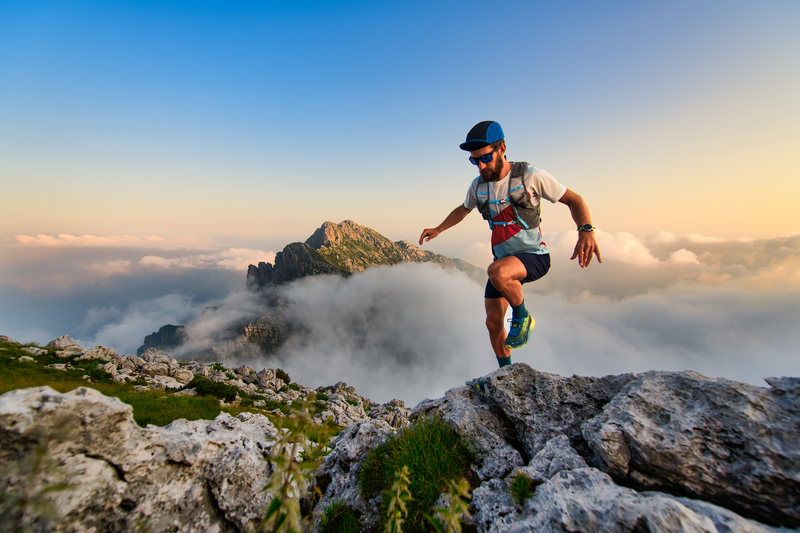
94% of researchers rate our articles as excellent or good
Learn more about the work of our research integrity team to safeguard the quality of each article we publish.
Find out more
ORIGINAL RESEARCH article
Front. Comput. Sci. , 07 January 2025
Sec. Human-Media Interaction
Volume 6 - 2024 | https://doi.org/10.3389/fcomp.2024.1499672
With the rapid development of digital technology, online fitness live streaming platforms have become effective tools for helping users enhance their physical activity and health. However, the attitudes of Generation Z users, who form a significant portion of the platform’s user base, remain unclear. Identifying the factors influencing Generation Z’s use of these platforms and improving user satisfaction can assist in refining platform design and services, promoting sustainable development. A mixed-methods approach was employed to collect 1,788 user reviews, which were consolidated into 40 items. A questionnaire was then distributed to 314 respondents. In the first round, 165 open-ended questionnaires were distributed to identify specific influencing factors through factor analysis, leading to the development of a user experience evaluation scale. In the second round, 314 scale-based questionnaires were distributed, and structural equation modeling (SEM) was employed to explore the relationships between various factors and user satisfaction, resulting in the construction of a user satisfaction model. The factors influencing Generation Z users’ engagement with the platforms include social interaction, usefulness, convenience, functional quality, and technical quality. Among these, social interaction is the most critical factor affecting user satisfaction. Generation Z has unique needs regarding digital platforms. Therefore, platforms should provide services tailored to their psychological and behavioral characteristics, optimize business operation models, and further enhance the user experience.
With the rapid advancement of internet and video streaming technologies, live streaming has become a highly popular form of social media (Giertz et al., 2022). This trend was particularly evident during the COVID-19 pandemic when many individuals turned to online fitness platforms for physical exercise (Parker et al., 2021), as a substitute for the inaccessibility of gyms (Nieman and Wentz, 2019; Damaševičius et al., 2022). As a result, fitness live streaming has emerged rapidly (Bentlage et al., 2020; Schmidt et al., 2020; Mutz et al., 2021), becoming a key solution for online fitness and an essential means for maintaining a healthy lifestyle (Petersen et al., 2019; Gao et al., 2020; Nyenhuis et al., 2020). Over the past five years, fitness live streaming has evolved into a prominent trend, significantly fueling public interest in fitness through its interactive viewing, communication, and sharing experiences (Fearnbach et al., 2021). Numerous users have engaged in real-time fitness training sessions through platforms such as Zoom (Guo and Fussell, 2022), Facebook Live (Gilmour et al., 2020), and YouTube Live (Jong and Drummond, 2020; Nyenhuis et al., 2020), meeting the needs of home-based fitness. The popularity of online live-streamed workout sessions has increased significantly, with over 85% of gym members now regularly exercising at home (Gottschall and Hastings, 2023), primarily due to the greater time flexibility and convenience these platforms offer (Silva-Jose et al., 2022). Additionally, several studies have shown that using online fitness platforms can mitigate the negative effects of reduced physical activity, help reduce health risks (Glynn et al., 2014; Yang and Koenigstorfer, 2020), and minimize the environmental pollution and resource waste associated with the construction and maintenance of sports facilities (Bralewska et al., 2022; Zhu et al., 2023), thereby promoting ecological sustainability.
In China, the live streaming market has grown at an exceptionally rapid pace, even outpacing other global regions (Li et al., 2021). In 2021, the Chinese government introduced the “National Fitness Plan (2021–2025)” (Menhas et al., 2021), recognizing fitness live streaming as a crucial tool to address the lack of physical activity among Chinese residents (Tian et al., 2016; Chen et al., 2019), aiming to improve personal physical fitness and enhance resistance to various diseases (Gao et al., 2020). Consequently, online fitness platforms like Keep, Spax, Fit, and Douyin in China have rapidly developed, offering more professional and interactive fitness live streaming experiences. This underscores the significant potential and broad application prospects of fitness live streaming as an emerging fitness method, which has quickly spurred further growth in the fitness industry.
The target user group for this study is Generation Z (Gen Z), born between the mid-1990s and early 2010s. As most physical, developmental, and social changes occur during this period (Stanes et al., 2015), Generation Z exhibits distinctive characteristics, making them a significant demographic in the contemporary market (Kymäläinen et al., 2021). Research indicates that this group’s consumer behavior is rapidly evolving, showing potential for adopting sustainable consumption habits (Fischer et al., 2017). Simultaneously, Generation Z has grown up during a time of rapid advancements in digital technology, leading to a lifestyle that heavily relies on technology (Hernandez-de-Menendez et al., 2020). They are accustomed to engaging in various activities such as learning, sharing, and exercising through online platforms. In terms of fitness, sports have become an integral part of the daily lives of Chinese Generation Z members. They choose sports activities to express their individuality and identity, with a strong desire for experience and social interaction during exercise (Wei, 2023). Consequently, Generation Z not only excels in digital technology and favors personalized experiences but also pursues a social lifestyle centered around fitness (Lienher, 2022), making them a critical driving force in influencing consumption trends and behaviors. Therefore, exploring the factors that influence Generation Z users’ engagement with online fitness live streaming platforms is crucial. It helps companies understand the psychological needs and behaviors of this emerging consumer group, optimize platform design and services, and promote the development of online fitness industries.
Meanwhile, marketing research has found that the primary reason consumers decide to repurchase a product or reuse a service is their level of satisfaction (Bearden and Teel, 1983; Oliver, 1993; Szymanski and Henard, 2001). User satisfaction is the comprehensive subjective evaluation of users’ experiences during their use of a fitness live streaming platform, having the most direct and significant impact on customer loyalty and willingness to continue using the platform (Lee, 2010; Mouakket and Bettayeb, 2015). It is the ultimate goal pursued by any technology provider. It can be concluded that understanding the consumer psychology and behavioral logic behind the use of fitness live streaming platforms can further enhance the platform’s marketing strategies. Although much research has been conducted on online fitness live streaming platforms, most of these studies focus on platform characteristics (Trent, 2016; Harris and Metzler, 2019; Guo and Fussell, 2022), the impact on users’ physical activity (Parker et al., 2021; Hsu et al., 2022; Liu et al., 2022), and interventions in health behavior (Riley et al., 2011; Petersen et al., 2019; Chang et al., 2021). There is a clear gap in research from the perspective of user experience, specifically examining Generation Z consumers’ usage behavior of fitness live streaming platforms. Therefore, this study aims to explore the relationship between platform attributes and user satisfaction, providing insights to help providers design strategies that can increase Generation Z’s usage of fitness live streaming platforms, thereby effectively promoting the operation and development of these platforms.
This study will adopt a mixed-methods approach, combining qualitative and quantitative research. Through qualitative methods such as user surveys and online open-ended questionnaires, we will identify the factors that users consider when evaluating fitness live streaming platforms and develop appropriate evaluation scales. Subsequently, data analysis and hypothesis testing in quantitative research will provide empirical support for the findings from qualitative analysis. In this process, factor analysis will be used to reduce the dimensionality of variables in the survey questionnaire, and structural equation modeling will further clarify the relationships among the influencing factors. This will lead to the construction of a theoretical model of user satisfaction for fitness live streaming platforms.
This study, from the perspective of user experience, comprehensively explores the psychological needs and behavioral patterns of Generation Z consumers, identifying the key factors that influence user satisfaction. It helps us better understand the mechanisms behind consumer decision-making. By examining the current trends in online fitness and using fitness live streaming platforms as an example, the paper delves into the intrinsic connection between platform attributes and user experience, filling a gap in the research on online fitness platforms. The findings of this study also assist fitness live streaming platform operators in formulating effective marketing strategies, enhancing market competitiveness while promoting the development of the online fitness industry and improving the overall physical health of the population. Therefore, this study makes contributions both in theory and practice.
Over the past decade, the fitness industry has undergone profound transformations, transitioning from a model primarily based on traditional gyms and face-to-face coaching to one increasingly centered on online fitness courses and live streaming training (Guo and Fussell, 2022). The rapid advancement of technology has driven continuous iterations in online fitness instruction, significantly enhancing user experience and broadening its reach (Yu and Song, 2024). Notably, during the COVID-19 pandemic, fitness live streaming emerged as a flexible and convenient approach to physical activity, quickly becoming a preferred choice for many individuals seeking to stay active (Schmidt et al., 2020). Presently, the global fitness live streaming market continues to grow, with particularly strong expansion potential observed in the Asia-Pacific region (Tian et al., 2022). Moreover, this model has gained widespread user favor due to its convenience and social interaction (Hsu et al., 2022), while its usefulness and effectiveness have been validated by empirical research (Glynn et al., 2014; Liu et al., 2022). In the post-pandemic era, although some users have gradually returned to offline fitness venues, fitness live streaming platforms have retained significant appeal owing to their unique features and the influence of prominent fitness influencers (Yu and Song, 2024). This has led to the emergence of hybrid “online + offline” models designed to accommodate diverse user fitness needs and preferences.
In recent years, academic research on fitness live streaming has increased significantly, providing multifaceted theoretical support for the development of this field. Some studies have focused on platform characteristics. For example, Guo and Fussell (2022), through semi-structured interviews, analyzed the technical and interactive challenges faced by users participating in live-streamed group fitness classes and proposed design optimization recommendations. Similarly, Trent (Trent, 2016) conducted a descriptive study examining the alignment of virtual fitness courses with curriculum design standards and suggested improving platform functionalities to better meet industry requirements. On the other hand, the role of fitness live streaming in promoting physical activity has also been empirically supported. Liu et al. (2022) found that live-streamed fitness classes significantly encouraged users to engage in physical exercise during COVID-19 lockdowns, helping them achieve recommended levels of physical activity. Likewise, Parker et al. (2021) discovered that adults and adolescents using fitness live streaming platforms were more likely to meet guidelines for Moderate-to-Vigorous Physical Activity (MVPA), Muscle-Strengthening Exercises (MSE), and comprehensive physical activity. Gottschall and Hastings (2023) further explored the physiological intensity and psychological experiences of group classes in fitness live streaming. Their findings revealed that live-streamed classes outperformed streaming and on-demand modes in terms of perceived exertion, enjoyment, and satisfaction.
Additionally, behavioral intervention studies targeting specific populations have highlighted the broad application potential of fitness live streaming. For instance, Chang et al. (2021), through a non-randomized controlled trial, demonstrated that using Facebook live streaming to guide community-dwelling older adults in exercise significantly improved their functional fitness. Similarly, Hong et al. (2017) and Hong et al. (2018) developed a remote live-streamed exercise format that successfully enabled real-time interaction between fitness coaches and older adults in the community. They further validated its significant effects in improving sarcopenia-related body composition and functional health among elderly individuals. In the medical field, the potential clinical applications of fitness live streaming have also become a research hotspot. Gagnon et al. (2023) and Kenis-Coskun et al. (2022), through clinical trials, investigated the effectiveness of fitness live streaming in improving the physical conditions of specific populations, such as patients with Duchenne muscular dystrophy (DMD) and stroke survivors. Their findings provide strong evidence supporting the adoption of fitness live streaming in healthcare contexts.
