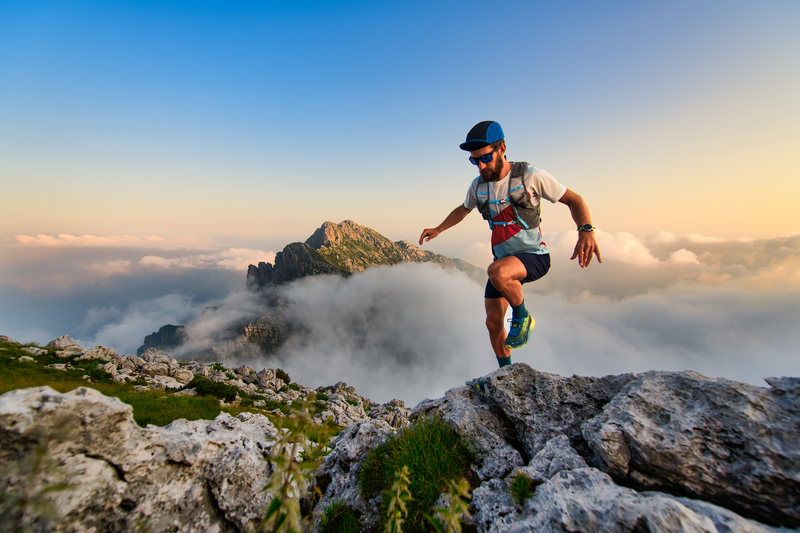
94% of researchers rate our articles as excellent or good
Learn more about the work of our research integrity team to safeguard the quality of each article we publish.
Find out more
SYSTEMATIC REVIEW article
Front. Comput. Sci. , 11 September 2024
Sec. Networks and Communications
Volume 6 - 2024 | https://doi.org/10.3389/fcomp.2024.1452961
This article is part of the Research Topic Sustainable Development in Artificial Intelligence, Blockchain and Internet of Things View all 5 articles
Background: The occurrence of diseases in rice leaves presents a substantial challenge to farmers on a global scale, hence jeopardizing the food security of an expanding global population. The timely identification and prevention of these diseases are of utmost importance in order to mitigate their impact.
Methods: The present study conducts a comprehensive evaluation of contemporary literature pertaining to the identification of rice diseases, covering the period from 2008 to 2023. The process of selecting pertinent studies followed the guidelines outlined by Kitchenham, which ultimately led to the inclusion of 69 studies for the purpose of review. It is worth mentioning that a significant portion of research endeavours have been directed towards studying diseases such as rice brown spot, rice blast, and rice bacterial blight. The primary performance parameter that emerged in the study was accuracy. Researchers strongly advocated for the combination of hybrid deep learning and machine learning methodologies in order to improve the rates of recognition for rice leaf diseases.
Results: The study presents a comprehensive collection of scholarly investigations focused on the detection and characterization of diseases affecting rice leaves, with specific emphasis on rice brown spot, rice blast, and rice bacterial blight. The prominence of accuracy as a primary performance measure highlights the importance of precision in the detection and diagnosis of diseases. Furthermore, the efficacy of employing hybrid methodologies that combine deep learning and machine learning techniques is exemplified in enhancing the recognition capacities pertaining to diseases affecting rice leaves.
Conclusion: This systematic review provides insight into the significant research endeavours conducted by scholars in the field of rice disease detection during the previous decade. The text underscores the significance of precision in evaluation and calls for the implementation of hybrid deep learning and machine learning methodologies to augment disease identification, presenting possible resolutions to the obstacles presented by these agricultural hazards.
One of the most widely used staple food of India is rice (Oryza sativa L). Across the world also, rice is considered as the main crop (Jiang et al., 2020). Asia is covered 90% by the production of rice crop only. Hence, it is a significant agricultural crop. It is expected that world’s population count will be approximately 9.6 billion which ultimately gives the indication of increase in demand of food production (Food Summit, 2009). This summons the requirement of more arable lands but stress on environment is affecting crop production a lot (Wani et al., 2022). So, this is pressing priority to enhance the crop production by providing the security to the livelihood to whole nation.
Crop diseases, on the other hand, can drastically lower productivity and quality, posing a serious danger to global food supplies. Many factors that threaten its security are changes in climatic conditions (Malik et al., 2023), pollinators, crop fields, and diseases in crops (Alkanan and Gulzar, 2024; Gulzar, 2024). Prediction and identification of rice diseases is necessity of an hour otherwise there will be a great loss for farmers as well as for industries (Islam et al., 2018). Spray consisting of Bacillus megaterium, chemical fungicide spray, etc. is used for controlling rice diseases but researchers observed that these are applicable only for particular rice diseases not for all types of rice diseases (Dhiman et al., 2023a,b). Hence, spraying method is not suitable for controlling rice disease. Therefore, to provide safety and security to the farmers, tools related to latest technology are required which can ultimately reduce the direct involvement of farmers for detection of rice disease.
As a result, disease management is crucial for rice production (Wang et al., 2021). The critical element of disease management is accurate and quick disease detection, which allows pesticide countermeasures to be addressed in a timely manner. Manual judgement based on disease exterior appearance is currently the most extensively used technique for diagnosing rice crop diseases. On large farms, automatic computer vision identification allows farmers to anticipate disease more efficiently and in less time. Farmers have failed to regulate numerous farming methods; therefore they failed to control them. If a farm is infected with a pest or illness, it should be treated as soon as possible. Leaf screening can detect the majority of plant illnesses and deficits. There are insufficient persons with the necessary expertise in the region to complete such activities in a timely way. Farmers used some methods to inspect the plant on a regular basis, and if no evidence of disease is seen, they prefer to use a fertiliser or pesticide. However, methods used by farmers also fail to identify the disease in the infected plant. This makes the fertilisers ineffective, which will subsequently harm the plant and soil. To make the diagnosis process of rice disease automatic is the greatest research nowadays. As a result, a more effective and accessible technique for diagnosing rice illness is desired. There exists variety of image processing techniques for the detection of rice disease (Joshi and Jadhav, 2016). From the last decade, many researchers have used the machine vision system and agriculture field for evaluating crop production. Various techniques have been utilized to diagnose the crop diseases using image processing, machine learning, hyperspectral, pattern recognition, classification and deep learning technique (Cubero et al., 2016).
