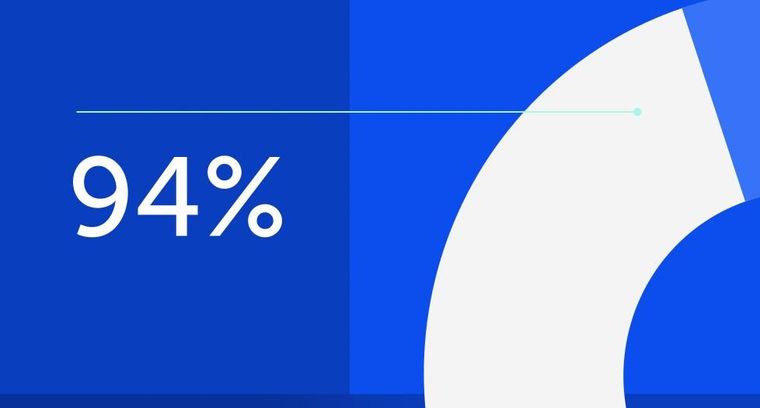
94% of researchers rate our articles as excellent or good
Learn more about the work of our research integrity team to safeguard the quality of each article we publish.
Find out more
ORIGINAL RESEARCH article
Front. Comput. Sci., 10 April 2025
Sec. Networks and Communications
Volume 7 - 2025 | https://doi.org/10.3389/fcomp.2025.1550677
Introduction: The accurate and timely diagnosis of skin diseases is a critical concern, as many skin diseases exhibit similar symptoms in the early stages. Most existing automated detection/classification approaches that utilize machine learning or deep learning poses privacy issues, as they involve centralized computing and require local storage for data training.
Methods: Keeping the privacy of sensitive patient data as a primary objective, in addition to ensuring accuracy and efficiency, this paper presents an algorithm that integrates Federated learning techniques into an IoT-based edge-computing environment. The purpose of the proposed technique is to protect the sensitive data by training the model locally on the edge device and transferring only the weights to the central server where the aggregation takes place. This process ensures data security at the edge level and eliminates the need for centralized storage. Furthermore, the proposed framework enhances the network’s real-time processing capabilities using IoT-integrated sensors, which in turn facilitates swift diagnoses. In addition, this paper also focuses on the design and execution of the federated framework, which includes the processing power, memory, and the number of nodes present in the network.
Results: The accuracy and effectiveness of the proposed algorithm are demonstrated using precise parameters, such as accuracy, precision, f1-score, and recall, along with all the intricacies of the secure federated approach. The accuracy achieved by the proposed algorithm is 98.6%. As the model was trained locally, the bandwidth utilization was almost negligible.
Discussion: The proposed model can assist skin specialists in diagnosing conditions. Additionally, with federated learning, the model continuously improves as new input data accumulates, enhancing the accuracy of subsequent training rounds.
They span a broad variety of demographic and geographic regions. These diseases are very common, making accurate assessment challenging, which is crucial for developing successful treatment and management programs (Li et al., 2021). Skin diseases range from common conditions, such as dermatitis and acne to complex conditions including melanoma and autoimmune disorders. Skin conditions not only cause physical discomfort but also affect a person’s psychological health and overall quality of life (Ahmad et al., 2020). Accurate and timely diagnostic techniques are essential as these disorders can manifest in various ways and degrees of severity. Statistics show that about one-fifth of Americans may develop skin cancer at some point in their life (Lim et al., 2017). Skin cancer is the most common type of cancer in the United States (Toğaçar et al., 2021), with melanoma having the highest mortality rate of any skin cancer, at 1.62% (Jowett and Ryan, 1985). According to American Cancer Society, there will be around 100, 350 new instances of melanoma in the US in 2020, resulting in 6,850 deaths (Hay et al., 2014). However, the most common type of skin cancer is the basal cell carcinoma (BCC). Although it is usually not fatal, it imposes a heavy burden on medical resources (Fried et al., 2005). The five-year survival rate for skin cancer may increase by approximately 14% with early detection and treatment (Fleischer et al., 2000).
Traditional approaches for the categorization and diagnosis of skin disorders have included visual inspections, manual exams by dermatologists, and, in certain cases, invasive procedures such as biopsies. Two fundamental challenges presented by these systems are the subjectivity in visual judgments and the potential for delays in receiving findings, particularly when relying on expert consultations (Ginsburg, 1996). While these approaches have proven to be somewhat effective, they also come with drawbacks. Furthermore, the increasing prevalence of skin conditions, combined with a global shortage of dermatologists, has spurred research into more efficient and advanced diagnostic techniques. It is quite challenging to diagnose a skin disease accurately because it involves several visual cues, such as the appearance, size distribution, color, scale, and arrangement of lesions (Karimkhani et al., 2017). The four most commonly used clinical diagnostic methods for Melanoma include the ABCD principles, pattern analysis, Menzies method, and 7-Point Checklist (Thanh et al., 2020). These methods require skilled medical professionals to make a reliable diagnosis (Diepgen, 2003). The practical accuracy ranges from 0.75 to 0.84 when an inexperienced practitioner tries to diagnose melanoma using dermoscopy pictures (Seth et al., 2017). A drawback of using human specialists for diagnosis is that they usually rely on subjective evaluations, which causes considerable differences across the experts (English et al., 2003; Ruiz et al., 2011). While these approaches often prioritize certain categories, they may not be adaptable enough to provide a comprehensive diagnosis across a range of skin disorders (Allugunti, 2022). Handcrafted features are less adaptable to various dermatological diseases because of their limited design (Srinivasu et al., 2021; Cai et al., 2023). A possible solution to this is feature learning, which automatically extracts useful features and eliminates the requirement of human feature engineering (Elston, 2020). In this context, many feature learning algorithms have been introduced in recent years (Siddique et al., 2024; Razmjooy et al., 2020), however most of them were designed with processing dermoscopy or histopathology pictures in mind, namely mitosis detection as a sign of malignancy (Goceri, 2021).
In this sense, a new era in the identification of skin disorders and associated medical issues has been brought about by the development of methods using deep learning (DL), machine learning (ML) (Dildar et al., 2021). These technologies utilize massive datasets to develop algorithms that can recognize patterns and anomalies that indicate various skin diseases (Siddique et al., 2023).
