpret pulmonary function tests. Eur Respir J. (2019) 54:1900829. doi: 10.1183/13993003.00829-2019
139. Mayo RC, and Leung J. Artificial intelligence and deep learning - radiology's next frontier? Clin Imaging. (2018) 49:87–8. doi: 10.1016/j.clinimag.2017.11.007
140. Fenton JJ, Taplin SH, Carney PA, Abraham L, Sickles EA, D'Orsi C, et al. Influence of computer-aided detection on performance of screening mammography. N Engl J Med. (2007) 356:1399–409. doi: 10.1056/NEJMoa066099
141. Alcusky M, Philpotts L, Bonafede M, Clarke J, and Skoufalos A. The patient burden of screening mammography recall. J Womens Health. (2014) 23(Suppl.):S11–9. doi: 10.1089/jwh.2014.1511
142. Wu KW, Wu E, Tan Y, Sorensen H, Wang G, Lotter M, et al. Validation of a deep learning mammography model in a population with low screening rates. arXiv (2019).
143. Aschwanden C. Artificial Intelligence Makes Bad Medicine even Worse. (2020). Available online at: https://www.wired.com/story/artificial-intelligence-makes-bad-medicine-even-worse/ (accessed October 5, 2020).
144. Holzinger A, Biemann C, Pattichis CS, and Kell DB. What do we need to build explainable AI systems for the medical domain? arXiv. Available onlie at: https://arxiv.org/abs/1712.09923
145. Bologna G, and Hayashi Y. Characterization of symbolic rules embedded in deep DIMLP networks: a challenge to transparency of deep learning. J Art Intel Soft Comput Res. (2017) 7:265–286. doi: 10.1515/jaiscr-2017-0019
146. Chaiyachati KH, Shea JA, Asch DA, Liu M, Bellini LM, Dine CJ, et al. Assessment of inpatient time allocation among first-year internal medicine residents using time-motion observations. JAMA Intern Med. (2019) 179:760–67. doi: 10.1001/jamainternmed.2019.0095
147. West CP, Dyrbye LN, and Shanafelt TD. Physician burnout: contributors, consequences and solutions. J Intern Med. (2018) 283:516–29. doi: 10.1111/joim.12752
148. Shah NR. Health care in 2030: will artificial intelligence replace physicians? Ann Intern Med. (2019) 170:407–8. doi: 10.7326/M19-0344
149. Topol EJ. High-performance medicine: the convergence of human and artificial intelligence. Nat Med. (2019) 25:44–56. doi: 10.1038/s41591-018-0300-7
150. Mintz Y, and Brodie R. Introduction to artificial intelligence in medicine. Minim Invasive Ther Allied Technol. (2019) 28:73–81. doi: 10.1080/13645706.2019.1575882
151. Prasad VK, and Citu AS. Ending Medical Reversal: Improving Outcomes, Saving Lives. Johns Hopkins Press Health Books. (2015). Available online at: https://jhupbooks.press.jhu.edu/title/ending-medical-reversal (accessed October 5, 2020).
