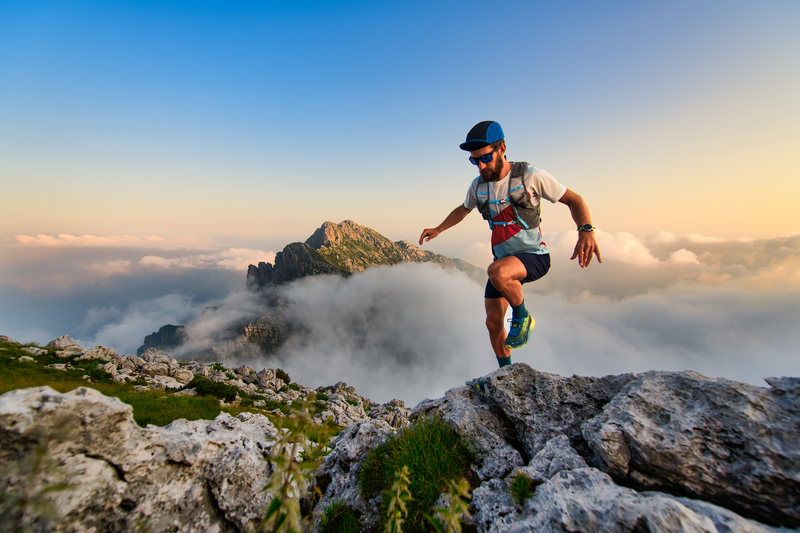
94% of researchers rate our articles as excellent or good
Learn more about the work of our research integrity team to safeguard the quality of each article we publish.
Find out more
REVIEW article
Front. Comput. Sci. , 06 January 2025
Sec. Human-Media Interaction
Volume 6 - 2024 | https://doi.org/10.3389/fcomp.2024.1521066
Leveraging Artificial Intelligence (AI) in decision support systems has disproportionately focused on technological advancements, often overlooking the alignment between algorithmic outputs and human expectations. A human-centered perspective attempts to alleviate this concern by designing AI solutions for seamless integration with existing processes. Determining what information AI should provide to aid humans is vital, a concept underscored by explainable AI's efforts to justify AI predictions. However, how the information is presented, e.g., the sequence of recommendations and solicitation of interpretations, is equally crucial as complex interactions may emerge between humans and AI. While empirical studies have evaluated human-AI dynamics across domains, a common vocabulary for human-AI interaction protocols is lacking. To promote more deliberate consideration of interaction designs, we introduce a taxonomy of interaction patterns that delineate various modes of human-AI interactivity. We summarize the results of a systematic review of AI-assisted decision making literature and identify trends and opportunities in existing interactions across application domains from 105 articles. We find that current interactions are dominated by simplistic collaboration paradigms, leading to little support for truly interactive functionality. Our taxonomy offers a tool to understand interactivity with AI in decision-making and foster interaction designs for achieving clear communication, trustworthiness, and collaboration.
Advances in Artificial Intelligence (AI) developments open new possibilities for supporting human decision making across a wide variety of applications. Decision making tasks in a broad range of applications share a process that starts when evidence is presented before making a decision within discrete choices, usually with follow-up effects. Within this framework, the decision-making process emerges as a scenario for human-AI teamwork where at a minimum two parties, i.e., the human and the AI, factor into finding a solution to the decision problem. The exact dynamics of how this collaboration occurs can vary from one situation to another, leading to multiple interaction options that range from simple recommendations to involved exchanges (Bansal et al., 2019; Lai et al., 2023; Bertrand et al., 2023). To bridge algorithmic suggestions and human expectations, embracing a human-centered approach in designing AI solutions is crucial to identify what information AI should provide to aid humans while ensuring a safe and transparent operation. It is equally crucial to understand how and when to best communicate the information for designing successful human-AI interactions. Explainable AI (XAI) expands model capabilities by providing not just so-called black box recommendations but also justifications tailored to end users' needs. The design of justifications from a human-centered perspective informs what AI models should deliver and it naturally involves careful thought on how to present this support, although it may not specifically address the ensuing interactions. The presentation style (how) and the strategic timing (when) for providing AI-generated insights are closely related to the type and sequence of interactions between humans with AI, which are ultimately enabled by the affordances of certain assistance elements (Lai et al., 2023). Despite the importance of and opportunities in interaction design within AI, there is not currently a common vocabulary to describe and differentiate these interactions.
Interactivity is a familiar concept to humans and widely studied in more specialized domains such as information visualization, interface and software design (Yi et al., 2007), and human-human interactions from a social perspective (Magnusson, 2018). Understanding interactions requires delving into multiple dimensions that involve subjects, modes, and purposes of interaction, and the context in which they take place (Schleidgen et al., 2023). Among these, interaction patterns emerge as sequences of behaviors that occur more often than by chance between agents and systems or artifacts. We have learned from these disciplines the importance of deliberate choices in selecting interaction types crucial for achieving specific goals, rather than imbuing unnecessary high levels of interactivity that do not result in better products (Sims, 1997). Likewise, finding the right balance of interactivity between humans and AI systems is not just a matter of enhancing user experience but is essential for achieving clear communication, trustworthiness, and meaningful collaboration. While current AI systems excel in offering problem-solving capabilities, there is often a disproportionate emphasis on the technological advancements, overlooking the critical aspects of user interface and experience. This oversight is apparent in many empirical studies in diverse domains and decision tasks (Lai et al., 2023; Bertrand et al., 2023), where interactions with AI agents are typically reduced to basic actions like menu selections or button clicks. However, the specific configurations needed to evaluate the effect of different AI assistance elements (or other context-related factors) on humans interacting with AI, can lead to multiple forms of collaboration or the actual interactions afforded.
Which forms of interactivity to incorporate in human-AI interactions is an open question and may depend on the overall context, emphasizing the need to deliberately study interaction patterns between humans and AI that can guide the development of better solutions. Psychology and social sciences have extensively studied human-human interactions, revealing patterns of reciprocal actions, such as “Question-Answer” and “Request-Offer” (Magnusson, 2018), which can also be applied in a setup where humans interact with AI. Likewise, the field of Human-Robot Interaction (HRI) has studied how humans and robots communicate, collaborate, and engage with each other, often through the analysis of observable patterns in their interactions (David et al., 2022; Sauppé and Mutlu, 2014; Ma and Cao, 2019). These patterns cover aspects of social interactions like greetings, attention, feedback, turn-taking, social cues, and farewell. All these elements foster a more natural and efficient communication experience, which can take place in AI technologies that incorporate natural language abilities. Other domains such as information visualization and user interface design have also developed taxonomies and libraries of interaction patterns based on different criteria such as user's intent, purpose, scope, abstraction level, and granularity (Yi et al., 2007; Silva-Rodŕıguez et al., 2020). These categorizations serve as a base to build on more comprehensive taxonomies grounded on and informed by evidence from empirical studies and incorporating knowledge from interaction design in other disciplines. In the context of AI, descriptions of possible interactions between humans and machines or AI have grouped them by user control and initiative (Van Berkel et al., 2021; Cheng et al., 2022), task nature (Parasuraman et al., 2000), and level of automation (Mackeprang et al., 2019). Existing classifications of human-AI interactions are often domain-specific, lacking a comprehensive framework that spans multiple domains. Without a shared vocabulary to describe human-AI interactions in decision-making tasks, it becomes difficult to compare approaches, aggregate findings, and synthesize knowledge across diverse studies, ultimately limiting the exploration of new interaction paradigms. This highlights the importance of a structured framework for categorizing different interaction patterns, helping designers understand and improve human-AI collaboration, and facilitating informed decisions in interaction design to enhance partnerships between humans and AI.
To address this gap, we first conducted an extensive systematic review on human-AI interactions that have been reported for human-AI decision making scenarios. We searched for relevant articles from five databases that cover Human-Computer Interaction (HCI) studies and related disciplines and selected 105 to conduct a detailed coding and analysis of the sequences of interactions that exist between humans and AI. Furthermore, we considered the task context and AI system involved in these empirical studies. Recent review works have characterized the design space in human-subject studies with AI for decision-making, providing insights about AI assistance elements (Lai et al., 2023) and methodologies to empirically evaluate human factors (Vereschak et al., 2021). XAI has been a main subject of reviews as well, not only to structure existing techniques (Sheu and Pardeshi, 2022), but also to investigate human factors that arise during decision making, including cognitive biases (Bertrand et al., 2022) and cognitive processes supported by interactive explanations (Bertrand et al., 2023). For our review, we selected empirical studies involving decision tasks where users are actively aware of the interaction and engaged in the decision-making process, as this focus was crucial for studying the dynamics of human-AI interactions. Grounded in the trends from our systematic review, we propose a taxonomy of interaction patterns that comprises seven interaction patterns that arise between humans and AI. To the best of our knowledge, there is not yet a comprehensive and structured classification of existing interaction patterns between humans and AI. We propose our taxonomy of interactions as a tool to better understand existing interaction patterns across domains and applications, allowing us to identify the occurrences of common interactions across multiple domains. We envision the use of taxonomy to foster dialogues on interactivity in AI-assisted decision making, encourage refinement and evaluation of novel patterns, and ultimately design better and more user-centered AI-based solutions.
This survey focuses on Human-AI interaction paradigms for explicit decision-making tasks, in contrast to proxy task where users are asked to simulate the AI outputs. Therefore, we aim to understand and evaluate the works that study human-AI interactions during decision-making tasks under AI assistance, instead of improvements of the model. Our survey covers studies conducted between 2013 and June 2023. Specifically, we searched within five databases: ACM Digital Library, IEEE Explore, Compendex, Scopus, and PubMed. The first four have extensive coverage of relevant studies in HCI covering conference proceedings and journal publications (Compendex and Scopus included papers from more subjects), while PubMed allowed us to capture research specifically related to medical applications of Human-AI interaction and decision-making. We were particularly interested in empirical studies with AI in the healthcare domain because of the need for human-subjects research in AI's development cycle and the importance of aligning algorithms with user needs and clinical workflows (Chen et al., 2022; van de Sande et al., 2024). The study of existing interactions between humans and AI support can suggest new directions and opportunities to enhance the experience and outcomes of using AI to support decision making in healthcare. We defined the search terms covering four dimensions: use of AI systems, human-AI interaction or collaboration studies, decision-making tasks, and interaction design. We included the last term since we wanted to focus on articles that evaluate interactions with AI systems during decision making tasks. The complete set of keywords used in our search can be found in the Appendix A. We defined the following inclusion criteria:
• The tasks in consideration are those related to decision-making, and in particular, we limit the study selection to those that implement complete decision making processes and not only evaluations of decision makers' perceptions, such as understanding, preferences, or judgments of AI's advice.
