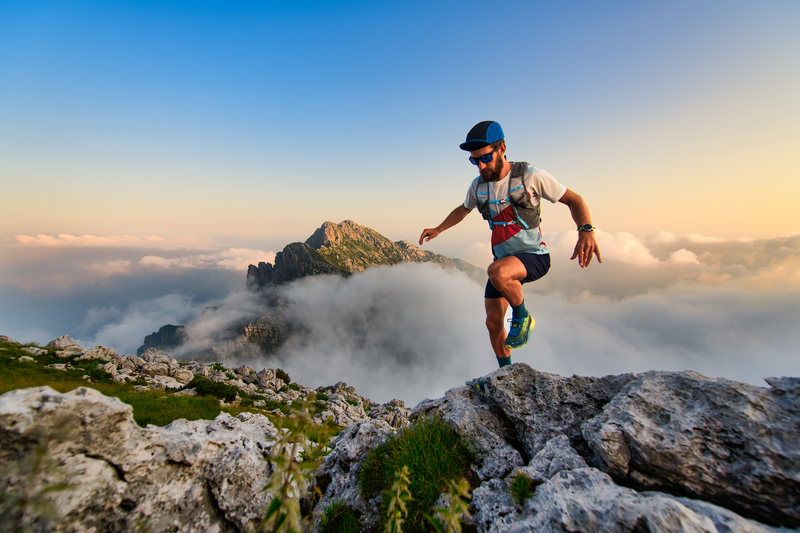
94% of researchers rate our articles as excellent or good
Learn more about the work of our research integrity team to safeguard the quality of each article we publish.
Find out more
ORIGINAL RESEARCH article
Front. Comput. Sci. , 20 February 2025
Sec. Computer Security
Volume 7 - 2025 | https://doi.org/10.3389/fcomp.2025.1545282
This article is part of the Research Topic Cyber Resilience in IoE: Integrating Artificial Intelligence for Robust Security View all articles
Providing cyber-resilient IoE systems has become the need of modern times. In particular, IoT drones are prone to several cyber attacks while navigating in the air. Deliberate transmission of deceptive GPS signals targeted at commercial applications can misdirect global positioning system (GPS)-guided drones, causing them to deviate from their intended paths. Thus, efficient anti-spoofing technology is required to guarantee the safety measures of drone operations. Many techniques for identifying GPS spoofing are available, but most of them need extra hardware, which may not be feasible for tiny or resource-constrained drones. In this regard, this study introduces a specialized method to identify GPS signal spoofing in these drones, called MobileNet. The MobileNet is a convolutional neural network-based transfer learning model that is adopted in this study for drone security along with Chi-square-selected features. The initial phase involves a series of steps to acquire and prepare the GPS signal dataset. Afterward, the dataset is prepared for modeling through preprocessing, data cleaning, and feature extraction. Extensive comparison analysis is performed to evaluate deep learning and transfer learning models. The experimental findings demonstrate the remarkable accuracy of 98.49% by the MobileNet model using Chi-square feature selection. This demonstrates the suitability and capability of the model to perform well in preventing GPS signal spoofing in the context of tiny drone operations.
In today's world, technology is progressing at a fast pace, particularly with the Internet of Everything (IoE) paradigm, where everything is being connected. Due to a large number of connected devices, threats to cyber security have increased exponentially. In particular, drones have become targets of malignant users due to lesser security protocols against cyber attacks. Drones commonly incorporate a variety of sensors, with global positioning system (GPS) receivers being more crucial and sensitive. By receiving signals from satellites, these devices play a crucial role in accurately tracking the drone's position, including its latitude and longitude, as well as its height above the ground. This enhances the drone's navigation accuracy and mission execution efficacy. Despite its crucial role, the inherent susceptibility of GPS signals to manipulation presents a significant threat across various critical domains, encompassing public safety, military operations, air travel, and navigation. Numerous studies have documented GPS signal spoofing-related security flaws (Liu et al., 2018; Liang et al., 2019).
Initially conceived for military purposes, the drones have been adopted into a multitude of civilian and commercial applications. One such instance is Germany DHL's logistics, which uses drones to deliver medicines to Juist island (Benarbia and Kyamakya, 2021). Additionally, the United States (US) Federal Aviation Administration has given Alphabet, and Google's parent firm, permission to use drones to carry meals (Moshref-Javadi and Winkenbach, 2021). Drones are used in different domains other than logistics, such as agriculture and natural resource management, emergency rescue, medicine, wildlife control, and photography (Mohsan et al., 2023). Drones and Internet of Things (IoT) sensors may be integrated to provide a variety of benefits, such as crop and land surveys, energy companies monitoring power infrastructure, and insurance companies inspecting assets and property (Motlagh et al., 2016).
The GPS tracking device industry is expanding rapidly and by 2025 it is expected to have a worth of around 3.38 billion (Jiang et al., 2022). GPS signals are essential for autonomous car safety since the navigation system uses them to calculate the current latitude, longitude, acceleration, and orientation. Malicious GPS assaults are becoming more likely despite their significance due to the proliferation of GPS-enabled gadgets and the reduced expenses of spoofing equipment. Malicious actors can interfere with legitimate GPS signals by using programmable radio devices such as HackRF or USRP. This might cause problems for the targeted vehicles' navigation systems. Using programs like HackRF, researchers have shown that they can change the routes taken by self-driving cars (Souli et al., 2021). Apart from navigation, numerous services and applications based on GPS data improve their efficacy and interaction styles (Kim et al., 2021).
