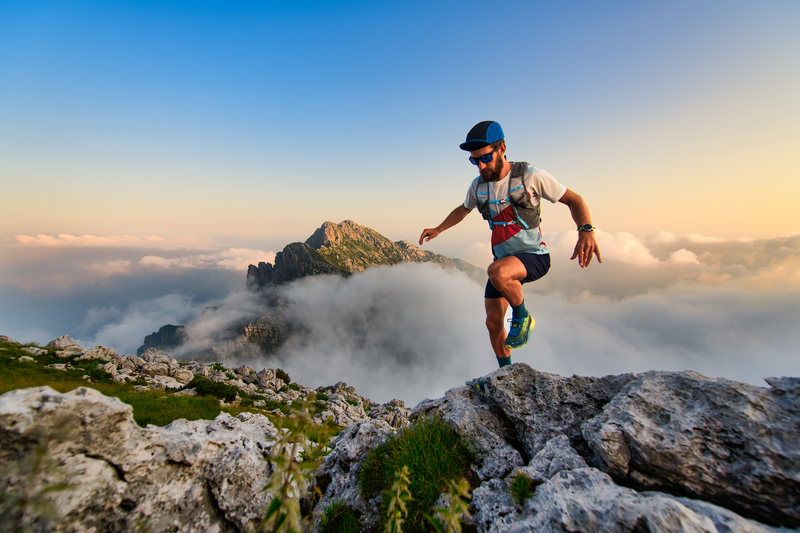
95% of researchers rate our articles as excellent or good
Learn more about the work of our research integrity team to safeguard the quality of each article we publish.
Find out more
ORIGINAL RESEARCH article
Front. Dev. Psychol. , 19 March 2025
Sec. Cognitive Development
Volume 3 - 2025 | https://doi.org/10.3389/fdpys.2025.1526486
This article is part of the Research Topic Children's Teaching View all 3 articles
Social robots are increasingly being designed for use in educational contexts, including in the role of a tutee. However, little is known about how robot behavior affects children's learning-through-teaching. We examined whether the frequency and type of robot mistakes affected children's teaching behaviors (basic and advanced), and subsequent learning, when teaching a social robot. Eight to 11-year-olds (N = 114) taught a novel classification scheme to a humanoid robot. Children taught a robot that either made no mistakes, typical mistakes (errors on untaught material; accuracy on previously taught material), or atypical mistakes (errors on previously taught material; accuracy on untaught material). Following teaching, children's knowledge of the classification scheme was assessed, and they evaluated their own teaching and both their own and the robot's learning. Children generated more teaching strategies when working with one of the robots that made mistakes. While children indicated that the robot that made typical mistakes learned better than the one that made atypical mistakes, children themselves demonstrated the most learning gains if they taught the robot that made atypical mistakes. Children who demonstrated more teaching behaviors showed better learning, but teaching behaviors did not account for the learning advantage of working with the atypical mistake robot.
Acquiring new knowledge is fundamental to children's development. While traditional teaching approaches are effective for the majority of students, many students continue to struggle to meet learning outcomes. For instance, results from an international assessment of achievement indicated that 8% of Canadian students failed to meet the minimum proficiency for mathematics, which is consistent with international statistics (O'Grady et al., 2021). Concerned that the needs of many students are not being met, educators and researchers have explored how technology can be leveraged to create novel, engaging, and impactful learning opportunities. One potential technology is social robots, which have been used with the aim of enhancing children's learning outcomes across educational contexts and academic subject areas (Ahmad et al., 2020; Belpaeme et al., 2018). In addition to guiding and directing children (i.e., as a tutor/teacher), another approach that increases children's motivation is when social robots are programmed to take the role of a “novice” or “teachable robot,” while the child acts as an instructor (Biswas et al., 2016). Thus far, teachers and children have reported cautious optimism in this technological learning approach (Ahmad et al., 2016a,b). However, much remains to be examined about the qualities in the robot and the robot-child interaction that are most effective for producing learning gains.
In the present work, we sought to determine whether, when teaching a robot a novel classification system, a robot's mistake behaviors affect children's teaching strategies, perceptions of teaching and learning, and acquisition of knowledge. This specific robot feature (i.e., mistakes) was selected due to the salience of this cue for children's teaching (i.e., as mistakes reveal gaps between a teacher's knowledge and the tutee's understanding; Ronfard and Corriveau, 2016) and for children's learning (Corriveau et al., 2009; Koenig et al., 2004; Koenig and Harris, 2005a,b). Together, this work increases the understanding of children's sensitivity to, and reactions toward, robot behavior in the context of teaching, which in turn has implications for the design of educational technology (e.g., how to engage students and foster learning).
Within the late preschool years/early school-age years, children understand the nature of teaching, share relevant information with others, and demonstrate skillfulness in their teaching strategies (Qui and Moll, 2022; Qiu et al., 2024b; Strauss et al., 2002). Moreover, teaching others has substantial value: children demonstrate increased learning gains when they are asked to teach others vs. learn for themselves. For example, children acting as peer tutors show greater progression in their own skills relative to children who are taught material (i.e., tutees), likely due to the increased effort children put forth in selecting and organizing learning material in a meaningful way when in the role of a teacher (Duran, 2017). Explaining concepts to someone else facilitates the process of reformulating information and consolidating one's own understanding. Further, children feel more motivated and exert greater effort to learn when having to teach someone else vs. just learning for themselves (i.e., the Protégé effect; Belpaeme et al., 2018). Given the learning benefits, asking students to engage in peer-to-peer teaching is a popular approach within elementary schools (Slavin, 2015).
To capitalize on the advantages of learning-by-teaching, researchers and developers of pedagogical agents have examined if/how children learn from teaching robots. Research on teachable robots has shown promising results, with children reporting enjoyment in, and demonstrating learning from, interactions where they are tasked with teaching a robot a concept or skill. For example, 6-to 8-year-old children's motor skills improved after being asked to teach a robot handwriting (Hood et al., 2015), elementary school children effectively learned scientific concepts after researching information to teach an agent called “Betty's Brain” (Biswas et al., 2016), and 3- to 6-year-old Japanese children who taught a robot learned more English than when learning without the robot (Tanaka and Matsuzoe, 2012). Given these promising findings, researchers have begun examining the different characteristics of social robots that may enhance learning; namely, the physical, behavioral, and conversational features of robots that maximize learning outcomes and motivation (Ceha et al., 2021; Tung, 2016; Woods, 2006). For example, robots perceived as curious induce more behavioral curiosity in undergraduate students in learning contexts (Ceha et al., 2019), with similar effects found for children (Chen et al., 2020). However, while the features of a robot affect users' reported enjoyment of the interaction, short-term learning gains are not always demonstrated (e.g., Ceha et al., 2021; Kennedy et al., 2015; Vogt et al., 2019), and there are even fewer findings on long-term learning gains. As such, it is important to understand the specific teachable agent qualities that relate to the acquisition of knowledge or skills, and, importantly, possible mechanisms supporting knowledge acquisition.
Without the recognition that there is a knowledge gap between the teacher and the learner, there is little reason for teaching to occur. Recognizing and understanding this gap in knowledge allows a child, in the role of a teacher, to appropriately gauge what, and how much, information would be useful to achieve learning goals. In the preschool years, children start to recognize that a knowledge gap between individuals is a key factor in determining who would assume a teacher role (Ziv and Frye, 2004); by 6 years, children understand that an aim of teaching is to expand a learner's knowledge (Sobel and Letourneau, 2016); and, within the early school years, children tailor the complexity of their teaching strategies to the developmental level of their tutee (Qiu et al., 2024a).