• The paper shows an implementation of the interface that was presented to human users to interact with AI.
• The modes of interaction encompass screen-based interfaces, virtual agents, and non-embodied setups.
• We have restricted our selection solely to papers featuring empirical user studies.
In addition to the inclusion criteria above, our search excluded studies in robotics and gaming by filtering out these keywords in the title and abstract. We excluded studies that involve robots because physical embodiment enables more dimensions of an interaction and those involving gaming scenarios because they are more complex, with less constraints to study how humans can interact with AI assistance. However, studies that implement decision making tasks through gamified tasks were included. Other survey papers or comments were also excluded by filtering out keywords in the title and abstract.
The initial search returned 3,770 papers, and 358 duplicates were found and deleted automatically. This left us with a total of 3,412 papers to screen. They were assigned to two authors to first go through title and abstract screening, followed by full-text screening. The screening phase was oriented toward the exclusion of papers that were not focused on human-AI interaction, i.e., limited to technical contributions, did not involve a complete decision-making task, were short papers (< 8 pages), involve gaming or robotics, have not been peer-reviewed, and were review or survey papers. We did not constrain our selection to works that directly manipulate the type of interaction between the human and AI. Our main interest was on which were the existing/available ways for humans to interact with AI agents in the evaluation of human-AI decision making. The total and abstract screening excluded 2,893 papers, and at the full-text review stage, 363 papers were filtered out. Lastly, 156 were considered for the information extraction stage, of which 51 were removed as a more detailed reading allowed us to identify that they did not satisfy the inclusion criteria of supporting actual AI-assisted decision making tasks. Appendix B summarizes the complete paper selection process. At the end, 105 articles were included in our review.
The data extraction template was developed by all authors and informed by previous surveys of empirical studies in human-AI interaction (Bertrand et al., 2023; Lai et al., 2023). Two authors distributed the final selected articles to be analyzed and coded the assigned articles independently. Then, one author checked the individual reports of each article to ensure consistency in the final extraction. Further discussions with the other authors took place to clarify discrepancies in the interactions or ambiguous cases. For the analysis of the interaction patterns, the authors reviewed the sequences of interactions and discussed how to group them into the design patterns that were repeated and are presented in this work. We iterated over the definition of each pattern to refine the actual components that constitute the interaction.
The following components comprised the extraction template:
We identified general information in which the decision making task takes place. This includes the domain and we adapted the categories initially proposed in this survey of AI-assisted decision making (Lai et al., 2023). Furthermore, we specified the decision making task to be completed by the human (e.g., detection of hate speech, sleep stage classification, price estimation, among others) and the level of expertise required to successfully complete the task.
As we are interested in humans interactions with AI agents, we retrieved the original goal of incorporating AI assistance in the decision making task, and briefly characterized the AI system used in the study. In particular, we extracted the technique supporting the AI's recommendations (whether a real model was used or the outcomes were simulated), its performance (if any evaluation metric was reported), and the terminology used to introduce the AI agent to participants in the user study. Further details such as data type and source, output type were not reported as the main focus of this survey is on the interactions rather than the type of AI methods as previously surveyed in Lai et al. (2023).
An interaction involves a reciprocal action or influence between two agents in the context of this survey (Schleidgen et al., 2023). To characterize this, we defined two elements: the action undertaken and the resulting output of that action. These two elements constitute the interaction building blocks that can be integrated into more complex interactions. In the definition of our taxonomy of human-AI interactions, we considered these building blocks as the main elements that constitute the interaction patterns. Our familiarity with prior studies on human-AI interactions served as an initial reference to ideate the (action - output) pairs available for the agents involved in the interaction, namely the human and AI parts. We further drew inspiration from existing interaction design patterns developed for prototyping human-robot interaction (Sauppé and Mutlu, 2014) and a taxonomy of interactivity techniques in XAI (Bertrand et al., 2023). We considered the actions listed in these works to characterize the interactions and functionality of explanations, respectively, and how they could be extended to agents involved in decision-making tasks. These building blocks primarily focus on the AI assisting the user in decision-making processes and are grouped based on the main action and specify the agent that can execute it. It is important to highlight that these building blocks can also occur sequentially, where one may be triggered in response to another. However, we have chosen to maintain a granular level of detail in the following descriptions to better capture the nuances of individual actions that later compose the interaction patterns.
• Predict - Outcome: The agent produces a solution to the primary decision-making task after receiving task information. This action is observed to be executed by either the AI or the user independently.
• Decide - Outcome: The agent integrates the assistance they received with the task-related information available to finalize the decision outcome. This action is typically observed to be executed by the user.
• Provide - Options: The agent offers solutions for a secondary task that, while not directly resolving the primary decision-making task, are still informative. This action is typically observed to be executed by the AI.
• Display - Information: The agent presents supplementary evidence (e.g., explanations, uncertainty values, alternate solutions) supporting a solution to the primary decision-making task. This action is observed to be predominately executed by the AI.
• Request - Outcome/Information: The agent actively seeks information or solutions from its counterpart. This action is observed to be either mandatory or optional and is typically executed by the AI when it requires user inputs, or by the user when they seek a direct solution or supplementary information from the AI.
• Collect - Inputs: The agent gathers task-related information and provides it to the other agent. This action is observed to be typically executed by the user when their input is needed for the AI to provide a solution to the decision-making task.
• Modify - Outcome/Information: The agent makes changes to the solutions or supplementary information provided by its counterpart. This action is typically observed to be optional and executed by the user.
• Delegate - Decision: The agent decides whether to retain responsibility for the task or transfer to its counterpart. This action is observed to be executed by either the AI or the user. Events after delegation can differ, ranging from complete surrender of agency to opportunities for supervising the other agent's decision-making process.
• Other: if an action does not fit the previous types.
These concepts were refined and iterated as we reviewed more works since there could be new actions supported for either agent, while trying to maintain the generalizability of the blocks across multiple studies. For each paper, we first identified all possible (action - output) pairs as the building blocks for each agent involved in the decision-making task and included a brief description in free text form. Then, we defined a sequence of interactions considering the order in which these events take place and the agent in charge. The sequences were not preset in advance in the extraction template since we wanted to discover the interaction patterns here. We note that depending on the experimental manipulation of the user study, different modes of interactivity with the user could be plausible and we separated these into different sequences.
We present seven categories of interaction patterns that we have identified in our corpus, illustrated in Figure 1. Interactions involve changes over time and we attempted to capture this evolution/progression in the interaction patterns presented below and in the diagrams that illustrate them. To formulate the taxonomy, we began by reviewing previous literature containing taxonomies of interaction techniques in other domains, such as information visualization (Yi et al., 2007), human-robot interaction (Sauppé and Mutlu, 2014), multi-agent systems (Cabri et al., 2002), and educational technologies (Sims, 1997). Contrasting the concepts in these taxonomies with a sample of studies in human-AI interaction that we were familiar with, we identified potential ways in which the types of interactions described previously could potentially apply to humans interacting with AI during decision making. As we analyzed the articles included in our review, we iteratively refined our taxonomy's definitions and structure, merging similar interaction types and creating new categories when existing ones did not adequately capture the observed patterns. In each pattern formulation, we considered an appropriate level of abstraction so that they can capture multiple actions of the agents and generalize over various studies included in the systematic review. The interaction patterns we defined in the taxonomy are comprised of the interaction building blocks and can borrow some of the other pattern categories. Furthermore, we included a “Other patterns” category to present the interaction patterns that did not exactly fit into the main categories. In the following descriptions, we assume that users already have some background knowledge or intuitions that can be used during the decision making task. Lastly, the classification of interaction patterns does not mean they are mutually exclusive events, but elements that can be consolidated and combined. After finalizing the taxonomy, we quantified the frequency of each interaction pattern across our corpus of 105 articles using a combination of an automated search for certain action-output pairs present in sequences of interactions that we characterized in the data extraction stage of the review, followed by manual inspection and verification. We then analyzed the application domains where these interactions were studied.
Figure 1. Taxonomy of interaction patterns identified in AI-assisted decision making. The user (human) and the AI are represented as separate agents and the temporal evolution of the interactions is illustrated from top to down. The boxes contain the building blocks (action-output) pairs that compose each pattern. The direction of arrows denote the agent who started the action. Dashed lines represent optional operations.
This pattern manifests when the decision-making problem and the AI-predicted outcome are simultaneously displayed to the user. As the ultimate decision maker, the user can choose to incorporate the AI's advice into their final decision or opt to disregard it. When task-related stimuli (e.g., images or case details) are presented alongside the AI-predicted outcome, the user is provided with a more comprehensive set of information to consider. In addition, the AI's outcome can be accompanied by support information as captured in the Display - Information building block. This pattern has been previously observed and referred to as the“concurrent paradigm” (Tejeda et al., 2022) or “one-step workflow” (Fogliato et al., 2022).
This pattern begins with the user forming an independent preliminary prediction given the decision-making problem. Following this initial judgment, the AI's predicted outcome is presented and may accompanied by support information. This procedure provides the user with a reference (their initial assessment) to compare against the AI's advice, and an opportunity to reassess their initial judgment. This approach has been identified as the “sequential paradigm” (Tejeda et al., 2022) or “two-step workflow” (Fogliato et al., 2022) and has been commonly used to evaluate the human's reliance on the AI's advice.
In this pattern, the AI offers information that does not serve as direct solutions to the decision-making problem. The user must interpret this supplementary information as an auxiliary task to determine its relevance and decide how to incorporate it into their primary decision-making process. We distinguish this as a unique interaction pattern because users may respond differently to direct assistance compared to more secondary assistance in their decision-making process. For example, machine learning models can predict risk values associated with certain profile information and the human's decision problem is to make an investment decision (Dikmen and Burns, 2022).