Multiple research works have been conducted for GPS spoofing detection. In Kwon and Shim (2020), the authors utilized acceleration error analysis techniques received from inertial measurement units (IMUs) and GPS receivers. In another research work (Feng et al., 2020), the authors utilized both GPS data and IMU data for accurate detection of GPS spoofing of attacks. Furthermore, Manesh et al. (2019) utilized artificial intelligence (AI) methods to analyze GPS signal features like distance errors, signal strength, and frequency shifts for promising results of GPS spoofing detection. This research highlights the importance of using AI techniques in this domain.
The study Qiao et al. (2017) further utilized techniques like motion detectors and cameras to check if someone physically tampered with the drone architecture. Researchers utilized both GPS data and sensor data for accurate detection. In research (Varshosaz et al., 2019), authors rely solely on the drone's camera to detect spoofing. This research utilizes motion camera sensor data with GPS coordinates to check the attack's exact location. Both these research works are focused on the detection of physical tampering with drone architecture. Similar ideas are explored in other recent studies (Arafat et al., 2023; Prasanna Srinivasan and Sathyadevan, 2023). These research works keep the dataset simple avoid mixing GPS data with sensor data and analyze both types of datasets separately.
Some previous studies (Mehdi et al., 2022) witness that small drones are more likely to become GPS spoofing attacks. To tackle this problem, researchers developed some methods like cooperative navigation, multiple positioning system integration, redundant antennas, and signal verification (Balador et al., 2018). Regardless of the development of multiple ways to deal with the spoofing problem, each technique comes with certain limitations like the requirement of complex hardware systems, environmental hazards, clock synchronization, etc. Another way of GPS spoofing attack detection and classification is done utilizing machine learning (ML) models (Feng et al., 2020).
This study also follows an ML-based approach for spoofing detection. The objective of this research work is to make use of transfer learning and deep learning models for GPS spoofing attack detection in small drones. The datasets used for training and testing these models consist of 13 characteristics extracted from real-time experiments involving GPS signals. Notable contributions of this study are the following:
• The study introduces a transfer learning technique, leveraging multiple types of data to enhance the accuracy and efficiency of detecting GPS signal spoofing in small drones.
• The study creates a systematic methodology for acquiring, preparing, and conducting controlled simulation tests on datasets, ensuring the reliability and validity of experimental data and strengthening the robustness of findings in GPS signal spoofing detection analysis.
• The study enhances GPS signal spoofing detection in small drones by employing the Chi-Square feature selection technique during data preprocessing, identifying important features and significantly improving the efficiency and overall performance of the detection process.
Section 2 presents a summary of current literature and notable advancements in IoT-driven methods. Section 4.1 outlines the dataset and methodologies employed in the carried-out experiments, whereas Section 3 provides details on the utilized feature selection technique and models. Section 4 delivers a thorough understanding of the experimental results, paired with a comprehensive analysis, and Section 5 is a conclusion Section, summarizing the paper.
This segment presents the latest developments, methods, and breakthroughs from diverse research works focused on unraveling the complexities associated with detecting and combating the manipulation of GPS signals. This section establishes the groundwork for the distinctive contributions discussed in the current research work by reviewing various methods, methodologies, and results documented in previous literature. Multiple research studies have been carried out to detect spoofed signals and other types of signals that can cause GPS spoofing. Intrusion detection in sensor networks has been a critical research area due to the increasing deployment of wireless sensor networks (WSNs) in security-sensitive applications. Various studies have proposed methods to safeguard these networks from malicious attacks (Ashraf and Ahmed, 2020). Traditional approaches rely on statistical anomaly detection or signature-based methods, which can fail in dynamic environments or against new attack patterns. Recent advancements in ML have introduced adaptive and intelligent intrusion detection systems (IDS) that leverage supervised and unsupervised learning techniques to identify anomalous behavior in real time (Saleem et al., 2020). Multiple studies highlight innovative cybersecurity-enhancing approaches. In Shala et al. (2017), optimized trust-based security in P2P M2M applications, while in Tanimu et al. (2024) authors utilized blockchain for collaborative intrusion detection. In Kolokotronis et al. (2022), advanced IoMT threat mitigation, complementing (Peratikou et al., 2021) work on federated cyber range networks and Gurung et al. (2022) feature elimination techniques for phishing detection.
In research work (Meng et al., 2021), researchers developed a computer-based system to estimate and predict drone movement without extra hardware. This greatly helps in the detection of GPS-spoofed signals. Similarly, Schmidt et al. (2020) employed multiple learning models to classify real and spoofed signals, improving detection accuracy and reducing false positive rates.
An ensemble learning approach is utilized in Shafique et al. (2021) where a voting classifier is used to classify spoofed GPS signals and shows robustness across different techniques. Meanwhile, Yoon et al. (2019) presents a simple ML-based approach for small drone attack detection. Researchers in Zhu et al. (2021) make use of support vector machines (SVM) for spoofed signal detection while in Dang et al. (2020), the authors focused on signal strength to enhance location accuracy while giving less importance to classifying real and spoofed signals. Furthermore, Khoei et al. (2022) compared multiple ML models for drone paths, affirming the efficacy of ML in detecting spoofed signals across various circumstances.
The implementation of deep learning (DL) techniques makes it easy to analyze the pattern of GPS spoofing. The DL technique is not only suitable for judging large-scale patterns and making decisions but it is also utilized for small drone attacks by Agyapong et al. (2021). By training the DL model to analyze the drone's movement and comparing it to expected patterns, researchers were able to detect inconsistencies. In Dang et al. (2022), the authors used DL models for signal strength detection and based on that accurately determined the drone's precise location and successfully classified real and spoofed signals. Moreover, DL models are being utilized for security applications other than drones. Researchers are investigating their use in protecting phasor measurement units (PMUs) from spoofing attacks (Almutairy et al., 2023), which shows the wide-ranging potential of deep neural networks (DNNs) in detecting and preventing anomalies across various applications.
