- 1Directorate F, European Commission, Joint Research Centre (JRC), Ispra, Italy
- 2Department of Endocrinology, Diabetes and Metabolic Diseases, University Medical Centre Ljubljana, Ljubljana, Slovenia
- 3Faculty of Medicine, University of Ljubljana, Ljubljana, Slovenia
Indicators are quantitative or qualitative measures used to gauge various aspects of society and assess change over time (such as monitoring the progress or effectiveness of a public policy). Ideally, indicators should be precisely defined and measured according to harmonized procedures that may not be feasible in practice, especially in domains such as health, where indicators are often derived from preexisting, heterogeneous datasets. Integrating such data has posed a persistent challenge, but semantic technologies offer advantages by enriching data in a relatively simple, linkable, and non-disruptive way. However, without harmonized frameworks, the difficulties associated with data integration are unlikely to be resolved. In this article, we propose a generic, domain-neutral indicator contextualization framework for structuring and linking distributed datasets with contextual metadata according to a standard model. The framework integrates the concepts of the International Organization for Standardization/International Electrotechnical Commission (ISO/IEC) 11179 metadata registry standard with the common core ontologies (CCO) mid-level ontology suite, and incorporates other semantic technologies to make it adaptable and interoperable within and across domains. Application of the framework to an example indicator illustrates the versatility and adaptability of the approach in a federated data architecture. The contextual information can be dereferenced using standard query tools to provide data users a comprehensive understanding and overview of the indicator. The framework is amenable to deep learning applications via the principles of semantic data models, linked open data, and knowledge organization systems. The ideas are presented to stimulate further reflection and consolidation of standard data contextualization frameworks.
1 Introduction
Indicators are ubiquitous in processes related to most domains. They are used for various purposes to gauge the process status or assess changes over time. For example, they are helpful in analyzing the progress or effectiveness of a public policy, and key performance indicators (KPIs) are useful in business domains for evaluating business performance to improve outcomes (Houston, 2021). Monitoring and evaluation (M&E) processes are common to all domains, requiring robust sets of indicators to improve the design of projects and programs and to understand the effectiveness of any interventions. In the context of scaled-up funding for climate-change adaptation, M&E frameworks combine qualitative and quantitative indicators to guarantee value for money (Lamhauge et al., 2013). In the field of healthcare, indicators monitor health status and health determinants of populations
to help identify existing or emerging health problems. Reliable comparison of health indicators over time and between regions/countries requires standard indicator definitions, similar data sources, and standardized data collection methods (Tolonen et al., 2021). All these pose a particular challenge in the heterogeneous health–data landscape of Europe. Whereas, the importance of indicators is widely acknowledged, there is no denying the difficulty of agreeing on them—nor the other difficulties of measuring them effectively—and perhaps more importantly, understanding them (Terzi et al., 2021; OECD, 2014; Lyytimäki et al., 2020).
The relevance of data integration and interoperability in the use of indicators has prompted an attempt to classify data sources and indicators according to the types of use (Kosten, 2016). The drawback is that all classification systems are somewhat arbitrary since the rules for making the classification can be formulated in many different ways (Moravcsik, 1988).
A further complication lies in the multidimensionality of the constituent elements feeding into the classification requiring every dimension to be treated separately. A composite analysis cannot be constructed without making value judgments about the relative weights of the different dimensions. Some significant failures of any classification may include omission of critical dimensions, contraction of separate dimensions into one, failure to specify classification rules in all dimensions, semantically imprecise wording, insufficient number of discrete categories in a given dimension, and an inadequate specification of the category boundaries themselves (Moravcsik, 1988).
Similar concerns have been elaborated by others in the field of healthcare quality, especially in regard to transparency, equity of application of individual measures, introduction of bias from missing measures and adequate adjustment of component measures, standardized banding onto consistent scales, choice of weights and any sensitivity analysis of the selection of weights, and presentation of uncertainties in the final composite rating (Barclay et al., 2018). The consequential impact of such concerns has been addressed in a critique on using quantified indicators in the UN's sustainable development goals (SDGs) based on a collection of highly contested concepts (Mair et al., 2018). Drawing on further critiques of indicators from the sociological, anthropological, and sustainability literature, the authors show that the reductive nature of indicators can create problems as they try to simplify and codify complex and subjective issues. Highlighting the case of poverty, where it has been demonstrated that the SDG indicators only represent a limited understanding of the term, the authors argue that any given indicator set should be understood as a necessarily incomplete and value-laden view of a concept. Furthermore, they claim that indicators often arbitrarily strip away relevant information because the latter is difficult to codify formally.
Any endeavor, therefore, to summarize complex phenomena into single numbers will necessitate theoretical and methodological assumptions requiring careful assessment to avoid producing results of dubious analytic rigor (Terzi et al., 2021). When researchers fail to define key concepts and choose indicators that are aligned with them, they may end up with “slippery indicators” that do not measure what they claim to measure (Fischer-Mackey and Fox, 2022). In particular, constructing a composite indicator can be seen as an obstacle course, from the availability of data to the choice of the individual indicators to their treatment to compare and aggregate them (Terzi et al., 2021). According to the good indicators guide (British National Health Service, 2024), a poorly designed or poorly chosen indicator with reliable data or a well-designed indicator with unreliable and/or untimely data has very little value and is sometimes positively dangerous.
Relevant contextual information is critical to understanding the limitations of indicators in any specific application. However, indicators for comparative purposes are often presented as numbers with little (or at least not easily accessible) information about how those numbers were derived. Examples in the health domain include European Core Health Indicators (ECHI; European Commission, 2013), cancer incidence in five continents (International Agency for Research on Cancer, 2024), Organization for Economic Co-operation and Development (OECD) health indicators (OECD, 2023), European cancer incidence and mortality indicators (European Commission, 2022), European cancer inequality indicators (European Commission, 2020), and global health indicators (Global Health Data, 2024). Notwithstanding the underlying processes involved in ensuring comparability of the indicators, without knowing how an indicator was derived, it is not easy to use it with any degree of confidence. Health service infrastructures vary widely across national/regional boundaries (Bogaert et al., 2018; Soldi, 2017) and have a critical impact on how data are collected and the type of data sources available (Tolonen et al., 2021). Such variability leads to assumptions that ought to be factored into any eventual indicator cross-comparisons and can be viewed as a general issue not solely restricted to the field of healthcare.
Acknowledging these challenges, several references (British National Health Service, 2024; Bowen and Kreindler, 2018; van den Berg et al., 2019; UNAIDS, 2010) emphasize the need to make contextual information accessible when comparing indicators but stop short of providing the practical mechanisms for realizing it. Where ground-breaking mechanisms have been proposed, they are generally addressed to the needs of targeted domains (del Mar Roldán-García et al., 2021; Fox, 2015), for which the design concepts are quite specific and impose individual custom models (Espinoza-Arias et al., 2019).
We were unable to find any existing solutions providing the means to contextualize existing data/indicator sets in a harmonized way, especially ones furnishing end users with a sufficiently comprehensive overview of the data, allowing them to make informed judgments on the soundness of comparing indicators derived from heterogeneous data sources, even if only in a qualitative way. In this article, the architectural concept of a generic metadata framework is proposed as a means of supporting this broader contextualization need.
2 Materials and methods
To provide the required degree of flexibility and scalability, the generic metadata framework has to draw from several standards, methodologies, and resources, a brief overview of which is provided in Table 1.
