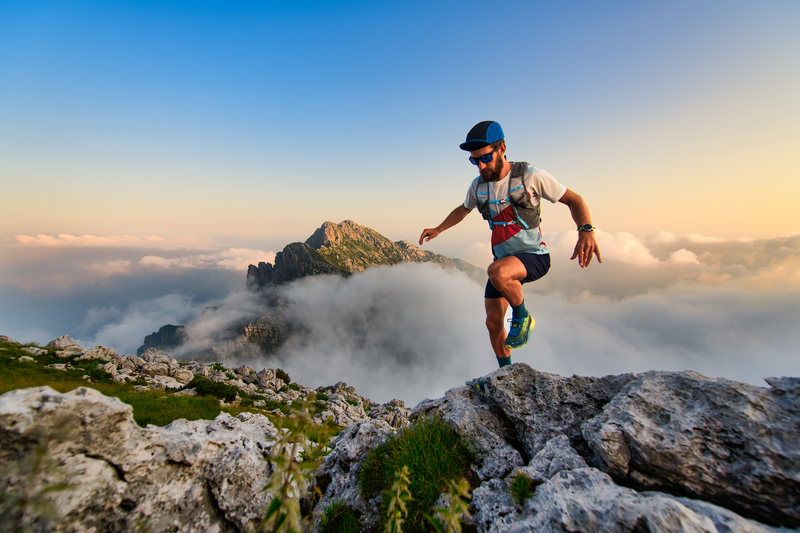
95% of researchers rate our articles as excellent or good
Learn more about the work of our research integrity team to safeguard the quality of each article we publish.
Find out more
ORIGINAL RESEARCH article
Front. Comput. Sci.
Sec. Computer Security
Volume 7 - 2025 | doi: 10.3389/fcomp.2025.1517346
This article is part of the Research Topic Cyber Security Prevention, Defenses Driven by AI, and Mathematical Modelling and Simulation Tools View all 5 articles
The final, formatted version of the article will be published soon.
You have multiple emails registered with Frontiers:
Please enter your email address:
If you already have an account, please login
You don't have a Frontiers account ? You can register here
Audio event detection, the application of scientific methods to analyze audio recordings, can be helpful in examining and analyzing audio recordings to preserve, analyze, and interpret sound evidence.Furthermore, it can be helpful in safety and compliance, security, surveillance, maintenance and predictive analysis. Audio event detection aims to recover meaningful information from audio recordings, such as determining the authenticity of the recording, identifying the speakers, and reconstructing conversations.However, filtering out noise for better accuracy in audio event detection is a major challenge. A greater sense of public security can be achieved by developing automated event detection systems that are both cost-effective and real-time. In response to these challenges, this study presented a method for identifying anomalous events based on noisy audio evidence and a real-time scenario to help the audio event detection investigator during the investigation. This study created a large audio dataset containing both noisy and original audio. The dataset includes diverse environmental background settings (e.g., office, restaurant, and park) and some abnormal events (e.g., explosion, car crash, and human attack). This study used an ensemble learning model to conduct experiments in an active learning environment. Nine methods are employed to create the feature vector. The experiments show that the proposed ensemble learning model using the active learning settings obtained an accuracy score of 99.26%, while the deep learning model obtained an accuracy of 95.35%. The proposed model was tested using noisy audio evidence and a real-time scenario. The experiment results show that the proposed approach can efficiently detect abnormal events from noisy audio evidence and a real-time scenario in real-time.
Keywords: Audio event detection, Forensics investigation, deep learning, machine learning, Noisy data
Received: 25 Oct 2024; Accepted: 04 Apr 2025.
Copyright: © 2025 Iqbal, Abbasi, Almadhor, Alsubai and Gregus. This is an open-access article distributed under the terms of the Creative Commons Attribution License (CC BY). The use, distribution or reproduction in other forums is permitted, provided the original author(s) or licensor are credited and that the original publication in this journal is cited, in accordance with accepted academic practice. No use, distribution or reproduction is permitted which does not comply with these terms.
* Correspondence:
Ahmad Almadhor, Jouf University, Sakakah, Saudi Arabia
Disclaimer: All claims expressed in this article are solely those of the authors and do not necessarily represent those of their affiliated organizations, or those of the publisher, the editors and the reviewers. Any product that may be evaluated in this article or claim that may be made by its manufacturer is not guaranteed or endorsed by the publisher.
Research integrity at Frontiers
Learn more about the work of our research integrity team to safeguard the quality of each article we publish.