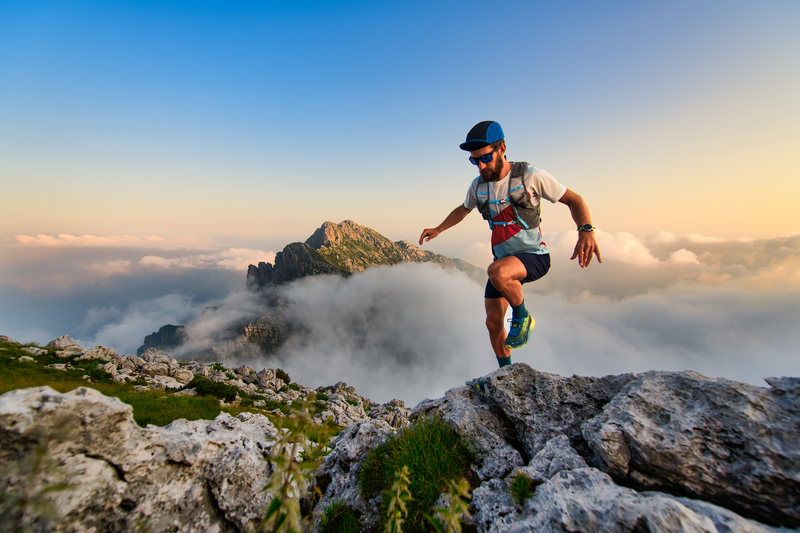
95% of researchers rate our articles as excellent or good
Learn more about the work of our research integrity team to safeguard the quality of each article we publish.
Find out more
ORIGINAL RESEARCH article
Front. Comput. Sci.
Sec. Networks and Communications
Volume 7 - 2025 | doi: 10.3389/fcomp.2025.1563942
This article is part of the Research Topic Deep Learning for Industrial Applications View all 5 articles
The final, formatted version of the article will be published soon.
You have multiple emails registered with Frontiers:
Please enter your email address:
If you already have an account, please login
You don't have a Frontiers account ? You can register here
In recent years, Deep Learning (DL) and Artificial Neural Networks (ANNs) have transformed industrial applications by providing automation in complex tasks such as anomaly detection and predictive maintenance. However, traditional DL models often need significant computational resources, making them unsuitable for resource-constrained edge devices. This paper explores the potential of sparse ANNs to address these challenges, focusing on their application in industrial settings. We perform an experimental comparison of pruning techniques, including the Pre-Training, In-Training, Post-Training, and SET method, applied to the VGG16 and ResNet18 architectures, and conduct a systematic analysis of pruning methodologies alongside the effects of varying sparsity levels, to analyze their efficiency in anomaly detection and object classification tasks. Key metrics such as training accuracy, inference time, and energy consumption are analyzed to assess the feasibility of deploying sparse models on edge devices.Our results demonstrate that sparse ANNs, particularly when pruned using the SET method, achieve energy savings without compromising accuracy, making them suitable for industrial applications. This work highlights the potential of sparse neural networks to boost sustainability and efficiency in industrial environments, paving the way for their large adoption in edge computing scenarios.
Keywords: sparse neural networks, Pruning techniques, Industrial applications, energy efficiency, Edge Devices -4 Linear ResNet-18 Outputs Feature Extraction Classification
Received: 20 Jan 2025; Accepted: 31 Mar 2025.
Copyright: © 2025 Douzandeh Zenoozi, Liotta, Erhan and Cavallaro. This is an open-access article distributed under the terms of the Creative Commons Attribution License (CC BY). The use, distribution or reproduction in other forums is permitted, provided the original author(s) or licensor are credited and that the original publication in this journal is cited, in accordance with accepted academic practice. No use, distribution or reproduction is permitted which does not comply with these terms.
* Correspondence:
Antonio Liotta, Free University of Bozen-Bolzano, Bolzano, Italy
Disclaimer: All claims expressed in this article are solely those of the authors and do not necessarily represent those of their affiliated organizations, or those of the publisher, the editors and the reviewers. Any product that may be evaluated in this article or claim that may be made by its manufacturer is not guaranteed or endorsed by the publisher.
Research integrity at Frontiers
Learn more about the work of our research integrity team to safeguard the quality of each article we publish.