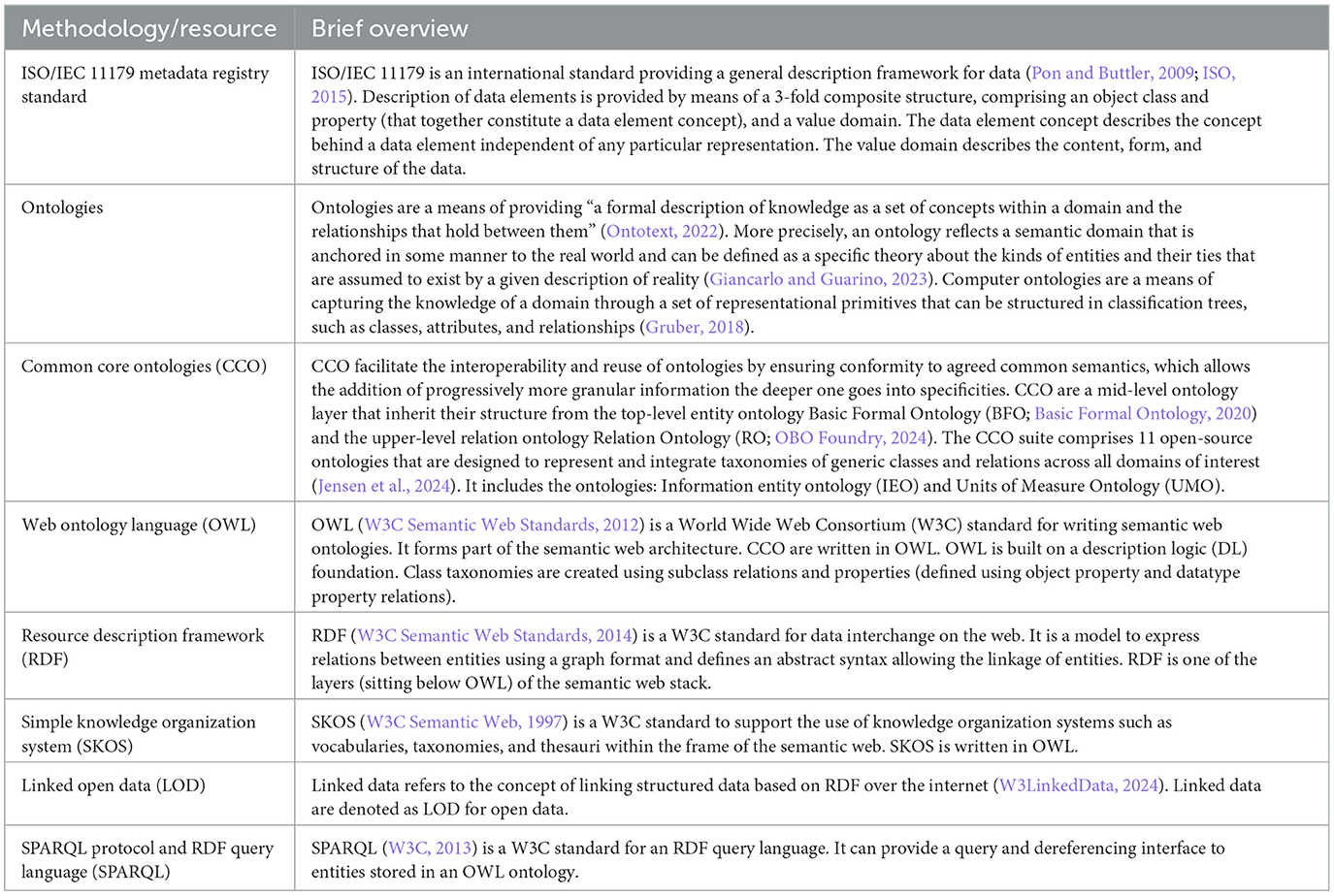
Table 1. Overview of the methodologies and resources applied to the generic indicator metadata framework.
2.1 Framework-building process
The first step in the framework-building process requires integrating the concepts of the International Organization for Standardization/International Electrotechnical Commission (ISO/IEC) 11179 with CCO. Employing a mid-level ontology suite such as CCO promotes interoperability between ontologies in the context of a distributed indicator framework. Also, it allows reuse of a foundational set of semantic relations without having to reinvent them each time.
Notwithstanding the advantages of interweaving the concepts of ISO/IEC 11179 with those of CCO, the process is not seamless. Although both methodologies share some similar principles, they deal with those principles in slightly different ways. From the point of view of generic scalability, in the relatively few instances where the differences could not be resolved, the structure of CCO was retained at the expense of relaxing the constraints of ISO/IEC 11179. Nevertheless, by mapping ISO/IEC 11179 to CCO rather than the other way around, some further functionality is provided to the metadata registry concept through the integrative design approach of CCO.
Figures 1, 2 show how ISO/IEC 11179 concepts can sit within CCO. The classes/relations of ISO/IEC 11179 and CCO are distinguished via prefixes in their names. The arrowed lines with an isA relationship depict subclasses and those with other named relationships model web ontology language (OWL) object properties. In CCO, some object properties are defined for instances of classes only (referred to by OWL as “individuals”), and these are identified in the figures with bold font.
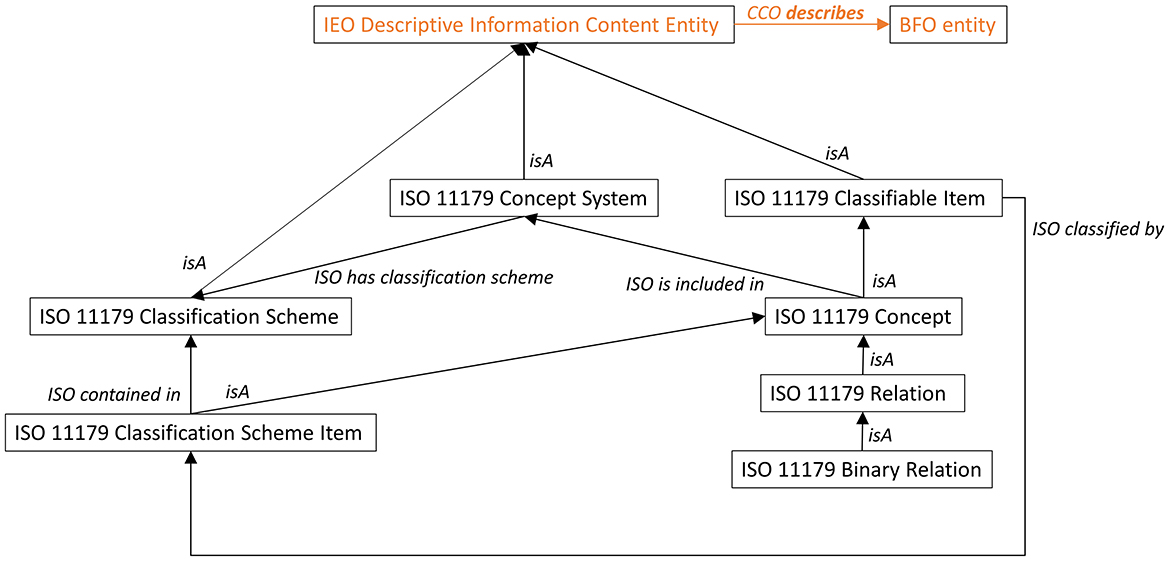
Figure 1. Classes and class relations involved in the International Organization for Standardization/International Electrotechnical Commission (ISO/IEC) 11179 classification concept.
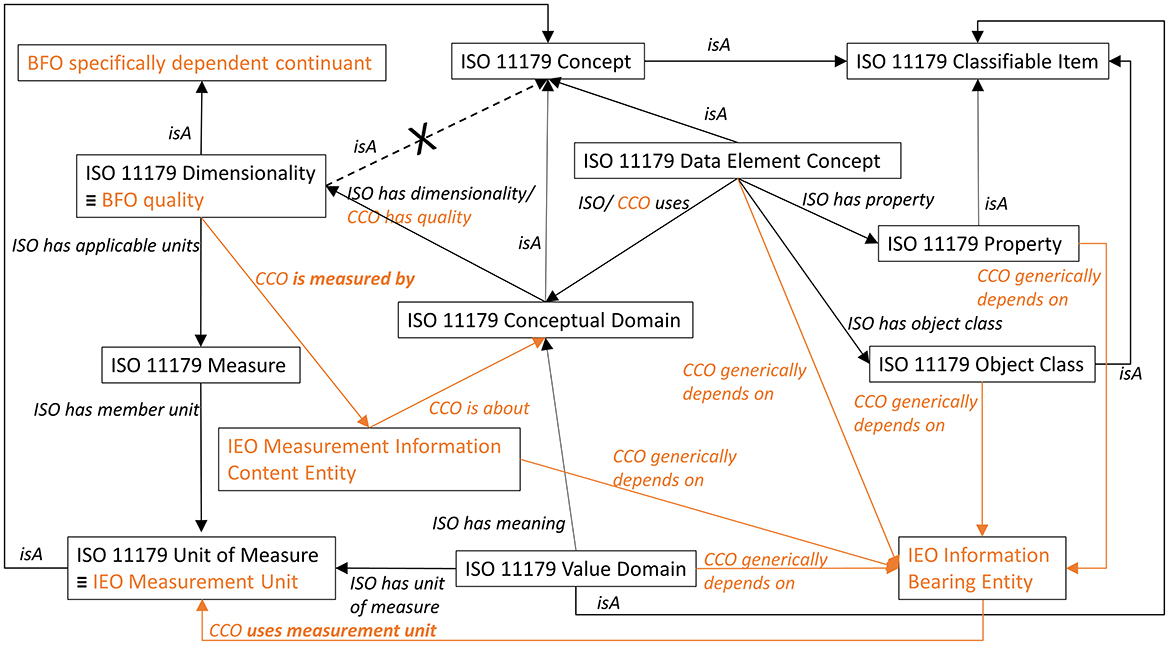
Figure 2. Integration with common core ontologies (CCO) of the International Organization for Standardization/International Electrotechnical Commission (ISO/IEC) 11179 entities.