Despite the numerous advantages of fitness live streaming in promoting physical activity and enhancing user experience revealed by existing studies, there remains a lack of in-depth exploration into the driving factors behind user behavior and the systematic evaluation of user experience. Most research has focused on the direct relationship between platform functionality and physical activity, while overlooking the mechanisms influencing users’ acceptance and usage behavior of fitness live streaming platforms. Addressing this research gap, the present study employs factor analysis and structural equation modeling to systematically investigate the key factors influencing users’ engagement with fitness live streaming platforms. The findings aim to provide theoretical foundations and practical recommendations for optimizing platform design and improving service quality.
In studies focusing on online fitness platforms, various behavioral theoretical models have been widely applied, offering multidimensional perspectives for understanding user behavior. For example, Hsu et al. (2022), using the Unified Theory of Acceptance and Use of Technology (UTAUT) model, analyzed the behavior of Facebook fitness live streaming platform users and developed a framework for user engagement behavior. Their findings revealed that the core factors of the UTAUT model had significant positive effects on users’ behaviors when using fitness live streaming platforms. This study provides theoretical support for optimizing user experience and enhancing user retention on fitness live streaming platforms. Tian et al. (2022) employed natural language processing techniques and adopted the BiLSTM-CNN model to conduct sentiment analysis on fitness live streaming-related posts on Weibo from July 2021 to June 2022, measuring the polarity of public sentiment (positive and negative). The results indicated that the Chinese public generally held a positive attitude toward fitness live streaming but expressed dissatisfaction regarding issues such as fitness injuries, celebrity influence, commercialization of live streaming, and COVID-19 policies. Based on these findings, the study proposed strategies to promote public health communication, offering practical guidance for future fitness live streaming content design and health communication strategies.
Menhas et al. (2023) integrated Self-Determination Theory (SDT), Social Cognitive Theory (SCT), the Technology Acceptance Model (TAM), and the Health Behavior Change Model to explore the impact of fitness applications on the interconnections between COVID-19 prevention strategies, virtual reality workouts, physical health, and mental well-being in Chinese society. Their study underscored the multidimensional value of fitness technologies in crisis contexts. Chiu et al. (2024), adopting a Cognitive Appraisal Theory perspective, investigated the intricate relationships between cognitive elements and emotional outcomes in fitness live streaming. Their findings revealed that these cognitive and emotional factors jointly shaped users’ exercise intentions, highlighting the platform’s potential in motivating exercise behaviors. Furthermore, Chen et al. (2024), based on the Stimulus-Organism-Response (SOR) framework, examined how visibility affordance in the context of cloud fitness indirectly influences users’ willingness to exercise alongside fitness influencers through psychological variables. This study extended the application scenarios of the SOR theory within the fitness domain.
It is evident that previous research on fitness live streaming platforms and online fitness applications has predominantly employed quantitative research methods, formulating hypotheses based on social phenomena or literature reviews and validating them through quantitative data analysis. However, such approaches cannot definitively identify the key influencing factors. Therefore, collecting authentic user evaluations of fitness live streaming platforms is essential. To address the limitations of traditional single-method quantitative research, this study adopts a mixed-methods approach. Through qualitative methods, including user interviews, user studies, and literature-based discussions, the study identifies the core factors that users prioritize when evaluating fitness live streaming platforms, thereby constructing a tailored user evaluation scale. Subsequently, quantitative methods, such as data analysis and hypothesis testing, are employed to further examine the interrelationships among these factors.
Satisfaction, as consumers’ overall evaluation of a product or service based on their personal consumption experiences, has long been a central theme in marketing and behavioral research. This is because satisfaction serves not only as a critical indicator of user experience but also has a direct impact on a company’s profitability and long-term growth (Descals et al., 2010). It is widely regarded as a key factor in building and maintaining a loyal consumer base (Nascimento et al., 2018), and its importance has been explicitly emphasized in numerous theoretical models. For instance, the Expectation-Confirmation Model proposed by Oliver (1980) and Bhattacherjee (2001) suggests that users’ willingness to continue using a service or system primarily depends on their satisfaction with prior experiences. Similarly, Locke (1973) satisfaction model further underscores the pivotal role of satisfaction in predicting consumer behavior. In the context of technology applications, satisfaction is even considered one of the core criteria for evaluating system success (Mouakket and Bettayeb, 2015; Cigdem and Ozturk, 2016).
In the context of fitness live streaming platforms, satisfaction directly influences whether users will continue using the platform. However, existing research has inadequately explored the relationship between the characteristics of fitness live streaming platforms and user satisfaction, particularly concerning the experiences and needs of Generation Z, a key user group. While a limited number of studies have examined the user experience of fitness live streaming platforms (Lee et al., 2013; Liao et al., 2023), these studies often rely on extracting factors from the literature and testing hypotheses. This approach is constrained by the subjectivity of factor selection, limiting the comprehensiveness of the research and failing to construct a complete and scientifically robust user experience evaluation scale. Therefore, it is essential to adopt more systematic and comprehensive research methods to thoroughly investigate the key factors affecting user satisfaction with fitness live streaming platforms.
Through a review of existing studies, this paper makes the following contributions: (1) By combining qualitative and quantitative research, this study systematically identifies the key factors influencing users’ engagement with fitness live streaming platforms through an integrated approach that includes literature review, user research, and data analysis. Based on these findings, a user evaluation scale tailored to fitness live streaming platforms is developed. Unlike traditional research methods, this study adopts a multi-method approach to ensure that the identified factors are both comprehensive and practically significant, addressing the limitations of relying solely on quantitative methods to test hypotheses. (2) The study further explores the relationships between these key factors and user satisfaction, constructing a theoretical model of satisfaction for fitness live streaming platforms. Existing literature contains limited theoretical research on user satisfaction in the context of fitness live streaming, and this model fills a critical academic gap in this field, providing a reference framework for future research. (3) This study specifically focuses on Generation Z users in China, systematically examining their attitudes and needs toward fitness live streaming platforms. As a primary consumer group in the digital age, Generation Z exhibits distinctive behaviors and preferences, yet research in this area remains scarce. This study provides theoretical support for understanding the preferences and behaviors of Generation Z users, while offering practical recommendations for optimizing platform design and operations to better meet their needs.
The purpose of this study is to identify the factors that influence Generation Z consumers’ use of fitness live streaming platforms and how these factors affect their user satisfaction experience. The research process primarily references the experimental methods of Wei et al. (2020) and Wang et al. (2022). The entire study is divided into three parts, as shown in Figure 1. In the first part, extensive feedback was collected from Generation Z users with experience using fitness live streaming platforms. Their evaluations were combined with observation variables from the literature review to form an initial set of evaluation items. These items were then used as an evaluation scale for a second-round survey, where factor analysis was employed to extract influencing factors, determine the specific elements impacting users’ use of fitness live streaming platforms, and develop a user experience evaluation scale. The second part involves constructing a satisfaction model. Based on a review of relevant literature, hypotheses were proposed. The “satisfaction” evaluation scale, adapted from established questionnaires, was combined with the scale from the first part to gather user feedback. The hypotheses were then tested using structural equation modeling (SEM). The third part focuses on the discussion of results. It begins by naming the factors derived from the first part through a comprehensive literature review to establish the specific dimensions of the evaluation scale. The relationships between the various elements are then explored based on the analysis results from the second part. Finally, this study provides constructive suggestions for the design and operation of fitness live streaming platforms. The questionnaires used during the data collection phase were evaluated using a seven-point Likert scale, where 1 represents “strongly disagree” and 7 represents “strongly agree.”
From May to July 2024, this study conducted in-depth interviews with 18 users of fitness live streaming platforms at a university in Jiangsu Province, China, using random sampling. The participants included 10 males and 8 females, all born between 1995 and 2010. Prior to the study, the research team conducted a screening process to ensure that participants maintained a healthy level of physical activity, possessed good fitness habits, and had at least one month of experience using fitness live streaming platforms. In terms of platform distribution, 6 participants used the Keep platform, 4 used Douyin, 4 used the Fit platform, and 4 used Spax. To address potential limitations arising from gender and occupational differences, the study also distributed 180 open-ended questionnaires via the online platform Credamo, targeting users across China. After excluding invalid responses, 165 valid questionnaires were obtained, aiming to collect random evaluations of Fitness Live Streaming platforms from Generation Z users. The online questionnaire adopted the same screening criteria as the in-person interviews and included geographical restrictions to ensure nationwide coverage and avoid regional sampling bias. The questionnaire results revealed that among the respondents, 33 were male and 132 were female, representing a diverse range of occupations. The largest group consisted of private enterprise employees (57.57%), followed by students (20.60%). Regarding platform preferences, Keep was the most commonly used platform (38 respondents), followed by Douyin (32 respondents), Yue Dong Quan (27 respondents), the Fit platform (25 respondents), Xiaohongshu (20 respondents), Spax (14 respondents), and Daily Yoga (9 respondents). Both the interview sample size (Slaton et al., 2017) and the open-ended questionnaire sample size (Wei et al., 2020) met the standards for experimental research. However, due to the adoption of fully random sampling, the study did not achieve complete balance in terms of gender and platform selection, which constitutes a limitation of this research.
After organizing and summarizing the data, the researchers released the questionnaire scale again for factor analysis. The questionnaire was still mainly collected through the “credamo” online platform, targeting Generation Z users. To ensure the coherence and consistency of respondents’ evaluations of the platform and satisfaction, the preliminary user experience evaluation scale was combined with widely used and well-established satisfaction scales (Lee and Jun, 2007; Zou et al., 2022) and distributed in a single questionnaire. A total of 350 questionnaires were collected. After removing invalid responses due to short completion time, repeated entries, or incomplete answers, 314 valid questionnaires remained. The survey response rate was 89.71%, and the sample size meets the requirements for factor analysis (Comrey and Lee, 2013; Lamash and Josman, 2020; Wei et al., 2020). Among the respondents, 38.21% were male, and 61.78% were female. Regarding educational background, 17.83% had a high school education or below, 61.46% held an associate or bachelor’s degree, and 20.70% had a master’s degree or higher. Detailed demographic information of the respondents is shown in Table 1. The initial user interviews were conducted by the study participants, and the content was summarized by three graduate students who were not involved in this study. These students merged similar or identical responses based on subjective evaluation during the content summarization phase of both the interviews and the online open-ended questionnaires. Upon completion, the summarized content was reviewed and assessed by two university professors with extensive research experience in the health field. These two professors were responsible for confirming the validity of the factor names during the naming discussions. Both experts have previously participated in related fitness research projects and have experience using fitness platforms for exercise, giving them a deep understanding of online fitness live streaming. They are from universities with nationally recognized key disciplines in design and have more than three years of teaching experience.
In terms of expert review criteria, relevant studies on the development of evaluation scales (Wang et al., 2022; Wang et al., 2024) were referenced. First, all item statements were required to be concise, unambiguous, and free from redundancy among different evaluations. Second, factor names had to employ professional academic terminology, clearly and accurately summarizing the items under each factor while ensuring distinct meanings across factors. Detailed information about the experts is shown in Table 2.
At the outset of this study, evaluations of fitness live streaming platforms were gathered through literature review, user interviews, and open-ended questionnaires. All respondents were selected using random sampling methods. Initially, a systematic review of relevant literature on fitness live streaming was conducted, and corresponding observational variables were extracted. The offline user interviews were semi-structured, with core interview questions listed in Table 3. Before the interviews began, respondents were informed about the study’s content and objectives, and they signed an informed consent form. During the online open-ended questionnaire phase, the informed consent form was included on the first page of the questionnaire, and respondents were required to read and agree to the study’s relevant considerations before completing the questionnaire.