It is projected that the use of AI and machine learning in dermatology will increase the accessibility, precision, and speed of skin disease detection (Nahata and Singh, 2020). However, these technologies pose serious concerns about the privacy of the sensitive patient data being utilized to train the models (Pacheco and Krohling, 2020). Medical imaging data contains sensitive patient information, which should be protected and comply with ethical and legal standards such as GDPR and HIPAA (Vidya and Karki, 2020). Centralized learning algorithms require data to be trained on the single location can heighten the risk of data breaches. Federated learning offers a promising solution by enabling collaborative model training without the need to share raw data (Monika et al., 2020; Zghal and Derbel, 2020). Through this process, the dataset for the training model never leaves the device and the training process takes place locally on the device. Federated learning is incorporated with an IoT-enabled environment to share their trained weights with the cloud or central server where it aggregates all the model weights and ultimately generates the global model that is both secure and accurate as required for the proposed work. IoT-enabled edge devices, connected within the network, are used to gather real-time data, allowing the system to utilize the algorithm and analyze it in real time (Tabrizchi et al., 2023).
In section 5 of this paper, the process of implementing federated learning integrated with IoT-enabled procedures is discussed in detail. In addition, the consideration of diverse edge devices with varying storage and processing capabilities are discussed. The proposed model is able to adapt to the increasing edge devices with minimum latency and if the number of nodes increases in the network, the aggregated model generated will be more accurate as it will have more diverse model weights trained on various nodes. It provides a resilient and robust solution to provide security for the dataset and at the same time train the model using the localized trained weights.
In Figure 1, the integrated IoT key enabling technologies are illustrated, showcasing the intricate framework employed in this research. In this framework, cloud computing plays a vital role by utilizing shared computing resources for model aggregation, ultimately obtaining a comprehensive global model.
Several articles can be found in recent literature that focus on ML/AI-based skin disease diagnosis, for instance, an auto encoder-based DL model consisting of spiking and convolutional neural networks is proposed in Bhatt et al. (2023), which works as a clinical assistance tool (Bhatt et al., 2023). A publicly available ISIC skin cancer dataset has been utilized. It contains 1,497 photographs of malignant tumors and 1,800 images of benign tumors. To diagnose skin cancer, the standard biopsy method is used, which is a procedure that is not only time-consuming but also expensive. Similarly, a complete examination of a variety of cutting-edge machine-learning approaches that are used for the diagnosis of skin cancer is presented by Thurnhofer-Hemsi and Domínguez (2021). Following the compilation of a number of studies, an inquiry into the efficacy of the k-nearest neighbors, support vector machine, and convolutional neural network approaches on benchmark datasets is carried out at the conclusion of the research. Besides, Kumar et al. (2020) present a deep learning method for skin cancer diagnosis. In order to construct a basic and a hierarchical (with two layers) classifier that is capable of distinguishing between seven distinct types of moles, transfer learning is used in five convolutional neural networks that are considered to be state-of-the-art. For testing purposes, the HAM10000 dataset, which is a massive collection of dermatoscopic images, is employed. Additionally, data augmentation techniques have been applied to improve the efficiency of investigations. In addition, an enhanced method is presented by Nawaz et al. (2022) to detect skin melanoma. The authors utilise region-based convolutional neural networks (RCNN) in conjunction with fuzzy k-means (FKM) and tested the proposed model on clinical images. Furthermore, an ensemble CNN approach combining Shifted GoogleNet and MobileNetV2 for skin lesion classification is proposed in Thurnhofer-Hemsi et al. (2021).
Han et al. utilize a deep convolutional neural network to classify 12 distinct skin disorders using a clinical dataset and the maximum reported accuracy is between 96 and 97%. In spite of the fact that this research does not have the capacity to conduct a full analysis of classifiers, a comprehensive analysis of explainable deep learning classifiers can be found in Barata et al. (2021).
The data acquired, can be vague and possess subpar characteristics, leading to a trained model that does not produce outputs with high accuracy and low error rates. This problem can be addressed through data augmentation. Data augmentation can improve image quality or increase the size of the dataset, facilitating more effective model training (Mondal et al., 2020). If the acquired dataset is not appropriate for training, it is necessary for it to pass through some pre-processing algorithms, this makes the dataset more comprehensive with added features that may facilitate the training procedure. The dataset used in this research is available on Kaggle named ISIC (Razzak et al., 2020). An illustration of a selection of image samples that were retrieved from the dataset is shown in Figure 2. In addition to the ISIC dataset, other publically available datasets such as HAM1000 and DermNet were also reviewed and some samples were selected from them to make the acquired dataset more diverse with different ethnicities and age groups. Synthetic data generation was employed using state-of-the-art techniques to replicate lesion characteristics from diverse populations. These synthetic images were created using generative algorithms trained on publicly available data.
In this paper, the dataset is subjected to uniform scaling, which ensures that the resolution remains constant at 299 × 299. During the process of image analysis, each image in the dataset is subjected to adjustments to continue maintaining the impartiality of individual pixels. Images are translated using the coordinate values (u, v), which represent the positions of pixels in the initial image. Obtaining new coordinates for the translated image is accomplished by subtracting the translation amounts (δx, δy) from the original coordinates (u − δx, v − δy) given in Equation 1. Substituting these new coordinates into the original image allows for the calculation of the pixel values that correspond to the translated image. Typically, the image coordinate system starts in the upper-left corner of the image and continues horizontally to the right and vertically downward. This is the conventional method. The amounts of translation, which are represented by the symbols δx and δy, respectively, are those which specify the amount of movement along the x-axis and the y-axis, respectively. The movement to the right is brought about by positive dx values, while the shift to the left is brought about by negative values. The contrary is also true: δy values that are positive bring about a downward movement, whereas δy values that are negative bring about an upward shift. It is because of this purposeful translation that the data have a larger degree of variability. This is helpful in computer vision, which is where tasks such as picture categorization are carried out (Razmjooy et al., 2020).