Figure 1 illustrates the subclass relationship of the ISO 11179 Concept class with respect to the Information Entity Ontology (IEO) Descriptive Information Content Entity class and the relationships between the components involved in the classification concept of ISO/IEC 11179. Any entity subclassed from ISO 11179 Classifiable Item inherits the semantic relations that allow it to be classified according to some classification scheme. It can additionally relate to any entity in the entire ontology via the semantic relation describes.
Figure 2 shows the integration of the ISO/IEC 11179 entities: data element concept (comprising object class and property), value domain, and conceptual domain. All these elements are subclassed from the ISO 11179 Classifiable Item class and so inherit the associated classification attributes and the attributes in turn of its parent class, IEO Descriptive Information Content Entity.
The ISO/IEC 11179 entities on the right-hand side of Figure 2 integrate relatively smoothly within CCO. However, the entities on the left-hand side prove less straightforward due to the different taxonomies of their associated specifications. To not break the semantic conformity to CCO, the parent–child class relationship between the ISO/IEC 11179 entities Dimensionality and Concept had to be removed (as indicated by the crossed broken line in Figure 2). This does not present undue difficulties since the classification relations attributed to the Concept class can be added explicitly to the Dimensionality class.
Another slight issue is that although the basic formal ontology (BFO) quality class and the ISO 11179 Dimensionality class are essentially equivalent, the BFO quality class is measured by the IEO Measurement Information Content Entity class. The latter's representation is given by the IEO Information Bearing Entity class that references an IEO Measurement Unit. In contrast, the ISO 11179 Dimensionality class has an applicable set of measurement units that can be referenced directly from the ISO 11179 value domain class. Although this adds some extra complexity, it does not result in semantic contention.
2.2 Extending the framework to indicator contextualization
The next step in building the framework is to address the indicator contextualization needs. These include provisions for adding descriptive terms, links to standard data resources (such as dictionaries, thesauri, ontologies, etc.), derivation processes, provenance, and direct or indirect links (depending on data access rights) to the underlying data sources. To furnish the resulting framework with an appropriate name able to capture the keywords behind the contextualization concept, we coin the term SOLICIT as an acronym for “semantic ontology-labeled indicator contextualization integrative taxonomy.”
SOLICIT is a framework described in terms of the integrated CCO ISO/IEC 11179 ontology. However, in contrast to the solutions referred to in Section 1, it is not deployed as a standalone ontology imposed on all entities that must conform to a given indicator definition. SOLICIT provides a taxonomy of metadata elements that can be extended at the domain level to incorporate the associated specificities (the “integrative taxonomy” part of SOLICIT's acronym) and map to existing definitions at a federated level. A general dataset can be described with reference to the ontology metadata elements, which can be linked to standard data dictionaries, thesauri, and ontologies (the “semantic ontology-labeled” part of SOLICIT's acronym). Indicator datasets can be further described in terms of contextual metadata elements (the “indicator contextualization” part of SOLICIT's acronym).
An important design aspect of SOLICIT is the structured, scalable, and linkable nature of indicator contextualization metadata. An indicator can be described the same way as a data element, the harmonization of which is often referred to as a common data element (CDE). The National Institutes of Health (NIH) defines a CDE as “a data element that is common to multiple data sets across different studies” (Cohen et al., 2015). CDEs encourage the reuse of metadata and contribute to making data interoperable (NIH National Library of Medicine, 2023). SOLICIT views CDEs as atomic elements that undergo processing in some predefined way to formulate an indicator. The two aspects of structured, scalable metadata and semantic linkage form the underpinning concepts of SOLICIT.
Figure 3 represents the ISO/IEC 11179 data element within the SOLICIT framework. In conformity with ISO/IEC 11179, the data element class is associated with a data element concept, a value domain, and a derivation class. The latter describes how the data element is derived from (and/or derives) other data elements via some derivation method. Subclassing the ISO 11179 Data Element Derivation class from the BFO process class makes it possible to associate with the derivation method dispositions such as bias and limitations. SOLICIT also associates an IEO Information Bearing Entity class via the relation continuant part of , which can be used to reference the data source in which the given data element instance inheres (c.f. Section 3.2.7). SOLICIT subclasses the Context Specifying Data Element class under the ISO 11179 Data Element class and defines it as the parent class for the Indicator class.
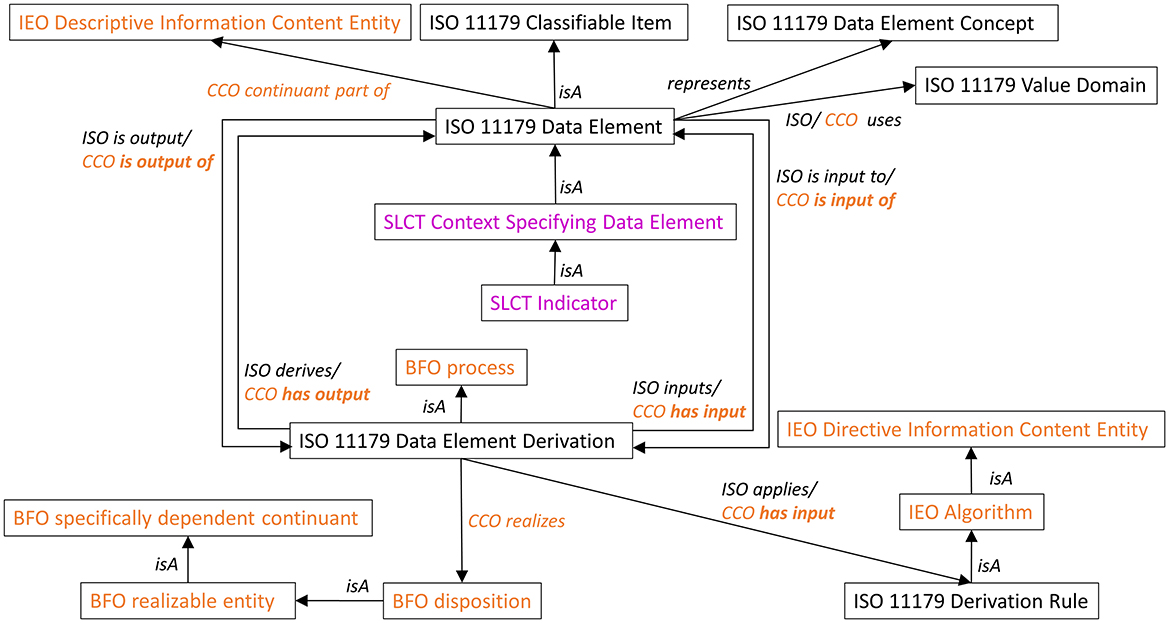
Figure 3. Representation of the International Organization for Standardization/International Electrotechnical Commission (ISO/IEC) 11179 data element within the semantic ontology-labeled indicator contextualization taxonomy (SOLICIT) framework.
Figure 4 illustrates the set of possible attributes of SOLICIT's Context Specifying Data Element class. Some attributes are inherited from its parent class. In contrast, others allow it to reference the following classes: BFO process, ISO 11179 Data Element, IEO Measurement Information Content Entity, and Extraction Process. Section 3 provides a more comprehensive description of these attributes with reference to a practical example of an indicator.