The online open-ended survey targeted users who had experience with fitness live streaming platforms and had a certain demand for fitness activities. Before completing the questionnaire, respondents were informed of the specific demographic criteria for this study. The questionnaire included basic information and several open-ended questions, such as, “What do you think are the advantages or disadvantages of using a fitness live streaming platform for fitness? (Please provide at least three opinions)” and “What additional features do you think could be added to the current fitness live streaming platform to make it more efficient or to make you more willing to use live streaming for fitness?” The validity of the questionnaire was determined based on whether the respondents had experience using fitness live streaming platforms and whether they belonged to Generation Z. For clarity, this study refers to user evaluations as “items,” while the subsequent results from factor analysis are referred to as “factors.” Ultimately, 249 initial evaluations were collected during the literature search phase, resulting in 26 items after summarization; 154 initial evaluations were collected during the offline interview phase, leading to 50 items after summarization; and 1,385 initial evaluations were collected during the online open-ended questionnaire phase, resulting in 62 items after summarization, with all negative evaluations being rephrased into positive statements. After combining the results from the three parts, a total of 1,788 initial evaluations were summarized into 40 items.
Before distributing the second round of the online evaluation scale questionnaire, a pilot test was conducted to verify the questionnaire’s design validity. In this phase, 50 questionnaires were distributed offline, and respondents were invited to provide feedback on the logical flow and clarity of the questionnaire content. Overall feedback was positive. A preliminary analysis of this small sample revealed that both reliability and validity met the required standards. While factor entanglement was observed during the factor analysis, this was likely influenced by the limited sample size in the pilot test phase. Overall, the pilot test results aligned with expectations, allowing the study to proceed to the formal large-scale questionnaire phase. After the formal distribution, 350 responses were collected. Following screening, invalid responses—such as those with excessively short completion times, repeated selections across multiple items, or missing answers—were excluded, resulting in 314 valid questionnaires, with a validity rate of 89.71%. This sample size met the requirements for factor analysis, providing a robust data foundation for subsequent research.
This study utilized the professional data analysis software SPSS 26.0 to conduct factor analysis. From the 314 data samples, five factors with eigenvalues greater than 1 were identified. The rotated variance explanation rates for the five factors were 19.770, 16.197, 10.813, 10.437, and 9.188%, respectively, with a total explanation rate of 66.405%. The Kaiser-Meyer-Olkin (KMO) value was 0.955, which is greater than the acceptable threshold of 0.6 (Nunnally, 1994). The reliability of each factor and the overall questionnaire exceeded the standard of 0.6 (Eisinga et al., 2013). The degrees of freedom (df) were 210, and the p-value was 0.000, meeting the requirements of Bartlett’s Test of Sphericity (p < 0.05) (Nunnally, 1994). In the confirmatory factor analysis, all standardized load coefficients were greater than 0.6 (Muilenburg and Berge, 2005), and both the Average Variance Extracted (AVE) was greater than 0.36 (Fornell and Larcker, 1981b), and the Composite Reliability (CR) was greater than 0.7 (Hair et al., 2016).
Subsequently, this study employed Structural Equation Modeling (SEM) to test the hypotheses and explore the effects of various factors on Generation Z users’ satisfaction. Data processing was conducted using the professional analysis software SPSSAU. SEM offers significant advantages in handling complex relational structures and latent variable analysis and has been widely applied in social science research (Lu et al., 2022). Compared to traditional regression analysis, SEM enables simultaneous examination of complex relationships between multiple independent and dependent variables, including direct and mediating effects (Hair et al., 2021). Additionally, SEM can indirectly measure latent variables through multiple observable variables, providing a more comprehensive analytical perspective. In this study, the research team integrated 1,788 original user reviews into 40 observed variables and, through factor analysis, extracted five latent variables related to the characteristics of fitness live streaming platforms. By employing SEM, the study effectively addressed the complex causal relationships between these variables and user satisfaction, comprehensively validated the research hypotheses, and further optimized the model structure. This approach enhances the reliability and scientific rigor of the research findings.
First, a reliability analysis was conducted to ensure that the constructs in the questionnaire met the study’s reliability requirements. The analysis showed that the overall Cronbach’s α coefficient for the questionnaire was 0.969, indicating excellent reliability and suitability for further analysis (Graham-Rowe et al., 2012). Next, to determine whether the data were appropriate for factor analysis, the study performed the KMO test and Bartlett’s test of sphericity. The KMO value and Bartlett’s test are used to evaluate the correlations among variables in the sample data and whether these correlations can be explained through factor analysis. Generally, a KMO value greater than 0.6 is required, and for Bartlett’s test, a p-value below the significance level (0.05) indicates significant correlations among variables, making them suitable for factor analysis. Additionally, the degrees of freedom (df) reflect the complexity of the variable relationships, with higher df values indicating more variables involved in the analysis, which better captures the overall correlation among variables. A combination of high df values and significant p-values further enhances the reliability and explanatory power of the model.
The results of this analysis showed a KMO value of 0.965, a Bartlett’s test approximate chi-square value of 8279.311, a df value of 780, and a p-value of 0.00. These results meet the required criteria, indicating that the data were suitable for factor analysis (Nunnally, 1994).
Next, an exploratory factor analysis (EFA) was conducted using the Varimax rotation method to identify the relationship between factors and research items. When the communalities of all research items exceeded 0.4, it indicated a strong correlation between the items and the factors, allowing effective extraction of information. When the absolute value of the factor loadings exceeded 0.4 (Kaiser, 1958), it demonstrated a good correspondence between the item and the factor. Ideally, each research item should correspond to only one factor, and the absolute value of the factor loading for the corresponding factor should exceed 0.4.
Based on these criteria, the first round of factor extraction was conducted, resulting in the identification of five factors, with the explained variance after rotation being 22.492, 11.679, 11.044, 8.721, and 7.231%, respectively. The cumulative variance explained after rotation was 61.167%, and the eigenvalues of the factors were 8.997, 4.672, 4.418, 3.488, and 2.892, all of which were greater than 1. However, during the process of identifying the correspondence between items using Varimax, it was found that some items corresponded to multiple factors, indicating factor entanglement. This necessitated the removal of some entangled items to optimize the results (Hair et al., 2011; Costello and Osborne, 2019). During the deletion process, we referred to the experimental procedures outlined by Wang et al. (2022) and Wei et al. (2020). An enumeration method was used to remove entangled items one by one, redoing the factor analysis after each deletion until factor entanglement was eliminated, and all indicators met the research standards. Throughout the process, the number of items deleted was minimized as much as possible.
After gradually removing the entangled items, the results of the factor analysis significantly improved. Subsequently, we conducted a second round of factor extraction, and the final results were as follows: Factor 1 included six items: Q7, Q19, Q20, Q34, Q22, and Q23; Factor 2 included six items: Q16, Q1, Q29, Q24, Q8, and Q5; Factor 3 included three items: Q12, Q2, and Q27; Factor 4 included three items: Q10, Q26, and Q18; Factor 5 included three items: Q31, Q14, and Q32. The eigenvalues of these five factors were 4.152, 3.401, 2.271, 2.192, and 1.929, all greater than 1. The variance explained after rotation was 19.770, 16.197, 10.813, 10.437, and 9.188%, respectively. Furthermore, the total variance explained increased from 61.167 to 66.405%. The variance explained rate plays a critical role in evaluating the rationality and explanatory power of a model in factor analysis. The results indicate that the five factors extracted in this study account for 66.405% of the variance in the original variables, demonstrating that the model has strong explanatory power and that the extracted factors effectively summarize the data characteristics (Wang et al., 2022).
Finally, a reliability analysis and correlation test were conducted on the remaining items, with the results presented in Tables 4, 5. The analysis indicated an overall Cronbach’s α coefficient of 0.941, demonstrating high reliability. The individual reliabilities of the five factors were 0.906, 0.859, 0.767, 0.725, and 0.736, all above the 0.6 standard (Eisinga et al., 2013). Additionally, the KMO value was 0.955, greater than 0.6, and Bartlett’s test of sphericity yielded a chi-square value of 3491.395, df of 210, and p-value of 0.000, all meeting the requirements for Bartlett’s sphericity test (p < 0.05). The analysis above indicates that after removing certain items, the factor analysis results still met the standards (Nunnally, 1994).
To ensure that the five factors obtained from the factor analysis have good discriminant validity and that the items within each factor correspond well, confirmatory factor analysis (CFA) was conducted. The results are shown in Table 6. In the confirmatory factor analysis, all standardized load coefficients were greater than 0.6, meeting the criteria set by Muilenburg and Berge (2005), indicating good significance of the items. Additionally, the Average Variance Extracted (AVE) exceeded 0.36, and the Composite Reliability (CR) exceeded 0.7, demonstrating strong convergent validity, indicating good convergent validity of the model (Fornell and Larcker, 1981a; Hair et al., 2016).
The “comparison of the square root of AVE with the correlation coefficients” method was used to analyze discriminant validity. As shown in Table 7, the square root of the AVE is highlighted on the diagonal of the table. The square root of the AVE for each factor was greater than the maximum correlation coefficient between that factor and other factors, indicating good discriminant validity between the factors (Fornell and Larcker, 1981a). Additionally, the above analysis results confirm that the user experience evaluation scale developed in this study fully meets the required standards. At this point, the final scale was established, comprising five factors and 21 research items. Both the reliability and validity of the scale were deemed satisfactory. The extracted factors will now be named.
Based on the factor analysis and validation results mentioned above, it is confirmed that the five factors identified in the study fully meet the research standards. On this basis, the study attempts to name the factors according to the content of the items they encompass, to identify the specific factors influencing users’ engagement with fitness live streaming platforms.
Firstly, Factor 1 includes six items, covering aspects such as diverse course content, prevention of exercise injuries, acquisition of professional fitness knowledge, helping users stay focused, improving physical fitness, promoting health, and achieving good exercise results. These aspects highlight the role of fitness live streaming platforms. Usefulness is typically defined as a dimension that effectively enhances individual efficiency (Kim, 2021), aligning conceptually with the content of Q7, Q19, Q20, Q34, Q22, and Q23. Therefore, this study names Factor 1 as Usefulness. In this context, Usefulness refers to Generation Z users’ overall evaluation of whether a fitness live streaming platform effectively helps them achieve their fitness goals. It directly reflects the practical value the platform offers to Generation Z users, influencing their depth and frequency of engagement on the platform. Since users tend to prioritize platforms that deliver significant practical value, the level of Usefulness strongly impacts their satisfaction. This factor is significant as it encourages relevant practitioners to enhance the practical effectiveness of platform services.
Secondly, Factor 2 consists of six items, including the reward feedback mechanism of fitness live streaming platforms, personalized fitness plans, scientific dietary advice, accompanying fitness equipment, reasonable training arrangements, and supervision functions. These items demonstrate the functional content that fitness live streaming platforms provide to Generation Z users, representing the way technical features are concretely presented to users. Items Q16, Q1, Q29, Q24, Q8, and Q5 align with this concept, leading this study to name Factor 2 as Functional Quality. In this study, Functional Quality serves as a critical dimension for evaluating the service capabilities and technical performance of fitness live streaming platforms. It encompasses aspects such as functionality richness, personalization, and practicality, reflecting whether the platform can meet Generation Z users’ fitness needs through high-quality features and services. In service research, Functional Quality is often interpreted as the perceived value users experience during their interaction with a product or service (Gronroos, 1988). This value is not limited to the content of the service itself but also includes the specific methods and effectiveness in helping users achieve their goals (Bernardo et al., 2012). Products or services with high Functional Quality can enhance user trust and satisfaction, fostering greater engagement with the platform. This factor is significant as it calls on relevant practitioners to enrich the platform’s functional features.
Thirdly, Factor 3 includes three items, reflecting users’ needs for exercising together with others, communicating, and integrating online and offline activities when using fitness live streaming platforms. This reflects Generation Z users’ processes and willingness to engage in communication and interaction with others while using such platforms, aligning with the content of Q12, Q2, and Q27. Therefore, this study names Factor 3 as Social Interaction. Social Interaction generally refers to the exchange of information through various communication activities among users (Li et al., 2023), with increasingly diverse forms. In this study, Social Interaction primarily captures Generation Z users’ needs and behaviors in establishing connections, sharing experiences, and participating in fitness activities with others on fitness live streaming platforms. Its core lies in the exchange of information and the formation of emotional bonds between users (Savci et al., 2022). As a vital component of the online fitness experience, Social Interaction serves not only as a means for users to enhance interpersonal connections through the platform but also as a key factor in motivating users to persist in fitness activities and improving their satisfaction. Additionally, it transforms the platform from a mere fitness tool into a social ecosystem that connects users. This factor is significant as it encourages relevant practitioners to enhance the platform’s social and interactive features, strengthening the connections between users and between users and streamers.