Image scaling is a technique that involves altering its size, which may either result in it being much bigger or significantly smaller than it was originally. This approach has a wide range of potential applications in several different areas. This can be performed using several different methods, some of which are Nearest Neighbor, Bilinear Interpolation, and Bicubic Interpolation, amongst others. The process of augmentation is performed on the dataset selected for this research using Bilinear interpolation. Bilinear interpolation produces better results as compared to the other more popular algorithms such as nearest-neighbor; it produces better results when it comes to the process of upscaling images in the dataset (Vidya and Karki, 2020).
To provide the mathematical basis for bilinear interpolation provided in Equation 2, complex computations are performed based on the pixel values of points that are next to one another. An original image I with dimensions (u, v) and a target image I′ with dimensions (u′, v′) to upscale, where u′ is more than u and v′ is greater than v. Bilinear interpolation makes use of the four pixels that are next to one another in the initial image and makes use of scaling factors Su and Sv to ascertain the value of a pixel I′ (u′, v′) in the newly reconstructed image. Where α and β are interpolation coefficients, Su and Sv represent scaling factors for the u and v axes, respectively, and α and β are interpolation coefficients as given in Equations 3, 4. Within the context of the enhancement of datasets for a wide range of applications, this in-depth description highlights the adaptability and accuracy of bilinear interpolation, which makes it a very important tool. Figure 3 represents the augmented image samples used for the proposed model.
Table 1 provides a detailed overview of how the dataset changed before and after augmentation, showing the different classes and their quantities. Each row has a number, showing the order. In the first part of the table, it lists the types of skin lesions and how many there were before augmentation. In the second part, it shows the same classes but with more instances after augmentation, all set to 600. This helps balance the dataset by making sure each class has the same amount of data.
To execute the proposed federated framework approach, four edge devices are set up and modified to guarantee seamless connection with the server that had hosted the base model. In this setting, the central server serves as the coordinator, and the edge nodes are the ones synchronized with the server and can take part in the learning process. The central server is the one that receives the request for the model updates from the edge devices and performs the learning procedure collaboratively. The representation of the collaborative learning procedure is given in Figure 4 as it learns from a variety of data sources in the form of nodes.
A distributed technique to improve edge device data allocation is needed to solve the data imbalance problem for an individual node. This technique assigns variable and distributed data to edge nodes for more precise, efficient, and balanced processing. The key challenge in federated learning is to ensure that devices in the networks are not overburdened with excessive data. To cater this issue, the proposed algorithm also incorporates adaptive model partitioning so selective identify nodes with less computational capacity and distribute data accordingly. By doing so, the devices with less computational power are assigned with adequate load to reduce the latency and computational bottlenecks.
Probabilistically distributing data over several edge nodes is Stochastic Data Distribution. Unlike deterministic allocation approaches, stochastic allocation distributes data to edge nodes randomly. Randomness gives edge nodes diverse data to analyze, helping them comprehend the data distribution. Network devices may have limited computing capacity. We partition the dataset into batches and give each edge device a batch to make training more efficient. However, dividing data into batches and sending them to edge devices may not be an ideal approach. Some edge nodes may contain simpler data than others, causing unequal task distribution and poor performance. Each training cycle randomizes and batches the dataset using stochastic data distribution. Edge nodes are randomly assigned to batches to ensure data complexity is distributed evenly among devices. This improves training and means edge nodes may work together to boost performance (Ganaie and Sheetlani, 2019). All edge devices maintain data integrity for correct outcomes. The data that is subject to random chance may be represented by a Probability Distribution Function (PDF) as given in Equations 5 and 6, which shows the chances of certain values occurring. If the data follows a normal distribution, for example, the PDF will also follow a normal distribution (Volos et al., 2018).
Edge nodes are chosen based on data source proximity, communications capabilities, and resource availability. The network’s edge devices are represented by Equations 7 and 8, where i is the number of devices.
Each row in Table 2 represents a distinct kind of skin lesion, such as Actinic keratosis, Basal cell carcinoma, Dermatofibroma, Melanoma, Nevus, Pigmented benign keratosis, Seborrheic keratosis, Squamous cell carcinoma, and Vascular lesion. The numerical values shown in the table cells indicate the number of data points attributed to each node for the relevant skin lesion category. Actinic keratosis is associated with 199 data points allocated to Node (1), 51 to Node (2), 189 to Node (3), and 161 to Node (4). Likewise, different kinds of skin lesions are distributed differently throughout the nodes, indicating the various characteristics of the dataset. This allocation technique guarantees that every node gets a representative portion of data for thorough analysis while evenly distributing the burden throughout the computer resources. However, some nodes are not provided with any images to display the efficient behavior of the federated learning approach when it aggregates the different models acquired from the nodes. Table 3 illustrates the distribution of data for both training and testing purposes. Dataset within each node is distributed in the training set and testing set, 85% is allocated for training and 15% is set for testing.
Table 4 represents the validation dataset allocated for the globally trained model at the centralized server, which will be transferred to the edge devices for edge-based classification. All the nine distinct classes have their specific number of validation samples; for example, Actinic keratosis has 49 validation samples, Melanoma has 46, Nevus has 48, and vice versa. These samples are the main source of evaluating the global model as it will use these very samples to predict their classes and generate a confusion matrix which is displayed in the result section.
The training data is further divided into batches and each batch contains 32 image samples; this indicates the amount of samples processed in each iteration during the training procedure. It is important to randomize the order of the samples, otherwise, the model may learn the sequence of the input data. To cater to this, a shuffle buffer of size 100 elements is utilized. Tensorflow provides this feature to use a shuffle buffer and maintain a buffer size of 100 elements throughout the training process. It randomly shuffles through the dataset to create shuffled batches that can be used to train the model. The total number of epoch selected is 14.
In this paper, an approach to classify skin diseases is introduced that makes use of IoT-enabled edge computing in a federated environment. The framework comprises three essential components that work together to make a federated system. The three components are: edge devices, a core federated server, and a joint federated learning system. The aim of the proposed algorithm is to fill the gaps in the traditional classification techniques that are based on a centralized computing model and replace it with the decentralized computing model, this feature of federated computing simultaneously promotes data security and confidentiality.
In the proposed scenario of federated learning, the base model selected is InceptionV3. It is a pretrained Deep learning CNN classifier specifically designed for image classification. It is widely utilized for numerous tasks based on applications related to computer vision. Table 5 represents an overview of the InceptionV3 base model used for the federated approach.