In this interaction pattern, the user has to actively seek information or solutions from the AI. Rather than the AI's inferences being automatically presented, the user can control when they want to receive the AI assistance. Meanwhile, the user can spend more time deliberating about the problem, a strategy known as cognitive forcing (Buçinca et al., 2021; Park et al., 2019). This pattern can be perceived as less intrusive to the user, as it empowers the user to “ask” for information or solutions from the AI, and allows the user to anticipate the AI's assistance in the decision-making process.
Within this interaction pattern, the AI facilitates a dialogue-like engagement with the user. Guided by the AI's instructions, the user responds by providing pertinent information. The iterative exchange continues until the AI's instruction requirements are satisfied, and is followed by the presentation of the AI's predicted outcome for the decision-making task. This responsive exchange not only involves retrieving and sharing information in line with the task but also ensures that the users recognize the influence of their inputs on the AI's predicted outcome. While this pattern has been commonly observed in humans interacting with conversational agents (Jiang et al., 2022; Gupta et al., 2022), it is not only limited to traditional conversational interfaces (Gomez et al., 2023).
Inspired by the taxonomy of interactive explanations recently proposed from a scoping review (Bertrand et al., 2023), we included an interaction pattern where humans can modify the outcome space of the AI agent. Typically, information flows from the AI to the user. However, in this pattern, the direction of flow is reversed, with humans providing the AI with feedback, corrections, or information to shape its inferences. While a detailed classification of potential modifications is beyond the scope of this survey, we distinguished cases in which the changes are merely visual updates in the interface or considered as feedback to improve the underlying AI models, as in interactive machine learning (Amershi et al., 2014).
In this interaction pattern, both the user and the AI leverage their unique strengths and capabilities to optimize the decision-making outcome. Delegation can be a strategic choice when one agent assesses its counterpart as better equipped for a particular task (Fügener et al., 2022). On the other hand, if an agent feels confident in their ability to complete the task, they will take the lead. Studies highlight that the complementary abilities of humans and AI when synergized properly, can enhance the decision-making outcome (Zhang et al., 2022).
We included within this category those patterns that involve a combination of the main interaction blocks and did not fit into the patterns described before. More complex interaction emerges when the decision making problem may involve multiple individual decisions, agents (more than two), and continuous interactions with an AI agent.
We identified the different categories of interaction patterns in the selected 105 articles. If a study included more than one interaction sequence, i.e., the experimental manipulation resulted in different ways in which users can interact with the AI, we considered them separately and counted patterns in each one. In total, we analyzed 131 sequences. The most common interaction pattern during empirical evaluations of AI-assisted decision making tasks was the AI-first assistance (n = 67), followed by the AI-follow assistance (n = 28). Furthermore, the AI's solutions to the decision making task were presented along with additional information in the majority of the cases, 81% and 68% during AI-first and AI-follow interactions, respectively. For instance, in the AI-first pattern for predicting student outcomes, the AI takes student-related features and predicts pass or fail with a confidence value (Rastogi et al., 2022). Participants then review this AI prediction alongside the student data to make their final decision. Meanwhile, in the AI-follow pattern for another binary task, participants make a initial prediction regarding the output of speed dating events, and then a final one after seeing AI's prediction. We observed 16 instances of the Secondary assistance pattern. In particular, we noticed that most of the decision making tasks required expertise (11/16). As an example, in the task of predicting gestational diabetes mellitus (GDM), healthcare professionals are presented with various descriptive features such as a history of diabetes mellitus, age, body weight, etc. (Du et al., 2022). The AI system processes this information and outputs a categorized risk of GDM, labeling it as either low or high. Rather than offering a direct solution, the AI presents this risk category alongside an explanation, thereby serving as a form of secondary assistance.
Sometimes during the decision making task, users had to actively seek support from the AI agent as specified in the Request-driven AI assistance (n = 25). More specifically, the requests could be for a direct a solution to the decision making task (n = 14) or for the presentation of support information (n = 13). In the former, only in three cases the request for the AI's solution was optional (Kumar et al., 2021; Tolmeijer et al., 2021; Baudel et al., 2021), meaning that users could come with a solution to the decision making task on their own. As illustrated in a house search scenario, users could choose to use an AI system to help them find a house that satisfies certain requirements, with the option to directly submit the suggested house or verify (Tolmeijer et al., 2021). Meanwhile, the support information at the user's discretion was identified in eight cases (Calisto et al., 2022; Vössing et al., 2022; Suresh et al., 2020; van der Waa et al., 2021; Liu et al., 2021; Molina and Sundar, 2022; Prabhudesai et al., 2023), for demanding explanations in particular. In the AI-first assistance pattern, where the user may not have an opportunity to form an independent assessment of the decision making problem, we observe cases (n = 10) in which users are given the ability to control when they want to receive the AI assistance via a request (Khadpe et al., 2020; Mackeprang et al., 2019; Baudel et al., 2021; Molina and Sundar, 2022; Gomez et al., 2023; Buçinca et al., 2021).
To a lesser extent, we found the interaction patterns that involve more exchange components between the human and the AI agent. For the AI-guided dialogic user engagement, as the name suggests, five out of the six interactions were supported via conversations with the AI agent. Through conversational interfaces, users had to provide some constraints given in the decision making problem for the AI to propose a candidate solution (Khadpe et al., 2020; Jiang et al., 2022; Gupta et al., 2022). However, such exchange of information does not necessarily rely on a conversational interface, as demonstrated in the evaluation of an AI system that could constantly provide guidance on a decision sub-problem for identifying bird categories (Gomez et al., 2023). The two-way interaction occurs as the AI requests and suggests bird attributes for description, culminating in a bird category suggestion. Users can actively engage by processing the attributes, considering the AI's input, and making decisions regarding the bird's attributes. Ultimately, they verify the AI's suggested bird category. Interactivity also support the adjustment of the AI's outcome space, and we found the User-guided interactive adjustments in nine cases that differed on the observed effect of the adjustment. For instance, manipulations of the inputs result in new AI's outcome computations for exploratory purposes (Liu et al., 2021; Gu et al., 2023; Nguyen et al., 2018; Zytek et al., 2022; Suresh et al., 2022). Such functionality can be incorporated into interactive explanations, where users can manipulate input values of a specific instance and observe the change in recidivism predictions (Liu et al., 2021). When adjustments did not directly translate into an updated AI's outcome, users' feedback was considered for future improvement of the model (Ashktorab et al., 2021; Molina and Sundar, 2022; Smith-Renner et al., 2020; Lee et al., 2021). Manual labeling after observing AI predictions can be leveraged to identify incorrect intent classifications from textual samples and re-train the models (Ashktorab et al., 2021).
Opportunities to delegate decisions were observed in nine sequences of interactions, though with differences in the conditions for delegation. For instance, in some cases the users “blindly” delegate the decision to the AI without having access to their outcome and not being able to supervise it later (Chiang and Yin, 2021; Maier et al., 2022; Zhang et al., 2020; Fügener et al., 2022). For example, in stock investment decisions, people can choose to invest directly in specific stocks or delegate a portion of their funds to the AI for future investment decisions (Maier et al., 2022). In others, the AI agent has the decision to delegate and the user is assigned some of the decision making tasks to be completed on their own (Hemmer et al., 2023; Fügener et al., 2022), or presented with the AI's outcome as support, resulting in the AI-first pattern (Bondi et al., 2022). In addition, the user can object to the delegation decision of the AI and take charge of the decision if considered appropriate (van der Waa et al., 2021).
Lastly, the articles that we included in the “Others” category of interaction patterns can be separated into three groups. First, decision making tasks that involved more than one decision outcome (Porat et al., 2019; M. A. Rahman et al., 2021) and corresponding support from the AI agent. Second, decision making problems where multiple instances of decision tasks can take place and the interaction with the AI agent is continuous (Van Berkel et al., 2022; Fan et al., 2022; Nourani et al., 2021; Lindvall et al., 2021; Reverberi et al., 2022). Third, interactions that involved a third agent (Wu et al., 2022; Brachman et al., 2022; Banas et al., 2022). In addition, a different case results when independent solutions, from the human and the AI agent, to the decision making problem are averaged as the final verdict (Xiong et al., 2023).
The selected articles included in this survey cover a broad range of different domain categories previously identified (Lai et al., 2023). Articles that included more than one experimental decision making task were counted toward more than one domain. Table 1 presents a summary of the major domains and the different decision making tasks evaluated. Overall, the majority of the studies conducted human-AI interaction evaluations in real-world applications, with less than 15% formulating artificial tasks. We included medical related databases in our search strategy, which contributes to the large representation of decision making tasks on the healthcare domain (26/108). In addition to healthcare, decision making tasks that may involve high-stakes outside of an experimental setup were identified in the finance and business (15/108), and law domains (6/108). The second most common domain was in the context of generic tasks (20/108) that are low-effort processing for humans but have mostly been used to develop AI benchmarks and demonstrate technical feasibility of algorithms. Other domains that typically include tasks targeted to non-expert users are social media (8), labeling (8), and leisure (1). We assigned tasks with unique applications to the Other domain (6/108). Even though we identified multiple decision making tasks in applications that require a specialized population, where recruiting large numbers of participants is often challenging, the majority of human-AI interactions have been evaluated with non-expert uses (60/108). The type of AI systems behind the interactions with users in the studies that covered these decision-making tasks were distributed among three categories: simulated models or Wizard of Oz experiments (39/105), deep learning-based models (34/105), and shallow models (35/105).
To better understand the existence and availability of the interaction patterns in different domains, we quantified the occurrence of patterns per domain and provide an overview describing the trends. Figure 2 shows the distribution of interaction patterns within our taxonomy for different domains. Values equal to zero mean that certain interaction pattern was not observed in the studies included in this survey for a specific domain. Tables in the Appendix C provide more details on the interactions patterns for each paper included in this review.
Figure 2. Percentage of interaction patterns observed in each domain of AI-assisted decision making tasks included in this review. The numbers in the cells denote the percentage values (e.g., 17% of the patterns identified in the healthcare domain correspond to Secondary assistance). One study can include multiple sequences of interaction and interaction patterns are not mutually exclusive.