One important cue that can be used to understand another's knowledge state is the nature and frequency of their mistakes (Corriveau et al., 2009; Koenig et al., 2004; Koenig and Harris, 2005a). Mistakes are a particularly salient cue for children when on the receiving end of information: preschool and school-age children are more likely to learn from accurate (vs. inaccurate) individuals (Birch et al., 2008) and appreciate the type and magnitude of individuals' errors in their selective learning (Einav and Robinson, 2010; Pasquini et al., 2007). Despite this preference for accurate information sources, mistakes can benefit children's learning, with greater learning gains shown when observing others making errors (Neu and Greer, 2019; Want and Harris, 2001). As such, children demonstrate an ability to detect and learn from the mistakes of others in an observer context.
Relevant for our inquiry, when teaching others, mistakes are an important indicator of knowledge gaps for both the instructor and student, allowing for more tailored teaching. Indeed, Ronfard and Corriveau (2016) found that preschoolers (especially older preschoolers, i.e., 5-year-olds) used the number of mistakes that puppets made during a simple game (i.e., moving the wrong color pieces inside a square) to infer the puppets' understanding and used this information to guide teaching strategies. That is, the older preschoolers showed responsiveness to the puppets' mistakes, providing instructions that were in line with the type of mistakes made. Corriveau et al. (2018) argue that the ability to reason about knowledge from mistakes is a crucial factor in children's ability to engage in teaching, drawing on both children's theory of mind and executive functioning to infer mental states in real-time and implement effective strategies. Thus, in the context of learning-by-teaching, a tutee's mistakes may elicit greater learning by directing attention to relevant knowledge gaps. Moreover, given that young children actively construct ideas about knowledge and ignorance through interactions with human peers (Harris et al., 2017; Ronfard et al., 2018; Ronfard and Harris, 2018), and children extend human qualities to robots (e.g., Beran and Ramirez-Serrano, 2010; Manzi et al., 2020; Okanda et al., 2021), within the context of robot/child interactions, a robot's mistakes may prompt children to seek to conceptualize the “knowledge,” or “mind” (or programming) of a robot.
Within child-robot interactions, children show sensitivity to a robot's accuracy. Preschool-age children express less trust in, and are less likely to learn from, robots that have previously made mistakes (Danovitch and Alzahabi, 2013; Geiskkovitch et al., 2019; Li and Yow, 2023). This sensitivity seems to emerge relatively early: by 5 years old, children are more likely to learn information from a robot that accurately labeled objects than a human informant who made labeling errors (Baumann et al., 2023, 2024). In contrast, in the context of teaching a robot, mistakes may be advantageous for children's learning. Certainly, adults view robots who make mistakes as more likable, though not less intelligent than, a robot who makes no mistakes (Mirnig et al., 2017). Children show increased engagement and positive affect when teaching a robot that makes errors (vs. having a knowledgeable robot tutor; Chen et al., 2020). Importantly, a key feature for successful learning when teaching robots includes a difference in knowledge between the child and the robot (Jamet et al., 2018). Therefore, robot mistakes could enhance awareness of the knowledge gap and thus facilitate learning. Moreover, as with human interactions, robot tutee mistakes may allow children opportunities to benefit from the learning-through-teaching paradigm through active consolidation, reflection, and deeper learning. However, it is unclear whether teachable robot mistakes affect children's learning, and if so, what accounts for this effect. Addressing this gap provides insight into how children infer robot knowledge acquisition through the types of mistakes made, adjust their teaching accordingly, and (potentially) learn during the process of teaching.
In the present work, we assessed whether the presence and type of mistake behavior demonstrated by a robot tutee impacted 8- to 11-year-old children's teaching behaviors and their own learning of the content. This age group was chosen as intrinsic motivation for academics shows a decline between grades 3 and 8, with such motivation relating to academic performance (Lepper et al., 2005), therefore making this a relevant age group to examine learning through alternative means, such as educational technologies. The teaching task that children administered involved a novel classification system, provided to the children on paper, which they then had to teach the robot. A classification task was chosen as children in this age range are typically familiar with classification in general through curricular elements (Ontario Ministry of Education, n.d.). However, we created the specific classification task material (i.e., home locations of aliens; inspired by educational resources used to teach taxonomy, e.g., Biology Junction, 2017; Lasher, 2020; Lyaserror, n.d.; NYLearns, n.d.; The Biology Corner, n.d.) to ensure that all participating children had no prior knowledge of the specific content.
We explored children's teaching, learning, and perceptions of teaching/learning after engaging with a robot that displayed one of three mistake behaviors (i.e., between-subject design): no mistakes, typical mistakes wherein the robot made errors on untaught material, and atypical mistakes wherein the robot made errors on material that was previously taught (or could be inferred through previously taught material). Typical mistakes therefore referred to those expected with learning (i.e., getting something wrong when it has not been taught, and being accurate on previously taught material), whereas atypical mistakes did not follow an expected learning pattern (i.e., being accurate on untaught material, but mistaken on taught material). As the number of mistakes was equivalent between the two mistake robots, any differences would be due to children's sensitivity to the type of mistake.
To determine whether children's teaching strategies varied based on the kind of mistakes the robot made, and, importantly, whether the teaching behavior accounted for possible group differences in their learning, we video-taped children's behavior during the interaction and coded their teaching behavior. Past work shows a developmental progression of teaching strategies from basic to more explanatory (Strauss et al., 2002); thus, we assessed both children's basic (e.g., confirming accuracy or inaccuracy of an answer) and advanced (e.g., educating on classification rules; see Method) teaching strategies. It was anticipated that children would engage in more teaching when the robot demonstrated less knowledge via mistake behavior (Ronfard and Corriveau, 2016) and that those who showed a greater number of teaching behaviors, and in particular more advanced teaching strategies (e.g., using elaboration on content vs. merely feedback on accuracy), would show greater learning gains (Fiorella and Mayer, 2013).
For children's learning, we hypothesized that teaching a robot that demonstrated mistakes (vs. interacting with a robot who made no mistakes) would be advantageous to children's learning in that it would create more reason for them to attend to the material (e.g., through correcting misunderstanding, Chen et al., 2020). In terms of whether there was an advantage of the type of mistake (typical vs. atypical) for learning, past work suggests reasonable hypotheses for either direction. On the one hand, the typical mistakes may allow for children to understand the learning style of the robot and thus facilitate a more rewarding and less frustrating experience (as the behavior is more humanlike; Breazeal et al., 2016). On the other hand, past work has shown that robot behaviors that deviate from expectations creates engagement, which is in line with learning theories that suggest novel or unexpected outcomes gain more attention (Lemaignan et al., 2015). Thus, children who teach a robot that makes atypical (vs. typical) mistakes may show increased engagement, and thus, increased learning. Given these discrepant rationales, we did not have a clear hypothesis for children's learning outcomes.