Fourthly, Factor 4 includes three items, involving easy platform operation, timely course reminders, and convenient playback of recorded sessions. These reflect Generation Z users’ demands for convenience in using fitness live streaming platforms, aligning with the content of Q10, Q26, and Q18. Thus, this study names Factor 4 as Convenience. Convenience is often regarded as one of the core objectives of technological design, aiming to simplify user operations and reduce the complexity of routine tasks (Obe and Balogun, 2007). It is widely referenced in consumer behavior research (Ng-Kruelle et al., 2002; Jih, 2007) and recognized as a critical factor in the success of mobile commerce and online services (Yi et al., 2006). In this study, Convenience refers primarily to Generation Z users’ needs for simplicity, accessibility, and efficiency when using fitness live streaming platforms. As a significant dimension influencing user experience, Convenience emphasizes the platform’s performance in saving users’ time, reducing usage difficulty, and streamlining operational processes. Its importance lies in encouraging industry practitioners to enhance the level of convenience in their services.
Finally, Factor 5 includes three items, involving few advertisements popping up during live sessions, stable network connections without delays, and ensuring user privacy protection. These items reflect Generation Z users’ demands for the technical aspects of the platform. This concept aligns with Q31, Q14, and Q32, leading this study to name Factor 5 as Technical Quality. Technical Quality generally refers to the maturity and usability of the technology within a system (Lin and Bhattacherjee, 2010), including features such as high-resolution displays, superior audio and video quality, stable network connections, and technical security measures. A lack of Technical Quality can result in user dissatisfaction, diminished experience, and reduced intention to use. In this study, Technical Quality reflects Generation Z users’ expectations regarding the maturity, stability, and security of the technology underpinning fitness live streaming platforms. As a vital component of user experience, this factor emphasizes the need for industry practitioners to deliver high levels of technical support to ensure the platform’s reliability and safety. Additionally, it highlights the importance of minimizing disruptions and creating a stable, efficient fitness environment for users.
Based on the results of factor analysis, this study aims to further explore the interrelationships among various factors and their associations with user satisfaction through an extensive review of the literature. Compared to traditional television or video-on-demand, the core feature of live streaming lies in its high degree of real-time interaction (Guo et al., 2022), which emphasizes real-time engagement between streamers and viewers as well as among viewers themselves (Long and Tefertiller, 2020; He et al., 2023; Liao et al., 2023). Numerous studies have demonstrated that online fitness applications and live streaming platforms with social interaction mechanisms can significantly enhance users’ joy and promote habitual use of the platform (Cavallo et al., 2012; Chen and Pu, 2014; Stragier et al., 2016), thereby encouraging users to sustain long-term physical activity (Almeida, 2008; Beets et al., 2010; Foster et al., 2010; Cavallo et al., 2014). Additionally, researchers have noted that the primary driving force behind public participation in fitness live streaming is its social attributes (Sokolova and Perez, 2021), leading to its recognition as a novel form of social interaction (Skjuve and Brandtzaeg, 2019; Hudders et al., 2021).
At the same time, the fitness effectiveness and functional quality of fitness live streaming are also key concerns for users. The initial motivation for most users to exercise through live streaming is to promote physical activity and maintain health (Nyenhuis et al., 2020; Hammami et al., 2022; Liu et al., 2022). Research has demonstrated that the effectiveness of live streaming workouts significantly influences users’ willingness to exercise (Hsu et al., 2022; Yu and Song, 2024), and the usefulness of these platforms has been validated in numerous studies (Take, 2009; Petersen et al., 2019; Chang et al., 2021; Parker et al., 2021). Therefore, social interaction and usefulness play crucial roles in users’ evaluation of their fitness experience and benefits on online fitness platforms. Extensive research confirms that social interaction has a direct positive impact on user satisfaction (Voss and Parasuraman, 1995; Grace and O'Cass, 2004; Srivastava and Kaul, 2014), and the usefulness of online services significantly enhances user satisfaction as well (Arbaugh, 2000; Bhattacherjee, 2001; Chiu et al., 2009). Consequently, this study identifies social interaction and usefulness as core mediating variables influencing user satisfaction with fitness live streaming platforms for in-depth exploration.
In addition to social interaction and usefulness, this study also discusses hypothesized relationships among other factors. Convenience not only influences users’ perceptions of platform functionality but is also closely associated with their technology acceptance. Kim et al. (2010) demonstrated that convenience has a significantly positive effect on the perceived usefulness of mobile payments. Similarly, Jang (2015) highlighted that convenience is a critical decision factor for millennial students choosing social media and collaborative technologies for team learning and interaction. Moreover, technical quality is regarded as a vital determinant of user experience. Shah and Attiq (2016) identified technical quality as a strong predictor of perceived usefulness in e-learning contexts. Biviji et al. (2021) further noted that high-quality technical services or applications are more likely to become topics of user engagement and communication. Galdolage (2021) emphasized that improving technical quality through self-service technologies (SSTs) not only diversifies service delivery but also brings greater convenience to users. In his service quality model for business executives, Grönroos (1984) explicitly stated that acceptable technical quality is a prerequisite for achieving successful functional quality. Regarding functional quality, Wang et al. (2023) found that it directly influences users’ evaluations of the usefulness of fitness services and acts as a key facilitator of fitness game practicality. Moulay et al. (2023), in their study on community livability, stressed that meeting functional requirements is a crucial factor in promoting social interaction within communities.
Therefore, this study proposes the following hypotheses:
H1: The usefulness of fitness live streaming platforms has a positive impact on user satisfaction.
H2: Social interaction on fitness live streaming platforms has a positive impact on user satisfaction.
H3: The convenience of fitness live streaming platforms has a positive impact on usefulness.
H4: The convenience of fitness live streaming platforms has a positive impact on social interaction.
H5: The technical quality of fitness live streaming platforms has a positive impact on usefulness.
H6: The technical quality of fitness live streaming platforms has a positive impact on social interaction.
H7: The technical quality of fitness live streaming platforms has a positive impact on convenience.
H8: The technical quality of fitness live streaming platforms has a positive impact on functional quality.
H9: The functional quality of fitness live streaming platforms has a positive impact on usefulness.
H10: The functional quality of fitness live streaming platforms has a positive impact on social interaction.
The purpose of this study is to further investigate the relationships between various factors and user satisfaction. In the second round of the questionnaire, widely cited survey scales were added to assess satisfaction. After incorporating the satisfaction items, factor analysis was conducted again, where the serial numbers of the satisfaction items were S1, S2, S3. This factor analysis extracted six factors, with the variance explained by the rotated factors being 17.091, 14.606, 10.146, 9.836, 8.347, and 8.095%, respectively, and the cumulative variance explained after rotation reaching 68.121%. The eigenvalues of the factors were 4.102, 3.505, 2.435, 2.361, 2.003, and 1.943, all greater than 1. Additionally, the overall Cronbach’s α coefficient was 0.948, and the independent reliability coefficients of the six factors were 0.906, 0.859, 0.831, 0.767, 0.725, and 0.736, all exceeding the 0.6 standard (Eisinga et al., 2013). The KMO value was 0.957, greater than 0.6, and the approximate chi-square value for Bartlett’s test of sphericity was 4196.014, with a degree of freedom (df) of 276 and a p-value of 0.000, all meeting the criteria for Bartlett’s test of sphericity (p < 0.05), and conforming to the standards for factor analysis (Nunnally, 1994). The results of this analysis are shown in Tables 8, 9.
To avoid the potential issue of factor entanglement between satisfaction and the five factors summarized in the Task 1 stage, a confirmatory factor analysis (CFA) was conducted on all six factors, including satisfaction. The results are presented in Tables 10, 11. All standardized loadings exceeded 0.6 (Muilenburg and Berge, 2005), all Average Variance Extracted (AVE) values were greater than 0.36 (Fornell and Larcker, 1981a), and all Composite Reliability (CR) values were above 0.7 (Hair et al., 2016), indicating good convergent validity of the model. Furthermore, the AVE square root test confirmed that the square root of each factor’s AVE was the largest in both horizontal and vertical comparisons, demonstrating good discriminant validity among the factors (Fornell and Larcker, 1981a).
Based on the previous hypotheses, this study conducted Structural Equation Modeling (SEM) analysis using SPSSAU software, with the model fit results shown in Table 12. Structural equation models involve multiple fit indices, and following the recommendations of Schumacker and Lomax (2004) and Kline (2023), this study considered several commonly used fit indices to ensure they fell within acceptable ranges. Commonly referenced indices include the chi-square to degrees of freedom ratio (χ2/df), GFI, RMSEA, CFI, and NFI. Specifically, χ2/df should be less than 3, GFI should exceed 0.9, RMSEA should be below 0.1, and CFI and NFI should generally be greater than 0.9. The results of this study indicated the following overall model fit metrics: χ2/df = 1.725 < 3, GFI = 0.902 > 0.9, RMSEA = 0.048 < 0.10, CFI = 0.957 > 0.9, NFI = 0.904 > 0.9, and NNFI = 0.905 > 0.9, all of which meet the fit standards, indicating that the model fits well (Browne and Cudeck, 1992; Hu and Bentler, 1999).
The regression coefficients of the model are shown in Table 13. When the p-value of the path relationship is less than 0.05, it indicates a significant effect (Chen and Zhang, 2022). In the research results, except for hypotheses H5, H6, and H10, all other hypotheses had p-values less than 0.05, meaning that the paths H1, H2, H3, H4, H7, H8, and H9 exhibit significant relationships (p < 0.05), confirming that the hypotheses are supported. The final theoretical model is illustrated in Figure 2.
The confirmation of hypotheses H1 and H2 indicates that the usefulness and social interaction of fitness live streaming platforms both positively influence user satisfaction. This finding aligns with the conclusions of Hsu et al. (2022) and Sokolova and Perez (2021). However, a comparison of path coefficients reveals that the path coefficient of social interaction on satisfaction is 0.679, significantly higher than the 0.255 for usefulness. This suggests that, for Generation Z users, social interaction is the most critical factor affecting their satisfaction experience. Compared to gaining fitness knowledge and achieving exercise outcomes, Generation Z users place greater importance on meaningful communication and interaction with other users and streamers. This tendency aligns with the unique social attributes of live streaming platforms and the strong social needs of Generation Z. Multiple studies have shown that Generation Z has a close relationship with technology, spending substantial amounts of time interacting within digital environments and frequently engaging in information exchange daily (Seemiller and Grace, 2016; Dolot, 2018). Therefore, timely feedback and guidance during these exchanges can significantly enhance their sense of immersion and satisfaction. He et al. (2023) also highlighted that social media developers and managers can increase user stickiness to live streaming platforms by fostering interaction between viewers as well as between viewers and streamers, thereby enhancing users’ sense of virtual community. Additionally, extensive literature emphasizes that commenting on social platforms and maintaining information exchanges with others are core behavioral characteristics of Generation Z (Csobanka, 2016; Talmon, 2019). This highlights their tendency to view online social networking as an integral part of their lives, relying on digital platforms to sustain daily relationships. Such findings further underscore the importance of creating a robust social interaction environment for Generation Z users on fitness live streaming platforms.
In contrast, the influence of usefulness on satisfaction is relatively lower, which may be attributed to the following characteristics of Generation Z users: First, Generation Z typically views the internet as their primary source of information and knowledge, enabling them to easily access and retrieve the information they need (Singh, 2014; Fisher, 2018). As a result, they tend to utilize various resources, such as on-demand courses and online videos, for learning fitness knowledge rather than relying solely on live streaming. Second, Generation Z generally pursues efficiency and rapid goal attainment, showing a reluctance to invest excessive time and effort (Dolot, 2018). This behavioral trait conflicts to some extent with the long-term commitment required for fitness activities to promote health. Therefore, platforms must maintain their appeal by incorporating engaging features such as timely interactive feedback and reward mechanisms to sustain users’ fitness intentions, thereby enhancing their perception of the platform’s usefulness.