It is clear from the studies that manual identification of the rice disease by farmers is very time consuming and expensive task (Islam et al., 2021). Uses of computer vision for the detection of disease via rice images have gained lot of attention nowadays. But this technology is not effective for larger datasets. Hence, to improve the identification rate, usage of DL technology has been done. Mainly work has been done on the rice diseases. High quality camera or rice images from laboratories have been used the collection of data as this is very major task in the recognition of diseased plant or healthy plant. Past few years have shown that extensive work is done the field of recognition of rice disease by using DL methods. Our study also gives attention to review the DL models which have been used either for detection or prediction of rice disease. Eight research questions have been considered and discussed in detail. Five major collection sites of our work are Springer, ScienceDirect, Scopus, IEEE Xplore, and ACM Digital library.
Our motivation behind this work is: The yield of a crop is majorly affected by the growth of diseased plant. Numerous researchers have worked upon the formula for the prediction of rice disease plant. It is observed that most of the rice loss is because of bacterial, insects, and fungal infection. The timely detection of rice disease is very important so as to prevent the financial, labor, and resources loss. To prevent such losses, systematic literature survey has been done for the detection of rice diseased plants. The main focus of our work is to provide the researchers with the systematic and exhaustive review by doing the extensive literature survey of different databases of rice diseases. Researchers have worked upon the formula for the prediction of rice disease plant (Khairnar and Dagade, 2014). It is observed that most of the rice loss is because of bacterial, insects, and fungal infection (Shrivastava et al., 2021). The timely detection of rice disease is very important so as to prevent the financial, labor, and resources loss. To prevent such losses, systematic literature survey has been done for the detection of rice diseased plants. The main focus of our work is to provide the researchers with the systematic and exhaustive review by doing the extensive literature survey of different databases of rice diseases.
The following section briefly describes the different kinds of disease present in rice plants (Appalanaidu and Kumaravelan, 2021). The commonly occurring rice diseases images are represented in Figure 1. Also brief introduction of some of these are discussed.
• Leaf blast: Dark patch to oval blotch with thin reddish-brown edges and grey or white centre are common signs of this disease.
• Brown spot: This disease affects the rice plant’s leaves. The disease reveals itself as round to oval lesions that are dark brown in colour.
• Sheath blight: The disease affects both the leaves and the stems. Oval, white or straw- coloured zones in the centre with reddish brown blotches are the symptoms.
• Leaf scald: Narrow reddish-brown broad stripes are the common symptoms. Lesions with yellow or golden borders are sometimes found along the leaf edges.
• Bacterial leaf blight: Symptoms include prolonged sores on the leaf blade that are few inches long and turn yellow to white because to the bacteria’s action.
• Narrow Brown spot: Disease occurs in potassium deficient soils during the late growth stages of the rice crop, starting at heading stage.
The organization of this paper is as follows: Section 2 provides the overview of the deep learning methods used for identification of rice diseased plants. Procedure of conducting systematic literature review is presented in section 3. Section 4 provides the discussion on research questions. Section 5 discusses the recommendations for researchers. Section 6 concludes the work and provides insight to the researchers for future.
There are several processes that are often included in the process of image processing. The subsequent subsections provide an explanation of the several stages involved in general image processing. Figure 2 illustrates the process to diagnose and classify the diseases and sequential procedures entailed in the process of image processing.
This initial stage involves the collection of the static or dynamic state of a specific object by the utilization of diverse equipment, such as cameras or scanners. Primarily, the images are acquired in digital format. If the obtained image is not in digital format, the process of converting it to digital through analog-to-digital conversion is applied to the acquired image. The selection of the image sensor is determined based on the specific application requirements. In various applications such as scanners, digital cameras, and video cameras, the predominant choice for image sensing technology is typically either CMOS (complementary metal oxide semiconductor) or CCD (charge- coupled device) (Rumy et al., 2021).
The initial stage of data preparation, known as pre-processing, involves transforming raw data into processed data. Digital computers are employed throughout the pre-processing phase. Before undergoing pre-processing, it is necessary to convert the images into a digital format. In the field of image processing, many operations, including data formatting, correction, and enhancement, are carried out to produce images of higher quality. The further stages in digital image processing, following pre-processing, encompass picture segmentation, feature extraction, and image classification (Bellapu et al., 2021; Ayoub et al., 2022, 2023).
Image segmentation is the computational process of dividing a picture into distinct sections or segments based on specific criteria. The number of segments depends on the application and user requirements. This process involves the precise separation of an image into components, extracting distinct contours for streamlined visual representation, which facilitates tasks like image editing, compression, and object recognition (Dhiman et al., 2023a,b) Thresholding algorithms are commonly used to enhance image quality during segmentation, where each pixel is assigned a label to group similar pixels together.
Diverse content characteristics are derived from visual content through extraction, which is crucial for classification and selection. Feature selection, a pre-processing technique, reduces feature count in classification tasks, ensuring only discriminative features are used. Incorrect feature choice can hinder classification effectiveness, making accurate feature extraction vital (Huang et al., 2023). The extracted features form a feature vector, representing the image. This process extracts attributes like edges, colour, morphology, and texture from the image. Key characteristics of image features are fundamental to digital image analysis and manipulation, essential for understanding image processing techniques.