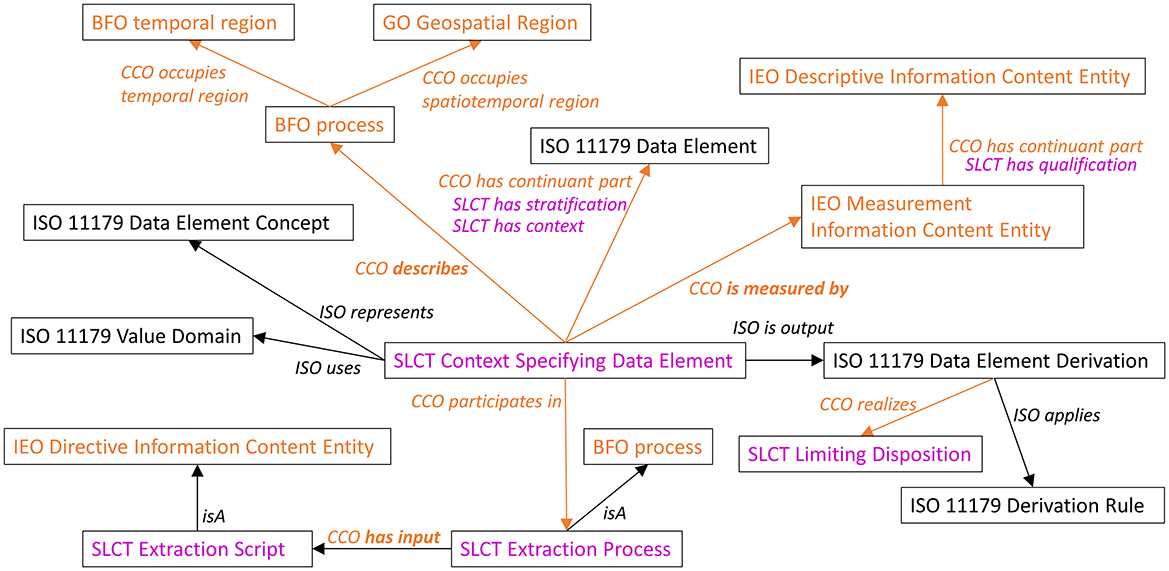
Figure 4. Attributes of semantic ontology-labeled indicator contextualization taxonomy's (SOLICIT's) Context Specifying Data Element class.
3 Results
In this section, several usage scenarios are presented, showing how SOLICIT can perform various functions that address many of the contextualization needs previously discussed in the introduction.
3.1 Definition of reusable metadata elements
Reusing metadata elements is desirable from the perspectives of metadata integrity and rigor of definitions. Segmenting metadata into the constituent parts of an ISO/IEC 11179 data element promotes reuse. For example, one can consider an epidemiological indicator measuring the incidence rates of a given disease. Incidence rates can be specified in several different ways, such as crude rates, age-specific rates, or age-standardized rates. The meanings of all these terms are common across disease domains; therefore, it would make sense to define them in an ontology at a broad disease domain level. Age-standardized rates are most helpful in comparing disease burdens across countries where the different population age structures could otherwise lead to misleading conclusions. Age-standardized rates are calculated by weighting the age-specific rates with the population distribution of a standard population. Since there are several standard populations (world standard population, European standard population, etc.), it is essential also to specify the population standard used in the calculation (especially where rates calculated with differed standard populations are compared between different world regions).
Drawing on its ISO/IEC 11179 foundation, SOLICIT allows the creation of an OWL class denoting age-standardized rate as an ISO/IEC 11179 object class. The OWL class can be comprehensively described using annotations and class hierarchies. The ISO/IEC 11179 property, also implemented as an OWL class, could then be defined as a particular population standard (e.g., European standard population) and reside in a class hierarchy with sibling classes denoting other population standards under a parent class denoting population standards in general.
An appropriate value domain could be the weighting factors for 5-year age brackets. The data element encapsulating this abstraction of age-standardized rates weighted by the European standard population in terms of 5-year age brackets is illustrated in the top left part of Figure 5, which shows part of the contextualization context of an example that will be described in Section 3.2.
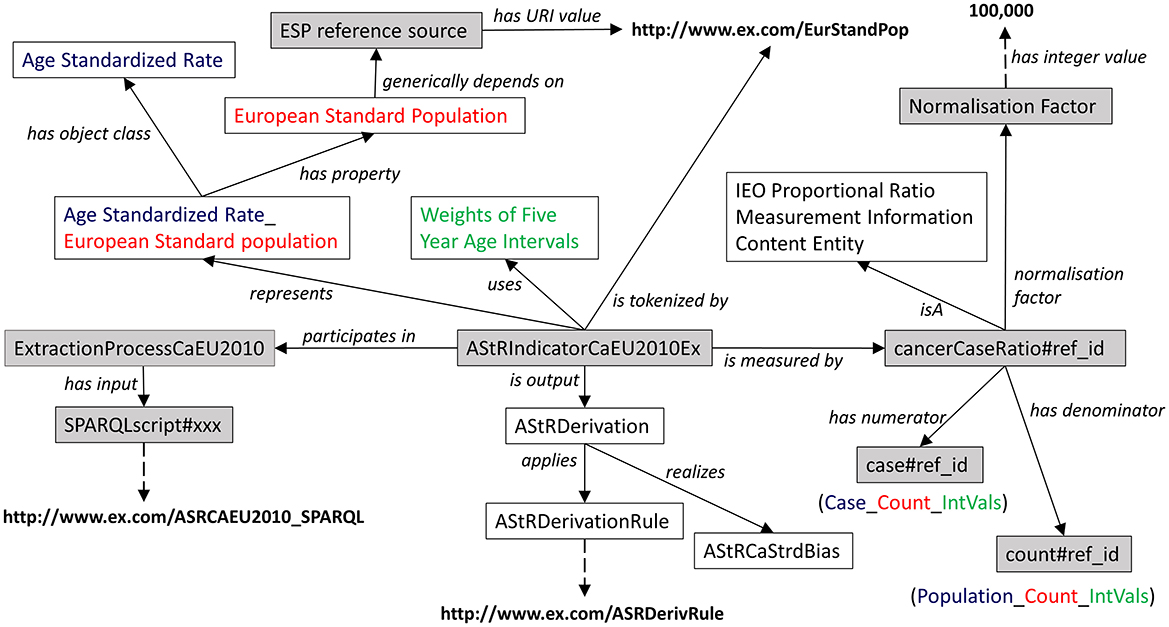
Figure 5. Encapsulation of an example indicator within the semantic ontology-labeled indicator contextualization taxonomy (SOLICIT) framework describing a subset of its semantic relations.
This composite approach to formulating metadata elements results in a relatively straightforward means for selecting and reusing existing metadata elements that can then be used to annotate data. In the SOLICIT acronym, this operation encapsulates the aspect of ontology labeling of a dataset or data variables within a dataset—a data user can dereference the entities in the SOLICIT ontology from the metadata links and understand the whole meaning of the associated metadata element(s).
3.2 Contextualization of indicators/data
An indicator dataset may be contextualized using the metadata labeling mechanisms of ISO/IEC11179 and via the semantic relationships described by the Context Specifying Data Element class (c.f. Figure 4). The age-standardized surveillance indicators for cancer cases in Europe are considered a practical example. These indicators are available from the European Cancer Information System (ECIS; European Commission, 2022). Data users can visualize and download the indicators from ECIS based on a set of interactive filters allowing the choice of country, sex, cancer site, year, and indicator type (i.e., incidence or mortality rates).
Figures 5–7 illustrate in further detail the semantic relations of an indicator shown in Figures 3, 4. SOLICIT's Indicator class derives from the Context Specifying Data Element class. The shaded boxes in the figures depict instances of ontology classes (individuals) instead of unshaded boxes, which depict ontology classes. Classes can be viewed as a general schema that can be made more specific at the individual level. In certain cases, the data element types of the individuals are indicated in parentheses in which the constituent parts of object class, property, and value domain are separated by underscores (object classes are denoted in blue font, properties in red font, and value domains in green font). Text marked in bold font denotes values of the respective individuals; broken arrows also refer to values of individuals but indicate that the intermediate steps (notably those from instances of the IEO Information Content Entity class to instances of the IEO Information Bearing Entity class, as required by CCO) have been omitted to preserve clarity in the figure.
The individual named AStRIndicatorCaEU2010Ex in the various figures contains the reference to the indicator. The indicator is contextualized by any number of attributes that can be extended to the degree necessary for comprehensively describing it. The semantic relations in which a SOLICIT Indicator class can participate are discussed below under their headings. The origin of these relations (from BFO, CCO, or SOLICIT) can be understood from Figures 3, 4.