The confirmation of hypotheses H3, H4, and H9 suggests that the convenience and functional quality of fitness live streaming platforms are direct determinants of usefulness, while convenience also exerts a significant positive impact on social interaction. A comparison of path coefficients reveals that the path coefficient of convenience on usefulness is 0.605, significantly higher than that of functional quality. This finding suggests that, compared to enhancing functional quality, reducing usage complexity is more effective in improving Generation Z users’ evaluations of platform usefulness. Although functional quality has traditionally been regarded as a critical factor influencing platform usefulness in previous studies (Wang et al., 2023), the unique characteristics of Generation Z users make the importance of convenience more pronounced. Specifically, Generation Z tends to prioritize timely feedback and quickly assess whether a product meets their needs through experimentation (Hernandez-de-Menendez et al., 2020), rather than investing time and effort to explore complex functional features. For this demographic, platform functionalities should be presented in an intuitive and straightforward manner, avoiding complex operational processes and deeply hidden settings. Furthermore, enhancing convenience also positively impacts users’ social interactions on the platform, consistent with the findings of Hsu et al. (2022). Hsu et al. (2022) highlighted that a convenient and user-friendly system is a key factor for users choosing Facebook for social activities. Similarly, in the context of fitness live streaming platforms, user-friendly interaction designs can lower entry barriers, encouraging more users to participate in real-time interactions within the platform. This, in turn, further enhances their social experience and satisfaction.
The confirmation of hypotheses H7 and H8 indicates that the technical quality of fitness live streaming platforms has a significant positive impact on both convenience and functional quality. This conclusion aligns with prior research findings (Grönroos, 1984; Galdolage, 2021). A mature and stable technical system not only enhances the ease of use but also allows the platform’s functional attributes to be showcased more comprehensively, thereby improving users’ overall experience and evaluation of the platform. Compared to previous generations, Generation Z users are more inclined to engage with content presented in formats such as images, videos, and audio (Hernandez-de-Menendez et al., 2020). This preference imposes higher demands on technical quality, as features like real-time high-definition video streaming, seamless interactive design, and stable network support are crucial for ensuring user satisfaction. The maturity of the technology directly determines the quality of these features, which in turn influences Generation Z users’ acceptance of and loyalty to the platform.
The rejection of hypotheses H5, H6, and H10 indicates that the functional quality and technical quality of fitness live streaming platforms do not directly influence social interaction, nor does technical quality directly impact usefulness. This suggests that merely improving platform functionality and technical quality is insufficient to effectively foster communication and interaction among users. For Generation Z users, immediate access to information and efficient interaction are of paramount importance (Cilliers, 2017). Compared to complex functionalities and technical features, simplicity in operation is the key to encouraging this generation to actively participate in interactions, as it minimizes their time and effort expenditure. Furthermore, service experience is a critical aspect valued by Generation Z when using platforms (Hernandez-de-Menendez et al., 2020). During live fitness sessions, users are already in a state of physical and mental exertion. If the platform’s functional design is overly complex or its operational processes are cumbersome, it may disrupt users’ fitness experience, thereby diminishing their willingness to engage with other users or streamers.
Similarly, the lack of a direct impact of technical quality on usefulness suggests that technical improvements alone are insufficient for Generation Z users to perceive the platform as valuable. As a generation raised in an environment dominated by the internet, smartphones, and digital media, Generation Z considers network and technological functionality as baseline requirements (Dolot, 2018), rather than decisive factors for evaluating platform usefulness. However, technical quality can still influence the platform’s usefulness indirectly by enhancing convenience and functional quality. Therefore, technical quality should be regarded as a foundational element in platform development and design. Developers must prioritize the stability and reliability of the platform’s technological infrastructure to meet Generation Z users’ basic expectations for digital platforms.
This study aims to enhance the user experience of fitness live streaming platforms by developing a user experience evaluation scale and employing a structural equation model to explore the relationships among influencing factors. The findings identify the needs and characteristics of Generation Z consumers, providing theoretical support for the design and operation of such platforms. Based on the results, this paper proposes a series of operational and design strategies to serve as practical references for optimizing services and developing products. These strategies not only aim to improve user experience but also create value for businesses. To better meet the needs of Generation Z users, platform design should place greater emphasis on intuitiveness, interactivity, and immediacy, aligning with their strong focus on technological proficiency, experiential feedback, and social interaction. The specific design and management recommendations are as follows.
Social interaction has a significant positive impact on user satisfaction, with its effect surpassing that of platform usefulness. As Generation Z users have grown up in a digital age, they are accustomed to real-time interaction and feedback, seeking to establish connections and gain recognition through social platforms. Therefore, fitness live streaming platforms should prioritize social interaction as a core design element, aiming to create immersive social experiences and enhance interactivity. Specific management recommendations include optimizing the communication environment within live-streaming rooms by regularly reviewing community content and real-time comments to remove inappropriate material, thus fostering a healthy and positive atmosphere for interaction. Additionally, platforms should establish a diverse community ecosystem by grouping users based on their interests, fitness levels, or goals, enabling them to quickly connect with like-minded individuals. Introducing tiered membership communities with exclusive content and dedicated interactive services can further enhance user engagement. Simplifying interaction processes between users and streamers is also essential, with features such as real-time comments, voting mechanisms, and gamified rewards like points or virtual currency to incentivize activities such as interaction, sharing, and check-ins. These rewards could be redeemed for discounts, gifts, or exclusive services, thereby increasing user loyalty and activity levels. Furthermore, platforms should integrate online and offline activities by collaborating with gyms, fitness clubs, and health brands to organize cross-platform social and fitness events. This approach not only broadens user participation but also enhances market influence through co-branding, enabling sustainable resource utilization and generating additional revenue through joint marketing. By implementing these measures, platforms can meet Generation Z users’ social needs, strengthen user retention, and enhance their competitive edge in the market.
The platform’s usefulness also directly impacts user satisfaction, making it a priority for designers to address this need, particularly for Generation Z users, who value personal experiences and aspire to continuous self-improvement. Platforms should allocate more resources to content offerings and user progress support. First, platforms should cater to diverse user needs by providing a wide range of course options, from beginner to advanced fitness programs, and regularly updating content to maintain its appeal and challenge level. Additionally, value-added services such as customized course packages and personal training sessions can meet varied demands while boosting platform revenue. To help users stay focused during training, platforms can introduce a distraction-free mode that silences unnecessary notifications and provides clear, concise guidance, ensuring users can concentrate fully on their workouts and optimize their experience. Furthermore, real-time user data can be leveraged to offer health assessments and risk analyses, enabling users to understand their physical condition better. Platforms should incorporate customized safety tips and instructions into courses to minimize the risk of injuries, while premium membership services could include more comprehensive health evaluations and personal guidance to enhance user loyalty and perceived value. Finally, regular user feedback surveys should be conducted to gather insights into user needs and satisfaction levels. These insights can be used to continuously refine products and services, ensuring the improvement of fitness quality and fostering long-term trust with users. By implementing these measures, platforms can not only meet users’ expectations for usefulness but also enhance their overall experience, driving improvements in both user satisfaction and platform value.
Convenience not only directly influences platform usefulness but also significantly impacts social interaction, indirectly affecting user satisfaction, making it a critical design element. Generation Z users value efficiency and seamless experiences, avoiding the frustrations of complex operations. Therefore, platforms must focus on delivering convenient and intuitive user experiences, minimizing unnecessary time and effort expenditure. First, the platform’s interface design should remain simple and well-organized, with functional modules arranged logically to allow users to quickly locate desired content, reducing usability barriers and enhancing retention rates. Intelligent search and personalized recommendation systems should be core features, enabling users to efficiently find courses or activities of interest while boosting product and service sales conversion rates. Second, to accommodate Generation Z’s fast-paced and flexible lifestyles, platforms should offer diverse course scheduling options and implement timely reminders to ensure users never miss important sessions. To meet their preference for flexible learning, platforms should also provide instant playback and recording features for completed courses, allowing users to review or catch up at their convenience and maintain consistent progress. For earlier course recordings, a pay-per-access model can be introduced to meet user needs while generating additional revenue for the platform. These measures enhance convenience, streamline user experiences, and lay a solid foundation for the platform’s long-term development.
Functional quality significantly enhances platform usefulness, and Generation Z users are often driven by positive feedback and a sense of achievement. Thus, platform operators should focus on delivering high-quality functional services and designing multi-level incentive mechanisms to sustain long-term user engagement, ensuring the platform’s continued profitability. Specific measures include the following: First, the platform can offer post-training summary feedback, encouraging users to record and share their workout achievements, helping them stay motivated. A diverse achievement system can be implemented, including milestones such as consecutive check-ins, course completions, and challenge accomplishments. Users can earn virtual badges, points, or participate in leaderboard activities through these achievements. Such incentives not only boost user retention but also increase the platform’s visibility through social media sharing. Second, the platform should collaborate with experienced coaches and fitness experts to design scientifically sound training courses, ensuring that workout intensity and schedules align with users’ physical conditions and goals. Additionally, partnerships with fitness equipment brands can encourage users to purchase suitable gear (e.g., wearable devices) to enhance their workout experience. The platform can provide detailed tutorials and instructional videos on equipment usage to help users operate them correctly. Revenue-sharing agreements or co-branded campaigns with equipment manufacturers can generate additional income while enhancing the platform’s professional image. Moreover, integrating dietary advice into training courses can educate users on the relationship between nutrition and exercise, optimizing overall health outcomes. Personalized fitness plans tailored to users’ physical conditions, fitness goals, and time availability can further enhance engagement. Finally, collaborating with celebrity trainers or renowned fitness experts to offer exclusive courses, including one-on-one guidance for precise technique correction and recommendations, can enrich user experiences and amplify the platform’s appeal.
Technical quality significantly impacts convenience and functional quality, thereby enhancing platform usefulness. Developers should regard technical quality as the foundation of platform construction. Firstly, the platform should offer comprehensive privacy settings, allowing users to flexibly control the visibility and permissions of their personal information. Transparent and easy-to-understand privacy policies should be established, clearly outlining the collection, use, and protection of user data to foster trust. This approach not only reduces user attrition but also boosts the platform’s reputation, attracting more users and creating a virtuous cycle of growth. Secondly, to ensure platform stability, developers must conduct regular performance and stress tests to guarantee smooth operation during peak periods. Optimizing server and network architecture is essential to enhance live-stream fluency, minimizing technical disruptions that could negatively affect user experience. This reliability increases user dependence and loyalty to the platform. Furthermore, to mitigate user aversion to advertisements, the platform should limit intrusive ad formats and instead adopt non-disruptive alternatives, such as sidebar ads or optional ads users can choose to watch. This strategy allows for revenue generation without compromising user experience. Additionally, introducing an ad-free paid option caters to users willing to pay extra to avoid advertisements, striking a balance between ad revenue and user satisfaction. These measures collectively improve user satisfaction and platform profitability while maintaining a seamless and trustworthy user experience.
Unlike previous studies that focused solely on quantitative research, this study comprehensively and systematically explores the key factors users consider when evaluating fitness live streaming platforms. By integrating literature review, user surveys, factor analysis, and structural equation modeling, the study constructs a user experience evaluation scale and a user satisfaction model, further enriching theoretical research in the field of user experience. In the user satisfaction model, this study delves into the underlying psychological and behavioral logic of users engaging with the service, providing theoretical support for subsequent platform design and operations.
The study specifically targets Generation Z, a group that grew up in the digital age, which distinguishes it from previous studies on fitness live streaming platforms that mainly focused on older adults (Chang et al., 2021) or middle-aged users (Hsu et al., 2022) less familiar with online platforms. This research expands the understanding of user characteristics and deepens insights into the behavior of Generation Z in using fitness live streaming platforms from the perspective of user experience. The satisfaction framework developed in the study explains how platform attributes influence users’ psychology and behavior, ultimately leading to increased user satisfaction. It provides a comprehensive perspective on the potential drivers of satisfaction experiences. This study fills a research gap in the user experience of fitness live streaming platforms, particularly making new contributions to the understanding of Generation Z’s behavior and needs.