Detection of fake GPS signals has become a top priority, particularly for drones. Researchers are investigating various techniques using multilayer perceptron (MLP). In Shafiee et al. (2017), researchers use the MLP model to check the timing and strength of the signal, for accurate identification of suspicious activity in drone security. In Jullian et al. (2022), researchers compared multiple learning models and found that the MLP attained better performance than all other models for accurately detecting spoofed signals in drones with over 80% accuracy. Furthermore, the usage of MLP models is not limited to drone signal spoofing detection but has also been used for cellular network tower signals (Dang et al., 2022). ML and DL models are further explored to combat fake GPS signals. For example, Sung et al. (2022) found that the ResNet model outperformed SVM in identifying fake signals. Another study Wu et al. (2023) developed a real-time cyberattack detection method using a combination of convolutional neural networks (CNN) and bidirectional long short-term memory (BiLSTM) models, achieving over 99% accuracy in simulations.
Despite several research efforts, there is still room for improvements in GPS detection and now the researchers are striving to design a framework that is not only accurate but also robust and less computationally complex for spoofing attack detection in small drones. Ensemble learning approaches are investigated that allow systems to learn from multiple models for enhanced adaptability as utilized in research works (Goudos and Athanasiadou, 2019; Rajadurai and Gandhi, 2020) for better-identifying attacks in wireless networks. This shows the importance of ensemble learning approaches in addressing complex spoofed GPS threats, as demonstrated by a framework that utilizes multiple models to detect spoofing in small drones (Sun et al., 2023).
Researchers are exploring a variety of ML and DL algorithms combined with a learning technique to find fake GPS signals in drones. These approaches show good results but have limitations and may not fully address all the different and complex ways, the intruders attack. Table 1 summarizes these studies and their limitations by following the pattern given in Ashraf et al. (2020). Table 1 describes the limitations of the existing work along with summarized details like the dataset, proposed model, and results.
This section provides a detailed explanation of the methodology employed in detecting GPS spoofing. The experiments incorporate DL and transfer learning models.
In chi-square feature selection, observed and expected values are key components used to determine the significance of the relationship between predicting features and the target variable in a dataset (Narra et al., 2022). Its application aids in discerning the most relevant features for predicting the target variable. The output of chi-square feature selection in our case is the selection of the best features among 13 features. The best results are obtained when we select the 8 features which are “DO, PRN, PD, CN0, PIP, RX, TOW, and LC.” The overview of the feature selection is provided here.
Observed values (O): Observed values are the actual frequencies or counts of occurrences observed in the data. When dealing with a feature and a target variable, the observed values represent the number of times a particular combination of feature value and target value occurs in the dataset.
Expected values (E): Expected values are the theoretical frequencies that would be expected if there were no association between the feature and the target variable. These values are calculated based on the assumption that the feature and the target variable are independent.
Calculating expected values: The expected value for a specific cell in a contingency table (which cross-tabulates the feature and target values) can be calculated using the formula:
Where
• Eij is the expected frequency for the cell in the ith row and jth column.
• Ri is the total number of observations in the ith row.
• Cj is the total number of observations in the jth column.
• N is the total number of observations in the dataset.
The critical value for evaluating correlation hypotheses in the chi-square contingency table is determined by the chi-square distribution and depends on two key factors:
Degrees of freedom (df): This is calculated based on the dimensions of the contingency table. For a contingency table with r rows and c columns, the degrees of freedom are given by:
Significance level (α): This is the probability threshold below which the null hypothesis (that there is no association between the variables) is rejected. Commonly used significance levels are 0.05 (5%) and 0.01 (1%).
Basis for setting the critical value
To set the critical value, you follow these steps:
i Determine the degrees of freedom: Calculate the degrees of freedom for the contingency table. For example, if you have a 3 × 2 table, the degrees of freedom would be:
ii Choose a significance level: Decide on the significance level you want to use. The most commonly used level is 0.05. This means you are accepting a 5% chance of rejecting the null hypothesis when it is actually true (Type I error).
iii Find the critical value from the chi-square distribution table: Using the degrees of freedom and the chosen significance level, you can look up the critical value in the chi-square distribution table.
Advanced ML has shown substantial potential across diverse medical sectors, encompassing the prognosis and diagnosis of various health conditions. In the context of predicting GPS spoofing attacks, sophisticated algorithms facilitate the thorough analysis of extensive data related to system behavior, contributing to the early and precise identification of potential threats. The effectiveness of these models heavily depends on the quality and quantity of the training data used to develop them. The availability of varied and representative datasets proves crucial in constructing reliable predictive models for GPS spoofing attack detection. This research employs a variety of advanced machine learning architectures, including CNN, MLP, ResNET, long short-term memory (LSTM), Inception, EfficientNetB4, MobileNet, and Xception.
An advanced form of the feed-forward neural network model that has more than one layer of neurons is called MLP (Juna et al., 2022). After passing through the input layers and hidden layers that introduce different degrees of abstraction, information is finally combined into predictions at the output layer. Three main layers are usually included in an MLP model: input, hidden, and output. In this instance, 64 neurons make up the hidden layer and 32 neurons are integrated with rectified linear unit (ReLU) activation in the input layer. The output layer uses a dropout layer (rate: 0.2) in conjunction with a single neuron that has a sigmoid activation function. Throughout 100 epochs, the Adam optimizer and a binary cross-entropy loss function are used for training.