Basically, InceptionV3 is made up of multiple convolutional layers that are used to extract features from an input image. It requires an image of resolution 299 × 299 × 3, where 3 represents the RGB color channel. These layers have distinct filters to detect low-level features like edges, textures, etc. that helps the model learn more abstract features when entering the deeper layers. Within each Inception module, the network performs a convolutional process having different size of kernels like 1 × 1, 3 × 3, and 5 × 5. The output of these modules is combined using concatenation or dimensionality reduction.
Federated learning offers more advantages as compared to the conventional centralized learning algorithms, particularly in areas where data must be kept private, and in areas with distributed infrastructure. Unlike centralized approaches, federated learning facilitates continuous model updates at the edge node without the need to transfer data to the central server. This feature can take new data on continuous bases and update the model with new dataset. This can alleviate concerns regarding communication delays and workload escalation. However, it is important to acknowledge that these advantages are context-dependent and come with trade-offs, such as increased computational demands on edge devices and challenges in handling heterogeneous data. The proposed framework promotes a swift response in mobile computing-based applications facilitated by low-latency network issues in real-time. Each edge device present in the network is equipped with an image sensory source capable of acquiring images in real time for classification and acts as a local training node that facilitates local model training on the available dataset. As soon as the base model is dispatched to the edge devices, they train their locally assembled dataset and intend to capture the intricate features of their respective datasets while customizing the model to the specific environment of each device. There is a possibility that one edge device may differ from the other edge device within the same network in terms of computational power, storage, or any other hardware-based feature. The edge device transmits the trained model weights to the central federated server where it aggregates the updated model parameters and refines the global model. The federated learning process exhibits a collaborative nature which enables it to enhance the model iteratively by utilizing the inputs from the edge devices and this process continues until the model converges and attains the optimal solution that contains the collective knowledge of all the edge devices within the network. The proposed model enables collaborative learning by decentralizing the training process to edge devices; this in turn allows the data to be locally on the edge devices. Only weights are transferred to the central server instead of the raw information such as medical images. While this approach significantly enhances privacy by keeping data localized, it is important to recognize that federated learning is not entirely immune to vulnerabilities, such as data reconstruction attacks or model inversion risks. To mitigate these concerns, the framework incorporates secure aggregation and differential privacy techniques, ensuring that data privacy and security are upheld throughout the training process while promoting collaborative efficiency. The reason of choosing Secure Aggregation is to make sure that the individual model updates remain private and only the centralized server receives the aggregated sum of encrypted gradients. This method effectively protects sensitive data without requiring additional techniques like Differential Privacy (DP). Given the computational and communication constraints of IoT-enabled edge devices, secure aggregation provides a balanced approach to prioritize security without any additional computational overheads, thus, significantly boosting the real-time processing capabilities of the model. Since the server never has access to raw model updates, gradient-based attacks such as model inversion become infeasible, ensuring robust privacy preservation.
Equation 9 demonstrates the cross-entropy loss function in which φ denotes the base model parameters, N is the total number of training samples, is the predicted probability of the positive class for input , and is the true label (0 or 1) for input .
The process of forward propagation is typically utilized to calculate this function through different neural network layers given in Equation 10. Every layer in the network uses the input information and applies transformation on it with the specified weights and biases, and the activation function. The output of each layer is obtained by passing it through the activation function with the sum of all the input data passing through the function. This process of iteration continues until the final layer that generates the desired output of the network is achieved.
Equation 11 demonstrates the gradient descent approach mostly used in the problem based on federated averaging. The symbol θ is the parameter that changes in every iterative process and η represents the learning rate which makes the process converge swiftly and robustly depending on its value and dictates the magnitude of each weight. The symbol represents the gradient of the local cost function depicts the current parameter values at the edge node, and it indicates the direction in which the gradient is increasing in each iteration. The procedure changes the parameter at each iterative process by subtracting η times the gradient from making it shift toward the optimal solution by minimizing the local cost function. It is possible for the cost function to shift the gradient to the opposite end by minimizing it in each iterative process by learning rate. Equation 12 demonstrates the model updates from various edge devices in the network to be aggregated and generate the global classification model. Here, K represents the total number of edge devices present in the network, Nk is the number of samples at edge node k and N represents the total number of samples in the entire dataset.
The central server typically receives the differences or updates Δθ between the current and prior model parameters instead of the model parameters directly being delivered. This helps in minimizing communication overhead. Equations 13 and 14 are crucial in federated learning as they illustrate the fundamental updating mechanism carried out by each client device in the training phase. This equation demonstrates how each client changes its local model parameters using its own dataset in federated learning. The updated parameters are then sent to a central server for aggregation. The update includes removing a portion of the gradient of the local loss function from the current parameters , which is then scaled by the ratio of the client’s dataset size to the overall dataset size across all clients N, and finally adjusted by the learning rate η. Raw data is kept on local devices, and only model updates are sent.
Each client calculates its model update Δθk based on its local data and model parameters. Before sending the update to the central server, it encrypts the update using cryptographic techniques, expressed as Encrypt (Δθk) in Equation 15. The encrypted updates from all clients are transmitted to the central server. The server performs the aggregation operation, denoted as Encrypted_Aggregation, on the encrypted updates to obtain the aggregated encrypted update Δθ represented in Equation 16. After receiving the aggregated update Δθ, the central server decrypts it using the corresponding decryption keys, represented as Decrypt (Δθ) in Equation 17. This leads to the final model update that may be implemented on the global model parameters for the subsequent training cycle.
By using differential privacy, noise is introduced to the client updates in order to safeguard the privacy of individual data given in Equation 18. The noise is drawn from a distribution that provides differential privacy guarantees with a specified privacy budget ϵ. The proposed approach involves adding a proximal term to the local goal function as shown in Equation 19 to encourage model parameters to remain near their prior values, which helps in achieving smoother updates and stability.
Basically, MPC is considered to be a cryptographic technique that enables different nodes present in the network to collaboratively compute a specific function or a task over their own private set of data while keeping them secure and confidential at the same time. The true aim of the MPC is to ensure privacy in collaborative processing where it is essential for all the nodes involved to keep their data confidential as it may be sensitive information and they do not wish to disclose it with other involved parties. MPC allows the involved nodes to perform computation on their own data without revealing it to the other parties involved. In the proposed scenario, N parties are taking part in the computation process which is denoted by ( ), each of these parties has its own set of data denoted by ( ) respectively. In the proposed collaborative scheme, these nodes are trying the train a model that would distinguish between skin diseases, let be the function these nodes are trying to perform which is to acquire a trained model for skin disease classification given in Algorithm 1. It displays the process of secure aggregation performed using TensorFlow Federated.