3.2.1 Relation: is measured by
The relation is measured by, c.f. Figure 5 is defined by CCO with a set of subrelations; it has the range IEO Measurement Content Entity and can be used to describe how the indicator is measured. The measure is a ratio subclassed from the IEO Proportional Ratio Measurement Content Entity class. SOLICIT provides the additional relations of has numerator and has denominator (both with ranges IEO Descriptive Content Entity) and normalization factor to give more granularity. SOLICIT also introduces the relation has qualification that can be used to qualify any standard contextual class. This is illustrated in Figure 6 for the denominator of our example indicator. The denominator has the data element type composed of Population (object class), Count (property), and integer values (value domain). Whereas, less relevant for age-standardized rates, further qualification might sometimes be necessary to indicate the type of population considered (e.g., only officially resident, those suffering from a particular ailment, etc.) or the date of the population census.
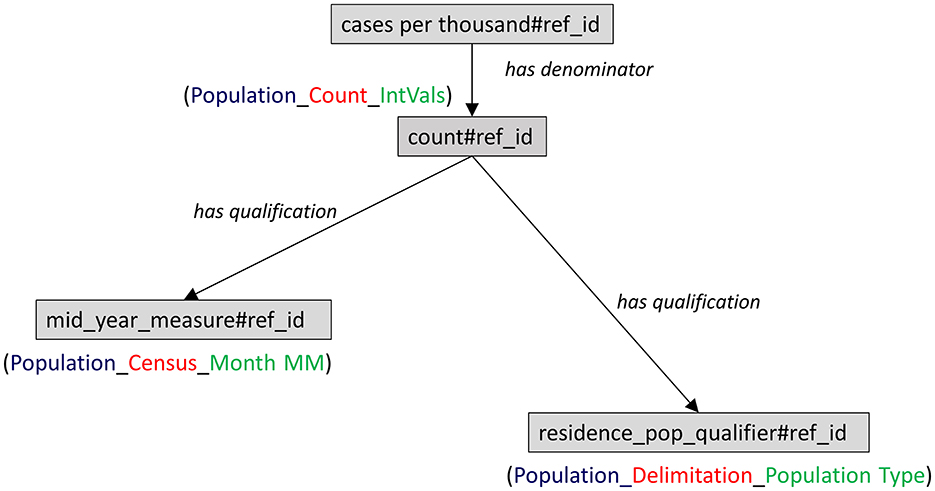
Figure 6. An example of qualifications assigned to the individual used as the denominator of the indicator shown in Figure 5.
3.2.2 Relation: is output
The relation is output describes how the indicator was derived; it has the range BFO process class, which defines the parent class of the ISO 11179 Data Element Derivation class (c. f. Figure 3). The latter can therefore be associated with the two further relations applies and realizes. The SOLICIT relation applies refers to the derivation rule (where the ISO 11179 Derivation Rule class is subclassed from the IEO Algorithm class). The relation realizes is defined by BFO with the range realizable entity, which is the parent class of the BFO class disposition. The latter can be used to describe any bias or limitation introduced by the indicator derivation method.
The derivation method of an indicator is necessary for understanding the quality and comparability of indicators derived using different methods. A common issue with indicators in the health domain is the heterogeneous types of underlying data sources which are dependent on the type of the health service infrastructure (Tolonen et al., 2021). In Figure 5, the derivation rule is indicated as a class but could as equally well be implemented at an individual level. Implementing it at both class and individual levels provides a useful way for specifying (a) a standard/preferred method for deriving the indicator and (b) the method applied for a given instance of the indicator (which may be necessary in relation to the primary data sources available). The standard method could be attributed to the class level and the method used at the instance/individual level.
3.2.3 Relation: participates in
In Figure 5, the BFO relation participates in (with the range of BFO process class) can be associated with the SOLICIT Extraction Process class used to describe the extraction process for the related data. The Extraction Process class uses the CCO relation has input with range IEO Directive Information Content Entity (c.f. Figure 4) to link to the extraction instructions. In the example indicator, the data file is assumed to be in RDF format, and the extraction method is a SPARQL script. However, the data extraction method could be specified in many different ways and provide access to datasets in any format, including binary, depending on the web services supported by the remote site.
3.2.4 Relation: has stratification
The SOLICIT has stratification relation is a subrelation of has qualification (c.f. Section 3.2.1). It has the range IEO Descriptive Information Content Entity and describes the variables according to which an indicator is stratified. In Figure 7, the European cancer age-standardized rates are stratified according to sex, geospatial code, indicator type (incidence/mortality), cancer site, and time period. Each of these stratification variables is, in fact, the data element named according to the convention of ISO/IEC 11179 in terms of an object class, property, and value domain, providing them with more modular description. The same data elements can be defined in SOLICIT for different domains, as illustrated in Figure 7, thereby facilitating reuse at the appropriate level of abstraction in the hierarchy of the domain spaces.
3.2.5 Relation: describes
The CCO relation describes (Figure 8) specifies the domain IEO Descriptive Information Content Entity and does not constrain its range. Therefore, a SOLICIT Indicator class can use this relation to describe many aspects of an indicator. Its primary use, however, is to describe the whole indicator process and, via the BFO and CCO relations pertaining to the BFO Process class, to specify the temporal and geospatial regions associated with the indicator. These regions can be described in terms of the associated CCO classes.
3.2.6 Relation: has context
The SOLICIT has context relation (Figure 8) is also a subrelation of has qualification and specifies the range IEO Descriptive Information Content Entity (and therefore, by association, the subclassed ISO/IEC 11179 data elements). The property components of these data elements could describe such aspects as the scope of the indicator, its uncertainties, assumptions, and limitations. This general relation can add any amount of descriptive information to the indicator. Dereferencing the composite data–element metadata fields would provide the explicit information of the associated contextual entity. In Figure 8, the value domains of these data elements are described in terms of text or keyword sets. However, they could equally well use more definitive types of value domains depending upon the needs of the indicator domain.
3.2.7 Relation: continuant part of
By adding the BFO relation continuant part of with reference to an IEO Descriptive Information Content Entity (c.f. top-left part of Figure 3), SOLICIT can associate an indicator with references to any other indicator instances. This functionality is helpful in cases where local and regional indicator sets feed a national or supranational indicator system. Moreover, these references can point to federated indicator sets and avoid the need to duplicate datasets at a central collection level. The bottom left-hand side of Figure 8 shows several individuals (ind set ref id_1... ind set ref id_n) associated with the indicator each via this relation. An extraction specification can therefore be defined for each individual to retrieve the related data and a reference to a SPARQL endpoint on the remote server. The latter can be queried to retrieve all the contextual information related to the remote indicator set. Figure 9 illustrates the mechanism in which the extraction process provides a SPARQL endpoint from which a “describe” query can be made on the name of the remote individual (local ind id 1) to ascertain and retrieve all the related contextual information. Since the remote indicator points to the data source from which it was derived, the end application can retrieve all of the data source's contextual information as well. From this contextual information, it can be determined whether the standard indicator derivation method or an alternative derivation method was used.
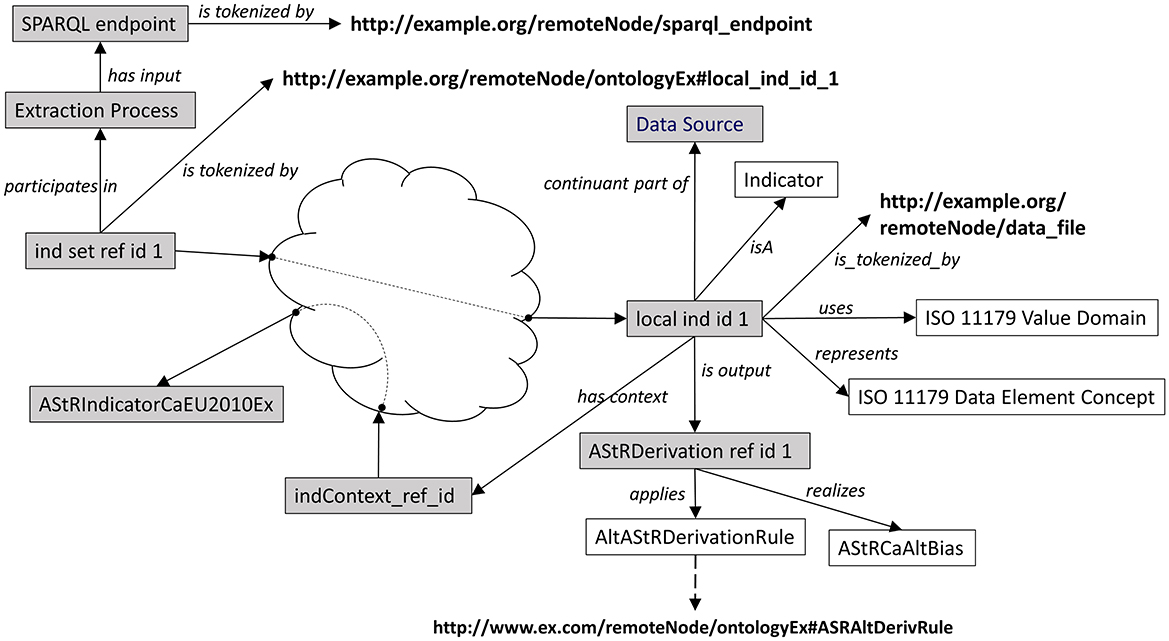
Figure 9. Semantic ontology-labeled indicator contextualization taxonomy (SOLICIT) provides the means to access an instance of an indicator residing in a remote node via a reference in the local node. In polling the information of the remote indicator, it is possible to retrieve any contextual information relating to the underlying data source(s) from which the indicator was constructed.