CNNs are recognized as resilient deep neural networks, adept at handling data preprocessing and computational complexities (Alturki et al., 2023). Essential elements encompass convolutional, pooling, flattening, activation, and dropout layers. Convolutional layers extract distinctive features from input images, and pooling layers diminish feature sizes to prevent overfitting. The activation function, ReLU, introduces non-linearity, while dropout layers help counteract overfitting. The model integrates max-pooling and dropout with rates of 0.2 and 0.5, respectively, for enhanced efficiency.
LSTMs demonstrate proficiency in capturing prolonged temporal dependencies, a crucial aspect in tackling various learning challenges linked with sequential data (Cascone et al., 2023). The gate mechanisms embedded within LSTM cells effectively regulate information flow, enabling efficient utilization of context. Despite concerns regarding the complexity of its architecture, LSTM's effectiveness in analyzing sequential data is widely acknowledged.
Aligned with the techniques outlined above, the principles of transfer learning and few-shot learning highlight the significance of transferring prior knowledge from a source task to a few-shot task. Two predominant transfer learning approaches encompass fine-tuning solely the classifier layers while maintaining the fixed weights of other model layers, and fine-tuning all layers to permit comprehensive weight adjustments.
ResNet introduces residual connections, which maintain layer weights during the backpropagation process (Wang et al., 2019). ResNet variations, such as ResNet101, ResNet152, and ResNet50, are differentiated by their layer count, prioritizing depth over width to achieve parameter efficiency.
EfficientNet utilizes compound scaling to harmonize network width, depth, and resolution, thereby improving both accuracy and efficiency (Zulfiqar et al., 2023). Variants such as B0, B3, and B4 incorporate Softmax activation and Adam optimization, utilizing convolutional layer weights derived from ImageNet.
An improved version of Inception-V1, Inception-V3 has a large architecture and maximizes network depth (Mujahid et al., 2022). The last layer is modified to make task-specific modifications while the earlier layers are preserved in transfer learning.
Xception uses depthwise and pointwise separable convolutions, taking inspiration from InceptionV3 (Salim et al., 2023). Xception is a computationally efficient model with a depth of 71 layers and a parameter count of 22.9 million, but it requires large datasets for efficient training.
A CNN architecture called MobileNet was created to operate effectively on mobile devices. It makes use of an inverted residual structure with bottleneck layer residual connections. MobileNetV2 tackles information degradation in deep networks by utilizing inverted residual bottleneck layers (Srinivasu et al., 2021). Its specifications include 32 initial filters, 19 bottleneck layers, dropout, batch normalization, and a kernel size of 3 × 3. Segmentable by Depth Convolution efficiently reduces processing and model size by splitting the convolution process into depthwise and pointwise convolutions. By adjusting the number of channels, the Width Multiplier option is intended to further reduce computing costs. The resolution multiplier affects both computing cost and model size simultaneously by giving control over picture resolution. Together, these methods help to maximize the convolutional neural network architecture's effectiveness and resource use.
This research work proposes an innovative approach for GPS spoofed signals detection utilizing chi-square significant features and a MobileNet transfer learning model. The dataset is based on 13 features of signals that are gathered in a controlled simulated environment. The experiments are conducted using two scenarios with and without chi-square significant features. Results reveal that utilization of feature extraction technique as pre-processing gives notably better results with all learning models especially MobileNet for securing small drones. The proposed approach of this research is presented in Figure 1.
The proposed methodology begins with the utilization of a GPS spoofing dataset, which includes data on both spoofed and legitimate signals under various conditions, such as urban and rural environments and different weather scenarios. This dataset is then subjected to a data preprocessing stage, where noise and irrelevant entries are removed, missing values are handled, and the data is normalized to ensure consistency and uniformity. The cleaned dataset is then processed using the Chi-square feature selection technique to identify the most statistically significant features relevant to distinguishing spoofed signals from legitimate ones. This step reduces computational complexity while enhancing the model's focus on the critical aspects of the data.
Following feature selection, the dataset is split into training and testing subsets with a 70%–30% split. The training data is used to train the MobileNet model, leveraging transfer learning techniques to adapt pre-trained weights for the task of GPS spoofing detection. Once trained, the model is evaluated using the reserved testing dataset to assess its performance on unseen data. Key performance metrics, such as accuracy, precision, recall, and F1 score are computed to quantify the model's effectiveness.
This section performs a thorough examination of the performance demonstrated by the MobileNet model on a dataset related to GPS spoofing. This study applies a variety of DL and transfer learning models for identifying GPS spoofing attacks. In order to train the predictive models, 70% of the dataset is used as training data while 30% is used for testing the trained models. Model performance evaluation utilizes a variety of metrics. All experiments are conducted within a Python environment, leveraging various libraries. A comprehensive assessment is conducted, involving various metrics together. These metrics function as evaluation criteria to determine the effectiveness of the model compared to established methodologies as presented under:
where TP, TN, FP, and FN denote true positive, true negative, false positive, and false negative, respectively.
This research work used a collection of genuine and spoofed GPS signals (dataset) (Aissou et al., 2022). Genuine signals are gathered from different places involving both stationary and moving vehicles. While collecting the data, 13 different features are obtained from 8 different parts of the device at different stages (like tracking, figuring out location, and finding the signal). They also created fake signals in three different ways: simple, medium, and hard. In total, they collected 158,170 records of data, with an equal mix of real and fake signals including all three difficulty levels of fake signals. Table 2 describes each of the 13 features of the dataset.