Against the backdrop of rapid advancements in digital technology and the emergence of fitness live streaming as a popular trend, this study targeted Generation Z, a cohort with distinctive characteristics, to systematically investigate the key factors influencing their use of fitness live streaming platforms. By employing a mixed-methods approach that integrates qualitative and quantitative analysis, this research developed a comprehensive user experience evaluation scale for fitness live streaming platforms. Using structural equation modeling (SEM), the relationships between influencing factors and user satisfaction were clarified, culminating in the construction of a satisfaction model for fitness live streaming platforms. The findings indicate that user satisfaction is primarily influenced directly by usefulness and social interaction, while other factors exert indirect effects on satisfaction through these two dimensions.
This study addresses gaps in prior research and proposes a series of design and operational strategies aimed at enhancing user engagement and experience on fitness live streaming platforms. These strategies are expected to improve user retention and usage rates, offering both theoretical and practical implications. The main conclusions of this research are as follows.
First, the user experience evaluation scale for fitness live streaming platforms comprises five dimensions: social interaction, usefulness, convenience, functional quality, and technical quality. These dimensions collectively form the core elements of user experience. Platforms should prioritize these aspects during design and optimization to maximize user satisfaction and meet their needs.
Second, model analysis reveals that social interaction and usefulness directly impact user satisfaction, with social interaction having a more pronounced effect. Convenience has a greater influence on usefulness compared to functional quality and is the only factor directly affecting social interaction, underscoring its critical role in user experience. Technical quality, by enhancing convenience and functional quality, exerts indirect positive effects on other factors and serves as the foundation of platform design.
Third, unlike general users, Generation Z’s core needs on fitness live streaming platforms are centered around social interaction, reflecting their tendency to maintain social connections through online networks. Although Generation Z users also value the usefulness of fitness content, their limited patience and proficiency in acquiring fitness information through other channels lead them to prioritize interaction with others while using the platform. Additionally, convenience is particularly crucial for Generation Z, surpassing the importance of platform functionalities and highlighting their high expectations for the intuitiveness and immediacy of digital products. Furthermore, the study finds that functional quality and technical quality do not significantly impact social interaction. This indicates that overly complex feature designs might disrupt Generation Z’s fitness experience and reduce their willingness to interact. Similarly, technical quality does not directly affect usefulness, further confirming that robust network technology has become a baseline expectation for Generation Z users. Their expectations for technical stability are more implicit requirements rather than explicit factors influencing satisfaction.
This study has certain limitations. Firstly, this study is a limited cross-sectional study with respondents primarily from China, lacking research on users from other countries. Future research could expand the nationality characteristics of respondents to construct a more universally applicable theoretical model. Secondly, due to the long duration of data collection in this study and the use of random sampling for the survey, it was difficult to strictly control the gender ratio and platform usage types among the respondents, which may have led to some unevenness in the sample distribution. For example, during the online user evaluation phase, the number of female respondents was 132, while male respondents numbered only 33. Furthermore, users of mainstream platforms such as Keep and Douyin were relatively more prevalent, while users of other platforms were somewhat fewer. Therefore, future studies should aim to balance participant data, ensuring a more representative sample to obtain more comprehensive data.
Additionally, this study chose to use SEM instead of PLS-SEM for analyzing the complex relationships between variables. The primary reason for this choice is that SEM is better suited for handling large sample sizes (typically requiring more than 200 samples) and focuses on overall model fit, allowing for a more precise examination of causal relationships while evaluating the model’s suitability through fit indices [129]. In contrast, PLS-SEM emphasizes the predictive ability of variables in explaining the outcome variable, focusing on variance explained (R2) and the significance of path coefficients, and is typically applied to small sample data [75]. This study collected a total of 314 valid samples from the questionnaire, aiming to explore the complex causal relationships between variables and user satisfaction, with an emphasis on overall model fit, making SEM the more suitable choice. However, PLS-SEM offers unique advantages for exploratory research in areas where theoretical development is still in its early stages. Therefore, in future studies on other features of fitness live streaming platforms, we plan to use PLS-SEM for exploratory analysis in small sample data contexts to broaden and deepen the research.
Finally, this study primarily focuses on fitness live streaming platforms that rely on real-time interaction with live hosts and mobile video streaming. However, with the advancement of digital technologies, more diverse and intelligent live streaming tools are emerging, such as AI-driven trainers, VR/AR fitness systems, and smart fitness mirrors. These tools offer different experiences in terms of functionality and interaction. Although some elements of the evaluation scale developed in this study may align with these new services, future research should further explore the distinctive user experiences of these advanced fitness tools, providing additional theoretical support for the development of the online fitness industry.
The original contributions presented in the study are included in the article/Supplementary material, further inquiries can be directed to the corresponding author/s.
Ethical review and approval was not required for the study on human participants in accordance with the local legislation and institutional requirements. Written informed consent from the participants or participants legal guardian/next of kin was not required to participate in this study in accordance with the national legislation and the institutional requirements.
MW: Conceptualization, Formal analysis, Investigation, Software, Validation, Visualization, Writing – original draft. ZW: Conceptualization, Methodology, Writing – review & editing. RD: Supervision, Writing – review & editing.
The author(s) declare that financial support was received for the research, authorship, and/or publication of this article. This research was funded by the National Social Science Foundation of China General Programme of Art ‘Research on Lifestyle Design for Active Health’ (21BG131).
Thanks to Jiangnan University for supporting this study.
The authors declare that the research was conducted in the absence of any commercial or financial relationships that could be construed as a potential conflict of interest.
All claims expressed in this article are solely those of the authors and do not necessarily represent those of their affiliated organizations, or those of the publisher, the editors and the reviewers. Any product that may be evaluated in this article, or claim that may be made by its manufacturer, is not guaranteed or endorsed by the publisher.
The Supplementary material for this article can be found online at: https://www.frontiersin.org/articles/10.3389/fcomp.2024.1499672/full#supplementary-material
Almeida, F. A. (2008). The relationship between social networks, social support, physical activity and self-rated health: An exploratory study. Ann Arbor, MI: University of Denver.
Arbaugh, J. B. (2000). Virtual classroom characteristics and student satisfaction with internet-based MBA courses. J. Manag. Educ. 24, 32–54. doi: 10.1177/105256290002400104
Bearden, W. O., and Teel, J. E. (1983). Selected determinants of consumer satisfaction and complaint reports. J. Mark. Res. 20, 21–28. doi: 10.1177/002224378302000103
Beets, M. W., Cardinal, B. J., and Alderman, B. L. (2010). Parental social support and the physical activity-related behaviors of youth: a review. Health Educ. Behav. 37, 621–644. doi: 10.1177/1090198110363884
Bentlage, E., Ammar, A., How, D., Ahmed, M., Trabelsi, K., Chtourou, H., et al. (2020). Practical recommendations for maintaining active lifestyle during the COVID-19 pandemic: a systematic literature review. Int. J. Environ. Res. Public Health 17:6265. doi: 10.3390/ijerph17176265
Bernardo, M., Marimon, F., and del Mar Alonso-Almeida, M. (2012). Functional quality and hedonic quality: a study of the dimensions of e-service quality in online travel agencies. Inf. Manag. 49, 342–347. doi: 10.1016/j.im.2012.06.005
Bhattacherjee, A. (2001). Understanding information systems continuance: An expectation-confirmation model. MIS Q. 25, 351–370. doi: 10.2307/3250921
Biviji, R., Williams, K. S., Vest, J. R., Dixon, B. E., Cullen, T., and Harle, C. A. (2021). Consumer perspectives on maternal and infant health apps: qualitative content analysis. J. Med. Internet Res. 23:e27403. doi: 10.2196/27403
Bralewska, K., Rogula-Kozłowska, W., and Bralewski, A. (2022). Indoor air quality in sports center: assessment of gaseous pollutants. Build. Environ. 208:108589. doi: 10.1016/j.buildenv.2021.108589
Browne, M. W., and Cudeck, R. (1992). Alternative ways of assessing model fit. Sociol. Methods Res. 21, 230–258. doi: 10.1177/0049124192021002005
Cavallo, D. N., Tate, D. F., Ries, A. V., Brown, J. D., DeVellis, R. F., and Ammerman, A. S. (2012). A social media–based physical activity intervention: a randomized controlled trial. Am. J. Prev. Med. 43, 527–532. doi: 10.1016/j.amepre.2012.07.019
Cavallo, D. N., Tate, D. F., Ward, D. S., DeVellis, R. F., Thayer, L. M., and Ammerman, A. S. (2014). Social support for physical activity—role of Facebook with and without structured intervention. Transl. Behav. Med. 4, 346–354. doi: 10.1007/s13142-014-0269-9
Chang, S.-H., Wang, L.-T., Chueh, T.-Y., Hsueh, M.-C., Hung, T.-M., and Wang, Y.-W. (2021). Effectiveness of facebook remote live-streaming-guided exercise for improving the functional fitness of community-dwelling older adults. Front. Med. 8:734812. doi: 10.3389/fmed.2021.734812
Chen, P., Li, F., and Harmer, P. (2019). Healthy China 2030: moving from blueprint to action with a new focus on public health. Lancet Public Health 4:e447. doi: 10.1016/S2468-2667(19)30160-4
Chen, Y., and Pu, P. (2014). HealthyTogether: exploring social incentives for mobile fitness applications. In Proceedings of the Second International Symposium of Chinese CHI (Chinese CHI ‘14). Association for Computing Machinery, New York, NY, USA, 25–34.
Chen, D., and Zhang, B. (2022). A home fitness satisfaction model for Chinese residents during the COVID-19 pandemic based on SEM analysis. Front. Public Health 10:947223. doi: 10.3389/fpubh.2022.947223
Chen, X., Zhu, Y., and Xu, X. (2024). Being there and being with them: the effects of visibility affordance of online short fitness video on users’ intention to cloud fitness. Front. Psychol. 15:1267502. doi: 10.3389/fpsyg.2024.1267502
Chiu, C. M., Chang, C. C., Cheng, H. L., and Fang, Y. H. (2009). Determinants of customer repurchase intention in online shopping. Online Inf. Rev. 33, 761–784. doi: 10.1108/14684520910985710
Chiu, W., Kim, H. S., Oh, Y. S., and Lee, Y. H. (2024). Unveiling the forces behind exercise intent in sports and fitness live streaming: a combination of symmetric and asymmetric modeling approaches. Int. J. Sports Mark. Spons. 25, 1219–1239. doi: 10.1108/IJSMS-01-2024-0015
Cigdem, H., and Ozturk, M. (2016). Factors affecting students’ behavioral intention to use LMS at a Turkish post-secondary vocational school. Int. Rev. Res. Open Distrib. Learn. 17, 276–295. doi: 10.19173/irrodl.v17i3.2253
Cilliers, E. J. (2017). The challenge of teaching generation Z. PEOPLE international journal of. Soc. Sci. 3, 188–198. doi: 10.20319/pijss.2017.31.188198
Comrey, A. L., and Lee, H. B. (2013). A first course in factor analysis. New York: Psychology Press.
Costello, A. B., and Osborne, J. (2019). Best practices in exploratory factor analysis: four recommendations for getting the most from your analysis. Pract. Assess. Res. Eval. 10:7. doi: 10.4135/9781412995627.d8
Csobanka, Z. E. (2016). The Z generation. Acta Educationis Generalis 6, 63–76. doi: 10.1515/atd-2016-0012
Damaševičius, R., Kim, J., and Dourado, V. Z. (2022). Use of smartphone applications to increase physical activity and fitness. Front. Med. SA 9. doi: 10.3389/fpubh.2021.713306
Descals, F. J. P., Aznal, A. S., and Prieto, J. G. (2010). E-Satisfacción: Una aproximación cualitativa. Acción psicológica 7, 75–85. doi: 10.5944/AP.7.1.209
Dolot, A. (2018). The characteristics of Generation Z, The characteristics of generation Z. E-mentor 74, 44–50. doi: 10.15219/em74.1351
Eisinga, R., Te Grotenhuis, M., and Pelzer, B. (2013). The reliability of a two-item scale: Pearson, Cronbach, or spearman-Brown? Int. J. Public Health 58, 637–642. doi: 10.1007/s00038-012-0416-3
Fearnbach, S. N., Flanagan, E. W., Höchsmann, C., Beyl, R. A., Altazan, A. D., Martin, C. K., et al. (2021). Factors protecting against a decline in physical activity during the COVID-19 pandemic. Med. Sci. Sports Exerc. 53, 1391–1399. doi: 10.1249/MSS.0000000000002602
Fischer, D., Böhme, T., and Geiger, S. M. (2017). Measuring young consumers’ sustainable consumption behavior: development and validation of the YCSCB scale. Young Consum. 18, 312–326. doi: 10.1108/YC-03-2017-00671
Fisher, G. (2018). Engaging generation Z: A study on Facebook group implementation in language courses and in multiple contexts. Theses and Dissertations University of New England.