3.3 Classification and linkage to external terminology systems
ISO/IEC 11179 allows metadata items to be classified by classification schemes (c.f. Figure 1). This mechanism permits each of the data element components to be classified under a knowledge system, such as the simple knowledge organization system (SKOS), and linked via linked open data (LOD) principles to relevant entities defined and described in external terminology systems. Table 2 shows possible linkages for two data element components of the stratification data element Anatomical Site_Delimitation_ENCRCaSite (c.f. Figure 7). The classification relations are automatically inherited by the data element components from the ISO 11179 Classifiable Item class (c.f. Figures 1, 2).
To establish a link to a terminology system, SOLICIT uses a technique described initially by Anil Sinaci and Laleci Erturkmen (2013). The technique involves creating a classification scheme class for the terminology server (c.f. the example depicted in Figure 10 for the object class in Table 2 that links to an entity in the Genomic Epidemiology Application Ontology). Next, a classification scheme item (CSI) object is created that references the classification scheme via the semantic relation contained in. The CSI object is then populated with the type of relationship (such as a SKOS relation) to the relevant code of the classification system. The code is dereferenced via the associated uniform resource identifier (URI).
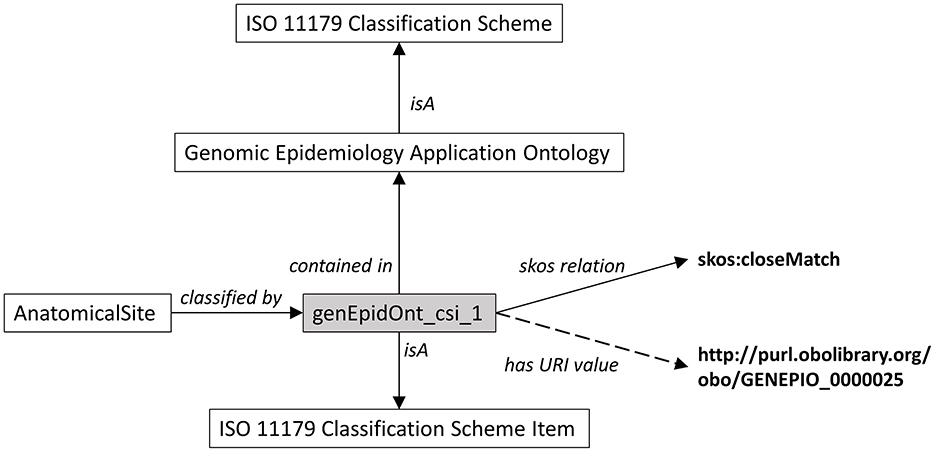
Figure 10. Example of how the second element of Table 2 can be linked and related to a specific code in a terminology system.
3.4 Dynamic tailoring of an indicator definition template
The complete definition of an indicator is essentially provided by the complementarity of an appropriate set of semantic relations and can, moreover, be formalized by drawing on the underlying description logic (DL) of OWL. Adding contextual information would serve to refine the definition. It could, in principle, also be possible to find all the related datasets adhering strictly to a given indicator definition by linking the definition with a federated query across the domain, providing a mechanism for updating indicators dynamically.
3.5 Searching and retrieving contextual information
Section 3.2 describes many types of queries that SOLICIT can support. Two further functionalities worth mentioning are discussed in the following subsections.
3.5.1 Cataloging facility
Each domain-level ontology can be queried to retrieve all the indicators registered within the domain. All indicator reference objects are subclassed from the SOLICIT class Indicator and thus a simple SPARQL query can retrieve them. If the ontologies of the registered nodes are set up as a SPARQL endpoint, a distributed search can be made to retrieve all indicators within the entire framework. Such functionality is useful, for instance, in a domain such as non-communicable diseases that consists of many subdomains of specific diseases.
3.5.2 Search by metadata element
SPARQL queries can also determine all the different ISO/IEC 11179 metadata elements (e.g., data element concept, data element, object class, property, and value domain) held in a SOLICIT-derived ontology. All the indicators using a given metadata element can therefore be retrieved across the entire framework. Moreover, any SKOS links established in the metadata descriptions and definitions can be followed using LOD principles to build up a comprehensive picture of what a given metadata element describes.
4 Discussion
SOLICIT is a generic indicator contextualization framework that aims to implement a domain-neutral starting point for developing indicator contextualization applications in specific domains. The framework is intended to be implemented as a set of domain-level ontologies that can each extend the more generic classes of SOLICIT's base ontology. Notably, the domain-level ontologies do not impose constraints on the data-providing mechanisms within the domain apart from describing the data with the SOLICIT-derived annotations. The prime incentive behind SOLICIT is to provide a standardized way in which to add any degree of required contextual information to an indicator (or data element) using common metadata description principles that promote the reuse and linkage of metadata in a scalable way.
SOLICIT draws on several standards and semantic web architectures to enable such a solution. The genericity is ensured to a large extent by building on a metadata standard (ISO/IEC 11179) and the formal interfaces of top- and mid-level ontology layers. SOLICIT uses CCO, although in principle it could use any mid-level ontology layer. The CCO suite was chosen due to its extensive nature across general domains and because it derives from BFO, an international standard (ISO/IEC 21838-2:2021). The main challenge is to integrate the metadata standard into the ontology interface.
Within SOLICIT, contextualization metadata can be described in a structured form (such as the stratification variables) or unstructured text. Whereas, a framework of this type should allow a degree of flexibility, there are specific contextual fields that would benefit from standard constructs—in particular, those able to frame some quality metric for the indicator (e.g., assumption, limitation, uncertainty, bias, etc.). Other descriptive entities are more directly related to the quality of the underlying data, such as accuracy, consistency, completeness, validity, and uniqueness. They can be dereferenced from the semantic relations linking the data elements to the underlying data sources. Describing data quality is a field of active research, and although various quality assessment methods and frameworks have been proposed (Cichy and Rass, 2019; Ozonze et al., 2023; Bian et al., 2020; Shekhovtsov and Eder, 2020), they are yet to gain broad consensus, and there is divergence even on the meaning and scope of the individual quality dimensions themselves. In view of the difference of opinion on this topic, SOLICIT leaves it to the specificities of the domain, but in future extensions could integrate standard quality frameworks when mature. Indeed, the greater the extent to which contextual information can be structured, the easier and more straightforward it will be to provide. A concept such as SOLICIT could then potentially furnish an automatic means (e.g., via DL) for inferring a set of quality metrics based on standard contextual classes selected for a given indicator or dataset.
SOLICIT can add an extra degree of functionality to common data models (CDMs) such as the Observational and Medical Outcomes Partnerships (OMOP) CDM. The OMOP CDM is designed to standardize the structure, format, and terminologies of otherwise disparate datasets to facilitate systematic analyses across a federated data network (Kent et al., 2021). CDMs are an effective instrument for allowing interoperable data exchange, but they do not capture the whole picture behind the datasets. Even though data are standardized to the interoperable degree necessary for inclusion into a given analytical study, the study's results may be severely compromised by an uncircumspect use of the data arising from unwarranted assumptions about their relative quality or applicability. SOLICIT can provide such information.