Finally, we were interested in assessing children's perceptions of the robot's learning, as well as their own learning and teaching. By including these self-ratings, we were able to evaluate multiple areas of interest: First, this allowed us to assess whether children detected differences in the robot's behavior, in particular whether children were indeed sensitive to the different types of robot mistakes. Children show sensitivity to the magnitude of mistakes (Einav and Robinson, 2010; Pasquini et al., 2007) and take into account whether a mistake was understandable or not (Kondrad and Jaswal, 2012), thus, we anticipated that children would differentiate type of mistakes made by a robot tutee. Second, we were able to compare children's perceptions of themselves and their behavior. We did not have specific hypotheses, but undertook these analyses as they provide information about the level of insight children show into their own teaching and learning. With respect to perceptions of teaching, children's understanding of teaching develops during the preschool-years (Ziv and Frye, 2004), and their ability to distinguish effective from ineffective teaching occurs by 5-years-old (Ziv et al., 2008). As they get older, they are able to identify qualities of effective teaching in more specific terms (Beishuizen et al., 2001; Bullock, 2015; Kutnick and Jules, 1993; Thomas and Montomery, 1998). However, less is known about the degree to which they show insight into their own teaching behaviors and their teaching efficacy, and whether this differs by tutee behavior. In terms of learning, understanding one's own knowledge base is an important aspect of learning (Fisher, 1998; Kuhn, 2021): through the act of teaching a robot, children's insight into their own learning may be more or less obvious to them, with this potentially varying by if/how the robot makes mistakes.
Children aged 8 to 11 years-old were recruited from a laboratory database of families interested in participating in research, as well as research flyers distributed throughout the community. The initial sample consisted of 124 children from a midsize Canadian city. Ten children's data were excluded from analyses due to: experimenter error (e.g., robot script entered incorrectly, n = 8), or the participant not understanding the teaching task, which was administered in English (i.e., due to language barrier, n = 2). The final sample consisted of 114 children (45 girls, Mage = 8.77 years, SDage = 0.88 years). Parents' responses to an open-ended question about their children's ethnicity were grouped in categories (according to guidelines for bias-free language; APA Style, n.d.): White/European (n = 61), South Asian/South Asian Canadian (n = 28), East Asian/East Asian Canadian (n = 18), Middle Eastern (Iran/Lebanon/Kurdish, n = 7), Latinx/Hispanic (n = 4), Black (n = 1), Indigenous Peoples (n = 1), and 5 parents did not provide information (numbers add to more than 114 due to some participants providing multiple ethnoracial backgrounds). Most parents (92%) had a college degree or higher. There were no exclusion criteria for participation (outside of age) to reflect variability within a community sample.
Children in the three robot groups, Correct (n = 37), Typical Mistakes (n = 39), and Atypical Mistakes (n = 38), did not differ by age [F(2, 111) = 0.79, p = 0.456], verbal skills [F(2, 107) = 0.56, p = 0.573], parent-reported executive functioning [F(2, 104) = 1.65, p = 0.20], theory of mind [F(2, 108) = 1.59, p = 0.21),1 or gender [ = 0.93, p = 0.630].
Following parental consent and child assent, children participated in a 1 hr individual session with a researcher and robot. Children completed measures of socio-cognitive abilities and then engaged in a teaching task with a humanoid robot (NAO; SoftBank Robotics), named “Beta” (Figure 1). Utilizing a Wizard of Oz approach, Beta's communication was controlled by a researcher on the other side of a one-way mirror using NAOqi OS (Aldebaran Robotics, n.d.). Beta's responses were scripted to maintain standardization. To orient the child to interacting with a robot, Beta initially asked the child questions about their name, their favorite color, what they ate for breakfast, and their grade in school, with scripted responses from Beta.
To introduce the teaching task, children were told that researchers at the university wanted to know how robots learn, and that Beta needs to learn about aliens who live on two different planets, each with different countries, provinces, and cities. They were then told that the aliens that live in each place look different from each other and that the participant's job was to use the classification map (provided to participants so that they had the information, see Figure 2) to teach Beta. Next, children were provided with a card with a picture of an alien (Figure 3), with a question on the back to ask the robot (e.g., “Beta, what planet/country/province/city is this alien from?”). Beta's responses (regardless of condition) followed the same general format: Beta indicated that they noticed the key features of the alien (e.g., “I see this alien is red and has arms”) and then provided a location (e.g., “I think this means the alien is from the country Chatt”). The accuracy of this latter phrase varied by the robot mistake condition: in the Correct condition, Beta always provided an accurate answer; in the Typical Mistake condition, Beta made an error if this was the first question asked at that level (i.e., planet/country/province/city), but provided the correct answer if this was the second question at that level. That is to say, Beta was inaccurate on new material, but accurate on material that would be known based on the previous question; for example, if Beta previously incorrectly answered that a blue alien was from Blorb, they could infer that it is actually the case that a red alien is from Blorb. In the Atypical Mistake condition, Beta provided a correct answer if it was the first question at that level (i.e., new material), but provided an incorrect answer when the second question was at the same level; for example, if Beta previously correctly indicated that a blue alien was from Colla, they did not use this information to infer that the red alien was from Blorb. Importantly, the number of mistakes made by the Typical Mistake robot and the Atypical Mistake robot were the same; the difference was in the positioning of the mistakes relative to the presented content.
Figure 3. Examples of alien cards given to participants to teach robot. Alien illustrations by Bernadette Ho-Sum Cheng.
There were 20 alien cards (i.e., 20 opportunities for children to teach Beta about that specific alien classification), with these presented in a manner that ensured there was no sequential patterning in Beta's mistakes (e.g., alternating correct/incorrect). Following each level of the classification system (e.g., planet, country, province, or city) the researcher indicated that that level was complete, put the alien cards from that level on the table, and asked if the child would like to let Beta know anything more about that level before moving to the next one. There were four of these transition points, which meant that in total there were 24 opportunities for children to teach Beta. Following Beta's response (trials) or after being asked if they would like to provide any more information (transitions), the researcher allowed time for the child to teach Beta. The child was allowed to teach however they wished until they indicated they were done or stopped teaching for 3 seconds. If 3 seconds passed without the child doing/saying anything (or said they had no further instruction), the researcher proceeded with the next trial.
Children's teaching behavior following Beta's responses at each classification level, as well as between levels, was video recorded and behaviorally coded. To examine whether the between-subject robot conditions elicited different amounts of teaching behaviors that were more/less elaborative (and whether teaching related learning), children's teaching behaviors were tabulated as basic or advanced teaching strategies. Basic teaching behaviors were those behaviors that allowed for a child to determine and communicate whether the robot was accurate or not, but without any further elaboration—i.e., referencing the answer key map (i.e., checking if the robot answered correctly), giving verbal feedback (e.g., saying “Yes/No” to the robot to indicate accuracy), and giving non-verbal feedback (e.g., nodding yes/no to the robot to indicate accuracy). Advanced teaching behaviors were those that included elaboration beyond just providing the robot with information about their accuracy: i.e., providing the correct answer to the robot (e.g., “This alien is actually from planet Colla, not planet Blorb”) or providing the classification rule from the answer key to Beta (e.g., “This alien is blue, so that means it is from planet Colla). Children were given credit for each specific teaching act once (e.g., if they said “No, no, no, that's not right” they were given credit for providing feedback, thus one basic teaching strategy; if they said, “No, this one is from Colla because aliens from Colla are blue.” they would receive credit for providing feedback), providing an answer and providing an explanation, equating to one basic and two advanced strategies. Thus, at each teaching opportunity (i.e., during a trial or transition between levels), a child received a score of the number of basic teaching strategies and the number of advanced teaching strategies. These scores were averaged across the teaching opportunities (i.e., during trials and transitions), providing us with each child's average number of basic and advanced teaching strategies within a teaching opportunity.