Human-AI interactions in AI-advised decision makings in the healthcare domain mostly adhered to the AI-first assistance pattern (n = 14), followed by Secondary assistance (n = 7). Request-driven AI assistance was observed in a few cases (n = 4) as well as AI-follow assistance (n = 4). We identified one interaction that supported AI-guided dialogic user engagement, three interactions in the User-guided interactive adjustments, and one in which delegation was an option. Five sequences of interactions were in the “Others” category due to the higher complexity of the interactions. In the domain of finance and business, AI-first assistance was the most common type of interaction (n = 6), followed by AI-follow assistance (n = 4) and Secondary assistance (n = 4). Some interactions supported Request-driven AI assistance (n = 3), AI-guided dialogic user engagement (n = 2), and delegation (n = 3). We did not observe support for User-guided interactive adjustments. We identified four types of interaction patterns in the law and civic domain: AI-first assistance (n = 4), AI-follow assistance (n = 2), Request-driven assistance (n = 2), and User-guided interactive adjustments (n = 2). Decision-making tasks that involve professional related topics mostly followed AI-first assistance (n = 4). Request-driven AI assistance and user-guided interactive adjustments were observed in one case each one. The other interaction patterns were not observed. Interaction patterns during decision making tasks involved in social media contexts were mostly of the AI-first (n = 4), AI-follow (n = 4), and Request-driven AI assistance types (n = 4). We identified Secondary assistance in one case, and two cases in which User-guided interactive adjustments were enabled, in particular, for updating relevant terms for content moderation purposes. No Delegation or AI-guided dialogic user engagement was observed and two cases fell into the “Others” patterns category. During decision making tasks in generic applications, most of the interactions were dominated by the AI-first assistance (n = 21), while only two cases involved the AI-follow assistance interaction. Regarding the more interactive patterns, two cases supported Request-driven AI assistance, two AI-guided dialogic user engagement, and one User-guided interactive adjustments. Only in one case the type of assistance was secondary. Delegation was featured in five cases. Labeling tasks mostly included AI-first assistance patterns (n = 6), followed by Request-driven AI (n = 4) and Secondary assistance (n = 3), mostly clustering similar data points. We further identified AI-follow assistance and User-guided interactive adjustments once each one, and two types of interactions in the “Others” patterns category. Artificial decision making tasks were mostly dominated by AI-follow follow (n = 8) and AI-first assistance (n = 7). Request-driven AI assistance was featured in four cases and four interactions were in the “Others” patterns category. Lastly, AI-guided dialogic user engagement was the type of interaction in the leisure-related task and AI-first assistance in the task within the education domain. Interactions during decision-making tasks that belong to other domains mainly contained AI-first (n = 4) and AI-follow (n = 3) assistance types, or patterns in the “Others” category (n = 3). Further, one case supported Request-driven AI assistance.
In establishing the interaction patterns presented in this paper, we drew from our observations of the Human-AI interactions used in AI-advised decision-making scenarios in prior empirical studies. Constructing a taxonomy is inherently challenging due to the wide array of potential approaches that can be adopted. Specifically, in the domain of Human-AI interaction, interactions can be examined through various lenses (e.g., system-centric, oriented around user goal/task, distinguished by varying levels of granularity in interaction techniques). In this work, we have taken a preliminary step to structure an approach by integrating our perspectives with observations of interaction paradigms used in existing studies. Below, we discuss the findings from our systematic review in combination with the taxonomy, showcasing how it allowed us to identify trends and opportunities for the study of human-AI interactions.
Using our taxonomy, we characterized existing interactions adopted in empirical studies. In general, while the most common patterns were AI-first, AI-follow, or Secondary assistance, in which the human role was limited to supervising the AI predictions, we did also note the presence of more dynamic interactions (e.g., Request-driven AI assistance, AI-guided dialogic user engagement, User-guided interactive adjustments), although less frequently. Interactive elements can enhance the communication of explanations allowing users to interpret AI predictions through selection, mutation, and dialogue as suggested by Bertrand et al. (2023). Likewise, supporting human input and review in the field of Interactive Machine Learning requires the design of interface elements for sample review, model inspection, or feedback assignment (Dudley and Kristensson, 2018). Beyond supervising AI outputs, a new paradigm arises when humans and AI co-create solutions, blending human intuition and expertise with AI's computational strengths for more robust outcomes. Conversational interfaces play a key role in enabling this two-way interaction. Moreover, the concept of distributing decision-making responsibilities among different agents, as seen in delegation patterns, extends the assistance beyond individual decisions (Lai et al., 2022). This leads to diverse collaborative strategies, ranging from working in parallel—as exemplified in delegation scenarios where AI operates autonomously but in alignment with human intent—to more coordinated efforts, such as the turn-taking dynamic inherent in conversational AI.
A finer analysis per domain revealed the limited use and support for diverse interaction patterns, represented by most cell values equal to zero in Figure 2. However, it is worth noticing that for high-stake domains, such as healthcare and finance, multiple interaction patterns have been explored when AI provides decision support. The choice of specific interaction patterns can be influenced by several factors including both design choices made by researchers and the intrinsic nature of the problems being addressed. In the former, the research intent and available resources may affect design choices. In the later, ethical and legal considerations play an important role, specially in high-stakes domains. In more specialized fields, domain experts have their unique set of capabilities that can directly influence the choice and efficacy of interactions with the AI. For these experts, Secondary assistance can be beneficial, since they have the insights to effectively use the supplementary AI information for the primary decision-making task. However, for non-experts, the most suitable and beneficial choice of interaction pattern is unclear. There remain questions about the universality of certain interaction patterns across varied user groups and task scenarios. Furthermore, with the common usage of the AI-first and AI-follow approaches, understanding their pros and cons is crucial in developing AI systems that align with human cognitive processes and decision-making styles.
The popularity of the AI-first assistance pattern can be attributed to the straightforwardness of demonstrating the effects of incorporating AI assistance into a decision-making task. However, it presents challenges, notably the difficulty in measuring the actual influence of AI assistance on user decision-making. Since the AI's solution is revealed before the user has had the opportunity to process the task independently, it can be convenient for the user to either dismiss or follow the AI's recommendation without sufficient reflection. Interaction patterns may engender different types of biases and knowing them in advance may help guard against biases. For instance, the AI-first interaction interaction can make the user susceptible to the “anchoring bias,” a phenomenon where a person's judgment is biased based on initial information. This bias can be avoided through the use of Secondary assistance, where the user must interpret supplementary information, determine its relevance, and decide how to incorporate it into their primary decision-making process. Directly presenting a solution to the decision making problem can result in over-reliance (Nourani et al., 2020), whereas Secondary assistance can avoid anchoring effects, but may not satisfy user needs. In addition, direct presentation of AI inferences can lead to a lack of “sense of agency” for the user, which refers to the subjective feeling of controlling one's actions, and influencing external events through them (Wen and Imamizu, 2022). Request-driven AI assistance can empower the user with the choice to view AI inferences and foster a sense of agency; however, it may also introduce risks of confirmation bias or anchoring bias, especially when users seek explanations for decision verification or knowledge acquisition (Barda AJ et al., 2020).
In contrast, in the AI-follow assistance pattern, the user is given a chance to solve the problem on their own, thus, potentially minimizing anchoring bias. Yet, whether users actually restart their decision making process is open to question. An article found that participants in this “two-step” workflow rarely revised their provisional diagnoses when the AI inferences differed from their earlier assessment (Fogliato et al., 2022). This hints at confirmation bias, a person's tendency to seek supporting evidence for their current hypothesis. In case the user does re-evaluate their prior assessments, the cognitive costs increase. Cognitive costs of re-examination, when new information becomes available, can be viewed as analogous to interruption and recovery on the initial task with new information (Fogliato et al., 2022). Being the second most common pattern, the prevalence of the AI-follow pattern likely arises from a strong interest in disentangling the influence of AI advice on the human's decision (Vereschak et al., 2021). We primarily focused on biases in the most common interaction patterns, as the higher number of studies aids in identifying them. However, exploring potential biases in less frequent paradigms is also encouraged.
From our thorough exploration of interaction patterns in a sample of over 100 articles, several gaps and opportunities emerge for advancing the design of human-AI interactions. First, most studies focus on single-user and single-AI interactions, overlooking the potential of multi-agent collaboration, which could unlock new dynamics and enhance teamwork in complex tasks. Moreover, as interaction patterns grow more complex, existing frameworks like the Delegation pattern, where the agents can reassign decisions, could be expanded to explore how agents coordinate and allocate tasks among themselves. Lastly, much of the HCI literature on AI assistance has concentrated on intermittent scenarios (i.e., turn-taking). This is in contrast to continuous user interaction scenarios, where user input is sustained and can receive AI feedback at any given moment for a more realistic and organic setup. Likewise, many of the human-AI interactions took place in artificial tasks that may not capture real-world complexities. For example, most studies end after decisions are made, without exposing decision-makers to the full consequences of their choices (Kirkebøen et al., 2013), which can influence user engagement even in scenarios labeled as high stakes. We must carefully consider differences in how users behave in experimental tasks and in equivalent real-life scenarios to assess whether AI assistance truly adds value to decision problems and capitalize on the findings of experimental evaluations.
The design of Human-AI interactions requires deliberate choices informed by cross-disciplinary expertise. By establishing a shared terminology, our taxonomy can facilitate conversations and collaboration between researchers in AI, HCI, human factors engineering, and domain experts. This common ground can ensure consistency in methodology and foster innovation in research, development, and user experiences, ultimately leading to more informed and participative decision-making processes. The taxonomy captures reusable interaction components and their relationships, providing insights to guide concrete choices about enabling interactions between the agents involved and the corresponding interface elements needed (van Bekkum et al., 2021). We illustrate how the taxonomy serves as a framework to aggregate knowledge about the interactions present in empirical studies, revealing trends about how humans and AI collaborate in decision-making processes and identifying unexplored opportunities in human-AI collaboration. From a design perspective, it provides a collection of repeatable solutions to problem types that can be incorporated into prototypes for faster iterations. As the field of human-AI interaction continues to evolve, this taxonomy can be expanded and refined to incorporate new interaction patterns and ideas from emerging research.