• Secret sharing: All the involved Nodes splits their confidential input data into n number of shares.
• Secure computation: All the involved parties are now engaged in a protocol to collaboratively compute the function f. This process is done securely which ensures that the data of each node is kept private.
• Reconstruction: As soon as the reconstruction is complete, all the involved parties are now able to reconstruct the encrypted information by computing their share of the output generated through the function. This only reveals the output of the function and keeps the subsequent input of each node private.
Algorithm 1. Federated averaging procedure utilizing multi-party computation (MPC) a secure aggregation process.
The secret-sharing method ensures that all the involved nodes holds n shares denoted by of the input , denoted in Equation 20, where ⨁ represents the bitwise XOR operator. Subsequently, the output of the function f can be computed as given in Equation 21.
In this paper, extensive evaluation has been performed to validate the effectiveness of the proposed federated learning algorithm integrated with IoT-enabled edge computing. Evaluation is performed between the classification accuracy of the proposed federated approach and other centralized classification algorithms. The objective of the proposed technique is to accurately classify skin disease and at the same time make the patient’s confidential data secure. The protection of patient’s confidential information is of utmost importance thus the utilization of the federated algorithm is justified in this regard as it facilitates decentralized training as its trait. Scalability of the federated approach may become challenging if the node far exceeds the desired quantity, it may cause communication delay or latency. The network’s ability to cater to the increasing number of edge nodes is crucial and it must accommodate them and ascertain their suitability for diverse network settings. Evaluating the federated learning algorithm’s ability to generate a globally optimally performing model, including convergence speed and efficiency, was a critical component of this paper. For the evaluation of the proposed framework, we have utilized accuracy, precision, f1-score, and recall as the key performance parameters to understand the model behavior within the federated learning environment. The framework is built on the Python platform utilizing tools like TensorFlow for model generation and also for orchestration. The dataset used for this research is sourced from Kaggle and its name is ISIC which contains a diverse range of images in nine different skin disease classes. Preprocessing is an important aspect of the deep learning process as it is used to standardize image resolution, eliminate artifacts, and also ensure the consistent representation of the dataset across all edge devices in the network.
The trajectory of the global model’s training and validation accuracy is visually depicted in Figure 5 (left), providing a comprehensive illustration of the federated learning process across multiple epochs. Along the x-axis lie the epochs of training, while the y-axis showcases accuracy metrics. The training accuracy curve vividly showcases the model’s iterative refinement on the training dataset, highlighting a consistent improvement trend over successive epochs. Complementing this, the validation accuracy curve serves as a testament to the model’s ability to generalize to new validation data, affirming its robust performance across diverse input images. Figure 5 (right) presents a representation of the training and validation loss patterns of the global model throughout all federated learning cycles. Here, the x-axis delineates the training epochs, while the y-axis denotes the loss value. The training loss curve perceptibly illustrates the progressive reduction in loss across epochs, indicative of model parameter refinement and consequent minimization of prediction errors within the training data. Concurrently, the validation loss curve serves as a critical tool for monitoring the model’s performance on validation data, effectively detecting potential issues related to overfitting or underfitting. The convergence observed between the training and validation loss curves signals successful model training, striking a balance between model complexity and generalization capacity. The accuracy of 98.68% was reported which highlights the model’s promising potential. However, accuracy alone does not factor in performance based on real world data which is influenced by factors such as data representativeness and the ability to handle edge cases while generalization of unseen data. To address these considerations, our study includes a diverse dataset and emphasizes rigorous testing across varied conditions to enhance the model’s robustness and applicability. Figure 6 represents the accuracy and loss of each individual node that participated in the training process.
The confusion matrix depicted in Figure 7 depicts the classification performance of the proposed federated approach. It shows the distribution of the positive classification in diagonal entries with false positive or false negative classification presented in non-diagonal form within each distinct category. Actinic keratosis and basal cell carcinoma achieved the classification count of 49 and 44 which is amongst the highest in the confusion matrix. There are also cases of misclassification, such as dermatofibroma being wrongly classified as nevus, and melanoma samples wrongly classified as Nevus. Although there are few misclassifications, the model achieved high performance with minimum error. Accuracy, precision, f1-score, and sensitivity are among the performance parameters used to evaluate the proposed model and demonstrated in Equations 22–25. Precision measures the positive predictions as compared to the total number of positive samples. Recall assesses the proportion of genuine positive predictions among the total number of actual positive cases. F1-score considers both accuracy and recall in the event of class imbalance. A detailed evaluation matrix is provided in Table 6 demonstrating the performance of the proposed model in classifying different skin disease categories.
Table 7 demonstrates the comprehensive comparison of the skin disease classification algorithm. There is different algorithms proposed by researchers around the world in this particular field, for instance, the SENet154 architecture (Li et al., 2020) successfully attained a an accuracy of 0.87 indicating the successful classification of the positive category and achieving a high level of overall accuracy. The Ensemble CNN algorithm presented in Thurnhofer-Hemsi et al. (2021) demonstrates an accuracy of 0.84. I. Goitis (Giotis et al., 2015) proposed a system MED-NODE, which achieved good results with an accuracy of 0.81 and a sensitivity of 0.80. D. Ruiz in Ruiz et al. (2011) utilised a multilayer perceptron (MLP) approach that exhibited an classification rate of 0.806 and classifies melanoma accurately with sensitivity of 0.745. R. Amelard in Amelard et al. (2012) utilised a high-level intuitive feature extraction approach for skin lesion classification with an accuracy and sensitivity of 0.873 and 0.907, respectively. The modified CNN model in Inthiyaz et al. (2023) achieved an accuracy of 0.874 and an AUC of 0.87, on the other hand, Mobile net in Hossen et al. (2022) achieved a sensitivity of 0.931, f1-score of 0.901 and an accuracy of 0.963 showcasing its better performance as compared to the VGG model. The proposed federated framework achieved an accuracy of 0.986 demonstrating its effectiveness in classifying positive categories Table 7.