The colour characteristic is widely utilized in picture categorization as a visual attribute. The characterization of images based on colour is advantageous due to several reasons, including reduced storage needs, simplified implementation, increased resilience, computational efficiency, and enhanced effectiveness (Ding Z. et al., 2023).
In the context of image content description, form is regarded as a fundamental and visual characteristic. The precise characterization of shape content description poses challenges due to the crucial task of discerning dissimilarities between shapes. Therefore, it is imperative to employ a similarity measurement technique to compare the derived features in shape-based picture retrieval. Form descriptors can be categorized into two primary groups: contour-based descriptors, which utilize local cues such as boundary segments, and region-based descriptors, which consider the entire area of an item for form description (Ding B. et al., 2023).
Texture plays a significant role in the retrieval process, serving as a robust geographical descriptor and a crucial characteristic of a picture. The capacity to identify similar images is limited in terms of texture; nonetheless, it possesses the capability to distinguish between textured and non-textured images. Furthermore, it integrates the textured images with an additional visual characteristic, such as colour, in order to optimize the effectiveness of the retrieval process. Textural features encompass several characteristics such as fractals, contrast, entropy, wavelets, homogeneity, and statistical metrics. There exists a variety of extraction strategies that can be employed to extract pertinent and captivating characteristics from the input image.
Image classification is a computer vision procedure that categorizes an image based on its visual characteristics. For example, an algorithm can be designed to detect the presence of a human figure in an image. While people easily detect objects, reliable image classification is a major challenge in computer vision. It requires multiple image samples of similar objects and a well-designed classification scheme. Users can choose a classification system based on their needs, using methodologies like fuzzy logic, artificial neural networks, and expert systems (Salaba and Chan, 2023).
Machine learning is a subfield of computational intelligence and AI focused on developing techniques and models based on statistics. It enables computer systems to acquire knowledge and improve performance through experience without explicit programming. Machine learning involves creating systems that mimic human behavior and decision-making by learning from data. Algorithms are trained to identify patterns and extract features from extensive datasets, allowing them to make informed decisions and predictions on new data (Jordan and Mitchell, 2015). The learning process is automated and enhanced by accumulating experiences. High-quality data is used to build models, and various techniques train computers. Machine learning models are now widely used in crop pathogen detection and categorization.
Deep learning is a specialized domain within the broader field of artificial intelligence, which is concerned with the advancement and utilization of ANN. Deep model is a contemporary machine learning methodology that focuses on algorithms that draw inspiration from the configuration and functionality of the brain, namely artificial neural networks with representation learning. The term “deep” typically denotes the quantity of concealed layers within the neural network. Traditional neural networks typically consist of a limited number of hidden layers, typically ranging from 2 to 3. In contrast, deep neural networks have the capacity to incorporate a significantly larger number of hidden layers, allowing for increased complexity and potential for improved performance. The emergence of deep learning has introduced a range of innovative algorithms that have reduced the necessity for manually designed characteristics prior to the classification process. Deep learning has been incorporated in many for problem solving such as healthcare (Khan et al., 2023; Mehmood et al., 2023), finance (Gulzar et al., 2023), as well agriculture (Amri et al., 2024).
Various machine learning and deep learning techniques are employed for detecting rice plant diseases. These advanced methods leverage the power of computational algorithms to analyze and interpret complex patterns in plant data. This section explores the diverse range of techniques based on ML and DL methods for detection of rice diseased plants.
Numerous machine learning techniques are employed for identifying diseased rice plants. Researchers have proposed various novel algorithms to enhance the detection accuracy of rice plant diseases.
The segmentation technique based on Fermi energy has been proposed to classify the infected portion of the image. A novel algorithm was developed to characterize the symptoms of the disease with the help of domain experts using colour, shape and position features of the disease. In order to minimize the loss of information and to reduce the complexity of the model, the rough set theory was used to select the relevant features. After the feature selection process rule-based classification was implemented to classify the images into two classes of healthy and infected images (Wang et al., 2023).
Three types of rice diseases namely bacterial leaf blight, brown spot and leaf smut are detected by using Ostu’s segmentation technique. After that various features were extracted from the segmented image by using histogram of oriented gradients and local binary pattern. Support vector machine with polynomial kernel and HOG is used to classify the features (Chen et al., 2021). Pest infection significantly degrades the quality and quantity of rice crops, with insufficient domain knowledge exacerbating the issue (Zhou et al., 2023). The proposed model uses machine vision and a genetic algorithm to detect pest infection, achieving 92.5% accuracy with artificial neural networks and 87.5% with support vector machines (Thakur et al., 2023). New techniques detect and classify rice diseases using image processing to determine RGB values of affected regions. Naive Bayes classifier identifies rice bacterial blight, rice blast, and brown spot with minimal computation cost by focusing on RGB values (Islam et al., 2018).
Machine learning based algorithm was used to detect the plant disease automatically. Total 300 healthy and infected leaves images were used by the proposed model. Training accuracy achieved by the model for the infected and healthy images are 99 and 100%, respectively. The test accuracy is achieved to be 90 and 86% for the infected and the healthy leaf images, respectively, (Cubero et al., 2016). The image data set of the plants show variability in different kinds of features like texture colour and size. The image processing techniques using K mean clustering algorithm have been used by the paper in order to improve the quality of image dataset. The combination of SVM, CNN and K mean clustering algorithm-based classification techniques is used for the detection of disease in rice crops. The training accuracy achieved by the model is 95% (Daniya and Vigneshwari, 2022). The ADSNN-BO model, based on MobileNet and augmentation techniques, detects four types of rice plant diseases using a public dataset. Optimized with Bayesian techniques, the mobile-capable model achieves 94.65% test accuracy, with filter visualization and activation map analysis confirming its interoperability (Ahad et al., 2023). The image processing technique has been used to classify the diseases in rice plants with the help of leaves images. Different features like colour shape and texture are extracted and classified using well established models on a public data set of the rice leaf images (Ganatra and Patel, 2020). Features play an important role for image classification in machine learning applications (Kitpo and Inoue, 2018). Enhanced deep learning networks improve disease identification using deep features. Classifying four rice leaf diseases with 5,932 field images, the study shows that an SVM, used alongside a CNN with transfer learning, outperformed the CNN alone (Gayathri Devi and Neelamegam, 2019).
