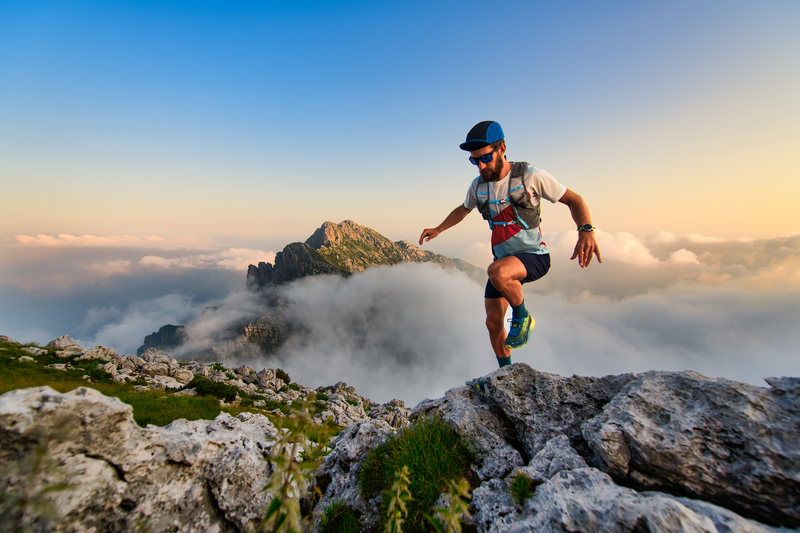
95% of researchers rate our articles as excellent or good
Learn more about the work of our research integrity team to safeguard the quality of each article we publish.
Find out more
ORIGINAL RESEARCH article
Front. Comput. Sci.
Sec. Computer Graphics and Visualization
Volume 7 - 2025 | doi: 10.3389/fcomp.2025.1549693
The final, formatted version of the article will be published soon.
You have multiple emails registered with Frontiers:
Please enter your email address:
If you already have an account, please login
You don't have a Frontiers account ? You can register here
Motion synthesis using machine learning has seen rapid advancements in recent years. Unlike traditional animation methods, utilizing deep learning to generate human movement offers the unique advantage of producing slight variations between motions, similar to the natural variability observed in real examples. While several motion synthesis methods have achieved remarkable success in generating highly varied and probabilistic animations, controlling the synthesized animation in real-time while retaining stochastic elements remains a serious challenge. The main purpose of this work is to develop a Conditional Generative Adversarial Network to generate real-time controlled motion that balances realism and stochastic variability. To achieve this, three novel Generative Adversarial models were developed. The models differ in the architecture of their generators that utilize: a Mixture-of-Experts method, a Latent-Modulated Noise Injection technique, and a Transformer-based architecture respectively. We consider the latter to be the main contribution of this work and we evaluate our method by comparing it to the other two models on both stylized locomotion data and complex, aperiodic dance sequences, assessing its ability to generate diverse, realistic motions while responding to user control. Our findings highlight the trade-offs between motion quality, variety, and computational efficiency in real-time motion synthesis, contributing to the ongoing development of more flexible and varied animation techniques.
Keywords: motion synthesis, Gans, transformers, Mixture of experts, deep learning, Character control
Received: 21 Dec 2024; Accepted: 26 Mar 2025.
Copyright: © 2025 Belesis, Loi and Moustakas. This is an open-access article distributed under the terms of the Creative Commons Attribution License (CC BY). The use, distribution or reproduction in other forums is permitted, provided the original author(s) or licensor are credited and that the original publication in this journal is cited, in accordance with accepted academic practice. No use, distribution or reproduction is permitted which does not comply with these terms.
* Correspondence:
Antonis Belesis, University of Patras, Patras, Greece
Konstantinos Moustakas, University of Patras, Patras, Greece
Disclaimer: All claims expressed in this article are solely those of the authors and do not necessarily represent those of their affiliated organizations, or those of the publisher, the editors and the reviewers. Any product that may be evaluated in this article or claim that may be made by its manufacturer is not guaranteed or endorsed by the publisher.
Research integrity at Frontiers
Learn more about the work of our research integrity team to safeguard the quality of each article we publish.