A primary coder, who was unaware of study hypotheses, coded all children's teaching behaviors. To ensure reliability, a second coder, who was also unaware of hypotheses, coded 25 participants (22% of the data). Interrater reliability for basic teaching (ICC = 0.905) behaviors and advanced teaching (ICC = 0.957) behaviors were found to be strong. The majority of children always demonstrated some sort of teaching behavior when provided the opportunity: across robot conditions, 87% of children demonstrated some form of teaching after the robot provided a correct answer, 92% always taught after the robot made a mistake, and 68% always did some form of teaching during the transition points.
Following the teaching task, a large blank diagram of the different alien locations was put on the table and the classification key that participants used during teaching was removed. The researcher provided the child with eight alien cards and instructed them to put them in the correct location. There were two cards at each level of the classification system (planet/country/province/city). Children's performance on this task was used as the measure of their learning of the classification system (/8).
Children were asked to respond to questions about their perceptions, including how good they were at teaching, how much Beta learned, and how much they learned about alien geography from teaching Beta, using a Likert scale that ranged from 1 (none at all) to 5 (a great deal).
All data were analyzed for missing values. For children's perception ratings, there were missing data for Beta's learning (n = 2), their own teaching (n = 3), and their own learning (n = 2). Given the low numbers of missing data (<2% of perception data), list wise deletion was used. Five children did not have data for teaching behaviors as they did not assent to video recording.
No outliers were detected in the questionnaire data and skew and kurtosis were within acceptable limits (|skew| ≤ 3; |kurtosis| ≤ 10; Kline, 1998). Univariate outliers on the basic (1 outlier) and advanced (1 outlier) teaching composites were winsorized to be ±3SD. No multivariate outliers were detected for participant's coded data and skew and kurtosis were also within acceptable limits.
A mixed ANOVA was performed to compare the impact of robot mistakes on the average number of children's teaching behaviors within each teaching opportunity with robot type (Correct, Typical Mistake, Atypical Mistake) as the between-subject factor and type of teaching (basic, advanced) as the within-subject factor (Figure 4). There were significant main effects of robot type [F(2, 106) = 17.62, = 0.25, p < 0.001], which were followed up with comparisons using Games-Howell (as per a significant Levene's test of homogeneity of variance). Comparisons revealed that, when collapsing across teaching type, children who taught a Correct robot (M = 0.66, SE = 0.031; total teaching per opportunity = 1.31) demonstrated fewer behaviors compared to the Typical (M = 0.89, SE = 0.029; total teaching per opportunity = 1.78), Mdiff = −0.24, SEdiff = 0.04, p < 0.001, 95% CI: [−0.34 to −0.14] and Atypical robot (M = 0.86, SE = 0.030; total teaching per opportunity = 1.72), Mdiff = −0.21, SEdiff = 0.04, p < 0.001, 95% CI [−0.31, −0.10]. Children in the two mistake robot conditions (i.e., Atypical vs. Typical) did not differ in their number of teaching behaviors (collapsed across type), p = 0.729. The ANOVA also revealed a main effect of type of teaching [F(1, 106) = 998.74, = 0.90, p < 0.001], with children demonstrating more basic teaching behaviors (M = 1.35, SE = 0.03) than advanced teaching behaviors (M = 0.26, SE = 0.02), but no interaction between robot condition and teaching type (p = 0.466).
A one-way ANOVA was performed to compare the impact of robot mistakes on children's learning, with robot type (Correct, Typical Mistake, Atypical Mistake) as the independent variable and Post-Teaching Test Performance (number of cards classified correctly from/8) as the dependent variable. There was a significant difference in test performance by condition, F(2, 111) = 6.83, η2 = 0.11, p = 0.002. Follow-up independent t-tests revealed that children in the Atypical Mistakes (M = 5.87, SD = 1.96) condition demonstrated greater learning compared to children in the Typical Mistakes [M = 4.69, SD = 1.61 t(75) = 2.88, d = 0.66, p = 0.005] or Correct conditions [M = 4.43, SD = 1.82, t(73) = 3.29, d = 0.76, p = 0.002]. No significant difference was observed between the Typical Mistakes and Correct condition, t(74) = 0.66, p = 0.511. These results remained statistically significant when a Bonferroni correction was applied for multiple comparisons, p = 0.017 (0.05/3 comparisons).
We were interested in understanding how children's teaching behavior related to their learning. As shown in Table 1, across all conditions children whose average demonstration of teaching behaviors (both basic and advanced) demonstrated better learning of the classification system. Interestingly, within the Correct and Typical conditions (but not Atypical) advanced teaching related to learning. While the pattern of data did not suggest that the learning advantage found for children in the Atypical condition is driven by children's teaching behavior, to examine this premise directly, PROCESS mediation analysis (Hayes, 2022) with 5,000 bootstrapped sample estimates was conducted. The predictor was a dummy code for the Atypical Condition (relative to other conditions). Children's average number of basic and advanced teaching behaviors were entered as the mediators. The dependent variable was children's learning (test performance). The model did not support an indirect effect, but did demonstrate main effects of both the Atypical condition and advanced (but not basic) teaching on children's test performance (Figure 5).
Table 1. Bivariate correlations between children's average number of teaching behaviors, learning, and perceptions.
Figure 5. Associations of atypical mistake condition and teaching behaviors on children's learning. Results for Advanced teaching in black and for Basic teaching in gray.
Robot tutee mistakes (typical and atypical) elicit more teaching behaviors (basic and advanced), and children who showed more advanced teaching behaviors demonstrated the most learning gains. However, children who taught an atypical mistakes robot showed the most learning gains, with this effect not explained by children's teaching.
A MANOVA compared the impact of robot mistakes (i.e., robot condition) on children's perceptions of Beta's and their own learning/teaching. The MANOVA revealed a marginally significant effect of robot condition on children's perceptions of Beta's learning, F(2, 108) = 2.95, η2 = 0.05, p = 0.056. Follow-up comparisons using Games-Howell, revealed a significant difference between the Atypical Mistakes condition (M = 3.43, SE = 0.15) and Typical Mistakes condition (M = 3.92, SE = 0.15), Mdiff = −0.49, SEdiff = 0.18, p = 0.019, 95% CI [−0.91, −0.07]. Comparisons between mistake conditions and the Correct condition (M = 3.80, SE = 0.15) were not significant, ps > 0.27. Thus, showing sensitivity to the mistake patterns, children who taught the Typical mistakes robot perceived their robot learned more than those who taught the Atypical robot. There was not a significant difference between robot conditions in children's perceptions of their own learning (p = 0.497), with their average ratings across the conditions being 3.69 (SD = 0.81) on a 5-point scale. As well, there was not an effect of robot condition on children's perceptions of their own teaching (p = 0.607), with an average rating of 3.74 (SD = 0.89) on a 5-point scale. Thus, children's perceptions of their own learning and teaching were not influenced by the type of robot they taught. They generally felt they learned “A Lot” about alien geography through teaching Beta and were “A Lot” good at teaching Beta during the teaching task.
