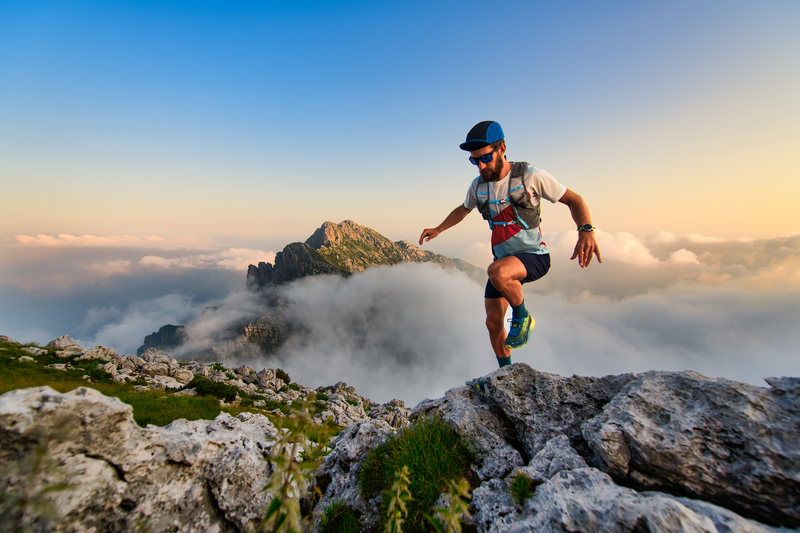
94% of researchers rate our articles as excellent or good
Learn more about the work of our research integrity team to safeguard the quality of each article we publish.
Find out more
ORIGINAL RESEARCH article
Front. Comput. Sci.
Sec. Computer Security
Volume 7 - 2025 | doi: 10.3389/fcomp.2025.1574211
The final, formatted version of the article will be published soon.
You have multiple emails registered with Frontiers:
Please enter your email address:
If you already have an account, please login
You don't have a Frontiers account ? You can register here
Background: Network intrusion detection is a critical component of maintaining network security, especially as cyber threats become increasingly sophisticated. While deep learningbased intrusion detection algorithms have shown promise, they often struggle with highdimensional datasets containing outliers, anomalies, or rare events. This study addresses these challenges by proposing a novel approach that combines the Improved Gravitational Search Algorithm (IGSA) with the Soft Actor-Critic (SAC) reinforcement learning algorithm, aiming to enhance detection accuracy and computational efficiency. Methods: We introduce the IGSA-SAC intrusion detection model, which leverages an enhanced Gravitational Search Algorithm (IGSA) to improve robustness against outliers and dynamically adjust the explorationexploitation balance. This is achieved through fitness normalization with an Adaptive Search Radius and a sigmoid function to modulate the gravitational constant. The IGSA-SAC method effectively navigates the search space to identify the most relevant features for intrusion detection, reducing dimensionality and computational complexity. Additionally, we design a reinforcement learning reward function to guide the learning process, encouraging the agent to improve detection effectiveness while minimizing false alarms and missed detections. Results: Experiments were conducted on the NSL-KDD and AWID datasets to evaluate the performance of IGSA-SAC. The results demonstrate that IGSA-SAC achieves an accuracy of 84.15% and an F1-score of 84.85% on the NSL-KDD dataset. On the AWID dataset, IGSA-SAC surpasses 98.9% in both accuracy and F1-score, outperforming existing intrusion detection algorithms. Conclusions: The proposed IGSA-SAC method significantly improves intrusion detection performance by effectively handling high-dimensional datasets and reducing computational complexity. The results highlight the potential of IGSA-SAC as a robust and efficient solution for real-world network intrusion detection systems, offering enhanced accuracy and reliability in identifying cyber threats.
Keywords: intrusion detection, Feature Selection, gravitational search algorithm, soft ActorCritic, Reinforcement learning algorithm
Received: 14 Feb 2025; Accepted: 17 Mar 2025.
Copyright: © 2025 Jin, Fan, Han and Cui. This is an open-access article distributed under the terms of the Creative Commons Attribution License (CC BY). The use, distribution or reproduction in other forums is permitted, provided the original author(s) or licensor are credited and that the original publication in this journal is cited, in accordance with accepted academic practice. No use, distribution or reproduction is permitted which does not comply with these terms.
* Correspondence:
Lizhong Jin, Taiyuan University of Science and Technology, Taiyuan, China
Disclaimer: All claims expressed in this article are solely those of the authors and do not necessarily represent those of their affiliated organizations, or those of the publisher, the editors and the reviewers. Any product that may be evaluated in this article or claim that may be made by its manufacturer is not guaranteed or endorsed by the publisher.
Research integrity at Frontiers
Learn more about the work of our research integrity team to safeguard the quality of each article we publish.