152. Ilan Y. Immune rebalancing by oral immunotherapy: a novel method for getting the immune system back on track. J Leukoc Biol. (2019) 105:463–72. doi: 10.1002/JLB.5RU0718-276RR
153. Ilan Y. beta-glycosphingolipids as mediators of both inflammation and immune tolerance: a manifestation of randomness in biological systems. Front Immunol. (2019) 10:1143. doi: 10.3389/fimmu.2019.01143
154. Shaw J, Rudzicz F, Jamieson T, and Goldfarb A. Artificial intelligence and the implementation challenge. J Med Internet Res. (2019) 21:e13659. doi: 10.2196/13659
155. Roda G, Jharap B, Neeraj N, and Colombel JF. Loss of response to anti-TNFs: definition, epidemiology, and management. Clin Transl Gastroenterol. (2016) 7:e135. doi: 10.1038/ctg.2015.63
156. Pagnini C, Pizarro TT, and Cominelli F. Novel pharmacological therapy in inflammatory bowel diseases: beyond anti-tumor necrosis factor. Front Pharmacol. (2019) 10:671. doi: 10.3389/fphar.2019.00671
157. Rovini E, Maremmani C, and Cavallo F. How wearable sensors can support Parkinson's disease diagnosis and treatment: a systematic review. Front Neurosci. (2017) 11:555. doi: 10.3389/fnins.2017.00555
158. Khoury T, and Ilan Y. Introducing patterns of variability for overcoming compensatory adaptation of the immune system to immunomodulatory agents: a novel method for improving clinical response to anti-TNF therapies. Front Immunol. (2019) 10:2726. doi: 10.3389/fimmu.2019.02726
159. Kenig A, and Ilan Y. A personalized signature and chronotherapy-based platform for improving the efficacy of sepsis treatment. Front Physiol. (2019) 10:1542. doi: 10.3389/fphys.2019.01542
160. Ilan Y. Generating randomness: making the most out of disordering a false order into a real one. J Transl Med. (2019) 17:49. doi: 10.1186/s12967-019-1798-2
161. Vickers AJ, Cronin AM, Elkin EB, and Gonen M. Extensions to decision curve analysis, a novel method for evaluating diagnostic tests, prediction models and molecular markers. BMC Med Inform Decis Mak. (2008) 8:53. doi: 10.1186/1472-6947-8-53
162. Huynh-Thu VA, Saeys Y, Wehenkel L, and Geurts P. Statistical interpretation of machine learning-based feature importance scores for biomarker discovery. Bioinformatics. (2012) 28:1766–74. doi: 10.1093/bioinformatics/bts238
163. Collins GS, Reitsma JB, Altman DG, Moons KG, and Group T. Transparent reporting of a multivariable prediction model for individual prognosis or diagnosis (TRIPOD): the TRIPOD statement. The TRIPOD Group. Circulation. (2015) 131:211–9. doi: 10.1161/CIRCULATIONAHA.114.014508
164. Collins GS, and Moons KGM. Reporting of artificial intelligence prediction models. Lancet. (2019) 393:1577–9. doi: 10.1016/S0140-6736(19)30037-6
165. Ilan Y. Overcoming randomness does not rule out the importance of inherent randomness for functionality. J Biosci. (2019) 44:132. doi: 10.1007/s12038-019-9958-3
166. Ilan Y. Advanced tailored randomness: a novel approach for improving the efficacy of biological systems. J Comput Biol. (2020) 27:20–9. doi: 10.1089/cmb.2019.0231
167. Ilan Y, and Ber Ilan T. A Subject Sepcific System and Method for Prevention of Body Adapatation for Chronic Treatment of Disease. WO 2019/008571 Al 2019 (2019). Available online at: https://patents.google.com/patent/WO2019008571A1/en (accessed October 5, 2020).
168. Ilan Y. Methods and System for Modulating Physiological States Between Biological Entities. Patent 2018. US 2018./0328917 A1. (2018).