Effective preprocessing of the data improves the accuracy of features and model performance. The chi-square approach is used in the analysis, cleaning, and feature selection phases of data preparation. To begin, the .shape() function is used to inspect the size and general details of the GPS spoofing dataset like the number of rows and columns. The use of the .info() function yields data types and the number of missing values for variables. After that, the distributions of variables are explored. After that, the dataset is examined for missing values, and Chi-square feature selection is used to maximize pertinent features.
A thorough examination contrasts the performance of transfer learning and DL classifiers utilizing the entire feature set of the GPS spoofing detection dataset. While certain classifiers exhibit suboptimal performance, others surpass expectations. This study employs transfer learning and DL models for GPS spoofing detection. Assessment of these models' performance using all 13 features is shown in Table 3.
As per the findings, Xception surpasses others when utilizing all features, attaining an accuracy of 91.88%, along with 91.25% recall, 90.43% precision, and a 91.05% F1 score. MobileNet achieves an accuracy of 90.78% and an F-Score of 91.02%. The Inception classifier records an accuracy of 87.78%, with recall at 89.31%, precision at 88.42%, and an F-Score of 88.87%. The CNN achieves an accuracy score of 80.43%. However, LSTM demonstrates the lowest performance for GPS spoofing prediction, with an accuracy of 78.43%, recall of 79.79%, a precision of 78.37%, and an F-Score of 79.38%.
This research also illustrates the significance of features by utilizing selective features through Chi-square for feature selection. The best results are obtained when we select the 8 best features which are “DO, PRN, PD, CN0, PIP, RX, TOW, and LC.” The outcomes of DL and transfer learning models are presented in Table 4. The MLP model outperformed CNN and LSTM, achieving an accuracy of 89.49%, precision of 83.78%, recall of 82.74%, and an F1 score of 82.35%. Similarly, the other models have their corresponding performance metrics listed in the table. The Inception classifier secures a 95.07% recall, a 94.85% F1-Score, 94.38% precision, and 92.67% accuracy. The deep learning model CNN records an accuracy score of 86.36%. However, LSTM exhibits the lowest performance for GPS spoofing prediction, with an accuracy of 82.45%, recall of 81.96%, precision of 84.68%, and an F-Score of 82.48%. MobileNet demonstrated superior performance, surpassing all other models with an accuracy of 98.49%, precision of 99.13%, recall of 99.27%, and an impressive F1 score of 99.20% on features selected through Chi-square.
Measures are implemented to validate the reliability of the model through the utilization of K-fold cross-validation. The outcomes of 5-fold cross-validation are presented in Table 5, revealing the superior performance of the suggested framework compared to other models in terms of recall, accuracy, precision, and F1 score. The minimal standard deviation indicates a stable and consistent performance of the proposed model. These outcomes support the robustness, dependability, and reliability of the suggested strategy by confirming its consistently good performance across several folds.
Figure 2 compares the performance of models evaluated on all 13 features vs. Chi-square-based selective features. It can be observed that utilizing selective features which are statistically more significant, produces better accuracy. Chi-squares-based features proved to be more effective in training DL and transfer learning models and showed better performance.
In the analysis of results utilizing all features, the transfer learning model Xception emerged as the top-performing classifier, achieving an accuracy of 90.88%. This outcome suggests that leveraging transfer learning with Xception can significantly enhance GPS spoofing detection when considering the complete feature set. Notably, MobileNet also demonstrated competitive performance with an accuracy of 89.67%, indicating its effectiveness in this context. However, conventional deep learning models, such as CNN, exhibited comparatively lower accuracy, emphasizing the advantage of transfer learning approaches. When examining results utilizing selective features through Chi-square, the performance dynamics shifted. The MLP model outperformed CNN and LSTM, achieving an accuracy of 88.38%. This notable increase in the accuracy of spoofed signal detection shows the importance of chi-square significant features. In this scenario of utilizing chi-square features, InceptionNet and MobileNet both transfer learning models show great improvement in terms of accuracy, f1-score, recall, and precision. These early and accurate results with significant features show that this framework works well in the real-world environment. Figure 2 provides a visual depiction of the performance differences, aiding in a thorough grasp of the classifiers' effectiveness in diverse scenarios.
The proposed method, while effective for small drones, may face scalability challenges when applied to large-scale drone networks with varying hardware capabilities and operational complexities. Furthermore, the dataset used in this study, though diverse, may not fully encompass all real-world scenarios, such as extreme weather conditions or complex urban environments with high GPS interference.
In this study, an innovative approach is proposed for global positioning system (GPS) spoofing signal detection. The dataset analyzed in this research work for GPS spoofed signals detection is gathered in a controlled simulation environment. The uniqueness of this research work lies in the usage of significant chi-square features for accurate and early detection of spoofed signals. Subsequently, a transfer learning model was developed to identify spoofed GPS signals. Confusion matrices are a reliable assessment tool that was essential in determining the computational efficiency of the model. Experimental results highlight the exceptional performance of the proposed model, achieving an impressive accuracy rate of 98.49%. Notably, actual GPS spoofing signal data were employed, preserving crucial data features essential for GPS signal manipulation, thereby enhancing reliability compared to simulation-based datasets. The proposed MobileNet demonstrated significant efficacy in identifying spoofing actions in drones. Future work will explore and implement adversarial training techniques to enhance the model's robustness against sophisticated spoofing attacks.
The original contributions presented in the study are included in the article/supplementary material, further inquiries can be directed to the corresponding author.
AH: Conceptualization, Data curation, Writing – original draft. MS: Data curation, Formal analysis, Writing – original draft. RA: Methodology, Software, Writing – original draft. AA: Investigation, Visualization, Writing – original draft. IA: Supervision, Validation, Writing – review & editing.