Rice, a major food in India, requires humid, hot conditions (above 25°C) and over 0.1 mm rainfall for healthy growth. Despite favorable conditions, diseases and infections hinder production. A convolutional neural network with probabilistic neural network architecture is proposed to classify rice crops as healthy or infected (Goluguri et al., 2021). Agriculture is a key pillar of India’s economy, but diseases reduce plant growth and production, leading to financial losses. Early disease detection through computer vision systems can help control the spread by classifying data from digital images (Faizal Azizi and Lau, 2022; Dhiman, 2014). The images are pre-processed and segmentation techniques are applied to produce the better outcome. A machine learning approach is used to detect and classify leaf diseases. The results are analyzed and compared with support vector machines, Nearest Neighbor, Artificial neural networks and random forest. The random forest techniques outperformed the other techniques (Hasan et al., 2019).
The utilization of numerous deep learning techniques has become a prominent approach for the identification of diseased rice plants. Researchers have proposed a variety of novel algorithms, including advanced deep learning models, in an effort to enhance the detection accuracy of rice plant diseases (Pan et al., 2023). This area of study has garnered significant attention within the scientific community, as the accurate and timely identification of plant diseases is crucial for maintaining healthy crop yields and ensuring food security.
Researchers have recently focused on detecting diseased rice plants to prevent losses due to delayed identification. This overview discusses DL approaches for identifying rice plant diseases. Deep learning uses hierarchical artificial neural networks to facilitate learning, enabling computers to categorize data through direct exposure to image data. Training deep networks involves large annotated datasets and architectures that extract features directly from input data, eliminating the need for manual feature extraction. Various advanced deep learning architectures and visualization techniques have been employed to identify and categorize plant diseases. A key benefit of DL algorithms is their ability to incrementally learn high-level features from data. Over the past decade, deep learning has become a prominent research topic in image recognition and classification. A novel disease detection method using convolutional neural networks was proposed, utilizing a dataset of 500 images of healthy and infected rice leaves. The model detected 10 common rice plant diseases with 95.48% accuracy (Chumthong et al., 2016). A deep convolutional neural network model classified different types of plant leaves with an average accuracy of 99.5%. The dataset included 27 classes of six crops with complex intra- and inter-class variations. Results showed the deep learning system classified images efficiently with a time complexity of 0.016 s without compromising accuracy (Ghosal and Sarkar, 2020). Diseases affecting wheat and rice can negatively impact growth and production. Three rice leaf diseases and wheat leaf diseases were analyzed using 40 images. The VGG16 deep learning model, using transfer learning, detected diseases with accuracies of 97.22% for rice and 98.75% for wheat (Ghyar and Birajdar, 2017). Enhanced deep learning networks have shown improved results in disease identification using deep features. Four types of rice leaf diseases (blast, brown spot, tangram, and bacterial blight) were classified using 5,932 field images. A CNN model with a transfer learning approach and SVM was used, with SVM outperforming CNN (Gayathri Devi and Neelamegam, 2019; Simhadri and Kondaveeti, 2023). Although CNNs have advanced manual diagnostics, their computational complexity limits real-time remote sensing applications. Filter pruning algorithms address this, and a new approach called Pruning Filter with Attention Mechanism enhances CNN speed and compression by selecting filters for pruning through a correlation-based approach with an attention module (Verma et al., 2023). This method outperformed current benchmarks on three remote sensing datasets.
Detecting microbial diseases in rice fields using computationally intensive algorithms on mobile devices presents challenges, especially in areas with limited internet connectivity. Farms adopting digital agriculture need plant-specific solutions to address crop viruses effectively (Mique and Palaoag, 2018). Current microbial illness detection technology struggles with real-time inferences on portable devices. This study proposes RiceBioS, a handheld AI and deep learning-powered device, to detect abnormalities in rice crops using mobile computing capabilities. RiceBioS utilizes Edge-as-a-Service to categorize rice plant images into infected and healthy, employing pruning to reduce model complexity. The user-friendly RiceBioS app provides real-time insights for farmers, aiding informed decision-making with advanced technologies (Zhang et al., 2020).
Efficient disease diagnosis is crucial for mitigating financial losses in agriculture. The ADSNN-BO system, using MobileNet and an augmented learning algorithm with a Bayesian optimizer, achieves accurate disease diagnosis in rice plants. Lightweight CNN architectures like MobileNet and NasNet Mobile, optimized for mobile devices, offer high accuracy with reduced model size, demonstrating efficacy in practical applications (Shrivastava et al., 2021; Phadikar et al., 2013).
The guidelines given by Kitchenman are performed to carry out the systematic literature review. Figure 3 shows the step by step execution of the given protocol. The basic steps to follow in this study are:
• Identifying the research questions: This phase describes the relevant research questions that are linked with the identification of rice diseased plant.
• Constructing the system for search: This phase analyzes the study that will assist in answering our research questions. This can be done with the help of appropriate search string and examining the literature sources for performing the related search.
• Assessment of study quality: This phase identifies those studies from the selected study that tunes with our study. This selection is done based on inclusive and exclusive criteria. Selection of appropriate study is very important here, as it will strengthen our study.