To understand how much insight children had into their own teaching and learning, correlations were conducted between children's perceptions and teaching behaviors across conditions and separately by robot condition (Table 1). In terms of teaching behaviors, children's self-assessment of their teaching did not relate to their teaching behaviors. In contrast, children showed some insight into their learning, with those children who rated their learning higher also performing better on the classification test across conditions. However, when examined separately, it was primarily the children in the Correct condition whose self-assessment of learning related to their actual learning. Using Fisher's z-test, the strength of this correlation was significantly different from that for children in the Atypical Mistakes condition (p = 0.02) and marginally different from those in the Typical Mistakes condition (p = 0.08).
This project aimed to examine children's learning through teaching a social robot, with particular interest in whether a robot's mistake behavior affected children's teaching behaviors, children's learning, and children's appraisals of their own teaching and learning.
Addressing our first research question, namely, whether the robot mistake behavior elicited different teaching behaviors from the children, we examined whether the average observations of basic and advanced teaching behaviors differed by robot condition. We found that children who taught a robot that made no mistakes produced fewer teaching behaviors generally than those children who taught a robot that made mistakes (typical or atypical). Thus, consistent with work demonstrating that by late preschool years, children infer knowledge from mistakes and teach accordingly (Ronfard and Corriveau, 2016), we found that tutee mistakes elicit more teaching behaviors when children work with humanoid robots. As predicted, across the conditions, children who generated more teaching behaviors showed better learning of the classification system. Looking closer at correlation patterns within conditions, children who engaged in more explanatory or elaborate teaching strategies (i.e., advanced teaching) learned the content for themselves better. This finding is consistent with peer tutoring (Roscoe and Chi, 2008), wherein tutors who provide explanations (vs. just preparing to explain) show a deeper understanding of the material (Fiorella and Mayer, 2013). However, importantly, this was only found when children worked with either a robot that made no errors or with a robot that made typical mistakes. That is, children who taught a robot that did not follow a typical learning pattern (Atypical condition) did not show an association between the degree to which they generated advanced teaching strategies and their learning outcomes, potentially because teaching becomes disconnected from learning when the tutee learning pattern is atypical.
These associations are interesting to consider in light of the group effects found in children's learning (as per our second research question): children learned more when teaching a robot that made atypical mistakes (relative to when a robot either made no mistake or made typical mistakes). Moreover, mediation analyses suggested that while advanced teaching was important for children's learning, it did not account for the learning advantages of working with an Atypical robot. That is, there was no indirect effect. Thus, while teaching (in particular, advanced teaching) was important for children's learning, factors other than teaching behavior are needed to explain the learning benefit of working with a robot that makes atypical mistakes.
We speculate that the unexpected and novel nature of the atypical robot's learning behavior may have elicited curiosity (Ceha et al., 2019) and prompted children to more closely attend to the content and/or engage more fully in figuring out and remembering the classification system, thereby enhancing learning. Such an explanation is in keeping with the notion that violations of expectations promote children's learning of new information (Stahl and Feigenson, 2015, 2017, 2019). In the present work, it may be that because the atypical robot was behaving in a way that violated children's expectations for what learning ‘should' look like (both in terms of their mistakes and their accuracy), children engaged in enhanced attention toward the teaching material. Children's perceptions of robot learning support our notion that children were indeed sensitive to the specific type of mistake behavior of the robot: when Beta followed a typical learning pattern (mistakes first, then accurate responses) relative to an atypical learning pattern (accuracy first, then mistake) they indicated that Beta learned more. Thus, extending past work showing children's sensitivity to the relative accuracy of informants (Pasquini et al., 2007), we show that children can (impressively) use the specific placement of mistakes (relative to presented material) to make determinations about others' (in this case, a robot's) acquisition of knowledge. Further, when teaching a robot that demonstrates unexpected mistake behavior children show enhanced learning.
Given the importance of children's ability to assess their own knowledge in relation to learning (Fisher, 1998; Kuhn, 2021), our third research question was whether the type of robot mistake behavior affects children's perception of their own learning and teaching. We did not find that children's rating of their own teaching or learning varied by condition. Thus, even though, as a group, children demonstrated more teaching behaviors when teaching robots that made mistakes and learned more with an atypical robot, their perceptions did not mirror these effects (in general, children rating themselves as fairly strong in terms of their teaching/learning across all three conditions). We sought to further explore their perceptions at an individual level; namely, assessing whether their self-ratings of learning and teaching matched their behavior (i.e., test performance, teaching behaviors). Across conditions, children's rating of their own learning was related to their test performance. However, within conditions, this was only the case when children taught a robot that never made mistakes. Thus, while robot mistakes may assist with learning (particularly if atypical), it may make it difficult for children to have a sense of their own learning, potentially because they are more focused on the robot's acquisition of knowledge rather than their own and/or because children's learning happens in a more subtle or unconscious way. In no robot condition did children's perception of their teaching relate to the frequency of their teaching behavior, suggesting that within our teaching context children did not have a good sense as to how well they were teaching. This finding is perhaps not that surprising as children likely used various cues to determine teaching success, including both what they did as well as how Beta responded, with these each providing different messages. For instance, in the Correct condition, they could do very little teaching but still perceive their teaching to be decent given that the robot tutee was performing well.
While this work presents novel findings, there are limitations to consider. First, the interaction between the child and Beta was somewhat limited as per our use of a predetermined script. Future work may use voice detection software and/or large language models to generate a greater variety of responses provided by the social robot, which may also allow for a more qualitative inquiry (e.g., Lee et al., 2021). Second, because this was a between-subject (vs. within-subject) design, we were not able to assess how children shift their teaching behaviors according to robot tutee behavior (including before/after mistakes), as well as limiting sensitivity in detecting differences in perceptions. Third, our age range was relatively small, and future work with a wider range would be useful to understand the developmental course as to when children show sensitivity to robot mistakes and adjust behaviors accordingly, with a recent meta-analysis on selective teaching suggesting there may be shifts around the age of 4 (Qiu et al., 2024a). Finally, we did not conduct a parallel study with human tutees to determine whether the pattern of findings regarding children's learning-by-teaching is comparable with human vs. robot tutees, as well how responses to robot tutee mistakes compare to responses to human tutee mistakes. This was not an initial objective for the study, but such a comparison would be useful to address questions about how educational technologies may offer benefit beyond traditional approaches. Indeed, recent work suggests that each context (i.e., robot tutee vs. child tutee) offers different learning advantages/limitations (Pareto et al., 2022). As well, conceptually speaking, a comparison with a human tutee would allow for determining whether children hold similar sensitivity/expectations for the “learning” style of robots as they do for humans. It is possible that with human tutees, atypical mistakes create even more of a violation of expectations such that learning is further enhanced. Alternatively, children may experience frustration when teaching a human tutee vs. a robot tutee because the atypical learning pattern. Finally, it would also be useful for future work to examine whether children's individual characteristics play a role in how they respond to different robot behaviors in the context of teaching (e.g., theory of mind; Davis-Unger and Carlson, 2008; Strauss et al., 2002).
In the context of teaching robots a novel classification system, the presence of robot tutee mistakes (regardless of type) elicited more teaching behaviors by children. Moreover, those children who demonstrated more advanced teaching behaviors in particular showed better learning of the classification system. Children who taught a robot that made mistakes that did not follow a typical learning pattern showed the greatest learning gains of a novel classification system, but this was not explained through their teaching behavior. Together, our work extends the developmental literature demonstrating children's sensitivity to types of inaccuracy, including from robots (Baumann et al., 2023), within a teaching context (Ronfard and Corriveau, 2016). This work is novel, generating preliminary findings which provide a foundation from which further discovery can be based, including how children conceptualize the “mind” of robot tutees through a robot's behavior, and adjust their own teaching behavior accordingly, as well as what type of tutee behavior works best for which children within learning-by-teaching paradigms. Addressing these questions are crucial for designing pedagogical agents, particularly for those children who may struggle with traditional teaching methods.