In order to evaluate the practicality of the proposed model in this setting we performed an analysis based on computational overhead and energy utilization of the edge device, given in Table 8. To measure these parameters, we have used a Raspberry Pi 4 as one of the edge node. In IoT-based edge computing environments, resource constraints such as energy consumption and computational capacity play a critical role in determining the feasibility of deploying machine learning frameworks. Energy usage was measured in watts (W) during both the training and inference phases of the model on Raspberry Pi 4 edge devices. The time required for training, inference, and communication with the central server was recorded to assess the computational burden on the edge devices. The transfer of data between the edge node and the central server was also monitored to estimate the bandwidth requirement and some potential bottlenecks in the environment where resources are less.
• Energy consumption: Measures the power usage during training (5.6 W), inference (3.2 W), and communication (2.8 W).
• Computational time: Shows the time required for training (120 s per epoch), inference (1.5 s per prediction), and communication (0.8 s per data batch).
• Bandwidth usage: Evaluates network data usage, with no bandwidth required for local computation during training, 0.5 MB per prediction for inference, and 2.2 MB per data batch during communication.
This paper presented a privacy-preserving framework to accurately classify skin disorders. The proposed framework integrated federated learning into an IoT-enabled edge-computing environment. In addition to offering accurate and timely skin disease classification, it ensured the security of the patient’s confidential information. It used distributed computing to facilitate the training process at the edge nodes in the network, unlike centralized computing where the data is collected at the server to commence the training procedure. Through rigorous testing and evaluation, the proposed algorithm achieved an accuracy of 98.6% outperforming the different centralized learning models. Federated learning makes use of the collective intelligence of the existing edge devices that contain distinct datasets in the network to train the global model. It strives to achieve an optimal model through each iterative process by sharing the trained updates with the centralized federated server where they are aggregated to generate a global model. These features of the proposed federated learning process demonstrate promise in real-world applications including skin disease classification and other disease diagnoses. By sharing the workload with the edge devices and aggregating model updates at the central server in a privacy-preserving manner makes the proposed algorithm scalable, efficient, and a model solution for skin disease classification. This approach holds significant potential for driving meaningful progress in dermatological healthcare practices.
Publicly available datasets were analyzed in this study. This data can be found at: https://www.kaggle.com/datasets/nodoubttome/skin-cancer9-classesisic/data.
Ethical approval was not required for the study involving humans in accordance with the local legislation and institutional requirements. Written informed consent to participate in this study was not required from the participants or the participants’ legal guardians/next of kin in accordance with the national legislation and the institutional requirements. Written informed consent was obtained from the individual(s) for the publication of any potentially identifiable images or data included in this article.
NA: Conceptualization, Writing – original draft, Data curation, Supervision. JA: Writing – original draft, Methodology. AS: Writing – original draft, Software, Visualization. OS: Writing – review & editing, Formal Analysis, Software. AM: Resources, Writing – review & editing. AR: Formal analysis, Writing – review & editing. RU: Supervision, Writing – review & editing. MK: Investigation, Resources, Writing – review & editing.
The author(s) declare that financial support was received for the research and/or publication of this article. This work is funded by Princess Nourah bint Abdulrahman University Researchers Supporting Project number (PNURSP2025R760), Princess Nourah bint Abdulrahman University, Riyadh, Saudi Arabia. The authors extend their appreciation to the Deanship of Scientific Research at King Khalid University for funding this work through a Small Group Research Project under grant number RGP1/405/44.
The authors declare that the research was conducted in the absence of any commercial or financial relationships that could be construed as a potential conflict of interest.
The authors declare that no Gen AI was used in the creation of this manuscript.
All claims expressed in this article are solely those of the authors and do not necessarily represent those of their affiliated organizations, or those of the publisher, the editors and the reviewers. Any product that may be evaluated in this article, or claim that may be made by its manufacturer, is not guaranteed or endorsed by the publisher.
Ahmad, B., Usama, M., Huang, C.-M., Hwang, K., Hossain, M. S., and Muhammad, G. (2020). Discriminative feature learning for skin disease classification using deep convolutional neural network. IEEE Access 8, 39025–39033. doi: 10.1109/ACCESS.2020.2975198
Allugunti, V. R. (2022). A machine learning model for skin disease classification using convolution neural network. Int. J. Comput. Program. Database Manage. 3, 141–147. doi: 10.33545/27076636.2022.v3.i1b.53
Al-Rakhami, M. S., AlQahtani, S. A., and Alawwad, A. (2024). Effective skin cancer diagnosis through federated learning and deep convolutional neural networks. Appl. Artif. Intell. 38:2364145. doi: 10.1080/08839514.2024.2364145
Amelard, R., Wong, A., and Clausi, D. A. (2012). “Extracting morphological high-level intuitive features (HLIF) for enhancing skin lesion classification, 2012 Annual International Conference of the IEEE Engineering in Medicine and Biology Society,” San Diego, CA, USA, 4458–4461, doi: 10.1109/EMBC.2012.6346956
Barata, C., Celebi, M. E., and Marques, J. S. (2021). Explainable skin lesion diagnosis using taxonomies. Pattern Recognition, 110:107413. doi: 10.1016/j.patcog.2020.107413
Bhatt, H., Shah, V., Shah, K., Shah, R., and Shah, M. (2023). State-of-the-art machine learning techniques for melanoma skin cancer detection and classification: a comprehensive review. Intell. Med. 3, 180–190. doi: 10.1016/j.imed.2022.08.004
Cai, G., Zhu, Y., Wu, Y., Jiang, X., Ye, J., and Yang, D. (2023). A multimodal transformer to fuse images and metadata for skin disease classification. Vis. Comput. 39, 2781–2793. doi: 10.1007/s00371-022-02492-4
Chen, P., He, G., Qian, J., Zhan, Y., and Xiao, R. (2021). Potential role of the skin microbiota in inflammatory skin diseases. J. Cosmet. Dermatol. 20, 400–409. doi: 10.1111/jocd.13538
Chen, X., Li, Y., and Zhang, H. (2021). A deep convolutional neural network-based approach for skin cancer classification. J. Biomed. Inform. 115:103663.