169. Ilan Y. Order through disorder: the characteristic variability of systems. Front Cell Dev Biol. (2020) 8:186. doi: 10.3389/fcell.2020.00186
170. de Lorenzo V., and Schmidt M. Biological standards for the knowledge-based bioeconomy: what is at stake. N Biotechnol. (2018) 40:170–80. doi: 10.1016/j.nbt.2017.05.001
171. Gsponer J, and Babu MM. The rules of disorder or why disorder rules. Prog Biophys Mol Biol. (2009) 99:94–103. doi: 10.1016/j.pbiomolbio.2009.03.001
172. Buckle AM, and Borg NA. Integrating experiment and theory to understand TCR-pMHC dynamics. Front Immunol. (2018) 9:2898. doi: 10.3389/fimmu.2018.02898
173. Lodygin D, and Flugel A. Intravital real-time analysis of T-cell activation in health and disease. Cell Calcium. (2017) 64:118–29. doi: 10.1016/j.ceca.2016.12.007
174. Montevil M, Mossio M, Pocheville A, and Longo G. Theoretical principles for biology: variation. Prog Biophys Mol Biol. (2016) 122:36–50. doi: 10.1016/j.pbiomolbio.2016.08.005
175. Longo G, Montevil M, Sonnenschein C, and Soto AM. In search of principles for a theory of organisms. J Biosci. (2015) 40:955–68. doi: 10.1007/s12038-015-9574-9
176. Longo G, Montevil M, and Pocheville A. From bottom-up approaches to levels of organization and extended critical transitions. Front Physiol. (2012) 3:232. doi: 10.3389/fphys.2012.00232
177. Buiatti M, and Longo G. Randomness and multilevel interactions in biology. Theory Biosci. (2013) 132:139–58. doi: 10.1007/s12064-013-0179-2
178. Yang X. Multitissue multiomics systems biology to dissect complex diseases. Trends Mol Med. (2020) 26:718–28. doi: 10.1016/j.molmed.2020.04.006
179. Varella MAC. The biology and evolution of the three psychological tendencies to anthropomorphize biology and evolution. Front Psychol. (2018) 9:1839. doi: 10.3389/fpsyg.2018.01839
180. El-Haj M, Kanovitch D, and Ilan Y. Personalized inherent randomness of the immune system is manifested by an individualized response to immune triggers and immunomodulatory therapies: a novel platform for designing personalized immunotherapies. Immunol Res. (2019) 67:337–47. doi: 10.1007/s12026-019-09101-y
182. Trewavas A. A brief history of systems biology. “Every object that biology studies is a system of systems.” Francois Jacob 1974. Plant Cell. (2006) 18:2420–30. doi: 10.1105/tpc.106.042267
183. Tsimring LS. Noise in biology. Reports on progress in physics. Phys Soc. (2014) 77:026601. doi: 10.1088/0034-4885/77/2/026601
184. Latta RG. Conservation genetics as applied evolution: from genetic pattern to evolutionary process. Evol Appl. (2008) 1:84–94. doi: 10.1111/j.1752-4571.2007.00008.x
185. Latta R. Natural selection, variation, adaptation, and evolution: a primer of interrelated concepts. Int J Plant Sci. (2010) 131:930–44. doi: 10.1086/656220
186. van Boxtel C, van Heerden JH, Nordholt N, Schmidt P, and Bruggeman FJ. Taking chances and making mistakes: non-genetic phenotypic heterogeneity and its consequences for surviving in dynamic environments. J R Soc Interface. (2017) 14:20170141. doi: 10.1098/rsif.2017.0141
187. Hardy CM, Burke MK, Everett LJ, Han MV, Lantz KM, and Gibbs AG. Genome-wide analysis of starvation-selected drosophila melanogaster-a genetic model of obesity. Mol Biol Evol. (2018) 35:50–65. doi: 10.1093/molbev/msx254
188. Hermisson J, and Wagner GP. The population genetic theory of hidden variation and genetic robustness. Genetics. (2004) 168:2271–84. doi: 10.1534/genetics.104.029173
189. Paaby AB, and Rockman MV. Cryptic genetic variation: evolution's hidden substrate. Nat Rev Genetics. (2014) 15:247–58. doi: 10.1038/nrg3688
190. Ledon-Rettig CC, Pfennig DW, Chunco AJ, and Dworkin I. Cryptic genetic variation in natural populations: a predictive framework. Integr Comp Biol. (2014) 54:783–93. doi: 10.1093/icb/icu077
191. Rego-Costa A, Debarre F, and Chevin LM. Chaos and the (un)predictability of evolution in a changing environment. Evolution. (2018) 72:375–85. doi: 10.1111/evo.13407
192. Freeman WJ, Kozma R, and Werbos PJ. Biocomplexity: adaptive behavior in complex stochastic dynamical systems. Bio Syst. (2001) 59:109–23. doi: 10.1016/S0303-2647(00)00146-5
193. Ramsey PC. Chance in Evolution. The University of Chicago Press Books (2016). Available online at: https://www.press.uchicago.edu/ucp/books/book/chicago/C/bo24550500.html (accessed October 5, 2020).