The works included in this survey are limited to published manuscripts that conducted empirical evaluations of human-AI interactions. This focus, while intentional, could have introduced certain limitations. The terminology used in the search could have excluded relevant work if the interaction design was not explicitly mentioned in the title or abstract, or even in the body of the text. Moreover, publication bias may have resulted in the exclusion of works relevant to this review. Since our search included only a healthcare-focused database alongside more general ones, the frequency of interactions we observed across various domains where AI was used for decision-making may not remain consistent if additional domain-specific libraries were included in our search. We encourage further investigations in other domains of interest to researchers to build a more comprehensive understanding of human-AI interactions. We also constrained our analysis to screen-based interfaces for AI-assistance, acknowledging that embodied AI might support additional interactions. Our strict selection criteria centered on studies encompassing complete decision-making tasks to ensure actual Human-AI interactions. Given the diversity of experimental designs and factors in the papers reviewed in this survey, we abstracted the interactions to discern patterns across the varied studies. While many of these studies effectively described their interfaces and user study procedures, there were instances where the information provided was not sufficient for a complete recreation of the interactions. Consequently, we had to carefully interpret and encode the interactions from these papers to the best of our abilities. Even though we demonstrate a use case of the taxonomy to characterize the AI-assisted decision making literature, further use case evaluations can help in assessing the utility of the taxonomy and refine its definition.
In this paper, we presented a systematic review of human-AI interactions in AI-advised decision making tasks that informed and grounded the formulation of a taxonomy of interaction patterns. Our proposed taxonomy of interaction patterns provides a structured foundation for understanding and designing these crucial interactions. It reveals that current practices often lean toward AI-driven or human-led decision processes, with limited emphasis on fostering interactive functionalities throughout the interactions. Recognizing the significance of interaction design, we advocate for deliberate choices in system development to enhance collaboration between humans and AI. Moving forward, the taxonomy presented here serves as a valuable resource to inform the design and development of AI-based decision support systems, ultimately fostering more productive, engaging, and user-centered collaborations.
CG: Conceptualization, Data curation, Formal analysis, Investigation, Methodology, Visualization, Writing – original draft, Writing – review & editing. SC: Formal analysis, Visualization, Writing – original draft, Writing – review & editing, Investigation. SK: Data curation, Formal analysis, Investigation, Methodology, Writing – original draft. C-MH: Conceptualization, Investigation, Methodology, Supervision, Writing – review & editing. MU: Conceptualization, Funding acquisition, Investigation, Methodology, Project administration, Resources, Supervision, Writing – review & editing.
The authors declare financial support was received for the research, authorship, and/or publication of this article. This research was supported in part by Johns Hopkins Internal Funds.
The authors declare that the research was conducted in the absence of any commercial or financial relationships that could be construed as a potential conflict of interest.
The author(s) declare that Gen AI was used in the creation of this manuscript. Generative AI (chatGPT 4.0) was used to process the text originally produced by the author(s). The processing was done for improving the clarity and structure of the sentences and not to create new content. All the generated outputs were validated before using and adapting them for the manuscript.
All claims expressed in this article are solely those of the authors and do not necessarily represent those of their affiliated organizations, or those of the publisher, the editors and the reviewers. Any product that may be evaluated in this article, or claim that may be made by its manufacturer, is not guaranteed or endorsed by the publisher.
The Supplementary Material for this article can be found online at: https://www.frontiersin.org/articles/10.3389/fcomp.2024.1521066/full#supplementary-material
Allan, K., Oren, N., Hutchison, J., and Martin, D. (2021). In search of a goldilocks zone for credible AI. Sci. Rep. 11:13687. doi: 10.1038/s41598-021-93109-8
Alufaisan, Y., Marusich, L. R., Bakdash, J. Z., Zhou, Y., and Kantarcioglu, M. (2021). “Does explainable artificial intelligence improve human Decision-Making?” in Proceedings of the AAAI Conference on Artificial Intelligence, 6618–6626. doi: 10.1609/aaai.v35i8.16819
Amershi, S., Cakmak, M., Knox, W. B., and Kulesza, T. (2014). Power to the people: the role of humans in interactive machine learning. Ai Magaz. 35, 105–120. doi: 10.1609/aimag.v35i4.2513
Appelganc, K., Rieger, T., Roesler, E., and Manzey, D. (2022). How much reliability is enough? A Context-Specific view on human interaction with (artificial) agents from different perspectives. J. Cogn. Eng. Decis. Mak. 16, 207–221. doi: 10.1177/15553434221104615
Ashktorab, Z., Desmond, M., Andres, J., Muller, M., Joshi, N. N., Brachman, M., et al. (2021). “AI-Assisted human labeling: Batching for efficiency without overreliance,” in Proceedings of the ACM on Human-Computer Interaction, 5. doi: 10.1145/3449163
Banas, J. A., Palomares, N. A., Richards, A. S., Keating, D. M., Joyce, N., and Rains, S. A. (2022). When machine and bandwagon heuristics compete: understanding users' response to conflicting AI and crowdsourced fact-checking. Hum. Commun. Res. 48, 430–461. doi: 10.1093/hcr/hqac010
Bansal, G., Nushi, B., Kamar, E., Lasecki, W. S., Weld, D. S., and Horvitz, E. (2019). “Beyond accuracy: the role of mental models in human-ai team performance,” in Proceedings of the AAAI Conference on Human Computation and Crowdsourcing, 2–11. doi: 10.1609/hcomp.v7i1.5285
Bansal, G., Wu, T., Zhou, J., Fok, R., Nushi, B., Kamar, E., et al. (2021). “Does the whole exceed its parts? The effect of AI explanations on complementary team performance,” in Proceedings of the 2021 CHI Conference on Human Factors in Computing Systems, 1–16. doi: 10.1145/3411764.3445717
Barda, A. J., Horvat, C. M., and Hochheiser, H. (2020). A qualitative research framework for the design of user-centered displays of explanations for machine learning model predictions in healthcare. BMC Med. Inform. Decis. Mak. 20:257. doi: 10.1186/s12911-020-01276-x
Baudel, T., Verbockhaven, M., Cousergue, V., Roy, G., and Laarach, R. (2021). “ObjectivAIze: Measuring performance and biases in augmented business decision systems,” in Human-Computer Interaction INTERACT 2021: 18th IFIP TC 13 International Conference, Bari, Italy, August 30 September 3, 2021, Proceedings, Part III 18 (Springer International Publishing), 300–320. doi: 10.1007/978-3-030-85613-7_22
Bernard, J., Hutter, M., Zeppelzauer, M., Fellner, D., and Sedlmair, M. (2018). Comparing Visual-Interactive labeling with active learning: an experimental study. IEEE Trans. Vis. Comput. Graph. 24, 298–308. doi: 10.1109/TVCG.2017.2744818
Bertrand, A., Belloum, R., Eagan, J. R., and Maxwell, W. (2022). “How cognitive biases affect XAI-assisted decision-making: a systematic review,” in Proceedings of the 2022 AAAI/ACM Conference on AI, Ethics, and Society, 78–91. doi: 10.1145/3514094.3534164
Bertrand, A., Viard, T., Belloum, R., Eagan, J. R., and Maxwell, W. (2023). “On selective, mutable and dialogic XAI: a review of what users say about different types of interactive explanations,” in Proceedings of the 2023 CHI Conference on Human Factors in Computing Systems, 1–21. doi: 10.1145/3544548.3581314
Bhattacharya, A., Ooge, J., Stiglic, G., and Verbert, K. (2023). “Directive explanations for monitoring the risk of diabetes onset: Introducing directive Data-Centric explanations and combinations to support What-If explorations,” in Proceedings of the 28th International Conference on Intelligent User Interfaces, 204–219. doi: 10.1145/3581641.3584075
Bondi, E., Koster, R., Sheahan, H., Chadwick, M., Bachrach, Y., Cemgil, T., et al. (2022). “Role of Human-AI interaction in selective prediction,” in Proceedings of the AAAI Conference on Artificial Intelligence, 5286–5294. doi: 10.1609/aaai.v36i5.20465
Brachman, M., Ashktorab, Z., Desmond, M., Duesterwald, E., Dugan, C., Joshi, N. N., et al. (2022). “Reliance and automation for Human-AI collaborative data labeling conflict resolution,” in Proceedings of the ACM on Human-Computer Interaction, 6(CSCW2). doi: 10.1145/3555212
Buçinca, Z., Lin, P., Gajos, K. Z., and Glassman, E. L. (2020). “Proxy tasks and subjective measures can be misleading in evaluating explainable AI systems,” in Proceedings of the 25th International Conference on Intelligent User Interfaces, 454–464. doi: 10.1145/3377325.3377498
Buçinca, Z., Malaya, M. B., and Gajos, K. Z. (2021). “To trust or to think: cognitive forcing functions can reduce overrelianceon AI in AI-assisted decision-making,” in Proceedings of the ACM on Human-Computer Interaction. doi: 10.1145/3449287
Bunde, E. (2021). “AI-assisted and explainable hate speech detection for social media moderators - a design science approach,” in Proceedings of the 54th Hawaii International Conference on System Sciences, 1264–1273. doi: 10.24251/HICSS.2021.154
Cabitza, F., Campagner, A., Natali, C., Parimbelli, E., Ronzio, L., and Cameli, M. (2023). Painting the black box white: experimental findings from applying XAI to an ECG reading setting. Mach. Lear. Knowl. Extr. 5, 269–286. doi: 10.3390/make5010017
Cabrera, Á. A., Perer, A., and Hong, J. I. (2023). “Improving Human-AI collaboration with descriptions of AI behavior,” in Proceedings of the ACM on Human-Computer Interaction, 1–21. doi: 10.1145/3579612
Cabri, G., Leonardi, L., Zambonelli, F., et al. (2002). “Modeling role-based interactions for agents,” in The Workshop on Agent-Oriented Methodologies at OOPSLA (Citeseer).