4.1 Ability to address indicator recommendations
The good indicators guide (British National Health Service, 2024) suggests that most of the essential metadata elements of an indicator can be clarified by considering ten basic questions relating to aspects such as measurement criteria, data provenance, accuracy and limitations, rationale, etc. SOLICIT contains the constructs that can cater well to most of the requirements. Examples of SPARQL scripts demonstrating how SOLICIT can provide such contextual information are included for an example indicator in the data distribution referenced in the Data availability statement.
4.2 A facilitating framework
SOLICIT was not devised to solve a specific domain-focused issue but rather to address the general and domain-independent need for a harmonized means of contextualizing data. SOLICIT is a flexible and versatile framework that can be used for many different purposes largely due to the standards and semantic web technologies on which it was built.
The query and retrieval mechanisms discussed in Section 3 could be handled without difficulty in a dedicated user interface. This would allow the development of data portals at a domain level to search and retrieve indicator sets across all the subdomains and different countries/regions.
The contextualization entities and their cross-references to standard data dictionaries and thesauri via LOD principles are a useful aid for deep learning and artificial intelligence (AI) tools to draw inferences on the usage and integration of the contextualized data. These inferences could then be traced to the contextual descriptions, providing the means for verifying the reasoning processes. SOLICIT can also provide the contextual “glue,” allowing analyses of data described by heterogeneous data standards.
5 Conclusion
Comparing indicators in a meaningful way across distributed heterogeneous entities is a complex process that needs to consider relevant contextual information for a correct interpretation. However, few tools are available describing contextual information in a harmonized, scalable, and non-prescriptive way, while allowing effective reuse of metadata. This need has motivated the concept of the SOLICIT framework proposed here.
SOLICIT is a pragmatic approach to providing a generic, domain-neutral indicator and data contextualization framework. By drawing on the dual strengths of ISO/IEC 11179 and CCO, SOLICIT provides a practical means for structuring and linking contextual metadata according to a standard model that also allows the inclusion of domain specificities to any degree of granularity. It, therefore, serves as a standard and harmonized starting point from which to develop comprehensive, domain-specific indicator contextualization metadata.
The contextual information is machine-readable, given its ontological representation in OWL. It can be dereferenced on a per need basis, making it usable by AI applications and machine-learning algorithms. In particular, it facilitates the automatic inferencing processes, allowing downstream data processes to make informed decisions about the applicability of the indicator/data for a given purpose. Moreover, it provides the means of independent confirmation of any resulting analyses and can add additional value to common data standards.
SOLICIT may be considered a critical enabler toward “FAIR-ification” of data (Wilkinson et al., 1970) and indicators. Not only can data be found over distributed data sources via searches on the ISO/IEC 11179 object class and property keywords, but data access is achieved via the data extraction specification. The tripartite linkage of the ISO/IEC 11179 data element components using LOD principles enables mapping each constituent metadata element to standard terminology systems for interoperability purposes. SOLICIT permits the integration of data from different domains by providing users a complete overview of the context of the data for application in the ways intended (and can, therefore, also serve to extend the shelf-life and utility of legacy data, which is particularly important in the health domain).
It is hoped that the ideas presented here will stimulate further reflection and contribution in formulating standard frameworks for contextualizing data. There remains a critical need to agree on an adaptable solution able to enrich and contextualize datasets in a comprehensive way that is not overly prescriptive and can serve to search and integrate data distributed across different domains and subdomains.
Data availability statement
The datasets presented in this study can be found in online repositories. The names of the repository/repositories and accession number(s) can be found at: SOLICIT Indicator Contextualization Framework, https://data.jrc.ec.europa.eu/collection/id-00410.
Author contributions
NN: Writing – review & editing, Writing – original draft, Software, Methodology, Investigation, Formal analysis, Conceptualization. IŠ: Writing – review & editing, Writing – original draft, Methodology, Investigation, Formal analysis, Conceptualization.
Funding
The author(s) declare that no financial support was received for the research, authorship, and/or publication of this article.
Acknowledgments
This study was conducted using Protégé.
Conflict of interest
The authors declare that the research was conducted in the absence of any commercial or financial relationships that could be construed as a potential conflict of interest.
Publisher's note
All claims expressed in this article are solely those of the authors and do not necessarily represent those of their affiliated organizations, or those of the publisher, the editors and the reviewers. Any product that may be evaluated in this article, or claim that may be made by its manufacturer, is not guaranteed or endorsed by the publisher.
Abbreviations
AI, artificial intelligence; BFO, basic formal ontology; CCO, common core ontologies; CDE, common data element; CDM, common data model; CSI, classification scheme item; DL, description logic; ECHI, European core health indicators; ECIS, European cancer information system; ENCR, European Network of Cancer Registries; FAIR, findable, accessible, interoperable, reusable; IEO, information entity ontology (part of CCO); KPI, key performance indicator; LOD, linked open data; M&E, monitoring and evaluation; NIH, National Institutes of Health; OMOP, observational medical outcomes partnership; OWL, web ontology language; RDF, resource description framework; RO, relation ontology; SDG, sustainable development goals; SKOS, simple knowledge organization system; SOLICIT, semantic ontology-labeled indicator contextualization integrative taxonomy; SPARQL, SPARQL protocol and RDF query language; UMO, units of measure ontology (part of CCO); CCO, common core ontologies; ISO/IEC, International Organization for Standardization/International Electrotechnical Commission; W3C, World Wide Web Consortium.
References
Anil Sinaci, A., and Laleci Erturkmen, G. B. (2013). A federated semantic metadata registry framework for enabling interoperability across clinical research and care domains. J. Biomed. Informat. 46, 784–794. doi: 10.1016/j.jbi.2013.05.009
Barclay, M., Dixon-Woods, M., and Lyratzopoulos, G. (2018). The problem with composite indicators. Br. Med. J. Qual. Saf. 28, 338–344. doi: 10.1136/bmjqs-2018-007798
Basic Formal Ontology (2020). Basic Formal Ontology. Available at: https://basic-formal-ontology.org (accessed July 10, 2024).
Bian, J., Lyu, T., Loiacono, A., Mendoza Viramontes, T., Lipori, G., Yi, G., et al. (2020). Assessing the practice of data quality evaluation in a national clinical data research network through a systematic scoping review in the era of real-world data. J. Am. Med. Informat. Assoc. 27, 1999–2010. doi: 10.1093/jamia/ocaa245
Bogaert, P., van Oers, H., and Van Oyen, H. (2018). Towards a sustainable EU health information system infrastructure: a consensus driven approach. Healthc. Pol. 122, 1340–1347. doi: 10.1016/j.healthpol.2018.10.009
Bowen, S., and Kreindler, A. S. (2018). Indicator madness: a cautionary reflection on the use of indicators in healthcare. Healthc. Pol. 3, 41–48. doi: 10.12927/hcpol.2013.19918
British National Health Service Institute for Innovation and Improvement. (2024). The Good Indicators Guide: Understanding How to Use and Choose Indicators. Available at: https://www.england.nhs.uk/improvement-hub/wp-content/uploads/sites/44/2017/11/The-Good-Indicators-Guide.pdf (accessed July 10, 2024).
Cichy, C., and Rass, S. (2019). An overview of data quality frameworks. IEEE Access 7, 24634–24648. doi: 10.1109/ACCESS.2019.2899751
Cohen, M. Z., Thompson, C. B., Yates, B., Zimmerman, L., and Pullen, H. C. (2015). Implementing common data elements across studies to advance research. Nurs. Outlook 63, 181–188. doi: 10.1016/j.outlook.2014.11.006
del Mar Roldán-García, M., García-Nieto, J., Maté, A., Trujillo, J., and Aldana-Montes, J. F. (2021). Ontology-driven approach for KPI meta-modelling, selection and reasoning. Int. J. Inform. Manag. 58:102018. doi: 10.1016/j.ijinfomgt.2019.10.003
Espinoza-Arias, P., Poveda-Villalón, M., García-Castro, R., and Corcho, O. (2019). Ontological representation of smart city data: from devices to cities. Appl. Sci. 9:32. doi: 10.3390/app9010032
European Commission (2013). European Core Health Indicators (ECHI). Available at: https://health.ec.europa.eu/indicators-and-data/european-core-health-indicators-echi_en (accessed July 10, 2024).
European Commission (2020). European Cancer Inequalities Registry (ECIR). Available at: https://cancer-inequalities.jrc.ec.europa.eu (accessed July 10, 2024).