194. Clarke A, and Crame JA. Evolutionary dynamics at high latitudes: speciation and extinction in polar marine faunas. Philos Trans R Soc London Series B Biol Sci. (2010) 365:3655–66. doi: 10.1098/rstb.2010.0270
195. Farahpour F, Saeedghalati M, Brauer VS, and Hoffmann D. Trade-off shapes diversity in eco-evolutionary dynamics. eLife. (2018) 7:e36273. doi: 10.7554/eLife.36273
196. Ilan Y. Randomness in microtubule dynamics: an error that requires correction or an inherent plasticity required for normal cellular function? Cell Biol Int. (2019) 43:739–48. doi: 10.1002/cbin.11157
197. Singh N, Moneghetti KJ, Christle JW, Hadley D, Froelicher V, and Plews D. Heart rate variability: an old metric with new meaning in the era of using mhealth technologies for health and exercise training guidance. Part two: prognosis and training. Arrhythm Electrophysiol Rev. (2018) 7:247–55. doi: 10.15420/aer.2018.30.2
198. Lees T, Shad-Kaneez F, Simpson AM, Nassif NT, Lin Y, and Lal S. Heart rate variability as a biomarker for predicting stroke, post-stroke complications and functionality. Biomark Insights. (2018) 13:1177271918786931. doi: 10.1177/1177271918786931
199. Herssens N, Verbecque E, Hallemans A, Vereeck L, Van Rompaey V, and Saeys W. Do spatiotemporal parameters and gait variability differ across the lifespan of healthy adults? A systematic review. Gait Posture. (2018) 64:181–90. doi: 10.1016/j.gaitpost.2018.06.012
200. Henriques T, Munshi MN, Segal AR, Costa MD, and Goldberger AL. “Glucose-at-a-Glance”: new method to visualize the dynamics of continuous glucose monitoring data. J Diabetes Sci Technol. (2014) 8:299–306. doi: 10.1177/1932296814524095
201. Tosato F, Bernardi D, Sanzari MC, Pantano G, and Plebani M. Biological variability of lymphocyte subsets of human adults' blood. Clin Chim Acta. (2013) 424:159–63. doi: 10.1016/j.cca.2013.06.001
202. Cobb M. 60 years ago, Francis Crick changed the logic of biology. PLoS Biol. (2017) 15:e2003243. doi: 10.1371/journal.pbio.2003243
203. Noble D. Evolution viewed from physics, physiology and medicine. Interface Focus. (2017) 7:20160159. doi: 10.1098/rsfs.2016.0159
204. Corre G, Stockholm D, Arnaud O, Kaneko G, Vinuelas J, Yamagata Y, et al. Stochastic fluctuations and distributed control of gene expression impact cellular memory. PLoS ONE. (2014) 9:e115574. doi: 10.1371/journal.pone.0115574
205. Deaton AM, and Bird A. CpG islands and the regulation of transcription. Genes Dev. (2011) 25:1010–22. doi: 10.1101/gad.2037511
206. Kupiec J-J. The Origin of Individuals: a Darwinian Approach to Developmental Biology. London, UK: World Scientific Publishing Company (2009). doi: 10.1142/6359
207. Zhang Z, and Gerstein M. Patterns of nucleotide substitution, insertion and deletion in the human genome inferred from pseudogenes. Nucleic Acids Res. (2003) 31:5338–48. doi: 10.1093/nar/gkg745
208. Birky CW Jr, Adams J, Gemmel M, and Perry J. Using population genetic theory and DNA sequences for species detection and identification in asexual organisms. PLoS ONE. (2010) 5:e10609. doi: 10.1371/journal.pone.0010609
209. Lynch M, Ackerman MS, Gout JF, Long H, Sung W, Thomas WK, et al. Genetic drift, selection and the evolution of the mutation rate. Nat Rev Gen. (2016) 17:704–14. doi: 10.1038/nrg.2016.104
210. Zhou JHS, Markham JF, Duffy KR, and Hodgkin PD. Stochastically timed competition between division and differentiation fates regulates the transition from B lymphoblast to plasma cell. Front Immunol. (2018) 9:2053. doi: 10.3389/fimmu.2018.02053