The raw data supporting the conclusions of this article will be made available by the authors, without undue reservation.
The studies involving humans were approved by the University of Waterloo Research Ethics Board. The studies were conducted in accordance with the local legislation and institutional requirements. Written informed consent for participation in this study was provided by the participants' legal guardians/next of kin.
CB-S: Conceptualization, Data curation, Formal analysis, Investigation, Methodology, Project administration, Writing – original draft, Writing – review & editing. CA: Data curation, Investigation, Methodology, Project administration, Writing – review & editing. TM: Investigation, Writing – review & editing. EL: Conceptualization, Funding acquisition, Resources, Writing – review & editing. EN: Conceptualization, Data curation, Formal analysis, Funding acquisition, Investigation, Methodology, Project administration, Resources, Supervision, Writing – original draft, Writing – review & editing.
The author(s) declare that financial support was received for the research and/or publication of this article. This work was funded through a Social Sciences and Humanities Research Council (SSHRC) Insight Development Grant (430-2023-00288) awarded to EN and EL and a Robert Harding Humanities Social Sciences Endowment awarded through the University of Waterloo.
We would like to thank Elaria Ebeid for her assistance with data collection and Navya Roby and Barbara Ledezma for their assistance with behavioral coding. Thank you to Bernadette Ho-Sum Cheng for the alien illustrations and Kexin Yang and Apoorva Chauhan for contributions to early discussions of the project. We are grateful to the families who dedicated their time to participate in this research.
The authors declare that the research was conducted in the absence of any commercial or financial relationships that could be construed as a potential conflict of interest.
The author(s) declare that no Gen AI was used in the creation of this manuscript.
All claims expressed in this article are solely those of the authors and do not necessarily represent those of their affiliated organizations, or those of the publisher, the editors and the reviewers. Any product that may be evaluated in this article, or claim that may be made by its manufacturer, is not guaranteed or endorsed by the publisher.
1. ^Verbal skill was assessed by the NIH Toolbox vocabulary measure (Slotkin et al., 2012), ToM was assessed through videos depicting second-order false belief stories from Coull et al. (2006), and executive functioning was assessed through the Childhood Executive Functioning Inventory (CHEXI; Thorell and Nyberg, 2008).
Ahmad, M. I., Khordi-moodi, M., and Lohan, K. S. (2020). Social Robot for STEM Education. Cambridge, UK: Association for Computing Machinery Digital Library. doi: 10.1145/3371382.3378291
Ahmad, M. I., Mubin, O., and Orlando, J. (2016a). “Children's views on social robot's adaptations in education,” in The 28th Australian Conference on Human-Computer Interaction (HCI) (Launceston, Australia). doi: 10.1145/3010915.3010977
Ahmad, M. I., Mubin, O., and Orlando, J. (2016b). “Understanding behaviors and roles for social and adaptive robots in education: teacher's perspective,” in The 4th Annual International Conference on Human-Agent Interaction (Biopolis, Singapore). doi: 10.1145/2974804.2974829
Aldebaran Robotics. (n.d.). Key concepts - Aldebaran 2.1.4.13 documentation. Available on: http://doc.aldebaran.com/2-1/dev/naoqi/index.html#distributed (accessed January 6, 2025).
APA Style (n.d.). Racial and Ethnic Identity. Available on: https://apastyle.apa.org/style-grammar-guidelines/bias-free-language/racial-ethnic-minorities (accessed January 23, 2025).
Baumann, A. E., Goldman, E. J., Cobos, M. M., and Poulin-Dubois, D. (2024). Do preschoolers trust a competent robot pointer? J. Exp. Child Psychol. 238:105783. doi: 10.1016/j.jecp.2023.105783
Baumann, A. E., Goldman, E. J., Meltzer, A., and Poulin-Dubois, D. (2023). People do not always know best: preschoolers' trust in social robots. J. Cogn. Dev. 24, 535–562. doi: 10.1080/15248372.2023.2178435
Beishuizen, J. J., Hof, E., van Putten, C. M., Bouwmeester, S., and Asscher, J. J. (2001). Students' and teachers' cognitions about good teachers. Br. J. Educ. Psychol. 71, 185–201. doi: 10.1348/000709901158451
Belpaeme, T., Kennedy, J., Ramachandran, A., Scassellati, B., and Tanaka, F. (2018). Social robots for education: a review. Sci. Robot. 3, 1–9. doi: 10.1126/scirobotics.aat5954
Beran, T., and Ramirez-Serrano, A. (2010). “Do children perceive robots as alive? Children's attributions of human characteristics,” in 2010 5th ACM/IEEE International Conference on Human-Robot Interaction (HRI) (IEEE), 137–138. doi: 10.1109/HRI.2010.5453226
Biology Junction (2017). Alien taxonomy project. Available on: https://biologyjunction.com/alien-taxonomy-project/ (accessed January 6, 2025).