European Commission (2022). European Cancer Information System (ECIS). Available at: https://ecis.jrc.ec.europa.eu (accessed July 10, 2024).
Fischer-Mackey, J., and Fox, J. (2022). Pitfalls of “slippery indicators”: the importance of reading between the lines. Dev. Pract. 33, 665–674. doi: 10.1080/09614524.2022.2104220
Fox, S. M. (2015). The role of ontologies in publishing and analyzing city indicators. Comput. Environ. Urb. Syst. 54, 266–279. doi: 10.1016/j.compenvurbsys.2015.09.009
Giancarlo, G., and Guarino, N. (2023). Semantics, Ontology and Explanation. Cornell Tech, Cornell University.
Global Health Data (2024). Health Indicators. Available at: https://globalhealthdata.org/health-indicators (accessed July 10, 2024).
Gruber, T. (2018). “Ontology,” in Encyclopedia of Database Systems, eds. L. Liu and M. T. Özsu (New York, NY: Springer), 2574–2576.
Houston, M. (2021). KPIs: What Are They, and Why Are They Important? Forbes. Available at: https://www.forbes.com/sites/melissahouston/2021/12/29/kpis-what-are-they-and-why-are-they-important (accessed July 10, 2024).
International Agency for Research on Cancer (2024). Cancer Incidence in Five Continents (CI5). Available at: https://ci5.iarc.who.int (accessed July 10, 2024).
ISO (2015). Information Technology—Metadata Registries (MDR)—Part 1: Framework. Available at: https://www.iso.org/obp/ui/#iso:std:iso-iec:11179:-1:ed-3:v1:en (accessed July 10, 2024).
Jensen, M. G, De Colle Kindya, S., More, C., Cox, A. P., and Beverley, J. (2024). The common core ontologies. arXiv:2404.17758. doi: 10.48550/arXiv.2404.17758
Kent, S., Burn, E., Dawoud, D., Jonsson, P., Østby, J. T., Hughes, N., et al. (2021). Common problems, common data model solutions: evidence generation for health technology assessment. Pharmacoeconomics 39, 275–285. doi: 10.1007/s40273-020-00981-9
Kosten, A. J. (2016). Classification of the use of research indicators. Scientometrics 108, 457–464. doi: 10.1007/s11192-016-1904-7
Lamhauge, N., Lanzi, E., and Agrawala, S. (2013). The use of indicators for monitoring and evaluation of adaptation: lessons from development cooperation agencies. Clim. Dev. 5, 229–241. doi: 10.1080/17565529.2013.801824
Lyytimäki, J., Salo, H., Lepenies, R., Büttner, L., and Mustajoki, J. (2020). Risks of producing and using indicators of sustainable development goals. Sustain. Dev. 28, 1528–1538. doi: 10.1002/sd.2102
Mair, S. A, Jones, A. J, Ward, Christie, I., Druckman, A., et al. (2018). “A critical review of the role of indicators in implementing the sustainable development goals,” in Handbook of Sustainability Science and Research, World Sustainability Series, ed. W. Leal Filho (Cham: Springer), 41–56.
Moravcsik, J. M. (1988). “Chapter 1—some contextual problems of science indicators,” in Handbook of Quantitative Studies of Science and Technology, ed. A. F. J. Van Raan (Amsterdam: Elsevier), 11–30.
NIH National Library of Medicine (2023). Common Data Elements: Standardizing Data Collection. Available at: https://www.nlm.nih.gov/oet/ed/cde/tutorial/03-100.html (accessed July 10, 2024).
OBO Foundry (2024). Relation Ontology. Available at: https://obofoundry.org/ontology/ro.html (accessed July 10, 2024).
OECD (2014). Measuring and Managing Results in Development Co-operation, a Review of Challenges and Practices Among DAC Members and Observers. Available at: https://www.oecd.org/development/peer-reviews/Measuring-and-managing-results.pdf (accessed July 10, 2024).
OECD (2023). Health at a Glance. Available at: https://www.oecd.org/health/health-at-a-glance (accessed July 10, 2024).
Ontotext (2022). What Are Ontologies? Available at: https://www.ontotext.com/knowledgehub/fundamentals/what-are-ontologies (accessed July 10, 2024).
Ozonze, O., Scot, P. J., and Hopgood, A. A. (2023). Automating electronic health record data quality assessment. Med. J. Syst. 47:23. doi: 10.1007/s10916-022-01892-2
Pon, R. K., and Buttler, J. D. (2009). “Metadata registry, ISO/IEC 11179,” in Encyclopedia of Database Systems, eds. L. Liu and M. T. Özsu (Boston, MA: Springer), 1724–1727.
Shekhovtsov, V. A., and Eder, J. (2020). Metadata quality for biobanks. Appl. Sci. 12:9578. doi: 10.3390/app12199578
Soldi, R. (2017). The Management of Health Systems in the EU Member States. Publications Office of the European Union, Brussels, Belgium.
Terzi, S., Otoiu, A., Grimaccia, E., Mazziotta, M., and Pareto, A. (2021). Open Issues in Composite Indicators, a Starting Point and a Reference on Some State-of-the-Art Issues, Roma TrE-Press. Available at: https://romatrepress.uniroma3.it/wp-content/uploads/2021/03/open-togmp.pdf (accessed July 10, 2024).
Tolonen, H., Reinikainen, J., Koponen, P., Elonheimo, H., Palmieri, L., Tijhuis, J., et al. (2021). Cross-national comparisons of health indicators require standardized definitions and common data sources—archives of Public Health. BioMed. Central 2021:586958. doi: 10.21203/rs.3.rs-586958/v1
UNAIDS (2010). An Introduction to Indicators. Available at: https://www.unaids.org/sites/default/files/sub_landing/files/8_2-Intro-to-IndicatorsFMEF.pdf (accessed July 10, 2024).
van den Berg, M., Achterberg, P., Hilderink, H., Verma, A., and Verschuuren, M. (2019). “Structuring health information: frameworks, models and indicators,” in Population Health Monitoring. Climbing the Information Pyramid, eds. M. Verschuuren and H. van Oers (Cham: Springer), 35–58.
W3C (2013). SPARQL 1.1 Query Language. Available at: https://www.w3.org/TR/sparql11-query/ (accessed August 20, 2024).
W3C and Semantic Web (1997). SKOS Simple Knowledge Organization System. Available at: https://www.w3.org/2004/02/skos/ (accessed August 20, 2024).
W3C and Semantic Web Standards (2012). Web Ontology Language (OWL). Available at: https://www.w3.org/OWL/ (accessed August 20, 2024).
W3C and Semantic Web Standards (2014). Resource Description Framework (RDF). Available at: https://www.w3.org/RDF/ (accessed August 20, 2024).
W3LinkedData, C. (2024). LinkedData. Available at: https://www.w3.org/wiki/LinkedData (accessed August 20, 2024).
Wilkinson, M. D., Dumontier, M., Aalbersberg, I. J., Appleton, G., Axton, M., Baak, A., et al. (1970). The Fair Guiding Principles for Scientific Data Management and Stewardship. Scientific Data. Available at: https://dash.harvard.edu/handle/1/26860037 (accessed October 01, 2024).
Keywords: federated indicator framework, linked open metadata, indicator contextualization, metadata registry, metadata architecture, common core ontologies, federated data, data representation
Citation: Nicholson N and Štotl I (2024) A generic framework for the semantic contextualization of indicators. Front. Comput. Sci. 6:1463989. doi: 10.3389/fcomp.2024.1463989
Received: 12 July 2024; Accepted: 25 September 2024;
Published: 14 October 2024.
Edited by:
Davide Lanti, Free University of Bozen-Bolzano, ItalyReviewed by:
Holger Stenzhorn, Saarland University Hospital, GermanyLaszlo Balkanyi, University of Pannonia, Hungary
Copyright © 2024 Nicholson and Štotl. This is an open-access article distributed under the terms of the Creative Commons Attribution License (CC BY). The use, distribution or reproduction in other forums is permitted, provided the original author(s) and the copyright owner(s) are credited and that the original publication in this journal is cited, in accordance with accepted academic practice. No use, distribution or reproduction is permitted which does not comply with these terms.
*Correspondence: Nicholas Nicholson, bmljaG9sYXMubmljaG9sc29uQGVjLmV1cm9wYS5ldQ==
†These authors share first authorship