Calisto, F. M., Santiago, C., Nunes, N., and Nascimento, J. C. (2022). BreastScreening-AI: evaluating medical intelligent agents for human-AI interactions. Artif. Intell. Med. 127:102285. doi: 10.1016/j.artmed.2022.102285
Cao, S., and Huang, C.-M. (2022). “Understanding user reliance on AI in assisted Decision-Making,” in Proceedings of the ACM on Human-Computer Interaction, 6. doi: 10.1145/3555572
Cau, F. M., Hauptmann, H., Spano, L. D., and Tintarev, N. (2023). “Supporting high-uncertainty decisions through ai and logic-style explanations,” in Proceedings of the 28th International Conference on Intelligent User Interfaces, 251–263. doi: 10.1145/3581641.3584080
Chen, H., Gomez, C., Huang, C.-M., and Unberath, M. (2022). Explainable medical imaging ai needs human-centered design: guidelines and evidence from a systematic review. NPJ Digital Med. 5:156. doi: 10.1038/s41746-022-00699-2
Cheng, R., Smith-Renner, A., Zhang, K., Tetreault, J. R., and Jaimes, A. (2022). Mapping the design space of human-ai interaction in text summarization. arXiv preprint arXiv:2206.14863. doi: 10.18653/v1/2022.naacl-main.33
Chiang, C.-W., and Yin, M. (2021). “You'd better stop! understanding human reliance on machine learning models under covariate shift,” in Proceedings of the 13th ACM Web Science Conference 2021, 120–129. doi: 10.1145/3447535.3462487
Chiang, C.-W., and Yin, M. (2022). “Exploring the effects of machine learning literacy interventions on laypeople's reliance on machine learning models,” in Proceedings of the 27th International Conference on Intelligent User Interfaces, 148–161. doi: 10.1145/3490099.3511121
David, P., Cakmak, M., Sauppé, A., Albarghouthi, A., and Mutlu, B. (2022). “Interaction templates: a data-driven approach for authoring robot programs,” in PLATEAU: 12th Annual Workshop at the Intersection of PL and HCI.
Desmond, M., Muller, M., Ashktorab, Z., Dugan, C., Duesterwald, E., Brimijoin, K., et al. (2021). “Increasing the speed and accuracy of data labeling through an AI assisted interface,” in Proceedings of the 26th International Conference on Intelligent User Interfaces, 392–401. doi: 10.1145/3397481.3450698
Dikmen, M., and Burns, C. (2022). The effects of domain knowledge on trust in explainable AI and task performance: a case of peer-to-peer lending. International J. Human Computer Studies 162:102792. doi: 10.1016/j.ijhcs.2022.102792
Du, Y., Antoniadi, A. M., McNestry, C., McAuliffe, F. M., and Mooney, C. (2022). The role of XAI in Advice-Taking from a clinical decision support system: a comparative user study of feature Contribution-Based and Example-Based explanations. Appl. Sci. 12:10323. doi: 10.3390/app122010323
Dudley, J. J., and Kristensson, P. O. (2018). A review of user interface design for interactive machine learning. ACM Trans. Inter. Intell. Syst. 8, 1–37. doi: 10.1145/3185517
Fan, M., Yang, X., Yu, T., Liao, Q. V., and Zhao, J. (2022). “Human-AI collaboration for UX evaluation: effects of explanation and synchronization,” in Proceedings of the ACM on Human-Computer Interaction, 6. doi: 10.1145/3512943
Feng, S., and Boyd-Graber, J. (2022). “Learning to explain selectively: a case study on question answering,” in Proceedings of the 2022 Conference on Empirical Methods in Natural Language Processing, 8372–8382. doi: 10.18653/v1/2022.emnlp-main.573
Fogliato, R., Chappidi, S., Lungren, M., Fisher, P., Wilson, D., Fitzke, M., et al. (2022). “Who goes first? Influences of human-AI workflow on decision making in clinical imaging,” in Proceedings of the 2022 ACM Conference on Fairness, Accountability, and Transparency, 1362–1374. doi: 10.1145/3531146.3533193
Fügener, A., Grahl, J., Gupta, A., and Ketter, W. (2022). Cognitive challenges in Human-Artificial intelligence collaboration: investigating the path toward productive delegation. Inf. Syst. Res. 33, 678–696. doi: 10.1287/isre.2021.1079
Gaube, S., Suresh, H., Raue, M., Lermer, E., Koch, T. K., Hudecek, M. F. C., et al. (2023). Non-task expert physicians benefit from correct explainable AI advice when reviewing x-rays. Sci. Rep. 13:1383. doi: 10.1038/s41598-023-28633-w
Gomez, C., Unberath, M., and Huang, C.-M. (2023). Mitigating knowledge imbalance in AI-advised decision-making through collaborative user involvement. Int. J. Hum. Comput. Stud. 172:102977. doi: 10.1016/j.ijhcs.2022.102977
Grgic-Hlaca, N., Engel, C., and Gummadi, K. P. (2019). “Human decision making with machine advice: an experiment on bailing and jailing,” in Proceedings of the ACM on Human-Computer Interaction, 3(CSCW). doi: 10.2139/ssrn.3465622
Gu, H., Liang, Y., Xu, Y., Williams, C. K., Magaki, S., Khanlou, N., et al. (2023). Improving workflow integration with XPath: design and evaluation of aHuman-AI diagnosis system in pathology. ACM Trans. Comput. -Hum. Interact. 30, 1–37. doi: 10.1145/3577011
Gupta, A., Basu, D., Ghantasala, R., Qiu, S., and Gadiraju, U. (2022). “To trust or not to trust: how a conversational interface affects trust in a decision support system,” in Proceedings of the ACM Web Conference 2022, 3531–3540. doi: 10.1145/3485447.3512248
Hemmer, P., Westphal, M., Schemmer, M., Vetter, S., Vøssing, M., and Satzger, G. (2023). “Human-AI collaboration: the effect of AI delegation on human task performance and task satisfaction,” in Proceedings of the 28th International Conference on Intelligent User Interfaces, 453–463. doi: 10.1145/3581641.3584052
Hofeditz, L., Clausen, S., Rieß, A., Mirbabaie, M., and Stieglitz, S. (2022). Applying XAI to an AI-based system for candidate management to mitigate bias and discrimination in hiring. Electr. Markets 32, 2207–2233. doi: 10.1007/s12525-022-00600-9
Holstein, K., De-Arteaga, M., Tumati, L., and Cheng, Y. (2023). Toward supporting perceptual complementarity in Human-AI collaboration via reflection on unobservables. Proc. ACM Hum. -Comput. Interact. 7, 1–20. doi: 10.1145/3579628
Hou, Y. T.-Y., and Jung, M. F. (2021). Who is the expert? Reconciling algorithm aversion and algorithm appreciation in AI-Supported decision making. Proc. ACM Hum. -Comput. Interact. 5, 1–25. doi: 10.1145/3479864
Hwang, J., Lee, T., Lee, H., and Byun, S. (2022). A clinical decision support system for sleep staging tasks with explanations from artificial intelligence: User-Centered design and evaluation study. J. Med. Internet Res. 24:e28659. doi: 10.2196/28659
Jacobs, M., Pradier, M. F., McCoy, T. H., Perlis, R. H., Doshi-Velez, F., and Gajos, K. Z. (2021). How machine-learning recommendations influence clinician treatment selections: the example of the antidepressant selection. Transl. Psychiatry 11:108. doi: 10.1038/s41398-021-01224-x
Jakubik, J., Schöffer, J., Hoge, V., Vössing, M., and Kühl, N. (2023). “An empirical evaluation of predicted outcomes as explanations in human-ai decision-making,” in Joint European Conference on Machine Learning and Knowledge Discovery in Databases (Cham: Springer Nature Switzerland), 353–368. doi: 10.1007/978-3-031-23618-1_24
Jiang, J., Kahai, S., and Yang, M. (2022). Who needs explanation and when? Juggling explainable AI and user epistemic uncertainty. Int. J. Hum. Comput. Stud. 165:102839. doi: 10.1016/j.ijhcs.2022.102839
Kahr, P. K., Rooks, G., Willemsen, M. C., and Snijders, C. C. P. (2023). “It seems smart, but it acts stupid: development of trust in AI advice in a repeated legal Decision-Making task,” in Proceedings of the 28th International Conference on Intelligent User Interfaces, 528–539. doi: 10.1145/3581641.3584058
Khadpe, P., Krishna, R., Fei-Fei, L., Hancock, J. T., and Bernstein, M. S. (2020). “Conceptual metaphors impact perceptions of Human-AI collaboration,” in Proceedings of the ACM on Human-Computer Interaction, 4(CSCW2). doi: 10.1145/3415234
Kim, T., and Song, H. (2023). Communicating the limitations of AI: The effect of message framing and ownership on trust in artificial intelligence. Int. J. Hum. Comput. Interact. 39, 790–800. doi: 10.1080/10447318.2022.2049134
Kirkebøen, G., Vasaasen, E., and Halvor Teigen, K. (2013). Revisions and regret: the cost of changing your mind. J. Behav. Decis. Mak. 26, 1–12. doi: 10.1002/bdm.756
Kumar, A., Patel, T., Benjamin, A. S., and Steyvers, M. (2021). “Explaining algorithm aversion with metacognitive bandits,” in Proceedings of the Annual Meeting of the Cognitive Science Society, 2780–2786.