The author(s) declare that no financial support was received for the research, authorship, and/or publication of this article.
The authors declare that the research was conducted in the absence of any commercial or financial relationships that could be construed as a potential conflict of interest.
The author(s) declare that no Generative AI was used in the creation of this manuscript.
All claims expressed in this article are solely those of the authors and do not necessarily represent those of their affiliated organizations, or those of the publisher, the editors and the reviewers. Any product that may be evaluated in this article, or claim that may be made by its manufacturer, is not guaranteed or endorsed by the publisher.
Agyapong, R. A., Nabil, M., Nuhu, A.-R., Rasul, M. I., and Homaifar, A. (2021). “Efficient detection of GPS spoofing attacks on unmanned aerial vehicles using deep learning,” in 2021 IEEE Symposium Series on Computational Intelligence (SSCI) (IEEE). doi: 10.1109/SSCI50451.2021.9659972
Aissou, G. A., Benouadah, S. B., EL ALAMI, H. E. A., and Kaabouch, N. K. (2022). A dataset for gps spoofing detection on autonomous vehicles. IEEE Dataport.
Almutairy, F., Scekic, L., Matar, M., Elmoudi, R., and Wshah, S. (2023). Detection and mitigation of GPS spoofing attacks on phasor measurement units using deep learning. Int. J. Electr. Power Energy Syst. 151:109160. doi: 10.1016/j.ijepes.2023.109160
Alturki, N., Umer, M., Ishaq, A., Abuzinadah, N., Alnowaiser, K., Mohamed, A., et al. (2023). Combining cnn features with voting classifiers for optimizing performance of brain tumor classification. Cancers 15:1767. doi: 10.3390/cancers15061767
Arafat, M. Y., Alam, M. M., and Moh, S. (2023). Vision-based navigation techniques for unmanned aerial vehicles: review and challenges. Drones 7:89. doi: 10.3390/drones7020089
Ashraf, S., and Ahmed, T. (2020). “Sagacious intrusion detection strategy in sensor network,” in 2020 International Conference on UK-China Emerging Technologies (UCET), 1–4. doi: 10.1109/UCET51115.2020.9205412
Ashraf, S., Alfandi, O., Ahmad, A., Khattak, A. M., Hayat, B., Kim, K. H., et al. (2020). Bodacious-instance coverage mechanism for wireless sensor network. Wirel. Commun. Mobile Comput. 2020:8833767. doi: 10.1155/2020/8833767
Balador, A., Kouba, A., Cassioli, D., Foukalas, F., Severino, R., Stepanova, D., et al. (2018). Wireless communication technologies for safe cooperative cyber physical systems. Sensors 18:4075. doi: 10.3390/s18114075
Benarbia, T., and Kyamakya, K. (2021). A literature review of drone-based package delivery logistics systems and their implementation feasibility. Sustainability 14:360. doi: 10.3390/su14010360
Cascone, L., Sadiq, S., Ullah, S., Mirjalili, S., Siddiqui, H. U. R., and Umer, M. (2023). Predicting household electric power consumption using multi-step time series with convolutional lstm. Big Data Res. 31:100360. doi: 10.1016/j.bdr.2022.100360
Dang, Y., Benzaid, C., Shen, Y., and Taleb, T. (2020). “GPS spoofing detector with adaptive trustable residence area for cellular based-UAVs,” in GLOBECOM 2020-2020 IEEE Global Communications Conference (IEEE). doi: 10.1109/GLOBECOM42002.2020.9348030
Dang, Y., Benzaid, C., Yang, B., and Taleb, T. (2021). “Deep learning for GPS spoofing detection in cellular-enabled UAV systems,” in 2021 International Conference on Networking and Network Applications (NaNA) (IEEE). doi: 10.1109/NaNA53684.2021.00093
Dang, Y., Benzaid, C., Yang, B., Taleb, T., and Shen, Y. (2022). Deep-ensemble-learning-based GPS spoofing detection for cellular-connected UAVs. IEEE Internet Things J. 9, 25068–25085. doi: 10.1109/JIOT.2022.3195320
Feng, Z., Guan, N., Lv, M., Liu, W., Deng, Q., Liu, X., et al. (2020). Efficient drone hijacking detection using two-step ga-xgboost. J. Syst. Archit. 103:101694. doi: 10.1016/j.sysarc.2019.101694
Goudos, S. K., and Athanasiadou, G. (2019). Application of an ensemble method to UAV power modeling for cellular communications. IEEE Antennas Wirel. Propag. Lett. 18, 2340–2344. doi: 10.1109/LAWP.2019.2926784
Gurung, G., Bendiab, G., Shiaele, M., and Shiaeles, S. (2022). “CIDS: collaborative intrusion detection system using blockchain technology,” in 2022 IEEE International Conference on Cyber Security and Resilience (CSR) (IEEE), 125–130. doi: 10.1109/CSR54599.2022.9850331
Jiang, P., Wu, H., and Xin, C. (2022). Deeppose: detecting GPS spoofing attack via deep recurrent neural network. Dig. Commun. Netw. 8, 791–803. doi: 10.1016/j.dcan.2021.09.006
Jullian, O., Otero, B., Stojilović, M., Costa, J. J., Verdú, J., and Pajuelo, M. A. (2022). “Deep learning detection of GPS spoofing,” in Machine Learning, Optimization, and Data Science (Springer International Publishing), 527–540. doi: 10.1007/978-3-030-95467-3_38
