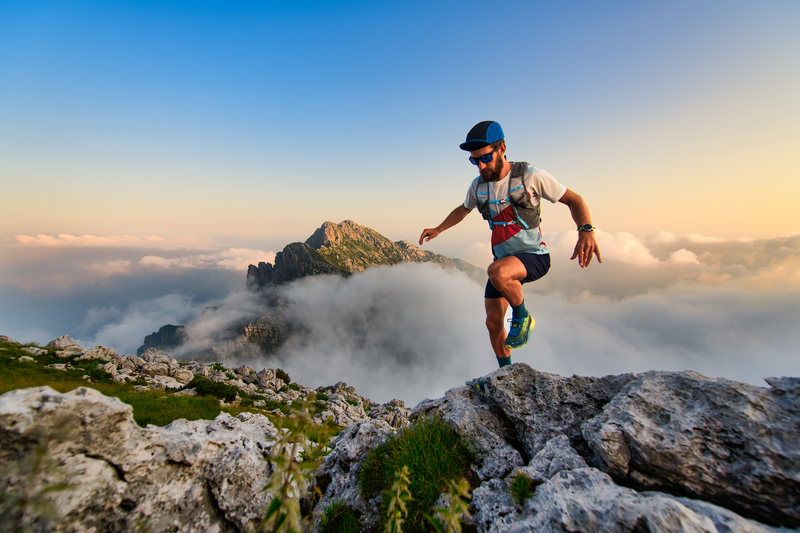
94% of researchers rate our articles as excellent or good
Learn more about the work of our research integrity team to safeguard the quality of each article we publish.
Find out more
EDITORIAL article
Front. Comput. Sci.
Sec. Computer Vision
Volume 7 - 2025 | doi: 10.3389/fcomp.2025.1597931
This article is part of the Research Topic Geometries of Learning View all 13 articles
The final, formatted version of the article will be published soon.
You have multiple emails registered with Frontiers:
Please enter your email address:
If you already have an account, please login
You don't have a Frontiers account ? You can register here
Despite the widespread application and empirical success of deep neural networks, the theoretical 10 development of these networks remains an under-explored area. The lack of theory prevents rigorous 11 explanation and prediction of networks' performance and hinders confident deployment of the 12 networks especially in safety-critical applications. Geometric analysis provides a unique perspective 13 to bridge this gap between the practice and the theory of deep neural networks. As complex real-14 world data often lie on low-dimensional manifolds, geometries of these underlying structures provide 15 informative insights for understanding the behavior of networks fitting to the data. 16In this Research Topic of Frontiers in Computer Science on Geometries of Learning, we aim to 17 present the latest advances on inspecting deep learning through the lens of geometry. We have 18 followed a rigorous peer review process and collected twelve articles that showcase the depth and 19 breadth of current approaches across applications of geometric analysis across diverse problem 20 domains from adversarial defense to object pose estimation. A summary of the collection is 21 introduced below. 22In the paper titled "Leveraging linear mapping for model-agnostic adversarial defense" Jamil et al. serve the core of recent developments in language modeling, promoting geometric and topological 97 interpretation of learning with direct links to semantics, and expanding the study for a wide range of 98 applications involving diverse data manifolds at real-world complexities. 99The guest editor team would like to extend our sincere gratitude to all the authors, reviewers and the 100 editorial team for their valuable contribution to this Research Topic. Our special thanks go to Dr. 101Bruce Draper for his vision and leadership in the research and discussions that laid the foundation of 102 this Research Topic as well as his guidance and support throughout the editing process. 103
Keywords: Geometries of Learning, Manifold Learning, deep neural networks, adversarial learning, topological data analysis
Received: 21 Mar 2025; Accepted: 26 Mar 2025.
Copyright: © 2025 Gong, Turaga, Fletcher and Divakaran. This is an open-access article distributed under the terms of the Creative Commons Attribution License (CC BY). The use, distribution or reproduction in other forums is permitted, provided the original author(s) or licensor are credited and that the original publication in this journal is cited, in accordance with accepted academic practice. No use, distribution or reproduction is permitted which does not comply with these terms.
* Correspondence:
Yunye Gong, SRI International, Menlo Park, United States
Disclaimer: All claims expressed in this article are solely those of the authors and do not necessarily represent those of their affiliated organizations, or those of the publisher, the editors and the reviewers. Any product that may be evaluated in this article or claim that may be made by its manufacturer is not guaranteed or endorsed by the publisher.
Research integrity at Frontiers
Learn more about the work of our research integrity team to safeguard the quality of each article we publish.