Birch, S. A., Vauthier, S. A., and Bloom, P. (2008). Three-and four-year-olds spontaneously use others' past performance to guide their learning. Cognition 107, 1018–1034. doi: 10.1016/j.cognition.2007.12.008
Biswas, G., Segedy, J. R., and Bunchongchit, K. (2016). From design to implementation to practice a learning by teaching system: Betty's Brain. Int. J. Artif. Intell. Educ. 26, 350–364. doi: 10.1007/s40593-015-0057-9
Breazeal, C., Harris, P. L., DeSteno, D., Kory Westlund, J. M., Dickens, L., and Jeong, S. (2016). Young children treat robots as informants. Top. Cogn. Sci. 8, 481–491. doi: 10.1111/tops.12192
Bullock, M. (2015). What makes a good teacher? Exploring student and teacher beliefs on good teaching. Rising Tides 7, 1–30. doi: 10.13189/ujer.2017.050118
Ceha, J., Chhibber, N., Goh, J., McDonald, C., Oudeyer, P. Y., Kulić, D., et al. (2019). “Expression of curiosity in social robots: design, perception, and effects on behaviour,” in Proceedings of the 2019 CHI Conference on Human Factors in Computing Systems, 1–12. doi: 10.1145/3290605.3300636
Ceha, J., Lee, K. J., Nilsen, E., Goh, J., and Law, E. (2021). “Can a humorous conversational agent enhance learning experience and outcomes?” in The CHI Conference on Human Factors in Computing Systems (CHI'21) (Yokohama, Japan). doi: 10.1145/3411764.3445068
Chen, H., Park, H. W., and Breazeal, C. (2020). Teaching and learning with children: impact of reciprocal peer learning with a social robot on children's learning and emotive engagement. Comput. Educ. 150:103836. doi: 10.1016/j.compedu.2020.103836
Corriveau, K. H., Meints, K., and Harris, P. L. (2009). Early tracking of informant accuracy and inaccuracy. Br. J. Dev. Psychol. 27, 331–342. doi: 10.1348/026151008X310229
Corriveau, K. H., Ronfard, S., and Cui, Y. K. (2018). Cognitive mechanisms associated with children's selective teaching. Rev. Philos. Psychol. 9, 831–848. doi: 10.1007/s13164-017-0343-6
Coull, G. J., Leekam, S. R., and Bennett, M. (2006). Simplifying second-order belief attribution: What facilitates children's performance on measures of conceptual understanding? Soc. Dev. 15, 548–563. doi: 10.1111/j.1467-9507.2006.00340.x
Danovitch, J., and Alzahabi, R. (2013). Children show selective trust in technological informants. J. Cogn. Dev. 14, 499–513. doi: 10.1080/15248372.2012.689391
Davis-Unger, A. C., and Carlson, S. M. (2008). Development of teaching skills and relations to theory of mind in preschoolers. J. Cogn. Dev. 9, 26–45. doi: 10.1080/15248370701836584
Duran, D. (2017). Learning-by-teaching. Evidence and implications as a pedagogical mechanism. Innov. Educ. Teach. Int. 54, 476–484. doi: 10.1080/14703297.2016.1156011
Einav, S., and Robinson, E. J. (2010). Children's sensitivity to error magnitude when evaluating informants. Cogn. Dev. 25, 218–232. doi: 10.1016/j.cogdev.2010.04.002
Fiorella, L., and Mayer, R. E. (2013). The relative benefits of learning by teaching and teaching expectancy. Contemp. Educ. Psychol. 38, 281–288. doi: 10.1016/j.cedpsych.2013.06.001
Fisher, R. (1998). Thinking about thinking: developing metacognition in children. Early Child Dev. Care 141, 1–15. doi: 10.1080/0300443981410101
Geiskkovitch, D., Thiessen, R., Young, J., and Glenwright, M. (2019). “What? That's not a chair! How robot informational errors affect children's trust towards robots,” in The 14th ACM/IEEE International Conference on Human-Robot Interaction (Daegu, Korea). doi: 10.1109/HRI.2019.8673024
Harris, P. L., Ronfard, S., and Bartz, D. (2017). Young children's developing conception of knowledge and ignorance: work in progress. Eur. J. Dev. Psychol. 14, 221–232. doi: 10.1080/17405629.2016.1190267
Hayes, A. F. (2022). Introduction to Mediation, Moderation, and Conditional Process Analysis: A Regression-Based Approach (3rd edition). New York: Guildford Press.
Hood, D., Lemaignan, S., and Dillenbourg, P. (2015). “When children teach a robot to write: an autonomous teachable humanoid which uses simulated handwriting,” in Proceedings of the Tenth Annual ACM/IEEE International Conference on Human-Robot Interaction, 83–90. doi: 10.1145/2696454.2696479
Jamet, F., Masson, O., Jacquet, B., Stilgenbauer, J. L., and Baratgin, J. (2018). Learning by teaching with humanoid robot: a new powerful experimental tool to improve children's learning ability. J. Robot. 2018:4578762. doi: 10.1155/2018/4578762
Kennedy, J., Baxter, P., and Belpaeme, T. (2015). Comparing robot embodiments in a guided discovery learning interaction with children. Int. J. Soc. Robot. 7, 293–308. doi: 10.1007/s12369-014-0277-4
Kline, R. B. (1998). Methodology in the Social Sciences. Principles and Practice of Structural Equation Modeling. New York: Guilford Press.
Koenig, M. A., Clément, F., and Harris, P. L. (2004). Trust in testimony: children's use of true and false statements. Psychol. Sci. 15, 694–698. doi: 10.1111/j.0956-7976.2004.00742.x
Koenig, M. A., and Harris, P. L. (2005a). Preschoolers mistrust ignorant and inaccurate speakers. Child Dev. 76, 1261–1277. doi: 10.1111/j.1467-8624.2005.00849.x
Koenig, M. A., and Harris, P. L. (2005b). The role of social cognition in early trust. Trends Cogn. Sci. 9, 457–459. doi: 10.1016/j.tics.2005.08.006
Kondrad, R. L., and Jaswal, V. K. (2012). Explaining the errors away: young children forgive understandable semantic mistakes. Cogn. Dev. 27, 126–135. doi: 10.1016/j.cogdev.2011.11.001
Kuhn, D. (2021). Metacognition matters in many ways. Educ. Psychol. 57, 73–86. doi: 10.1080/00461520.2021.1988603
Kutnick, P., and Jules, V. (1993). Pupils' perceptions of a good teacher: a developmental perspective from Trinidad and Tobago. Br. J. Educ. Psychol. 63, 400–413 doi: 10.1111/j.2044-8279.1993.tb01067.x
Lasher, D. (2020). Alien animal taxonomy: binomial nomenclature for kids. Big Ideas for Little Scholars. Available on: https://bigideas4littlescholars.com/alien-animal-taxonomy-binomial-nomenclature-for-kids/ (accessed January 6, 2025).
Lee, K. J., Chauhan, A., Goh, J., Nilsen, E., and Law, E. (2021). “Curiosity Notebook: The design of a research platform for learning by teaching,” in Proceedings of the ACM Human-Computer Interaction 5, CSCW (October 23-27, 2021), 1–26. doi: 10.1145/3479538
Lemaignan, S., Fink, J., Mondada, F., and Dillenbourg, P. (2015). You're doing it wrong! Studying unexpected behaviors in child-robot interaction. Soc. Robot. 9388, 390–400. doi: 10.1007/978-3-319-25554-5_39
Lepper, M. R., Corpus, J. H., and Iyengar, S. S. (2005). Intrinsic and extrinsic motivational orientations in the classroom: age differences and academic correlates. J. Educ. Psychol. 97, 184–196. doi: 10.1037/0022-0663.97.2.184
Li, X., and Yow, W. Q. (2023). Younger, not older, children trust an inaccurate human informant more than an inaccurate robot informant. Child Dev. 95, 988–1000. doi: 10.1111/cdev.14048
Lyas, J. (n.d.). Alien Taxonomy–A Classification Cart Sort Activity. TPT. Available on: https://www.teacherspayteachers.com/Product/Alien-Taxonomy-A-Classification-Card-Sort-Activity-3021759 (accessed January 6, 2025).
Manzi, F., Peretti, G., Di Dio, C., Cangelosi, A., Itakura, S., Kanda, T., et al. (2020). A robot is not worth another: exploring children's mental state attribution to different humanoid robots. Front. Psychol. 11:2011. doi: 10.3389/fpsyg.2020.02011
Mirnig, N., Stollnberger, G., Miksch, M., Stadler, S., Giuliani, M., and Tscheligi, M. (2017). To err is robot: how humans assess and act toward an erroneous social robot. Front. Robot. AI 4:21. doi: 10.3389/frobt.2017.00021
Neu, J. A., and Greer, R. D. (2019). Fifth graders learn math by observation faster when they observe peers receive corrections. Eur. J. Behav. Anal. 20, 126–145. doi: 10.1080/15021149.2019.1620044
NYLearns (n.d.). Curriculum Management and Standards-based System. Classification of Aliens by ECSDM. Available on: https://www.nylearns.org/module/content/search/item/5580/viewdetail.ashx#sthash.nBe1hZD0.dpbs (accessed January 6, 2025).
O'Grady, K., Rostamian, A., Monk, J., Tao, Y., Scerbina, T., and Elez, V. (2021). TIMSS 2019 Canadian results from the Trends in International Mathematics and Science Study. Canada: Council of Ministers of Education.
