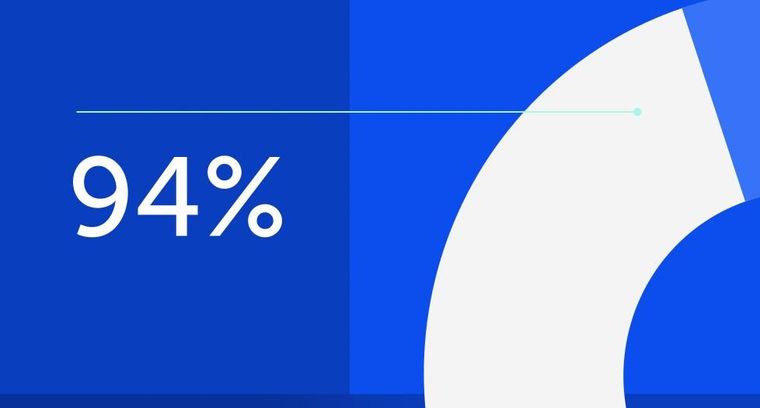
94% of researchers rate our articles as excellent or good
Learn more about the work of our research integrity team to safeguard the quality of each article we publish.
Find out more
ORIGINAL RESEARCH article
Front. Comput. Sci.
Sec. Computer Vision
Volume 7 - 2025 | doi: 10.3389/fcomp.2025.1437084
The final, formatted version of the article will be published soon.
Select one of your emails
You have multiple emails registered with Frontiers:
Notify me on publication
Please enter your email address:
If you already have an account, please login
You don't have a Frontiers account ? You can register here
Driver drowsiness or fatigue is among the most important factors that cause traffic accidents; therefore, a monitoring system is necessary to detect the state of a driver drowsiness or fatigue. In this paper, an automated vision-based system for real-time prediction of driver drowsiness or fatigue is presented, in which multiple visual ocular features such as eye closure, eyebrow shape, eye blinking, and other perfectly defined geometric facial features are employed as robust cues for driver's drowsiness. In addition, an efficient scheme is applied to extract local Gabor features of driver images based on Fisher's quantum information.A novel Fisher-Gabor descriptor (FGD) is then constructed from the extracted features, which is invariant to scale and rotation and also robust to changes in illumination, noise, and minor changes in viewpoint. The normalized FGDs are ultimately fed to a Latent Dynamic Conditional Random Field (LDCRF) classification model to predict whether the driver is drowsy/fatigued and a warning signal is thus issued (if required). A series of intensive experiments conducted on the benchmark NTHU-DDD video dataset show that the proposed system can predict driver drowsiness or fatigue effectively and efficiently, exceeding several state-of-the art alternatives by achieving a competitive detection accuracy
Keywords: Drowsy driving prediction, Fisher-Gabor facial features, LDCRF classification, NTHU-DDD dataset, Intelligent 5 Transportation Systems
Received: 23 May 2024; Accepted: 18 Mar 2025.
Copyright: © 2025 Bakheet, Al-Hamadi and Alanazi. This is an open-access article distributed under the terms of the Creative Commons Attribution License (CC BY). The use, distribution or reproduction in other forums is permitted, provided the original author(s) or licensor are credited and that the original publication in this journal is cited, in accordance with accepted academic practice. No use, distribution or reproduction is permitted which does not comply with these terms.
* Correspondence: Samy Bakheet, Prince Sattam Bin Abdulaziz University, Al-Kharj, Saudi Arabia
Disclaimer: All claims expressed in this article are solely those of the authors and do not necessarily represent those of their affiliated organizations, or those of the publisher, the editors and the reviewers. Any product that may be evaluated in this article or claim that may be made by its manufacturer is not guaranteed or endorsed by the publisher.
Supplementary Material
Research integrity at Frontiers
Learn more about the work of our research integrity team to safeguard the quality of each article we publish.