211. Hodgkin PD. Modifying clonal selection theory with a probabilistic cell. Immunol Rev. (2018) 285:249–62. doi: 10.1111/imr.12695
212. Mitchell S, Roy K, Zangle TA, and Hoffmann A. Nongenetic origins of cell-to-cell variability in B lymphocyte proliferation. Proc Natl Acad Sci USA. (2018) 115:E2888–97. doi: 10.1073/pnas.1715639115
213. Fuchs A, Gliwinski M, Grageda N, Spiering R, Abbas AK, Koenen AS, et al. Minimum information about T regulatory cells: a step toward reproducibility and standardization. Front Immunol. (2017) 8:1844. doi: 10.3389/fimmu.2017.01844
214. Liebers V, Kendzia B, Stubel H, Borowitzki G, Gering V, Monse C, et al. Cell activation and cytokine release ex vivo: estimation of reproducibility of the whole-blood assay with fresh human blood. Adv Exp Med Biol. (2018) 1108:25–36. doi: 10.1007/5584_2018_225
215. Orsini C, Dankulov MM, Colomer-de-Simon P, Jamakovic A, Mahadevan P, Vahdat A, et al. Quantifying randomness in real networks. Nat Commun. (2015) 6:8627. doi: 10.1038/ncomms9627
216. Rajdl K, Lansky P, and Kostal L. Entropy factor for randomness quantification in neuronal data. Neural Netw. (2017) 95:57–65. doi: 10.1016/j.neunet.2017.07.016
217. Longo G, and Bertolotti T. Comparing symmetries in models and simulations. In: Handbook of Model-Based Science. Cham: Springer Handbooks. Springer (2017). p. 1–15. doi: 10.1007/978-3-319-30526-4_38
218. Calude CL, and Longo G. Classical, quantum and biological randomness as relative unpredictability. Nat Comput. (2016) 15:263–78. doi: 10.1007/s11047-015-9533-2
219. Ghanjal A, Motaqi M, Arab Z, and Hatef B. Force variability in the short- and long-term type 2 diabetes mellitus. J Med Signals Sens. (2019) 9:50–8. doi: 10.4103/jmss.JMSS_24_18
220. Snijder B, and Pelkmans L. Origins of regulated cell-to-cell variability. Nat Rev Mol Cell Biol. (2011) 12:119–25. doi: 10.1038/nrm3044
221. Selvarajoo K. Complexity of biochemical and genetic responses reduced using simple theoretical models. Methods Mol Biol. (2018) 1702:171–201. doi: 10.1007/978-1-4939-7456-6_9
222. Brandao FG, Harrow AW, and Horodecki M. Efficient quantum pseudorandomness. Phys Rev Lett. (2016) 116:170502. doi: 10.1103/PhysRevLett.116.170502
223. Earnest TM, Cole JA, and Luthey-Schulten Z. Simulating biological processes: stochastic physics from whole cells to colonies. Reports on progress in physics. Phys Soc. (2018) 81:052601. doi: 10.1088/1361-6633/aaae2c
224. Bertolaso M, Capolupo A, Cherubini C, Filippi S, Gizzi A, Loppini A, et al. The role of coherence in emergent behavior of biological systems. Electromagn Biol Med. (2015) 34:138–40. doi: 10.3109/15368378.2015.1036069
226. Ilan-Ber T, and Ilan Y. The role of microtubules in the immune system and as potential targets for gut-based immunotherapy. Mol Immunol. (2019) 111:73–82. doi: 10.1016/j.molimm.2019.04.014
227. Mitchison T, and Kirschner M. Dynamic instability of microtubule growth. Nature. (1984) 312:237–42. doi: 10.1038/312237a0
228. Alberch P. The logic of monsters: evidence for internal constraint in development and evolution. Geobios. (1989) 22(Suppl.2):21–57. doi: 10.1016/S0016-6995(89)80006-3
229. Martinez-Lavin M, Infante O, and Lerma C. Hypothesis: the chaos and complexity theory may help our understanding of fibromyalgia and similar maladies. Semin Arthritis Rheum. (2008) 37:260–4. doi: 10.1016/j.semarthrit.2007.04.003