Okanda, M., Taniguchi, K., Wang, Y., and Itakura, S. (2021). Preschoolers' and adults' animism tendencies toward a humanoid robot. Comput. Human Behav. 118:106688. doi: 10.1016/j.chb.2021.106688
Ontario Ministry of Education (n.d.). 2022 Elementary science and technology curriculum: An overview. Government of Ontario. Available on: https://www.dcp.edu.gov.on.ca/en/science-tech-overview/learning-by-grade#g2
Pareto, L., Ekström, S., and Serholt, S. (2022). Children's learning-by-teaching with a social robot versus a younger child: comparing interactions and tutoring styles. Front. Robot. AI 9:875704. doi: 10.3389/frobt.2022.875704
Pasquini, E. S., Corriveau, K. H., Koenig, M., and Harris, P. L. (2007). Preschoolers monitor the relative accuracy of informants. Dev. Psychol. 43, 1216–1226. doi: 10.1037/0012-1649.43.5.1216
Qiu, F. W., Ipek, C., Gottesman, E., and Moll, H. (2024a). Know thy audience: Children teach basic or complex facts depending on the learner's maturity. Child Dev. 95, 1406–1415. doi: 10.1111/cdev.14077
Qiu, F. W., Park, J., Vite, A., Patall, E., and Moll, H. (2024b). Children's selective teaching and informing: a meta-analysis. Dev. Sci. 28:e13576. doi: 10.1111/desc.13576
Qui, F. W., and Moll, H. (2022). Children's pedagogical competence and child-to-child knowledge transmission: forgotten factors in theories of cultural evolution. J. Cogn. Cult. 22, 421–435. doi: 10.1163/15685373-12340143
Ronfard, S., Bartz, D. T., Chen, L., Chen, X., and Harris, P. (2018). Chapter Four–Children's developing ideas about knowledge and its acquisition. Adv. Child Dev. Behav. 54, 123–151. doi: 10.1016/bs.acdb.2017.10.005
Ronfard, S., and Corriveau, K. H. (2016). Teaching and preschoolers' ability to infer knowledge from mistakes. J. Exp. Child Psychol. 150, 87–98. doi: 10.1016/j.jecp.2016.05.006
Ronfard, S., and Harris, P. L. (2018). Children's decision to transmit information is guided by their evaluation of the nature of that information. Rev. Philos. Psychol. 9, 849–861. doi: 10.1007/s13164-017-0344-5
Roscoe, R. D., and Chi, M. T. H. (2008). Tutor learning. The role of explaining and responding to questions. Instruct. Sci. 36, 321–350. doi: 10.1007/s11251-007-9034-5
Slavin, R. E. (2015). Cooperative learning in elementary schools. Education 43, 3–13. doi: 10.1080/03004279.2015.963370
Slotkin, J., Kallen, M., Griffith, J., Magasi, S., Salsman, J., and Nowinski, C. (2012). NIH toolbox. Technical Manual.
Sobel, D. M., and Letourneau, S. M. (2016). Children's developing knowledge of and reflection about teaching. J. Exp. Child Psychol. 143, 111–122. doi: 10.1016/j.jecp.2015.10.009
Stahl, A. E., and Feigenson, L. (2015). Cognitive development. Observing the unexpected enhances infants' learning and exploration. Science 348, 91–94. doi: 10.1126/science.aaa3799
Stahl, A. E., and Feigenson, L. (2017). Expectancy violations promote learning in young children. Cognition 163, 1–14. doi: 10.1016/j.cognition.2017.02.008
Stahl, A. E., and Feigenson, L. (2019). Violations of core knowledge shape early learning. Top. Cogn. Sci. 11, 136–153. doi: 10.1111/tops.12389
Strauss, S., Ziv, M., and Stein, A. (2002). Teaching as a natural cognition and its relations to preschoolers' developing theory of mind. Cogn. Dev. 17, 1473–1487. doi: 10.1016/S0885-2014(02)00128-4
Tanaka, F., and Matsuzoe, S. (2012). Children teach a care-receiving robot to promote their learning: field experiments in a classroom for vocabulary learning. J. Hum.-Robot Interact. 1, 78–95. doi: 10.5898/JHRI.1.1.Tanaka
The Biology Corner. (n.d.). Taxonomy project–Design a system for aliens. Available on: https://www.biologycorner.com/worksheets/taxonomyproject.html
Thomas, J. A., and Montomery, P. (1998). On becoming a good teacher: reflective practice with regard to children's voices. J. Teach. Educ. 49, 372–380. doi: 10.1177/0022487198049005007
Thorell, L. B., and Nyberg, L. (2008). The Childhood Executive Functioning Inventory (CHEXI): A new rating instrument for parents and teachers. Dev. Neuropsychol. 33, 536–552. doi: 10.1080/87565640802101516
Tung, F. W. (2016). Child perception of humanoid robot appearance and behavior. Int. J. Hum. Comput. Interact. 32, 493–502. doi: 10.1080/10447318.2016.1172808
Vogt, P., van den Berghe, R., De Haas, M., Hoffman, L., Kanero, J., Mamus, E., et al. (2019). “Second language tutoring using social robots: a large-scale study,” in 2019 14th ACM/IEEE International Conference on Human-Robot Interaction (HRI) (IEEE), 497–505. doi: 10.1109/HRI.2019.8673077
Want, S. C., and Harris, P. L. (2001). Learning from other people's mistakes: causal understanding in learning to use a tool. Child Dev. 72, 431–443. doi: 10.1111/1467-8624.00288
Woods, S. (2006). Exploring the design space of robots: children's perspectives. Interact. Comput. 18, 1390–1418. doi: 10.1016/j.intcom.2006.05.001
Ziv, M., and Frye, D. (2004). Children's understanding of teaching: the role of knowledge and belief. Cogn. Dev. 19, 457–477. doi: 10.1016/j.cogdev.2004.09.002
Keywords: children, learning, social robots, teaching, mistakes, educational technology
Citation: Bowman-Smith CK, Aitken C, Mahenthiran T, Law E and Nilsen ES (2025) Teaching social robots: the effect of robot mistakes on children's learning-through-teaching. Front. Dev. Psychol. 3:1526486. doi: 10.3389/fdpys.2025.1526486
Received: 11 November 2024; Accepted: 19 February 2025;
Published: 19 March 2025.
Edited by:
Henrike Moll, University of Southern California, United StatesReviewed by:
Justin W. Bonny, Morgan State University, United StatesCopyright © 2025 Bowman-Smith, Aitken, Mahenthiran, Law and Nilsen. This is an open-access article distributed under the terms of the Creative Commons Attribution License (CC BY). The use, distribution or reproduction in other forums is permitted, provided the original author(s) and the copyright owner(s) are credited and that the original publication in this journal is cited, in accordance with accepted academic practice. No use, distribution or reproduction is permitted which does not comply with these terms.
*Correspondence: Elizabeth S. Nilsen, ZW5pbHNlbkB1d2F0ZXJsb28uY2E=
Disclaimer: All claims expressed in this article are solely those of the authors and do not necessarily represent those of their affiliated organizations, or those of the publisher, the editors and the reviewers. Any product that may be evaluated in this article or claim that may be made by its manufacturer is not guaranteed or endorsed by the publisher.
Research integrity at Frontiers
Learn more about the work of our research integrity team to safeguard the quality of each article we publish.