Fornell, C., and Larcker, D. F. (1981a). Evaluating structural equation models with unobservable variables and measurement error. J. Mark. Res. 18, 39–50. doi: 10.1177/002224378101800104
Fornell, C., and Larcker, D. F. (1981b). Structural equation models with unobservable variables and measurement error: Algebra and statistics, vol. 18. Los Angeles, CA: Sage publications Sage CA, 382.
Foster, D., Linehan, C., Kirman, B., Lawson, S., and James, G. (2010). Motivating physical activity at work: using persuasive social media for competitive step counting. In Proceedings of the 14th international academic mindtrek conference: envisioning future media environments (MindTrek ‘10). Association for Computing Machinery, New York, NY, USA, 111–116.
Gagnon, M.-A., Batcho, C. S., Bird, M.-L., Labbé, B., and Best, K. L. (2023). Feasibility of a remotely supervised home-based group eHealth fitness and mobility exercise program for stroke: French-Canadian version preliminary study. Top. Stroke Rehabil. 30, 169–179. doi: 10.1080/10749357.2021.2012008
Galdolage, B. S. (2021). Interplay between performance and convenience in customer choice of self-service technologies. Curr. J. Appl. Sci. Technol. 40, 80–91. doi: 10.9734/cjast/2021/v40i1031361
Gao, Z., Lee, J. E., McDonough, D. J., and Albers, C. (2020). Virtual reality exercise as a coping strategy for health and wellness promotion in older adults during the COVID-19 pandemic. MDPI 9:1986. doi: 10.3390/jcm9061986
Giertz, J. N., Weiger, W. H., Törhönen, M., and Hamari, J. (2022). Content versus community focus in live streaming services: How to drive engagement in synchronous social media. J. Serv. Manag. 33, 33–58. doi: 10.1108/JOSM-12-2020-0439
Gilmour, J., Machin, T., Brownlow, C., and Jeffries, C. (2020). Facebook-based social support and health: a systematic review. Psychol. Popular Media 9, 328–346. doi: 10.1037/ppm0000246
Glynn, L. G., Hayes, P. S., Casey, M., Glynn, F., Alvarez-Iglesias, A., Newell, J., et al. (2014). Effectiveness of a smartphone application to promote physical activity in primary care: the SMART MOVE randomised controlled trial. Br. J. Gen. Pract. 64, e384–e391. doi: 10.3399/bjgp14X680461
Gottschall, J. S., and Hastings, B. (2023). A comparison of physiological intensity and psychological perceptions during three different group exercise formats. Front. Sports Active Living 5:1138605. doi: 10.3389/fspor.2023.1138605
Grace, D., and O'Cass, A. (2004). Examining service experiences and post-consumption evaluations. J. Serv. Mark. 18, 450–461. doi: 10.1108/08876040410557230
Graham-Rowe, E., Gardner, B., Abraham, C., Skippon, S., Dittmar, H., Hutchins, R., et al. (2012). Mainstream consumers driving plug-in battery-electric and plug-in hybrid electric cars: a qualitative analysis of responses and evaluations. Transp. Res. A Policy Pract. 46, 140–153. doi: 10.1016/j.tra.2011.09.008
Grönroos, C. (1984). A service quality model and its marketing implications. Eur. J. Mark. 18, 36–44. doi: 10.1108/EUM0000000004784
Guo, J., and Fussell, S. R. (2022). " It's great to exercise together on zoom!": understanding the practices and challenges of live stream group fitness classes. Proceed. ACM Human-Comp. Interact. 6, 1–28. doi: 10.1145/3512918
Guo, Y., Zhang, K., and Wang, C. (2022). Way to success: understanding top streamer's popularity and influence from the perspective of source characteristics. J. Retail. Consum. Serv. 64:102786. doi: 10.1016/j.jretconser.2021.102786
Hair, J. F., Black, B., Babin, B. J., and Anderson, R. (2011). Multivariate Data Analysis : Multivariate Data Analysis.
Hair, J. F. Jr., Hult, G. T. M., Ringle, C., and Sarstedt, M. (2016). A primer on partial least squares structural equation modeling (PLS-SEM) sage publications. CA, USA: Thousand Oaks.
Hair, J. F. Jr., Hult, G. T. M., Ringle, C. M., Sarstedt, M., Danks, N. P., and Ray, S. (2021). Partial least squares structural equation modeling (PLS-SEM) using R: A workbook. Springer Nature.
Hammami, A., Harrabi, B., Mohr, M., and Krustrup, P. (2022). Physical activity and coronavirus disease 2019 (COVID-19): specific recommendations for home-based physical training. Manag. Sport Leis. 27, 26–31. doi: 10.1080/23750472.2020.1757494
Harris, M. T., and Metzler, M. (2019). Online personal fitness course alignment with national guidelines for online physical education. J. Teach. Phys. Educ. 38, 174–186. doi: 10.1123/jtpe.2018-0169
He, D., Yao, Z., Tang, P., and Ma, Y. (2023). Impacts of different interactions on viewers’ sense of virtual community: an empirical study of live streaming platform. Behav. Inform. Technol. 42, 940–960. doi: 10.1080/0144929X.2022.2053884
Hernandez-de-Menendez, M., Escobar Díaz, C. A., and Morales-Menendez, R. (2020). Educational experiences with generation Z. Int. J. Interact. Des. Manuf. (IJIDeM) 14, 847–859. doi: 10.1007/s12008-020-00674-9
Hong, J., Kim, J., Kim, S. W., and Kong, H.-J. (2017). Effects of home-based tele-exercise on sarcopenia among community-dwelling elderly adults: body composition and functional fitness. Exp. Gerontol. 87, 33–39. doi: 10.1016/j.exger.2016.11.002
Hong, J., Kong, H.-J., and Yoon, H.-J. (2018). Web-based telepresence exercise program for community-dwelling elderly women with a high risk of falling: randomized controlled trial. JMIR Mhealth Uhealth 6:e9563. doi: 10.2196/mhealth.9563
Hsu, C.-Y., Wang, M.-Y., Lee, T.-I., Chiang, H.-H., and Chien, C.-Y. (2022). Exploring the behavior of online fitness users by using the unified theory of acceptance and use of technology. SPORT TK-Revista EuroAmericana de Ciencias del Deporte 11:14. doi: 10.6018/sportk.509231
Hu, L. T., and Bentler, P. M. (1999). Cutoff criteria for fit indexes in covariance structure analysis: conventional criteria versus new alternatives. Struct. Equ. Model. Multidiscip. J. 6, 1–55. doi: 10.1080/10705519909540118
Hudders, L., De Jans, S., and De Veirman, M. (2021). The commercialization of social media stars: a literature review and conceptual framework on the strategic use of social media influencers. Soci. Media Infl. Strat. Commun., 24–67. doi: 10.4324/9781003181286-3
Jang, Y. (2015). Convenience matters: a qualitative study on the impact of use of social media and collaboration technologies on learning experience and performance in higher education. Educ. Inf. 31, 73–98. doi: 10.3233/EFI-150948
Jih, W.-J. (2007). Effects of consumer-perceived convenience on shopping intention in mobile commerce: an empirical study. Int. J. E-Business Res. 3, 33–48. doi: 10.4018/jebr.2007100102
Jong, S. T., and Drummond, M. J. (2020). “Exploring online fitness culture and young females” in Re-thinking leisure in a digital age (London: Routledge), 50–62.
Kaiser, H. F. (1958). The varimax criterion for analytic rotation in factor analysis. Psychometrika 23, 187–200. doi: 10.1007/BF02289233
Kenis-Coskun, O., Imamoglu, S., Karamancioglu, B., Kurt, K., Ozturk, G., and Karadag-Saygi, E. (2022). Comparison of telerehabilitation versus home-based video exercise in patients with Duchenne muscular dystrophy: a single-blind randomized study. Acta Neurol. Belg. 122, 1269–1280. doi: 10.1007/s13760-022-01975-4
Kim, M. (2021). Conceptualization of e-servicescapes in the fitness applications and wearable devices context: multi-dimensions, consumer satisfaction, and behavioral intention. J. Retail. Consum. Serv. 61:102562. doi: 10.1016/j.jretconser.2021.102562
Kim, C., Mirusmonov, M., and Lee, I. (2010). An empirical examination of factors influencing the intention to use mobile payment. Comput. Hum. Behav. 26, 310–322. doi: 10.1016/j.chb.2009.10.013
Kline, R. B. (2023). Principles and practice of structural equation modeling. New York: Guilford Publications.
Kymäläinen, T., Seisto, A., and Malila, R. (2021). Generation Z food waste, diet and consumption habits: a Finnish social design study with future consumers. Sustain. For. 13:2124. doi: 10.3390/su13042124
Lamash, L., and Josman, N. (2020). Full-information factor analysis of the daily routine and autonomy (DRA) questionnaire among adolescents with autism spectrum disorder. J. Adolesc. 79, 221–231. doi: 10.1016/j.adolescence.2020.01.011
Lee, M.-C. (2010). Explaining and predicting users’ continuance intention toward e-learning: An extension of the expectation–confirmation model. Comput. Educ. 54, 506–516. doi: 10.1016/j.compedu.2009.09.002
Lee, T., and Jun, J. (2007). Contextual perceived value? Investigating the role of contextual marketing for customer relationship management in a mobile commerce context. Bus. Process. Manag. J. 13, 798–814. doi: 10.1108/14637150710834569
Lee, M. Y., Kang, J. H., and Jeon, I. K. (2013). A study on the effect of smart phone fitness application (app)‘s service quality on satisfaction after use, trust, immersion and willingness to continue to use. Kor. J. Phys. Educ. 52, 379–396.
Li, R., Lu, Y., Ma, J., and Wang, W. (2021). Examining gifting behavior on live streaming platforms: An identity-based motivation model. Inf. Manag. 58:103406. doi: 10.1016/j.im.2020.103406
Li, C., Wu, F., and Sun, Q. (2023). Research on information spreading effects in the social interaction context. J. Manag. Eng. 37, 22–34.
Liao, J., Chen, K., Qi, J., Li, J., and Yu, I. Y. (2023). Creating immersive and parasocial live shopping experience for viewers: the role of streamers' interactional communication style. J. Res. Interact. Mark. 17, 140–155. doi: 10.1108/JRIM-04-2021-0114
Lienher, S. (2022). Understanding the customers’ needs of Generation Y & Z in the fitness industry. Bachelor thesis, Geneva School of Management.
Lin, C. P., and Bhattacherjee, A. (2010). Extending technology usage models to interactive hedonic technologies: a theoretical model and empirical test. Inf. Syst. J. 20, 163–181. doi: 10.1111/j.1365-2575.2007.00265.x
Liu, R., Menhas, R., Dai, J., Saqib, Z. A., and Peng, X. (2022). Fitness apps, live streaming workout classes, and virtual reality fitness for physical activity during the COVID-19 lockdown: an empirical study. Front. Public Health 10:852311. doi: 10.3389/fpubh.2022.852311
Locke, E. (1973). What is job satisfaction? Organizational behavior and human performance. Mot. Work Organiz.