230. Lipsitz LA, and Goldberger AL. Loss of 'complexity' and aging. Potential applications of fractals and chaos theory to senescence. JAMA. (1992) 267:1806–9. doi: 10.1001/jama.267.13.1806
231. Lipsitz LA. Dynamics of stability: the physiologic basis of functional health and frailty. J Gerontol A Biol Sci Med Sci. (2002) 57:B115–25. doi: 10.1093/gerona/57.3.B115
232. Kyriazis M. Applications of chaos theory to the molecular biology of aging. Exp Gerontol. (1991) 26:569–72. doi: 10.1016/0531-5565(91)90074-V
233. Soloviev MV. On possible role of chaotic behavior of the gene regulation system in aging. Adv Gerontol. (2001) 8:27–33.
234. Kyriazis M. Practical applications of chaos theory to the modulation of human ageing: nature prefers chaos to regularity. Biogerontology. (2003) 4:75–90. doi: 10.1023/A:1023306419861
235. Rezania V, Coombe D, and Tuszynski JA. A physiologically-based flow network model for hepatic drug elimination III: 2D/3D DLA lobule models. Theor Biol Med Model. (2016) 13:9. doi: 10.1186/s12976-016-0034-5
236. Contin M, Alberghini L, Candela C, Benini G, and Riva R. Intrapatient variation in antiepileptic drug plasma concentration after generic substitution vs stable brand-name drug regimens. Epilepsy Res. (2016) 122:79–83. doi: 10.1016/j.eplepsyres.2016.02.012
237. Del Bello, Congy-Jolivet N, Danjoux M, Muscari F, Lavayssiere L, Esposito L, et al. High tacrolimus intra-patient variability is associated with graft rejection, and de novo donor-specific antibodies occurrence after liver transplantation. World J Gastroenterol. (2018) 24:1795–802. doi: 10.3748/wjg.v24.i16.1795
238. Leino AD, King EC, Jiang W, Vinks AA, Klawitter J, Christians U, et al. Assessment of tacrolimus intrapatient variability in stable adherent transplant recipients: establishing baseline values. Am J Transpl. (2018) 19:1410–20. doi: 10.1111/ajt.15199
239. Gueta I, Markovits N, Yarden-Bilavsky H, Raichlin E, Freimark D, Lavee J, et al. High tacrolimus trough level variability is associated with rejections after heart transplant. Am J Transpl. (2018) 18:2571–8. doi: 10.1111/ajt.15016
240. Gueta I, Markovits N, Yarden-Bilavsky H, Raichlin E, Freimark D, Lavee J, et al. Intrapatient variability in tacrolimus trough levels after solid organ transplantation varies at different postoperative time periods. Am J Transpl. (2018) 19:611. doi: 10.1111/ajt.15134
241. Elgart V, Lin JR, and Loscalzo J. Determinants of drug-target interactions at the single cell level. PLoS Comput Biol. (2018) 14:e1006601. doi: 10.1371/journal.pcbi.1006601
242. Mougabure-Cueto G, and Sfara V. The analysis of dose-response curve from bioassays with quantal response: deterministic or statistical approaches? Toxicol Lett. (2016) 248:46–51. doi: 10.1016/j.toxlet.2016.03.001
243. Gisbert JP, and Panes J. Loss of response and requirement of infliximab dose intensification in Crohn's disease: a review. Am J Gastroenterol. (2009) 104:760–7. doi: 10.1038/ajg.2008.88
244. Billioud V, Sandborn WJ, and Peyrin-Biroulet L. Loss of response and need for adalimumab dose intensification in Crohn's disease: a systematic review. Am J Gastroenterol. (2011) 106:674–84. doi: 10.1038/ajg.2011.60