Juna, A., Umer, M., Sadiq, S., Karamti, H., Eshmawi, A., Mohamed, A., et al. (2022). Water quality prediction using knn imputer and multilayer perceptron. Water 14:2592. doi: 10.3390/w14172592
Khoei, T. T., Ismail, S., and Kaabouch, N. (2022). Dynamic selection techniques for detecting GPS spoofing attacks on UAVs. Sensors 22:662. doi: 10.3390/s22020662
Kim, J., Lee, S., and Jung, M. (2021). Case study on the user interface of GPS plotters to enhance their usability. J. Mar. Sci. Eng. 9, 57. doi: 10.3390/jmse9010057
Kolokotronis, N., Dareioti, M., Shiaeles, S., and Bellini, E. (2022). “An intelligent platform for threat assessment and cyber-attack mitigation in iomt ecosystems,” in 2022 IEEE Globecom Workshops (GC Wkshps) (IEEE), 541–546. doi: 10.1109/GCWkshps56602.2022.10008548
Kwon, K.-C., and Shim, D.-S. (2020). Performance analysis of direct GPS spoofing detection method with ahrs/accelerometer. Sensors 20:954. doi: 10.3390/s20040954
Liang, C., Miao, M., Ma, J., Yan, H., Zhang, Q., Li, X., et al. (2019). “Detection of GPS spoofing attack on unmanned aerial vehicle system,” in Machine Learning for Cyber Security: Second International Conference, ML4CS 2019, Xi'an, China, September 19–21, 2019, Proceedings 2 (Springer), 123–139. doi: 10.1007/978-3-030-30619-9_10
Liu, Y., Li, S., Fu, Q., and Liu, Z. (2018). Impact assessment of GNSS spoofing attacks on INS/GNSS integrated navigation system. Sensors 18:1433. doi: 10.3390/s18051433
Manesh, M. R., Kenney, J., Hu, W. C., Devabhaktuni, V. K., and Kaabouch, N. (2019). “Detection of GPS spoofing attacks on unmanned aerial systems,” in 2019 16th IEEE Annual Consumer Communications &Networking Conference (CCNC) (IEEE), 1–6. doi: 10.1109/CCNC.2019.8651804
Mehdi, M. A., Zukhraf, S. Z. N., and Maryam, H. (2022). “Analysis of vulnerabilities in cybersecurity in unmanned air vehicles,” in Computational Intelligence for Unmanned Aerial Vehicles Communication Networks (Springer), 131–143. doi: 10.1007/978-3-030-97113-7_8
Meng, L., Yang, L., Ren, S., Tang, G., Zhang, L., Yang, F., et al. (2021). An approach of linear regression-based UAV GPS spoofing detection. Wirel. Commun. Mobile Comput. 2021, 1–16. doi: 10.1155/2021/5517500
Mohsan, S. A. H., Othman, N. Q. H., Li, Y., Alsharif, M. H., and Khan, M. A. (2023). Unmanned aerial vehicles (UAVS): practical aspects, applications, open challenges, security issues, and future trends. Intell. Serv. Robot. 16, 109–137. doi: 10.1007/s11370-022-00452-4
Moshref-Javadi, M., and Winkenbach, M. (2021). Applications and research avenues for drone-based models in logistics: a classification and review. Expert Syst. Appl. 177:114854. doi: 10.1016/j.eswa.2021.114854
Motlagh, N. H., Taleb, T., and Arouk, O. (2016). Low-altitude unmanned aerial vehicles-based internet of things services: comprehensive survey and future perspectives. IEEE Internet Things J. 3, 899–922. doi: 10.1109/JIOT.2016.2612119
Mujahid, M., Rustam, F., Álvarez, R., Luis Vidal Mazón, J., Díez, I. T., and Ashraf, I. (2022). Pneumonia classification from x-ray images with inception-v3 and convolutional neural network. Diagnostics 12:1280. doi: 10.3390/diagnostics12051280
Mykytyn, P., Brzozowski, M., Dyka, Z., and Langendoerfer, P. (2023). “GPS-spoofing attack detection mechanism for UAV swarms,” in 2023 12th Mediterranean Conference on Embedded Computing (MECO) (IEEE). doi: 10.1109/MECO58584.2023.10154998
Narra, M., Umer, M., Sadiq, S., Karamti, H., Mohamed, A., Ashraf, I., et al. (2022). Selective feature sets based fake news detection for COVID-19 to manage infodemic. IEEE Access 10, 98724–98736. doi: 10.1109/ACCESS.2022.3206963
Peratikou, A., Louca, C., Shiaeles, S., and Stavrou, S. (2021). “On federated cyber range network interconnection,” in Selected Papers from the 12th International Networking Conference: INC 2020 12 (Springer), 117–128. doi: 10.1007/978-3-030-64758-2_9
Prasanna Srinivasan, S., and Sathyadevan, S. (2023). “GPS spoofing detection in UAV using motion processing unit,” in 2023 11th International Symposium on Digital Forensics and Security (ISDFS) (IEEE), 1–4. doi: 10.1109/ISDFS58141.2023.10131729
Qiao, Y., Zhang, Y., and Du, X. (2017). “A vision-based GPS-spoofing detection method for small UAVs,” in 2017 13th International Conference on Computational Intelligence and Security (CIS) (IEEE). doi: 10.1109/CIS.2017.00074
Rajadurai, H., and Gandhi, U. D. (2020). A stacked ensemble learning model for intrusion detection in wireless network. Neural Comput. Applic. 34, 15387–15395. doi: 10.1007/s00521-020-04986-5
Saleem, S., Ashraf, S., and Basit, M. K. (2020). CMBA-a candid multi-purpose biometric approach. ICTACT J. Image Video Proc. 11, 2211–2216. doi: 10.21917/ijivp.2020.0317