Lai, V., Carton, S., Bhatnagar, R., Liao, Q. V., Zhang, Y., and Tan, C. (2022). “Human-AI collaboration via conditional delegation: A case study of content moderation,” in Proceedings of the 2022 CHI Conference on Human Factors in Computing Systems, 1–18. doi: 10.1145/3491102.3501999
Lai, V., Chen, C., Smith-Renner, A., Liao, Q. V., and Tan, C. (2023). “Towards a science of human-AI decision making: An overview of design space in empirical human-subject studies,” in Proceedings of the 2023 ACM Conference on Fairness, Accountability, and Transparency, 1369–1385. doi: 10.1145/3593013.3594087
Lai, V., Liu, H., and Tan, C. (2020). “‘Why is ‘chicago' deceptive?' Towards building model-driven tutorials for humans,” in Proceedings of the 2020 CHI Conference on Human Factors in Computing Systems, CHI '20 (New York, NY, USA: Association for Computing Machinery), 1–13. doi: 10.1145/3313831.3376873
Lam Shin Cheung, J., Ali, A., Abdalla, M., and Fine, B. (2022). U“AI” testing: user interface and usability testing of a chest x-ray AI tool in a simulated Real-World workflow. Canadian Assoc. Radiol. J. 74, 314–325. doi: 10.1177/08465371221131200
Lee, M. H., Siewiorek, D. P., and Smailagic, A. (2021). “A human-ai collaborative approach for clinical decision making on rehabilitation assessment,” in Proceedings of the 2021 CHI Conference on Human Factors in Computing Systems, 1–14. doi: 10.1145/3411764.3445472
Leichtmann, B., Humer, C., Hinterreiter, A., Streit, M., and Mara, M. (2023). Effects of explainable artificial intelligence on trust and human behavior in a high-risk decision task. Comput. Human Behav. 139:107539. doi: 10.1016/j.chb.2022.107539
Liehner, G. L., Brauner, P., Schaar, A. K., and Ziefle, M. (2022). Delegation of moral tasks to automated Agents–The impact of risk and context on trusting a machine to perform a task. IEEE Trans. Technol. Soc. 3, 46–57. doi: 10.1109/TTS.2021.3118355
Lindvall, M., Lundström, C., and Löwgren, J. (2021). “Rapid assisted visual search: Supporting digital pathologists with imperfect AI,” in Proceedings of the 26th International Conference on Intelligent User Interfaces, 504–513. doi: 10.1145/3397481.3450681
Liu, H., Lai, V., and Tan, C. (2021). “Understanding the effect of out-of-distribution examples and interactive explanations on Human-AI decision making,” in Proceedings of the ACM on Human-Computer Interaction, 5(CSCW2). doi: 10.1145/3479552
Ma, K., and Cao, J. (2019). “Design pattern as a practical tool for designing adaptive interactions connecting human and social robots,” in Intelligent Human Systems Integration 2019: Proceedings of the 2nd International Conference on Intelligent Human Systems Integration (IHSI 2019): Integrating People and Intelligent Systems, February 7-10, 2019, San Diego, California, USA (Springer International Publishing), 613–617. doi: 10.1007/978-3-030-11051-2_93
Mackeprang, M., Müller-Birn, C., and Stauss, M. (2019). “Discovering the sweet spot of human–computer configurations: a case study in information extraction,” in Proceedings of the ACM on Human-Computer Interaction, 3(CSCW). doi: 10.1145/3359297
Magnusson, M. S. (2018). Temporal Patterns in Interactions: T-Patterns and Their Detection with THEMETM. Cambridge: Cambridge University Press, 323–353. doi: 10.1017/9781316286302.017
Maier, T., Menold, J., and McComb, C. (2022). The relationship between performance and trust in AI in E-Finance. Front. Artif. Intell. 5:891529. doi: 10.3389/frai.2022.891529
Matthiesen, S., Diederichsen, S. Z., Hansen, M. K. H., Villumsen, C., Lassen, M. C. H., Jacobsen, P. K., et al. (2021). Clinician preimplementation perspectives of a decision-support tool for the prediction of cardiac arrhythmia based on machine learning: near-live feasibility and qualitative study. JMIR Hum. Factors 8:e26964. doi: 10.2196/26964
Molina, M. D., and Sundar, S. S. (2022). When AI moderates online content: effects of human collaboration and interactive transparency on user trust. J. Comput. Med. Commun. 27:zmac010. doi: 10.1093/jcmc/zmac010
Morrison, K., Shin, D., Holstein, K., and Perer, A. (2023). Evaluating the impact of human explanation strategies on human-AI visual decision-making. Proc. ACM Hum. -Comput. Interact. 7, 1–37. doi: 10.1145/3579481
Naiseh, M., Al-Thani, D., Jiang, N., and Ali, R. (2023). How the different explanation classes impact trust calibration: the case of clinical decision support systems. Int. J. Hum. Comput. Stud. 169:102941. doi: 10.1016/j.ijhcs.2022.102941
Nguyen, A. T., Kharosekar, A., Krishnan, S., Tate, E., Wallace, B. C., and Lease, M. (2018). “Believe it or not: Designing a human-ai partnership for mixed-initiative fact-checking,” in Proceedings of the 31st Annual ACM Symposium on User Interface Software and Technology, 189–199. doi: 10.1145/3242587.3242666
Nourani, M., King, J., and Ragan, E. (2020). “The role of domain expertise in user trust and the impact of first impressions with intelligent systems,” in Proceedings of the AAAI Conference on Human Computation and Crowdsourcing, 112–121. doi: 10.1609/hcomp.v8i1.7469
Nourani, M., Roy, C., Block, J. E., Honeycutt, D. R., Rahman, T., Ragan, E., et al. (2021). “Anchoring bias affects mental model formation and user reliance in explainable AI systems,” in Proceedings of the 26th International Conference on Intelligent User Interfaces, 340–350. doi: 10.1145/3397481.3450639
Panigutti, C., Beretta, A., Giannotti, F., and Pedreschi, D. (2022). “Understanding the impact of explanations on advice-taking: a user study for AI-based clinical decision support systems,” in Proceedings of the 2022 CHI Conference on Human Factors in Computing Systems, 1–9. doi: 10.1145/3491102.3502104
Parasuraman, R., Sheridan, T. B., and Wickens, C. D. (2000). A model for types and levels of human interaction with automation. IEEE Trans. Syst. Man Cybern. 30, 286–297. doi: 10.1109/3468.844354
Park, J. S., Barber, R., Kirlik, A., and Karahalios, K. (2019). A slow algorithm improves users' assessments of the algorithm's accuracy. Proc. ACM Hum. -Comput. Interact. 3, 1–15. doi: 10.1145/3359204
Peng, A., Nushi, B., Kiciman, E., Inkpen, K., and Kamar, E. (2022). “Investigations of performance and bias in Human-AI teamwork in hiring,” in Proceedings of the AAAI Conference on Artificial Intelligence, 12089–12097. doi: 10.1609/aaai.v36i11.21468
Porat, T., Marshall, I. J., Sadler, E., Vadillo, M. A., McKevitt, C., Wolfe, C. D. A., et al. (2019). Collaborative design of a decision aid for stroke survivors with multimorbidity: a qualitative study in the UK engaging key stakeholders. BMJ Open 9:e030385. doi: 10.1136/bmjopen-2019-030385
Prabhudesai, S., Yang, L., Asthana, S., Huan, X., Vera Liao, Q., and Banovic, N. (2023). “Understanding uncertainty: how lay decision-makers perceive and interpret uncertainty in Human-AI decision making,” in Proceedings of the 28th International Conference on Intelligent User Interfaces, 379–396. doi: 10.1145/3581641.3584033
Rahman, M. A., Sadat, S. N., Asyhari, A. T., Refat, N., Kabir, M. N., and Arshah, R. A. (2021). A secure and sustainable framework to mitigate hazardous activities in online social networks. IEEE Trans. Sustain. Comput. 6, 30–42. doi: 10.1109/TSUSC.2019.2911188
Rastogi, C., Zhang, Y., Wei, D., Varshney, K. R., Dhurandhar, A., and Tomsett, R. (2022). “Deciding fast and slow: the role of cognitive biases in AI-assisted decision-making,” in Proceedings of the ACM on Human-Computer Interaction, 6(CSCW1). doi: 10.1145/3512930
Rechkemmer, A., and Yin, M. (2022). “When confidence meets accuracy: exploring the effects of multiple performance indicators on trust in machine learning models,” in Proceedings of the 2022 Chi Conference on Human Factors in Computing Systems, 1–14. doi: 10.1145/3491102.3501967
Reverberi, C., Rigon, T., Solari, A., Hassan, C., Cherubini, P., and Cherubini, A. (2022). Experimental evidence of effective human-AI collaboration in medical decision-making. Sci. Rep. 12:14952. doi: 10.1038/s41598-022-18751-2
Riveiro, M., and Thill, S. (2021). “That's (not) the output I expected!” on the role of end user expectations in creating explanations of AI systems. Artif. Intell. 298:103507. doi: 10.1016/j.artint.2021.103507
Riveiro, M., and Thill, S. (2022). “The challenges of providing explanations of AI systems when they do NotBehave like users expect,” in Proceedings of the 30th ACM Conference on User Modeling, Adaptation and Personalization, 110–120. doi: 10.1145/3503252.3531306
Robbemond, V., Inel, O., and Gadiraju, U. (2022). “Understanding the role of explanation modality in AI-AssistedDecision-Making,” in Proceedings of the 30th ACM Conference on User Modeling, Adaptation and Personalization, 223–233. doi: 10.1145/3503252.3531311
Sauppé, A., and Mutlu, B. (2014). “Design patterns for exploring and prototyping human-robot interactions,” in Proceedings of the SIGCHI Conference on Human Factors in Computing Systems, 1439–1448. doi: 10.1145/2556288.2557057
Schaekermann, M., Beaton, G., Sanoubari, E., Lim, A., Larson, K., and Law, E. (2020). “Ambiguity-aware AI assistants for medical data analysis,” in Proceedings of the 2020 CHI Conference on Human Factors in Computing Systems, 1–14. doi: 10.1145/3313831.3376506
Schemmer, M., Kuehl, N., Benz, C., Bartos, A., and Satzger, G. (2023). “Appropriate reliance on AI advice: Conceptualization and the effect of explanations,” in International Conference on Intelligent User Interfaces, inproceedings IUI, 410–422. doi: 10.1145/3581641.3584066
Schleidgen, S., Friedrich, O., Gerlek, S., Assadi, G., and Seifert, J. (2023). The concept of “interaction” in debates on human-machine interaction. Human. Soc. Sci. Commun. 10, 1–13. doi: 10.1057/s41599-023-02060-8
Schrills, T., and Franke, T. (2020). “Color for characters - effects of visual explanations of AI on trust and observability,” in Artificial Intelligence in HCI: First International Conference, AI-HCI 2020, Held as Part of the 22nd HCI International Conference, HCII 2020, Copenhagen, Denmark, July 19–24, 2020, Proceedings 22 (Springer International Publishing), 121–135. doi: 10.1007/978-3-030-50334-5_8