245. Ma C, Huang V, Fedorak DK, Kroeker KI, Dieleman LA, Halloran BP, et al. Crohn's disease outpatients treated with adalimumab have an earlier secondary loss of response and requirement for dose escalation compared to infliximab: a real life cohort study. J Crohns Colitis. (2014) 8:1454–63. doi: 10.1016/j.crohns.2014.05.007
246. Huang HC, Mallidi S, Liu J, Chiang CT, Mai Z, Goldschmidt R, et al. Photodynamic therapy synergizes with irinotecan to overcome compensatory mechanisms and improve treatment outcomes in pancreatic cancer. Cancer Res. (2016) 76:1066–77. doi: 10.1158/0008-5472.CAN-15-0391
247. Rueff J, and Rodrigues AS. Cancer drug resistance: a brief overview from a genetic viewpoint. Methods Mol Biol. (2016) 1395:1–18. doi: 10.1007/978-1-4939-3347-1_1
248. Bennabi D, Aouizerate B, El-Hage W, Doumy O, Moliere F, Courtet P, et al. Risk factors for treatment resistance in unipolar depression: a systematic review. J Affect Disord. (2015) 171:137–41. doi: 10.1016/j.jad.2014.09.020
249. Widdess-Walsh P, and Devinsky O. Antiepileptic drug resistance and tolerance in epilepsy. Rev Neurol Dis. (2007) 4:194–202.
250. Weiner WJ, Koller WC, Perlik S, Nausieda PA, and Klawans HL. Drug holiday and management of Parkinson disease. Neurology. (1980) 30:1257–61. doi: 10.1212/WNL.30.12.1257
251. Toni T, and Tidor B. Combined model of intrinsic and extrinsic variability for computational network design with application to synthetic biology. PLoS Comput Biol. (2013) 9:e1002960. doi: 10.1371/journal.pcbi.1002960
252. Rensing N, Han L, and Wong M. Intermittent dosing of rapamycin maintains antiepileptogenic effects in a mouse model of tuberous sclerosis complex. Epilepsia. (2015) 56:1088–97. doi: 10.1111/epi.13031
253. Ben-Horin S, Kopylov U, and Chowers Y. Optimizing anti-TNF treatments in inflammatory bowel disease. Autoimmun Rev. (2014) 13:24–30. doi: 10.1016/j.autrev.2013.06.002
254. Ferriols-Lisart R, and Ferriols-Lisart F. Dose modifications of anti-TNF drugs in rheumatoid arthritis patients under real-world settings: a systematic review. Rheumatol Int. (2015) 35:1193–210. doi: 10.1007/s00296-015-3222-4
255. Pontes C, Gratacos J, Torres F, Avendano C, Sanz J, Vallano A, et al. Evaluation of dose reduction versus standard dosing for maintenance of remission in patients with spondyloarthritis and clinical remission with anti-TNF (REDES-TNF): study protocol for a randomized controlled trial. Trials. (2015) 16:370. doi: 10.1186/s13063-015-0828-5
256. Inciarte-Mundo J, Hernandez MV, Rosario V, Ruiz-Esquide V, Cabrera-Villalba S, Ramirez J, et al. Reduction of biological agent dose in rheumatic diseases: descriptive analysis of 153 patients in clinical practice conditions. Reumatol Clin. (2014) 10:10–6. doi: 10.1016/j.reumae.2013.11.005
257. Strik AB, Mould S, Mathôt D, Ponsioen R, van den Brande C, Jansen J, et al. Dashboard driven vs. conventional dosing of infliximab in inflammatory bowel disease patients: the PRECISION trial. J Crohn's Colitis. (2019) 13:S063. doi: 10.1093/ecco-jcc/jjy222.090
258. Ilan Y. Why targeting the microbiome is not so successful: can randomness overcome the adaptation that occurs following gut manipulation? Clin Exp Gastroenterol. (2019) 12:209–17. doi: 10.2147/CEG.S203823
259. Stergiou N, and Decker LM. Human movement variability, nonlinear dynamics, and pathology: is there a connection? Hum Mov Sci. (2011) 30:869–88. doi: 10.1016/j.humov.2011.06.002