Salim, F., Saeed, F., Basurra, S., Qasem, S. N., and Al-Hadhrami, T. (2023). Densenet-201 and xception pre-trained deep learning models for fruit recognition. Electronics 12:3132. doi: 10.3390/electronics12143132
Schmidt, E., Gatsis, N., and Akopian, D. (2020). A GPS spoofing detection and classification correlator-based technique using the lasso. IEEE Trans. Aerosp. Electron. Syst. 56, 4224–4237. doi: 10.1109/TAES.2020.2990149
Shafiee, E., Mosavi, M. R., and Moazedi, M. (2017). Detection of spoofing attack using machine learning based on multi-layer neural network in single-frequency GPS receivers. J. Navigat. 71, 169–188. doi: 10.1017/S0373463317000558
Shafique, A., Mehmood, A., and Elhadef, M. (2021). Detecting signal spoofing attack in UAVS using machine learning models. IEEE Access 9, 93803–93815. doi: 10.1109/ACCESS.2021.3089847
Shala, B., Wacht, P., Trick, U., Lehmann, A., Ghita, B., and Shiaeles, S. (2017). “Trust integration for security optimisation in p2p-based m2m applications,” in 2017 IEEE Trustcom/BigDataSE/ICESS (IEEE), 949–954. doi: 10.1109/Trustcom/BigDataSE/ICESS.2017.335
Souli, N., Kolios, P., and Ellinas, G. (2021). Online relative positioning of autonomous vehicles using signals of opportunity. IEEE Trans. Intell. Vehic. 7, 873–885. doi: 10.1109/TIV.2021.3124727
Srinivasu, P. N., SivaSai, J. G., Ijaz, M. F., Bhoi, A. K., Kim, W., and Kang, J. J. (2021). Classification of skin disease using deep learning neural networks with mobilenet v2 and LSTM. Sensors 21:2852. doi: 10.3390/s21082852
Sun, Y., Yu, M., Wang, L., Li, T., and Dong, M. (2023). A deep-learning-based GPS signal spoofing detection method for small UAVs. Drones 7:370. doi: 10.3390/drones7060370
Sung, Y.-H., Park, S.-J., Kim, D.-Y., and Kim, S. (2022). GPS spoofing detection method for small UAVs using 1D convolution neural network. Sensors 22:9412. doi: 10.3390/s22239412
Tanimu, J., Shiaeles, S., and Adda, M. (2024). A comparative analysis of feature eliminator methods to improve machine learning phishing detection. J. Data Sci. Intell. Syst. 2, 87–99. doi: 10.47852/bonviewJDSIS32021736
Varshosaz, M., Afary, A., Mojaradi, B., Saadatseresht, M., and Parmehr, E. G. (2019). Spoofing detection of civilian UAVs using visual odometry. ISPRS Int. J. Geo-Inf. 9:6. doi: 10.3390/ijgi9010006
Wang, Z.-Y., Xia, Q.-M., Yan, J.-W., Xuan, S.-Q., Su, J.-H., and Yang, C.-F. (2019). Hyperspectral image classification based on spectral and spatial information using multi-scale resnet. Appl. Sci. 9:4890. doi: 10.3390/app9224890
Wu, S., Li, Y., Wang, Z., Tan, Z., and Pan, Q. (2023). A highly interpretable framework for generic low-cost UAV attack detection. IEEE Sens. J. 23, 7288–7300. doi: 10.1109/JSEN.2023.3244831
Yoon, H.-J., Wan, W., Kim, H., Hovakimyan, N., Sha, L., and Voulgaris, P. G. (2019). Towards resilient UAV: escape time in GPS denied environment with sensor drift. IFAC-PapersOnLine 52, 423–428. doi: 10.1016/j.ifacol.2019.11.280
Zhu, X., Hua, T., Yang, F., Tu, G., and Chen, X. (2021). Global positioning system spoofing detection based on support vector machines. IET Radar, Sonar Navig. 16, 224–237. doi: 10.1049/rsn2.12178
Keywords: internet of everything, cyber security, GPS signal spoofing, intrusion detection, machine learning
Citation: Hakeem A, Sabir M, Alhebshi RM, Almakky AG and Ashraf I (2025) Integrating artificial intelligence for improved security of IoT-drones through cyber-physical attack detection. Front. Comput. Sci. 7:1545282. doi: 10.3389/fcomp.2025.1545282
Received: 14 December 2024; Accepted: 07 February 2025;
Published: 20 February 2025.
Edited by:
Aikaterini Kanta, University of Portsmouth, United KingdomReviewed by:
Rajkumar Singh Rathore, Cardiff Metropolitan University, United KingdomCopyright © 2025 Hakeem, Sabir, Alhebshi, Almakky and Ashraf. This is an open-access article distributed under the terms of the Creative Commons Attribution License (CC BY). The use, distribution or reproduction in other forums is permitted, provided the original author(s) and the copyright owner(s) are credited and that the original publication in this journal is cited, in accordance with accepted academic practice. No use, distribution or reproduction is permitted which does not comply with these terms.
*Correspondence: Imran Ashraf, aW1yYW5hc2hyYWZAeW51LmFjLmty
Disclaimer: All claims expressed in this article are solely those of the authors and do not necessarily represent those of their affiliated organizations, or those of the publisher, the editors and the reviewers. Any product that may be evaluated in this article or claim that may be made by its manufacturer is not guaranteed or endorsed by the publisher.
Research integrity at Frontiers
Learn more about the work of our research integrity team to safeguard the quality of each article we publish.