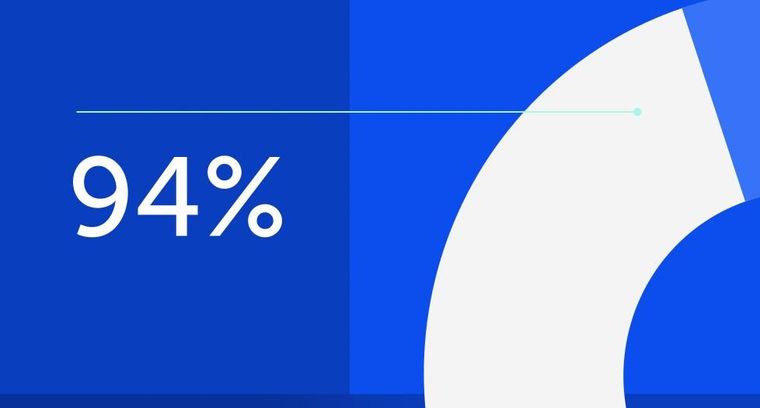
94% of researchers rate our articles as excellent or good
Learn more about the work of our research integrity team to safeguard the quality of each article we publish.
Find out more
EDITORIAL article
Front. Comput. Sci., 10 April 2025
Sec. Computer Vision
Volume 7 - 2025 | https://doi.org/10.3389/fcomp.2025.1585443
This article is part of the Research TopicComputer Vision and AI in Real-world Applications: Robustness, Generalization, and EngineeringView all 8 articles
Editorial on the Research Topic
Computer vision and AI in real-world applications: robustness, generalization, and engineering
Much progress has been made in computer vision and artificial intelligence over the last few years, with some techniques staying at the forefront and used in diverse domains and real scenarios.
This Research Topic collection aims to provide a broad view and an in-depth analysis of fields, such as image compression, learning from observations, crowd behavior classification, disease detection, animal species labeling, and semantic segmentation.
A description of the seven contributions will follow with hyperlinks to the full-content versions for readers interested in more in-depth analysis.
The first study, “Concurrent compression and meaningful encryption of images using chaotic compressive sensing,” proposes an optimisation method for image compression and encryption that relies on a chaotic sensing approach. Images are transformed into sparse representations using DCT (Discrete Cosine Transform) (Ashwini et al.). A measurement matrix is set with a chaotic map to run a compression step.
The input image embeds the compressed information by integrating the high-frequency elements that allow for a visually interpretable encrypted output.
The second collection study, “Applying learning-from-observation to household service robots: three task common-sense formulations,” delves into robotics tasks focusing on cluttered household environments (Ikeuchi et al.).
Common-sense, semi-conscious, and human movements are the three types of tasks analyzed to convey a method driving robots to be focused on what and where of their actions. Labanotation is used to deal with body movements' descriptions, contact webs to represent hand–finger interactions with tools, and physical and semantic constraints to check out dynamics between hands, tools, and environments.
RL (Reinforcement Learning) trains a skill-agent, allowing robots to run tasks by imitating human demonstrations and extending their adaptability to handle complex household tasks and environments.
Crowd is the keyword representing the contribution titled “A novel multi-scale violence and public gathering dataset for crowd behavior classification” (Elzein et al.). The article introduces a new dataset for crowd behavior analysis and surveillance, which consists of events categorized on two aspects: crowd size and level of perceived violence.
The aspects mentioned allow for the samples to be further grouped into the following three subsets: peaceful gatherings, violent incidents, and natural crowd movements. Preprocessing techniques are needed to make the dataset suitable for machine learning models.
Furthermore, the dataset undergoes an event-detection analysis through deep learning models that demonstrate their ability to increase automated event detection accuracy rates.
From a higher standpoint, the contribution lies within the broad AI-driven surveillance topic and enriches it by providing a new technique for more effective monitoring of public spaces.
Detection is among the most featured keywords in AI and ML articles due to the excellent inference capabilities proven by several techniques and approaches. The contribution, “OSPS-MicroNet: a distilled knowledge micro-CNN network for detecting rice diseases,” stands out as a lightweight deep learning approach to identifying rice plant diseases (Tharani Pavithra and Baranidharan).