• Extraction of data: Vital information is fetched based on research questions and tabular representation is done.
• Synthesis of data: Classification and grouping of data is done on the basis of research questions.
• Threats to the validity of search: This phase covers the threat that may be few of the relevant papers get skipped while collecting the relevant papers.
Our SLR focuses on identifying the empirical relevance of rice disease models that exist on the basis of deep learning. Keeping this in mind, few research questions that are analyzed and designed are mentioned in Table 1. Motivation behind the research questions is also discussed.
The purpose of constructing the system for search is to get assistance in answering the research questions. This can be done with the help of appropriate search string and examining the literature sources for performing the related search. The combination of following terms is done to initiate this phase of SLR:
• Discovering the terms from the designed research questions.
• Detection of synonyms of these terms.
• Observing the keywords from the study material.
• Use of boolean ‘OR’ to help the search system of synonym terms.
• Use of boolean ‘AND’ to connect the terms.
By considering all the relevant terms of rice diseases, the search string is made by using the combination of synonyms and ‘OR’ boolean operator along with the ‘AND’ boolean operator. All the apropos terms based on neural networks and deep learning that are applicable on the rice diseases are figured out. The most widely used search string is:
(rice AND (diseases OR disease OR infection) AND (neural net- works OR Deep learning OR Artificial networks))
After selecting papers through this search string, the papers from references of these papers are also taken into consideration.
The selection of appropriate search term is successful only if the search is done from the opposite sources. So, the search string we framed is applied in the following data sources:
• ACM Digital Library
• IEEE Xplore
• ScienceDirect
• Scopus
• SpringerLink
The selection of these databases is done after finding out that these sources are most widely used in the domain of rice disease.
After applying search string on the mentioned databases, the next step is to do selection on the basis of title, keywords, and abstract. The search is done from the studies of year 2009 and considered till latest research on the rice diseases. In total, 124 studies were found related to rice diseases, but after going through the criteria of inclusion/exclusion only 84 studies were left to be considered. 59 studies were finally left after doing the proper quality assessment on these shortlisted studies. These steps are illustrated in Figure 4.
The selection of those studies is done from the selected studies that tunes with our study. This selection is done based on inclusive and exclusive criteria. Selection of appropriate study is very important here, as it will strengthen our study. Here, selection of studies is done based on title, keywords, and abstract. During this selection, many papers were selected that were not appropriate according to the research questions designed. The inclusive and exclusive criteria of our approach are:
• Inclusion of journal articles only if paper is published in journal as well as conference.
• Finding those studies which provide proper methodology to the designed research questions.
• The studies provide clear results with discussions.
• The studies that are highly correlated with our research.
• Studies that are not mapping with the designed research questions.
• Studies whose empirical analysis is not done.
To finally select the studies that highly impacts our studies, few questionnaire for quality assessment criteria are designed. This questionnaire is designed on the basis of recommendations mentioned in (Dhiman et al., 2023a,b). The following questions were designed after doing brainstorming and critical thinking with the experts.
Q1: Are the objectives behind the research clear?
Q2: Is the dataset appropriate for the experiment?
Q3: Is the approach for the collection of the data defined?
Q4: Are the deep learning techniques defined in bold way?
Q5: Is the measurement of accuracy of proposed models done in definitive way?
Q6: Is the comparison of deep learning models done with the other methods?
Q7: Are the results and discussions palpable?
Q8: Is the study having a good average citation?
Vital information is fetched on the basis of designed research questions. Here, the manipulation of the selected studies is done on the basis of numerous synonyms that are used for deep learning models. The indirect collection of data is done by properly processing the collected data. For example, artificial neural networks, convolutional neural networks, recurrent neural networks, deep convolutional networks are the different name used for the representation of same model.
The main purpose of this step is to do the classification and grouping of data from the finally selected studies based on research questions. At this phase, there exists qualitative as well as quantitative data. The process of synthesis of data from the extracted data is done by using narrative Synthesis process (Singh et al., 2018). Explains the process of doing systematic review based on the most widely used text in the summarized findings. All designed research questions are evaluated through this technique only.
Selection of relevant studies is done on the basis of search string and designed research questions. But, this phase covers the threat that may be few of the relevant papers get skipped while collecting the relevant papers. There are some chances of missing relevant papers if the text of selected strings is not part of keywords, abstract and title. This risk of not including significant studies in our work is considered as threat.
In this section, the results of each research question are discussed in detail. To prove the findings, some relevant studies are also provided. Table 2 provides the overview of studies which provides answers to these research questions.
From the selected studies, it is observed that rice diseases are categorized into three types bacterial, pest-based, and fungal diseases. Most of the rice diseases identified are Rice Hispa, Stem Borer disease, Rice brown spot, Rice bacterial blight, Rice blast, Leaf smut, Rice streak spot, Rice sheath rot, Rice sheath blight, Nigrospora, Black bug, Golden apple snail, Tungro, Stripe blast, Leaf lesion, and Red blight. Table 3 the rice diseases identified from studies. Figure 5 depicts the different types of diseases. Figure 6 represents the distribution of disease count from selected studies.
For the identification of rice diseases from the leaves, various datasets are used. For the research community, these datasets are either available publicly or privately. The publicly available dataset can be accessed by anyone whereas private datasets are to be accessed by the owner of the dataset only. In considered studies also, it is observed that most of the researchers have created their own datasets by using various sensors and camera. The images of rice plants are being used for training the models as well as for the testing of models. Table 4 specifies the details of the datasets being used in the considered studies. It can be clearly observed that most of the studies have considered their private datasets.
RGB images of the rice leaves are most widely used rice images that are used for identification of rice disease. Few studies have also done colour conversion for the purpose of pre-processing. Very rare studies have used the other rice images like HSV for the recognition of rice diseases. Table 5 gives the details of the rice images used along with the reference of the studies.