260. Kessler A, Weksler-Zangen S, and Ilan Y. Role of the immune system and the circadian rhythm in the pathogenesis of chronic pancreatitis: establishing a personalized signature for improving the effect of immunotherapies for chronic pancreatitis. Pancreas. (2020) 49:1024–32. doi: 10.1097/MPA.0000000000001626
261. Kolben Y, Weksler-Zangen S, and Ilan Y. Adropin as a potential mediator of the metabolic system-autonomic nervous system-chronobiology axis: Implementing a personalized signature-based platform for chronotherapy. Obes Rev. (2020). doi: 10.1111/obr.13108
262. Ilan Y. Overcoming compensatory mechanisms toward chronic drug administration to ensure long-term, sustainable beneficial effects. Mol Ther Methods Clin Dev. (2020) 18:335–44. doi: 10.1016/j.omtm.2020.06.006
263. Potruch A, Khoury ST, and Ilan Y. The role of chronobiology in drug-resistance epilepsy: the potential use of a variability and chronotherapy-based individualized platform for improving the response to anti-seizure drugs. Seizure. (2020) 80:201–11. doi: 10.1016/j.seizure.2020.06.032
264. Forkosh E, Kenig A, and Ilan Y. Introducing variability in targeting the microtubules: review of current mechanisms and future directions in colchicine therapy. Pharmacol Res Perspect. (2020) 8:e00616. doi: 10.1002/prp2.616
265. Gelman R, Bayatra A, Kessler A, Schwartz A, and Ilan Y. Targeting SARS-CoV-2 receptors as a means for reducing infectivity and improving antiviral and immune response: an algorithm-based method for overcoming resistance to antiviral agents. Emerg Microbes Infect. (2020) 9:1397–406. doi: 10.1080/22221751.2020.1776161
266. Gilra A, and Gerstner W. Predicting non-linear dynamics by stable local learning in a recurrent spiking neural network. eLife. (2017) 6:28295. doi: 10.7554/eLife.28295
267. Y.Ilan I-B, T. A subject-specific system and method for prevention of body adaptation for chronic treatment of disease. (2019). Available online at: Available online at: https://patents.google.com/patent/WO2019008571A1/en (accessed October 5, 2020). WO 2019/008571 Al.
268. FDA Guidances with Digital Health Content. (2020). Available online at: https://www.fda.gov/media/122535/download; https://www.fda.gov/medical-devices/digital-health (accessed October 5, 2020).
269. Verghese A, Shah NH, and Harrington RA. What this computer needs is a physician: humanism and artificial intelligence. JAMA. (2018) 319:19–20. doi: 10.1001/jama.2017.19198
Keywords: precision medicine, artificial intelligence, algorithms, variability, complex systems
Citation: Ilan Y (2020) Second-Generation Digital Health Platforms: Placing the Patient at the Center and Focusing on Clinical Outcomes. Front. Digit. Health 2:569178. doi: 10.3389/fdgth.2020.569178
Received: 03 June 2020; Accepted: 02 October 2020;
Published: 03 December 2020.
Edited by:
Ali Zarrinpar, University of Florida, United StatesReviewed by:
Niamh Lennox-Chhugani, TaoHealth Research, United KingdomTyler John Loftus, University of Florida, United States
Copyright © 2020 Ilan. This is an open-access article distributed under the terms of the Creative Commons Attribution License (CC BY). The use, distribution or reproduction in other forums is permitted, provided the original author(s) and the copyright owner(s) are credited and that the original publication in this journal is cited, in accordance with accepted academic practice. No use, distribution or reproduction is permitted which does not comply with these terms.
*Correspondence: Yaron Ilan, ilan@hadassah.org.il