Sheu, R.-K., and Pardeshi, M. S. (2022). A survey on medical explainable AI (XAI): recent progress, explainability approach, human interaction and scoring system. Sensors 22:8068. doi: 10.3390/s22208068
Silva, A., Schrum, M., Hedlund-Botti, E., Gopalan, N., and Gombolay, M. (2023). Explainable artificial intelligence: evaluating the objective and subjective impacts of xAI on Human-Agent Interaction 39, 1390–1404. doi: 10.1080/10447318.2022.2101698
Silva-Rodríguez, V., Nava-Mu noz, S. E., Castro, L. A., Martínez-Pérez, F. E., Pérez-González, H. G., and Torres-Reyes, F. (2020). Classifying design-level requirements using machine learning for a recommender of interaction design patterns. IET Softw. 14, 544–552. doi: 10.1049/iet-sen.2019.0291
Sims, R. (1997). Interactivity: a forgotten art? Comput. Human Behav. 13, 157–180. doi: 10.1016/S0747-5632(97)00004-6
Smith-Renner, A., Fan, R., Birchfield, M., Wu, T., Boyd-Graber, J., Weld, D. S., et al. (2020). “No explainability without accountability: an empirical study of explanations and feedback in interactive ML,” in Proceedings of the 2020 CHI Conference on Human Factors in Computing Systems, 1–13. doi: 10.1145/3313831.3376624
Stites, M. C., Nyre-Yu, M., Moss, B., Smutz, C., and Smith, M. R. (2021). “Sage advice? The impacts of explanations for machine learning models on human Decision-Making in spam detection,” in International Conference on Human-Computer Interaction (Cham: Springer International Publishing), 269–284. doi: 10.2172/1878725
Suresh, H., Lao, N., and Liccardi, I. (2020). “Misplaced trust: Measuring the interference of machine learning in human Decision-Making,” in Proceedings of the 12th ACM Conference on Web Science, 315–324. doi: 10.1145/3394231.3397922
Suresh, H., Lewis, K. M., Guttag, J., and Satyanarayan, A. (2022). “Intuitively assessing ML model reliability through Example-Based explanations and editing model inputs,” in Proceedings of the 27th International Conference on Intelligent User Interfaces, 767–781. doi: 10.1145/3490099.3511160
Tejeda, H., Kumar, A., Smyth, P., and Steyvers, M. (2022). Ai-assisted decision-making: a cognitive modeling approach to infer latent reliance strategies. Comput. Brain Behav. 5, 491–508. doi: 10.1007/s42113-022-00157-y
Tolmeijer, S., Christen, M., Kandul, S., Kneer, M., and Bernstein, A. (2022). “Capable but amoral? Comparing AI and human expert collaboration in ethical decision making,” in Proceedings of the 2022 CHI Conference on Human Factors in Computing Systems, 1–17. doi: 10.1145/3491102.3517732
Tolmeijer, S., Gadiraju, U., Ghantasala, R., Gupta, A., and Bernstein, A. (2021). “Second chance for a first impression? Trust development in intelligent system interaction,” in Proceedings of the 29th ACM Conference on User Modeling, Adaptation and Personalization, 77–87. doi: 10.1145/3450613.3456817
Tschandl, P., Rinner, C., Apalla, Z., Argenziano, G., Codella, N., Halpern, A., et al. (2020). Human-computer collaboration for skin cancer recognition. Nat. Med. 26, 1229–1234. doi: 10.1038/s41591-020-0942-0
Tutul, A. A., Nirjhar, E. H., and Chaspari, T. (2021). “Investigating trust in Human-Machine learning collaboration: a pilot study on estimating public anxiety from speech,” in Proceedings of the 2021 International Conference on Multimodal Interaction, 288–296. doi: 10.1145/3462244.3479926
van Bekkum, M., de Boer, M., van Harmelen, F., Meyer-Vitali, A., and Teije, A. t. (2021). Modular design patterns for hybrid learning and reasoning systems: a taxonomy, patterns and use cases. Appl. Intell. 51, 6528–6546. doi: 10.1007/s10489-021-02394-3
Van Berkel, N., Opie, J., Ahmad, O. F., Lovat, L., Stoyanov, D., and Blandford, A. (2022). “Initial responses to false positives in AI-Supported continuous interactions: a colonoscopy case study,” in ACM Transactions on Interactive Intelligent Systems, 12. doi: 10.1145/3480247
Van Berkel, N., Skov, M. B., and Kjeldskov, J. (2021). Human-AI interaction: intermittent, continuous, and proactive. Interactions 28, 67–71. doi: 10.1145/3486941
van de Sande, D., Chung, E. F. F., Oosterhoff, J., van Bommel, J., Gommers, D., and van Genderen, M. E. (2024). To warrant clinical adoption AI models require a multi-faceted implementation evaluation. NPJ Digital Med. 7:58. doi: 10.1038/s41746-024-01064-1
van den Brandt, A., Christopher, M., Zangwill, L. M., Rezapour, J., Bowd, C., Baxter, S. L., et al. (2020). “GLANCE: visual analytics for monitoring glaucoma progression,” in VCBM, 85–96.
van der Waa, J., Verdult, S., van den Bosch, K., van Diggelen, J., Haije, T., van der Stigchel, B., et al. (2021). Moral decision making in human-agent teams: human control and the role of explanations. Front. Robot. AI 8:640647. doi: 10.3389/frobt.2021.640647
Vereschak, O., Bailly, G., and Caramiaux, B. (2021). “How to evaluate trust in ai-assisted decision making? A survey of empirical methodologies,” in Proceedings of the ACM on Human-Computer Interaction, 1–39. doi: 10.1145/3476068
Vodrahalli, K., Daneshjou, R., Gerstenberg, T., and Zou, J. (2022). “Do humans trust advice more if it comes from AI? An analysis of human-AI interactions,” in Proceedings of the 2022 AAAI/ACM Conference on AI, Ethics, and Society, 763–777. doi: 10.1145/3514094.3534150
Vössing, M., Kühl, N., Lind, M., and Satzger, G. (2022). Designing transparency for effective Human-AI collaboration. Inf. Syst. Front. 24, 877–895. doi: 10.1007/s10796-022-10284-3
Wang, R., Fu, G., Li, J., and Pei, Y. (2022). Diagnosis after zooming in: a multilabel classification model by imitating doctor reading habits to diagnose brain diseases. Med. Phys. 49, 7054–7070. doi: 10.1002/mp.15871
Wang, X., and Yin, M. (2021). “Are explanations helpful? A comparative study of the effects of explanations in AI-Assisted Decision-Making,” in Proceedings of the 26th International Conference on Intelligent User Interfaces, 318–328. doi: 10.1145/3397481.3450650
Wen, W., and Imamizu, H. (2022). The sense of agency in perception, behaviour and human-machine interactions. Nat. Rev. Psychol. 1, 211–222. doi: 10.1038/s44159-022-00030-6
Westphal, M., Vössing, M., Satzger, G., Yom-Tov, G. B., and Rafaeli, A. (2023). Decision control and explanations in human-AI collaboration: improving user perceptions and compliance. Comput. Human Behav. 144:107714. doi: 10.1016/j.chb.2023.107714
Wu, Y., Kim, K. J., and Mou, Y. (2022). Minority social influence and moral decision-making in human-AI interaction: the effects of identity and specialization cues. New Media Soc. 26, 5619–5637. doi: 10.1177/14614448221138072
Xiong, W., Wang, C., and Ma, L. (2023). Partner or subordinate? Sequential risky decision-making behaviors under human-machine collaboration contexts. Comput. Human Behav. 139:107556. doi: 10.1016/j.chb.2022.107556
Yi, J. S., ah Kang, Y., Stasko, J., and Jacko, J. A. (2007). Toward a deeper understanding of the role of interaction in information visualization. IEEE Trans. Vis. Comput. Graph. 13, 1224–1231. doi: 10.1109/TVCG.2007.70515
Yu, K., Berkovsky, S., Taib, R., Zhou, J., and Chen, F. (2019). “Do I trust my machine teammate? An investigation from perception to decision,” in Proceedings of the 24th International Conference on Intelligent User Interfaces, 460–468. doi: 10.1145/3301275.3302277
Zhang, Q., Lee, M. L., and Carter, S. (2022). “You complete me: Human-AI teams and complementary expertise,” in Proceedings of the 2022 CHI Conference on Human Factors in Computing Systems, 1–28. doi: 10.1145/3491102.3517791
Zhang, W., and Lim, B. Y. (2022). “Towards relatable explainable AI with the perceptual process,” in Proceedings of the 2022 CHI Conference on Human Factors in Computing Systems, 1–24. doi: 10.1145/3491102.3501826
Zhang, Y., Liao, Q. V., and Bellamy, R. K. (2020). “Effect of confidence and explanation on accuracy and trust calibration in AI-assisted decision making,” in Proceedings of the 2020 Conference on Fairness, Accountability, and Transparency, 295–305. doi: 10.1145/3351095.3372852
Zhou, J., Arshad, S. Z., Luo, S., and Chen, F. (2017). “Effects of uncertainty and cognitive load on user trust in predictive decision making,” in Human-Computer Interaction INTERACT 2017: 16th IFIP TC 13 International Conference, Mumbai, India, September 25-29, 2017, Proceedings, Part IV 16 (Springer International Publishing), 23–39. doi: 10.1007/978-3-319-68059-0_2
Keywords: artificial intelligence, human-AI interaction, decision-making, interaction patterns, interactivity
Citation: Gomez C, Cho SM, Ke S, Huang C-M and Unberath M (2025) Human-AI collaboration is not very collaborative yet: a taxonomy of interaction patterns in AI-assisted decision making from a systematic review. Front. Comput. Sci. 6:1521066. doi: 10.3389/fcomp.2024.1521066
Received: 01 November 2024; Accepted: 06 December 2024;
Published: 06 January 2025.
Edited by:
Stefan Kopp, Bielefeld University, GermanyReviewed by:
Styliani Kleanthous, Open University of Cyprus, CyprusCopyright © 2025 Gomez, Cho, Ke, Huang and Unberath. This is an open-access article distributed under the terms of the Creative Commons Attribution License (CC BY). The use, distribution or reproduction in other forums is permitted, provided the original author(s) and the copyright owner(s) are credited and that the original publication in this journal is cited, in accordance with accepted academic practice. No use, distribution or reproduction is permitted which does not comply with these terms.
*Correspondence: Mathias Unberath, dW5iZXJhdGhAamh1LmVkdQ==
Disclaimer: All claims expressed in this article are solely those of the authors and do not necessarily represent those of their affiliated organizations, or those of the publisher, the editors and the reviewers. Any product that may be evaluated in this article or claim that may be made by its manufacturer is not guaranteed or endorsed by the publisher.
Research integrity at Frontiers
Learn more about the work of our research integrity team to safeguard the quality of each article we publish.