Diepgen, T. L. (2003). Occupational skin-disease data in Europe. Int. Arch. Occup. Environ. Health 76, 331–338. doi: 10.1007/s00420-002-0418-1
Dildar, M., Akram, S., Irfan, M., Khan, H. U., Ramzan, M., Mahmood, A. R., et al. (2021). Skin cancer detection: a review using deep learning techniques. Int. J. Environ. Res. Public Health 18:5479. doi: 10.3390/ijerph18105479
Divya Anand, N., and Sharma, G. (2024). Convolutional neural network (CNN) and federated learning-based privacy preserving approach for skin disease classification. J. Supercomput. 80, 24559–24577. doi: 10.1007/s11227-024-06309-0
Elston, D. M. (2020). Occupational skin disease among health care workers during the coronavirus (COVID-19) epidemic. J. Am. Acad. Dermatol. 82, 1085–1086. doi: 10.1016/j.jaad.2020.03.012
English, J. S. C., Dawe, R. S., and Ferguson, J. (2003). Environmental effects and skin disease. Br. Med. Bull. 68, 129–142. doi: 10.1093/bmb/ldg026
Fleischer, A. B. Jr., Herbert, C.-R., Feldman, S. R., and O’Brien, F. (2000). Diagnosis of skin disease by nondermatologists. Am J Manag Care. 6:1149–56.
Fried, R. G., Gupta, M. A., and Gupta, A. K. (2005). Depression and skin disease. Dermatol. Clin. 23, 657–664. doi: 10.1016/j.det.2005.05.014
Ganaie, G. H., and Sheetlani, J. (2019). Study of structural relationship of interconnection networks. In Smart Intelligent Computing and Applications: Proceedings of the Third International Conference on Smart Computing and Informatics, Singapore: Springer Singapore. 2, 379–385. doi: 10.1007/978-981-32-9690-9_39
Ginsburg, I. H. (1996). The psychosocial impact of skin disease: an overview. Dermatol. Clin. 14, 473–484. doi: 10.1016/S0733-8635(05)70375-2
Giotis, I., Molders, N., Land, S., Biehl, M., Jonkman, M. F., and Petkov, N. (2015). MED-NODE: A computer-assisted melanoma diagnosis system using non-dermoscopic images. ESWA, 42, 6578–6585. doi: 10.1016/j.eswa.2015.04.034
Goceri, E. (2021). Diagnosis of skin diseases in the era of deep learning and mobile technology. Comput. Biol. Med. 134:104458. doi: 10.1016/j.compbiomed.2021.104458
Hay, R. J., Johns, N. E., Williams, H. C., Bolliger, I. W., Dellavalle, R. P., Margolis, D. J., et al. (2014). The global burden of skin disease in 2010: an analysis of the prevalence and impact of skin conditions. J. Invest. Dermatol. 134, 1527–1534. doi: 10.1038/jid.2013.446
Hossen, M. N., Panneerselvam, V., Koundal, D., Ahmed, K., Bui, F. M., and Ibrahim, S. M. (2022). Federated machine learning for detection of skin diseases and enhancement of internet of medical things (IoMT) security. IEEE J. Biomed. Health Inform. 27, 835–841. doi: 10.1109/JBHI.2022.3149288
Inthiyaz, S., Altahan, B. R., Ahammad, S. H., Rajesh, V., Kalangi, R. R., Smirani, L. K., et al. (2023). Skin disease detection using deep learning. Advances in Engineering Software, 175:103361. doi: 10.1016/j.advengsoft.2022.103361
Jowett, S., and Ryan, T. (1985). Skin disease and handicap: an analysis of the impact of skin conditions. Soc. Sci. Med. 20, 425–429. doi: 10.1016/0277-9536(85)90021-8
Karimkhani, C., Dellavalle, R. P., Coffeng, L. E., Flohr, C., Hay, R. J., Langan, S. M., et al. (2017). Global skin disease morbidity and mortality: an update from the global burden of disease study 2013. JAMA Dermatol. 153, 406–412. doi: 10.1001/jamadermatol.2016.5538
Kumar, M., Alshehri, M., AlGhamdi, R., Sharma, P., and Deep, V. (2020). A de-ann inspired skin cancer detection approach using fuzzy c-means clustering. Mobile Netw. Appl. 25, 1319–1329. doi: 10.1007/s11036-020-01550-2
Li, W. Zhuang, J., Wang, R., Zhang, J., and Zheng, W. -S. (2020). “Fusing Metadata and Dermoscopy Images for Skin Disease Diagnosis,” 2020 IEEE 17th International Symposium on Biomedical Imaging (ISBI), Iowa City, IA, USA, 1996–2000. doi: 10.1109/ISBI45749.2020.9098645
Li, H., Pan, Y., Zhao, J., and Zhang, L. (2021). Skin disease diagnosis with deep learning: a review. Neurocomputing 464, 364–393. doi: 10.1016/j.neucom.2021.08.096
Lim, H. W., Collins, S. A. B., Resneck, J. S. Jr., Bolognia, J. L., Hodge, J. A., Rohrer, T. A., et al. (2017). The burden of skin disease in the United States. J. Am. Acad. Dermatol. 76, 958–972.e2. doi: 10.1016/j.jaad.2016.12.043
Mondal, B., Das, N., Santosh, K. C., and Nasipuri, M. (2020). “Improved Skin Disease Classification Using Generative Adversarial Network,” 2020 IEEE 33rd International Symposium on Computer-Based Medical Systems (CBMS), Rochester, MN, USA, 520–525. doi: 10.1109/CBMS49503.2020.00104
Monika, M., Krishna, N. A. V., Kumari, C. U., Kumar, M. N. V. S. S., and Lydia, E. L. (2020). Skin cancer detection and classification using machine learning. Mater. Today Proc. 33, 4266–4270. doi: 10.1016/j.matpr.2020.07.366
Nahata, H., and Singh, S. P. (2020). Deep Learning Solutions for Skin Cancer Detection and Diagnosis. In: V. Jain and J. Chatterjee (eds) Machine Learning with Health Care Perspective. Learning and Analytics in Intelligent Systems, Springer, Cham. 13. doi: 10.1007/978-3-030-40850-3_8
Nawaz, M., Mehmood, Z., Nazir, T., Naqvi, R. A., Rehman, A., Iqbal, M., et al. (2022). Skin cancer detection from dermoscopic images using deep learning and fuzzy k‐means clustering. Microsc. Res. Tech 85, 339–351. doi: 10.1002/jemt.23908
Oliveira, D. F., He, L., Silva, T. M., Queiroz, J. P., and Santos, L. D. (2021). A robust deep learning model for skin cancer detection. J. Ambient. Intell. Humaniz. Comput. 12, 2881–2890.