Image processing involves the task of applying few operations on a selected portion of image so as to extract some useful features from an image. An image is given as input and after applying image processing tasks on an image, few required features are extracted from an image. Few following image processing tasks are uprooted from the studies taken into consideration:
1. Colour space conversion
2. Segmentation
3. Noise removal
4. Median filter
5. Image enhancement like cropping, rotation, resizing
6. Contrast enhancement
7. Masking
8. Principal component analysis
Table 6 gives the outline of the image processing techniques which are being used in studies selected for consideration. Image enhancement like cropping, rotation, resizing Segmentation and Segmentation are mostly used by researchers for processing of an image.
Following deep learning models are used for prediction of rice diseases in the considered studies:
1. Convolutional neural networks (CNN): Different frameworks of CNN
2. Recurrent Neural Network (RNN)
3. Deep convolutional neural networks (DCNN)
4. Artificial neural networks (ANN)
5. Long Short-Term Memory (LSTM)
6. Faster Recurrent convolutional networks (Faster R-CNN)
7. Hybrid convolutional networks (Hybrid CNN)
8. Generative Adversarial Network (GAN)
Table 7 shows the variety of deep learning techniques that are used for the identification of rice disease and Figure 7 shows distribution of DL techniques in selected studies.
For the evaluation of deep learning models, various performance parameters are being used. Recognition speed, Mean square error, Accuracy, Precision, Recall and kappa coefficient are the parameters used for evaluation of performance. Accuracy represents the recognition rate of the proposed model. Accuracy performance parameter is mentioned in all the considered studies. Table 8 represents various deep learning techniques used for detection of rice diseases.
The studies that have been taken into consideration includes 64% journals and 36% conference proceedings. Computers and Electronics in Agriculture journal is the most leading journal in this field.
Table 9 displays the illness types categorized by several researches together with the corresponding accuracies. Various image capture methods, such as those based on unmanned aerial vehicles (UAVs), multispectral imaging, and remote sensing, have been utilized by researchers to collect private data by means of digital cameras and sensors tailored to the specific region or orchards in question. Public datasets such as the BRRI, IRRI, Kaggle, plant village, www, and citrus image gallery datasets are frequently utilized in these investigations. The table also provides a brief explanation of the research’ limitations. The process of image classification in DL heavily depends on features, which play a crucial role in accurately identifying and distinguishing specific objects or patterns present in images. Deep-CNNs have emerged as a prominent technique for accurately identifying and classifying rice diseases by using deep features, resulting in promising outcomes Table 10. Offers the comprehensive review of relevant literature pertaining to the chosen studies within this particular topic of study.
The above survey provides some useful directions for the research to be done in the field of detection of rice disease. The summarized study of our work is: Most of the researchers have probed in rice brown spot, rice blast, and rice bacterial blight diseases whereas golden apple snail, stripe blast, red blight, and leaf lesion needs attention. Many researchers achieved the best results due to image pre- processing techniques being applied on the rice leaf images.
• Various deep learning techniques are used in this field but the hybrid approach is promoted for future work. Accuracy is the determined in almost all of the studies and to achieve good accuracy there is dependence on the datasets. Very few researchers have used the public datasets rather they have created their own datasets. Researchers have also provided the suggestions for creating the datasets and how to make it public.
• The research conducted in this study has successfully identified a range of rice diseases, encompassing bacterial, pest-induced, and fungal ailments. Various diseases such as Rice Hispa, Stem Borer disease, and Rice brown spot are cited. Various image processing techniques are employed in the identification of rice diseases, such as colour space conversion, segmentation, noise removal, and picture enhancement, among others.
• The selected studies frequently utilized segmentation and picture enhancement techniques. Various deep learning strategies are employed in the realm of rice disease identification, encompassing Convolutional Neural Networks (CNN), Deep Convolutional Neural Networks (DCNN), Artificial Neural Networks (ANN), and additional methodologies. The deep learning models that are most commonly employed are CNN and DCNN.
• Multiple studies have utilized a range of performance metrics, with accuracy being the predominant metric employed. The research included in the analysis reported the accuracy of deep learning models. Private databases derived from pictures of rice plants are frequently utilized by researchers. A limited number of studies made use of publicly accessible datasets. The majority of studies (64%) are disseminated through publication in academic journals, while the remaining portion is presented in conference proceedings. RGB images of rice leaves have been widely employed for the purpose of disease identification. Several investigations also utilized colour conversions such as HSV.
• This comprehensive literature analysis offers significant insights into the current state of research on rice disease detection utilizing deep learning approaches and provides guidance for future investigations in this domain.
This systematic literature review provides a comprehensive overview of different studies that utilize deep learning and image processing techniques to automate the detection and classification of rice leaf. The survey findings reveal that a majority of researchers have focused on developing a widely accepted framework for the diagnosis of leaf diseases in rice. Few limitations observed from the existing work are mentioned as follows:
• Currently, the majority of solutions for diagnosing rice diseases rely on offline images, and none of these studies have achieved acceptable accuracy in real-world scenarios. Although there are a few web portals and mobile applications available, such as Leaf Coder, at Leaf+ and Leaf Doctor, they only provide information on limited dysfunctions of paddy, and are specific to certain cultures. Therefore, there is a need to develop a mobile application and web portal that can directly predict paddy diseases using online images, providing more accurate and accessible assistance to the public.
• To effectively apply Convolutional Neural Networks (CNN) for various tasks, it is necessary to have an advanced architecture that has sufficient computational and memory resources. However, integrating the expert system concept into computer vision, machine learning and deep learning techniques can be a viable solution to this challenge. This could be a promising area of research for scholars and professionals in this field who are interested in developing such a system.