OSPS-MicroNet is conceived to find a tradeoff between detection accuracy rates and computational resource constraints. The proposed approach relies on CNNs in a limited-resource setting. A teacher–student learning framework is used to work around the given challenge.
The knowledge is transferred from a more sophisticated and bigger-sized “teacher” model to the lighter-weighted OSPS-MicroNet “student” model.
Data labeling is a well-known challenge in AI and Computer Vision. The article “Automatic labeling of fish species using deep learning across different classification strategies” addresses the labeling of a dataset collecting images from 19 fish species (Jareño et al.).
Pretrained CNNs represent the basis upon which stacking supervised classification layers. In greater detail, transfer learning specifies pre-trained CNNs on features from the given dataset. Afterward, Support Vector Machines (SVMs) and Linear Discriminant Anaysis (LDA) are used as final classificators.
The article “Deep learning-based classification of eye diseases using Convolutional Neural Network for OCT images” lays out a method to detect retinal disease from OCT (Optical Coherence Tomography) images (Elkholy and Marzouk). A preprocessing step based on Gaussian Blur Filtering is carried out before running OCT classification. A Convolutional Neural Network (CNN) runs OCT image classification into four categories: normal retina, Diabetic Macular Edema (DME), Choroidal Neovascular Membranes (CNM), and Age-related Macular Degeneration (AMD).
The article titled “EfficientNet family U-Net models for deep learning semantic segmentation of kidney tumors on CT images” presents a novel biomedical image segmentation method (Abdelrahman and Viriri).
Kidney cancer segmentation from CT (Computed Tomography) scans is approached by combining U-Net and EfficientNet. The latter is opted for due to its capabilities in image details extraction. The features detected by EfficientNet are paramount in accurately detecting the region of interest contours. Integrating the two architectures (U-Net and EfficientNet) delivers a more accurate segmentation of the kidney regions and the corresponding suspicious areas. The method is tested over KiTS19, a dataset of CT scans.
In summary, the collection of Research Topics hosts seven contributions presenting methods and techniques in optimisation, image classification, labeling, deep learning, and interdisciplinary applications. Compelling scenarios arise from the latest development of large multimodal language models (LMLs), which will probably lead to ripple effects on computer vision and AI applications that might pave the way for new case studies and techniques to be developed and tested.
AB: Writing – original draft, Writing – review & editing. PM: Writing – original draft, Writing – review & editing. NS: Writing – review & editing. BH: Writing – review & editing. MG: Writing – review & editing.
The authors declare that the research was conducted in the absence of any commercial or financial relationships that could be construed as a potential conflict of interest.
All claims expressed in this article are solely those of the authors and do not necessarily represent those of their affiliated organizations, or those of the publisher, the editors and the reviewers. Any product that may be evaluated in this article, or claim that may be made by its manufacturer, is not guaranteed or endorsed by the publisher.
Keywords: computer vision, theoretical advances, application scenarios, artificial intelligence, image processing
Citation: Bruno A, Mazzeo PL, Strisciuglio N, Hammer B and Gao M (2025) Editorial: Computer vision and AI in real-world applications: robustness, generalization, and engineering. Front. Comput. Sci. 7:1585443. doi: 10.3389/fcomp.2025.1585443
Received: 28 February 2025; Accepted: 26 March 2025;
Published: 10 April 2025.
Edited and reviewed by: Marcello Pelillo, Ca' Foscari University of Venice, Italy
Copyright © 2025 Bruno, Mazzeo, Strisciuglio, Hammer and Gao. This is an open-access article distributed under the terms of the Creative Commons Attribution License (CC BY). The use, distribution or reproduction in other forums is permitted, provided the original author(s) and the copyright owner(s) are credited and that the original publication in this journal is cited, in accordance with accepted academic practice. No use, distribution or reproduction is permitted which does not comply with these terms.
*Correspondence: Alessandro Bruno, YWxlc3NhbmRyby5icnVub0BpdWxtLml0
Disclaimer: All claims expressed in this article are solely those of the authors and do not necessarily represent those of their affiliated organizations, or those of the publisher, the editors and the reviewers. Any product that may be evaluated in this article or claim that may be made by its manufacturer is not guaranteed or endorsed by the publisher.
Research integrity at Frontiers
Learn more about the work of our research integrity team to safeguard the quality of each article we publish.