Pacheco, A. G. C., and Krohling, R. A. (2020). The impact of patient clinical information on automated skin cancer detection. Comput. Biol. Med. 116:103545. doi: 10.1016/j.compbiomed.2019.103545
Razmjooy, N., Ashourian, M., Karimifard, M., Estrela, V. V., Loschi, H. J., Nascimento, D., et al. (2020). Computer-aided diagnosis of skin cancer: a review. Curr. Med. Imaging 16, 781–793. doi: 10.2174/1573405616666200129095242
Razzak, I., Shoukat, G., Naz, S., and Khan, T. M.. (2020). “Skin Lesion Analysis Toward Accurate Detection of Melanoma using Multistage Fully Connected Residual Network,” 2020 International Joint Conference on Neural Networks (IJCNN), Glasgow, UK, 1–8. doi: 10.1109/IJCNN48605.2020.9206881
Ruiz, D., Berenguer, V., Soriano, A., and Sanchez, B. (2011). A decision support system for the diagnosis of melanoma: A comparative approach. ESWA, 38, 15217–15223. doi: 10.1016/j.eswa.2011.05.079
Santosh, K. C., Kaur, M., and Jain, S. (2021). A review on skin cancer detection using deep learning and artificial intelligence. Health Inf. Sci. Syst. 9, 1–10.
Seth, D., Cheldize, K., Brown, D., and Freeman, E. E. (2017). Global burden of skin disease: inequities and innovations. Curr. Derm. Rep. 6, 204–210. doi: 10.1007/s13671-017-0192-7
Siddique, A. A., Alasbali, N., Driss, M., Boulila, W., Alshehri, M. S., and Ahmad, J. (2024). Sustainable collaboration: federated learning for environmentally conscious forest fire classification in green internet of things (IoT). Internet Things 25:101013. doi: 10.1016/j.iot.2023.101013
Siddique, A. A., Boulila, W., Alshehri, M. S., Ahmed, F., Gadekallu, T. R., Victor, N., et al. (2023). Privacy-enhanced pneumonia diagnosis: IoT-enabled federated multi-party computation in industry 5.0. IEEE Trans. Consum. Electron. 70, 1923–1939. doi: 10.1109/TCE.2023.3319565
Srinivasu, P. N., SivaSai, J. G., Ijaz, M. F., Bhoi, A. K., Kim, W., and Kang, J. J. (2021). Classification of skin disease using deep learning neural networks with MobileNet V2 and LSTM. Sensors 21:2852. doi: 10.3390/s21082852
Tabrizchi, H., Parvizpour, S., and Razmara, J. (2023). An improved VGG model for skin cancer detection. Neural. Process. Lett. 55, 3715–3732. doi: 10.1007/s11063-022-10927-1
Thanh, D. N. H., Prasath, V. B. S., Hieu, L. M., and Hien, N. N. (2020). Melanoma skin cancer detection method based on adaptive principal curvature, colour normalisation and feature extraction with the ABCD rule. J. Digit. Imaging 33, 574–585. doi: 10.1007/s10278-019-00316-x
Thurnhofer-Hemsi, K., López-Rubio, E., Domínguez, E., and Elizondo, D. A. (2021). “Skin Lesion Classification by Ensembles of Deep Convolutional Networks and Regularly Spaced Shifting, in IEEE Access,” 9, 112193–112205. doi: 10.1109/ACCESS.2021.3103410
Toğaçar, M., Cömert, Z., and Ergen, B. (2021). Intelligent skin cancer detection applying autoencoder, MobileNetV2 and spiking neural networks. Chaos, Solitons Fractals 144:110714. doi: 10.1016/j.chaos.2021.110714
Vidya, M., and Karki, M.V.. (2020). Skin cancer detection using machine learning techniques. In 2020 IEEE international conference on electronics, computing and communication technologies (CONECCT), pp.1–5. IEEE.
Volos, H., Bando, T., and Konishi, K., (2018). “Latency Modeling for Mobile Edge Computing Using LTE Measurements, 2018 IEEE 88th Vehicular Technology Conference (VTC-Fall),” Chicago, IL, USA. 1–5. doi: 10.1109/VTCFall.2018.8691027
Keywords: federated learning, healthcare technology, internet of things (IoT), edge computing, decentralized network architecture, distributed computing
Citation: Alasbali N, Ahmad J, Siddique AA, Saidani O, Al Mazroa A, Raza A, Ullah R and Khan MS (2025) Privacy-enhanced skin disease classification: integrating federated learning in an IoT-enabled edge computing. Front. Comput. Sci. 7:1550677. doi: 10.3389/fcomp.2025.1550677
Received: 23 December 2024; Accepted: 13 March 2025;
Published: 10 April 2025.
Edited by:
Maheswar Rajagopal, KPR Institute of Engineering and Technology, IndiaCopyright © 2025 Alasbali, Ahmad, Siddique, Saidani, Al Mazroa, Raza, Ullah and Khan. This is an open-access article distributed under the terms of the Creative Commons Attribution License (CC BY). The use, distribution or reproduction in other forums is permitted, provided the original author(s) and the copyright owner(s) are credited and that the original publication in this journal is cited, in accordance with accepted academic practice. No use, distribution or reproduction is permitted which does not comply with these terms.
*Correspondence: Rahmat Ullah, cmFobWF0LnVsbGFoQGVzc2V4LmFjLnVr
Disclaimer: All claims expressed in this article are solely those of the authors and do not necessarily represent those of their affiliated organizations, or those of the publisher, the editors and the reviewers. Any product that may be evaluated in this article or claim that may be made by its manufacturer is not guaranteed or endorsed by the publisher.
Research integrity at Frontiers
Learn more about the work of our research integrity team to safeguard the quality of each article we publish.