• Extensive frameworks have been proposed to classify healthy and diseased rice images but it lacks a sufficient dataset for deep neural networks to learn profound and discriminative features. Although the dataset has been augmented using a few techniques, there is room for exploring more techniques to expand the dataset. Another issue is the difficulty of collecting real-time field-level data, which limits the applicability of the few proposed algorithms to only a few types of rice diseases.
• To overcome these limitations, future research could explore the use of agricultural sensors to collect images with varying illumination and angles of capture. Additionally, expanding the dataset through techniques such as flipping, cropping, and rotation could be explored to address the issue of small datasets. Over the next few decades, a major hurdle will be to create methods and strategies that can quickly identify disease outbreaks, especially those caused by new or developing plant pathogens, and precisely pinpoint the responsible agents (Singh et al., 2022).
• The availability of real and high-quality data is a significant challenge for the effectiveness of these models. New models need to be proposed to ensure they are provided with appropriate data for training. A current limitation is the presence of noise and outliers in existing data, which adversely affects model performance.
• Currently, most researchers have focused on accuracy as the primary performance parameter. However, many other performance parameters such as AUC-ROC, confusion matrix, training time, inference time, robustness, scalability, resource utilization, and loss function can also be explored in future studies.
Different modules were used to begin the process of detecting rice diseases across four separate classes of rice leaves, as shown in Figure 8 is used in this case study. The image collection for this study was gathered from online sources such as PlantVillage and Kaggle (Dhiman et al., 2023a,b).
Firstly, the image samples of rice plants undergo pre-processing by applying rescaling to each image in order to obtain enhanced photographs. Furthermore, the data augmentation technique is utilized to generate a greater quantity of image samples for training purposes. There are a wide range of techniques available for segmenting photos, including both traditional thresholding approaches and more advanced colour and frequency-domain techniques. The Otsu method and K-means clustering are widely regarded as the most dependable and well-liked algorithms for picture segmentation due to their straightforward computational process. Typically, the K value and the Region of Interest (ROI) are manually selected, relying on the user’s expertise. However, manually selecting the ROI is a time-consuming process and is susceptible to errors. In order to overcome this limitation, one can employ automated clustering methods to identify disease spots on rice plant leaves (Lu et al., 2023). The study employed K-means clustering to automatically categorize disease zones in rice leaves. The distinction between the colours of the diseased and healthy sections of the picture samples was achieved by employing a thresholding technique. Only the diseased region of the leaf was made visible by deliberately masking off pixels with lower red values relative to blue and green values. The utilization of segmentation approaches is crucial for finding and highlighting the specific areas that are pertinent to disease inquiry and classification. Next, feature extraction is utilized to categorize various illnesses present in the plant picture samples. This study utilized the Keras framework with a TensorFlow backend for model training. The CNN architecture is designed with a sequential structure, where each layer receives input images from the preceding layers. The initial Convolutional Layer accepts input images with dimensions of (256, 256, 16). CNNs are very suitable for a diverse array of challenging signal processing tasks. CNNs are highly suitable for a diverse array of intricate signal propagation applications. The Rectified Linear Unit (ReLU) activation function was used for its rapid training performance. The input layer is composed of the Convolution layer, ReLU activation layer, and pooling layer. The outcome of the implemented models has been analyzed using an 80 × 20 cross validation. The proposed model utilizes a cross entropy loss function to evaluate the effectiveness of the approach. The work utilizes the Adam optimizer to enhance the cross entropy function ratio. The suggested work is evaluated based on several assessment metrics, including precision (P), recall (R), F1 score (F), and accuracy (Acy). Table 11 displays the results of the multi-classification model utilizing the CNN model across different stages of case study. With 94.25% accuracy, 96.32% sensitivity, 92.73% specificity, 94.46% precision, and 95.91% fi-score, it achieved these results.
In this paper, systematic literature review has been done to identify rice diseases using deep learning models. This research compares and contrasts state-of-the-art machine vision algorithms used for categorization with various restrictions connected with the rice plants’ sensing mechanism. For the purpose of the rice plant detection system, this review discusses various image processing approaches. The selection of 69 studies has been done for the purpose of deeply analyzing their work and to uproot the relevant information. The selection of studies is done from the year 2008 to 2023. Most of the research work is taken from the studies of last 4 years. Total seven research questions are taken into consideration. Different types of rice diseases are identified from these studies. Different variety of deep learning techniques is explored in these studies. The best accuracy is observed in case of hybrid approaches of deep learning or deep transfer learning techniques. CNN and DCNN are most widely used by the researchers for the identification of rice diseases. All aspects of the categorization procedure have been thoroughly examined. Consideration of important sensor, feature, and classification algorithm properties is given in a comprehensive study. The present critical issues in this subject can be better understood by comparing the classification techniques that are in use. In future, the researchers may collaborate deep learning with the machine learning for creating hybrid approach and to achieve better results in recognition rate. More deep learning techniques can also be explored in this field to make this area more active and ultimately benefiting the practitioners of the rice field.
The original contributions presented in the study are included in the article/supplementary material, further inquiries can be directed to the corresponding author.
PS: Conceptualization, Data curation, Formal analysis, Investigation, Methodology, Software, Visualization, Writing – original draft. PD: Conceptualization, Data curation, Formal analysis, Investigation, Methodology, Software, Writing – original draft. YG: Conceptualization, Data curation, Formal analysis, Funding acquisition, Investigation, Methodology, Project administration, Resources, Software, Supervision, Validation, Visualization, Writing – original draft, Writing – review & editing. DK: Conceptualization, Data curation, Investigation, Methodology, Software, Supervision, Validation, Visualization, Writing – original draft, Writing – review & editing. SW: Conceptualization, Data curation, Formal analysis, Methodology, Writing – review & editing. CO: Conceptualization, Investigation, Methodology, Writing – review & editing.