Long, Q., and Tefertiller, A. C. (2020). China’s new mania for live streaming: gender differences in motives and uses of social live streaming services. Int. J. Human–Comp. Interact. 36, 1314–1324. doi: 10.1080/10447318.2020.1746060
Lu, F., Huang, X., and Wang, X. (2022). Willingness to pay for mobile health live streaming during the covid-19 pandemic: integrating tpb with compatibility. Sustain. For. 14:15932. doi: 10.3390/su142315932
Menhas, R., Dai, J., Ashraf, M. A., M, S., Khurshid, S., Mahmood, S., et al. (2021). Physical inactivity, non-communicable diseases and national fitness plan of China for physical activity. RMHP 14, 2319–2331. doi: 10.2147/RMHP.S258660
Menhas, R., Qin, L., Saqib, Z. A., and Younas, M. (2023). The association between COVID-19 preventive strategies, virtual reality exercise, use of fitness apps, physical, and psychological health: testing a structural equation moderation model. Front. Public Health 11:1170645. doi: 10.3389/fpubh.2023.1170645
Mouakket, S., and Bettayeb, A. M. (2015). Investigating the factors influencing continuance usage intention of learning management systems by university instructors: the blackboard system case. Int. J. Web Inform. Syst. 11, 491–509. doi: 10.1108/IJWIS-03-2015-0008
Moulay, A., Ujang, N., Maulan, S., Abu Bakar, N. A., and Ahmad, N. (2023). Linking park utilisation and place attachment: towards liveable neighbourhoods. Proceed. Inst. Civil Eng. Urban Design Plan. 177, 21–31. doi: 10.1680/jurdp.22.00043
Muilenburg, L. Y., and Berge, Z. L. (2005). Student barriers to online learning: a factor analytic study. Distance Educ. 26, 29–48. doi: 10.1080/01587910500081269
Mutz, M., Müller, J., and Reimers, A. K. (2021). Use of digital media for home-based sports activities during the COVID-19 pandemic: results from the German SPOVID survey. Int. J. Environ. Res. Public Health 18:4409. doi: 10.3390/ijerph18094409
Nascimento, B., Oliveira, T., and Tam, C. (2018). Wearable technology: what explains continuance intention in smartwatches? J. Retail. Consum. Serv. 43, 157–169. doi: 10.1016/j.jretconser.2018.03.017
Ng-Kruelle, G., Swatman, P. A., Rebne, D. S., and Hampe, J. F. (2002). The price of convenience: privacy and mobile commerce. Q. J. Electron. Commer. 3, 273–286.
Nieman, D. C., and Wentz, L. M. (2019). The compelling link between physical activity and the body's defense system. J. Sport Health Sci. 8, 201–217. doi: 10.1016/j.jshs.2018.09.009
Nyenhuis, S. M., Greiwe, J., Zeiger, J. S., Nanda, A., and Cooke, A. (2020). Exercise and fitness in the age of social distancing during the COVID-19 pandemic. The journal of allergy and clinical immunology. In Pract. 8, 2152–2155. doi: 10.1016/j.jaip.2020.04.039
Obe, O., and Balogun, V. (2007). Practice, trends and challenges of mobile commerce in Nigeria. Inf. Technol. J. 6, 448–456. doi: 10.3923/itj.2007.448.456
Oliver, R. L. (1980). A cognitive model of the antecedents and consequences of satisfaction decisions. J. Mark. Res. 17, 460–469. doi: 10.1177/002224378001700405
Oliver, R. L. (1993). Cognitive, affective, and attribute bases of the satisfaction response. J. Consum. Res. 20, 418–430. doi: 10.1086/209358
Parker, K., Uddin, R., Ridgers, N. D., Brown, H., Veitch, J., Salmon, J., et al. (2021). The use of digital platforms for adults’ and adolescents’ physical activity during the COVID-19 pandemic (our life at home): survey study. J. Med. Internet Res. 23:e23389. doi: 10.2196/23389
Petersen, J. M., Prichard, I., and Kemps, E. (2019). A comparison of physical activity mobile apps with and without existing web-based social networking platforms: systematic review. J. Med. Internet Res. 21:e12687. doi: 10.2196/12687
Riley, W. T., Rivera, D. E., Atienza, A. A., Nilsen, W., Allison, S. M., and Mermelstein, R. (2011). Health behavior models in the age of mobile interventions: are our theories up to the task? Transl. Behav. Med. 1, 53–71. doi: 10.1007/s13142-011-0021-7
Savci, C., Akinci, A. C., and Keles, F. (2022). The association of perceived sociability and social intelligence with loneliness in online learning among nursing students. Nurse Educ. Today 109:105226. doi: 10.1016/j.nedt.2021.105226
Schmidt, S. C., Anedda, B., Burchartz, A., Eichsteller, A., Kolb, S., Nigg, C., et al. (2020). Physical activity and screen time of children and adolescents before and during the COVID-19 lockdown in Germany: a natural experiment. Sci. Rep. 10:21780. doi: 10.1038/s41598-020-78438-4
Schumacker, R.E., and Lomax, R.G. (2004). A beginner's guide to structural equation modeling. Psychology Press.
Seemiller, C., and Grace, M. (2016). Generation Z Goes to college. San Francisco: John Wiley & Sons.
Shah, H. J., and Attiq, S. (2016). Impact of technology quality, perceived ease of use and perceived usefulness in the formation of consumer’s satisfaction in the context of e-learning. Abasyn J. Soc. Sci. 9, 124–140.
Silva-Jose, C., Nagpal, T. S., Coterón, J., Barakat, R., and Mottola, M. F. (2022). The ‘new normal’includes online prenatal exercise: exploring pregnant women’s experiences during the pandemic and the role of virtual group fitness on maternal mental health. BMC Pregnancy Childbirth 22:251. doi: 10.1186/s12884-022-04587-1
Singh, A. (2014). Challenges and issues of generation Z. IOSR J. Bus. Manag. 16, 59–63. doi: 10.9790/487X-16715963
Skjuve, M., and Brandtzaeg, P. B. (2019). Facebook live: a mixed-methods approach to explore individual live streaming practices and motivations on Facebook. Interact. Comput. 31, 589–602. doi: 10.1093/iwc/iwz038
Slaton, J. D., Hanley, G. P., and Raftery, K. J. (2017). Interview-informed functional analyses: a comparison of synthesized and isolated components. J. Appl. Behav. Anal. 50, 252–277. doi: 10.1002/jaba.384
Sokolova, K., and Perez, C. (2021). You follow fitness influencers on YouTube. But do you actually exercise? How parasocial relationships, and watching fitness influencers, relate to intentions to exercise. J. Retail. Consum. Serv. 58:102276. doi: 10.1016/j.jretconser.2020.102276
Srivastava, M., and Kaul, D. (2014). Social interaction, convenience and customer satisfaction: the mediating effect of customer experience. J. Retail. Consum. Serv. 21, 1028–1037. doi: 10.1016/j.jretconser.2014.04.007
Stanes, E., Klocker, N., and Gibson, C. (2015). Young adult households and domestic sustainabilities. Geoforum 65, 46–58. doi: 10.1016/j.geoforum.2015.07.007
Stragier, J., Abeele, M. V., Mechant, P., and De Marez, L. (2016). Understanding persistence in the use of online fitness communities: comparing novice and experienced users. Comput. Hum. Behav. 64, 34–42. doi: 10.1016/j.chb.2016.06.013
Szymanski, D. M., and Henard, D. H. (2001). Customer satisfaction: a meta-analysis of the empirical evidence. J. Acad. Mark. Sci. 29, 16–35. doi: 10.1177/0092070301291002
Take, S. W. (2009). Learning, teaching, and scholarship in a digital age. Educ. Res. 38, 246–259. doi: 10.3102/0013189X09336671
Talmon, G. A. (2019). Generation Z: What’s next? Med. Sci. Educ. 29, 9–11. doi: 10.1007/s40670-019-00796-0
Tian, Y., Jiang, C., Wang, M., Cai, R., Zhang, Y., He, Z., et al. (2016). BMI, leisure-time physical activity, and physical fitness in adults in China: results from a series of national surveys, 2000–14. Lancet Diab. Endocrinol. 4, 487–497. doi: 10.1016/S2213-8587(16)00081-4
Tian, R., Yin, R., and Gan, F. (2022). Exploring public attitudes toward live-streaming fitness in China: a sentiment and content analysis of China's social media Weibo. Front. Public Health 10:1027694. doi: 10.3389/fpubh.2022.1027694
Trent, M. (2016). Investigating virtual personal fitness course alignment with national guidelines for online physical education. Dissertation, Georgia State University.
Voss, G., and Parasuraman, A. (1995). Prepurchase preference and post purchase. Massachusetts Ave, Cambridge: Satisfaction in Service Exchange. Marketing Science Institute, 95–113.
Wang, Z., Deng, R., and Jiang, Q.-L. (2023). Exploration and study of the factors influencing users’ adoption of games for fitness behavior. Int. J. Human Comp. Interact. 39, 3461–3472. doi: 10.1080/10447318.2022.2097985
Wang, Z., Jiang, Q., and Li, Z. (2022). How to promote online education through educational software—an analytical study of factor analysis and structural equation modeling with Chinese users as an example. Systems 10:100. doi: 10.3390/systems10040100
Wang, Z., Wang, Z., and Deng, R. (2024). Assessment scale and behavioral model construction for AI chat information retrieval and processing service systems based on behavioral reasoning theory—taking ChatGPT-like tools as an example. Int. J. Human Comp. Interact., 1–22. doi: 10.1080/10447318.2024.2331855
Wei, X. (2023). “Generation Z consumers and new trends in the Chinese sports industry” in Routledge handbook of Sport in China (Routledge), 364–370.
Wei, W., Cao, M., Jiang, Q., Ou, S.-J., and Zou, H. (2020). What influences Chinese consumers’ adoption of battery electric vehicles? A preliminary study based on factor analysis. Energies 13:1057. doi: 10.3390/en13051057
Yang, Y., and Koenigstorfer, J. (2020). Determinants of physical activity maintenance during the Covid-19 pandemic: a focus on fitness apps. Transl. Behav. Med. 10, 835–842. doi: 10.1093/tbm/ibaa086
Yi, M. Y., Fiedler, K. D., and Park, J. S. (2006). Understanding the role of individual innovativeness in the acceptance of IT-based innovations: comparative analyses of models and measures. Decis. Sci. 37, 393–426. doi: 10.1111/j.1540-5414.2006.00132.x
Yu, J., and Song, W. (2024). Impact of fitness live streaming on public engagement in physical activities: a cross-sectional study. J. Physical Educ. Sport 24, 658–672. doi: 10.7752/jpes.2024.03078
Zhu, J., Liang, Z., Zhang, C., and Wei, X. (2023). How are sports management, renewable energy, and green finance related? A survey evidence. Renew. Energy 206, 39–46. doi: 10.1016/j.renene.2023.02.040
Keywords: fitness live streaming platforms, generation Z, satisfaction, factor analysis, structural equation model
Citation: Wang M, Wang Z and Deng R (2025) How to enhance generation Z users’ satisfaction experience with online fitness: a case study of fitness live streaming platforms. Front. Comput. Sci. 6:1499672. doi: 10.3389/fcomp.2024.1499672
Received: 27 September 2024; Accepted: 16 December 2024;
Published: 07 January 2025.
Edited by:
Andrej Košir, University of Ljubljana, SloveniaReviewed by:
Gergely Ráthonyi, University of Debrecen, HungaryCopyright © 2025 Wang, Wang and Deng. This is an open-access article distributed under the terms of the Creative Commons Attribution License (CC BY). The use, distribution or reproduction in other forums is permitted, provided the original author(s) and the copyright owner(s) are credited and that the original publication in this journal is cited, in accordance with accepted academic practice. No use, distribution or reproduction is permitted which does not comply with these terms.
*Correspondence: Zheng Wang, NzIzMDMwNjAyMUBzdHUuamlhbmduYW4uZWR1LmNu; Rong Deng, ZHI1MzBAamlhbmduYW4uZWR1LmNu
Disclaimer: All claims expressed in this article are solely those of the authors and do not necessarily represent those of their affiliated organizations, or those of the publisher, the editors and the reviewers. Any product that may be evaluated in this article or claim that may be made by its manufacturer is not guaranteed or endorsed by the publisher.
Research integrity at Frontiers
Learn more about the work of our research integrity team to safeguard the quality of each article we publish.