The author(s) declare that financial support was received for the research, authorship, and/or publication of this article. This work was supported by the Deanship of Scientific Research, the Vice Presidency for Graduate Studies and Scientific Research, King Faisal University, Saudi Arabia, under Project KFU241433.
The authors declare that the research was conducted in the absence of any commercial or financial relationships that could be construed as a potential conflict of interest.
All claims expressed in this article are solely those of the authors and do not necessarily represent those of their affiliated organizations, or those of the publisher, the editors and the reviewers. Any product that may be evaluated in this article, or claim that may be made by its manufacturer, is not guaranteed or endorsed by the publisher.
Ahad, M. T., Li, Y., Song, B., and Bhuiyan, T. (2023). Comparison of CNN-based deep learning architectures for rice diseases classification. Artif. Intell. Agric. 9, 22–35. doi: 10.1016/j.aiia.2023.07.001
Ahmed, K., Shahidi, T. R., Alam, S. M. I., and Momen, S. (2019). Rice leaf disease detection using machine learning techniques. 2019 international conference on sustainable Technologies for Industry 4.0 (STI).
Ahmed, I., and Yadav, P. K. (2023). Plant disease detection using machine learning approaches. Expert. Syst. 40:e13136. doi: 10.1111/exsy.13136
Alkanan, M., and Gulzar, Y. (2024). Enhanced corn seed disease classification: leveraging MobileNetV2 with feature augmentation and transfer learning. Front. Appl. Math. Stat. 9:1320177. doi: 10.3389/FAMS.2023.1320177
Amri, E., Gulzar, Y., Yeafi, A., Jendoubi, S., Dhawi, F., and Mir, M. S. (2024). Advancing automatic plant classification system in Saudi Arabia: introducing a novel dataset and ensemble deep learning approach. Model. Earth Syst. Environ. 10, 2693–2709. doi: 10.1007/s40808-023-01918-9
Appalanaidu, M. V., and Kumaravelan, G. (2021). Plant leaf disease detection and classification using machine learning approaches: a review. Innovations in computer science and engineering: Proceedings of 8th ICICSE.
Arinichev, I. V., Polyanskikh, S. V., Arinicheva, I. V., and Sergeeva, I. O. (2021). Applications of convolutional neural networks for the detection and classification of fungal rice diseases. IOP conference series: Earth and environmental science.
Asvitha, S., Dhivyaa, T., Divyasree, H., and Bhavadharini, R. M. (2024). A survey on plant diseases detection using different ML/DL techniques. In AIP conference proceedings.
Atole, R. R., and Park, D. (2018). A multiclass deep convolutional neural network classifier for detection of common rice plant anomalies. Int. J. Adv. Comput. Sci. Appl. 9, 67–70. doi: 10.14569/IJACSA.2018.090109
Ayoub, S., Gulzar, Y., Reegu, F. A., and Turaev, S. (2022). Generating image captions using Bahdanau attention mechanism and transfer learning. Symmetry 14:2681. doi: 10.3390/sym14122681
Ayoub, S., Gulzar, Y., Rustamov, J., Jabbari, A., Reegu, F. A., and Turaev, S. (2023). Adversarial approaches to tackle imbalanced data in machine learning. Sustain. For. 15:7097. doi: 10.3390/su15097097
Azim, M. A., Islam, M. K., Rahman, M. M., and Jahan, F. (2021). An effective feature extraction method for rice leaf disease classification. Telkomnika 19, 463–470. doi: 10.12928/telkomnika.v19i2.16488
Bandara, D., and Mayurathan, B. (2021). Detection and classification of rice plant diseases using image processing techniques. International Conference on Advanced Research in Computing (ICARC-2021).
Bari, B. S., Islam, M. N., Rashid, M., Hasan, M. J., Razman, M. A. M., Musa, R. M., et al. (2021). A real-time approach of diagnosing rice leaf disease using deep learning-based faster R-CNN framework. PeerJ Comput. Sci. 7, 1–27. doi: 10.7717/PEERJ-CS.432
Basit, A., and Ali, Z. (2020). Detection of disease onset in Rice Plant leaves in monochrome light. Nucleus 57, 100–105.
Bellapu, R. P., Tirumala, R., and Kurukundu, R. N. (2021). Evaluation of homogeneous and heterogeneous distributed ensemble feature selection approaches for classification of Rice Plant diseases. 2021 5th international conference on intelligent computing and control systems (ICICCS).
Bharanidharan, N., Chakravarthy, S. S., Rajaguru, H., Kumar, V. V., Mahesh, T. R., and Guluwadi, S. (2023). Multiclass Paddy disease detection using filter based feature transformation technique. IEEE Access. V. K. Shrivastava, M. K. Pradhan, and M. P. Thakur, Neural Networks for Rice Plant Disease Classification,” in International Conference on Artificial Intelligence and Smart Systems (ICAIS-2021).
Chaudhary, P., Chaudhari, A. K., Cheeran, A. N., and Godara, S. (2012). Colour transform based approach for disease spot detection on plant leaf. Int. J. Comput. Commun. Control 3, 65–70.
Chen, J., Zhang, D., Zeb, A., and Nanehkaran, Y. A. (2021). Identification of rice plant diseases using lightweight attention networks. Expert Syst. Appl. 169:114514. doi: 10.1016/j.eswa.2020.114514
Chiplunkar, N. N., and Padmanabha, V. (2021). “Identification and classification of Paddy crop diseases using big data machine learning techniques” in Data science and data analytics: Opportunities and challenges. ed. A. K. Tyagi (New York: Taylor & Francis).
Chumthong, A., Wiwattanapatapee, R., Viernstein, H., Pengnoo, A., and Kanjanamaneesathian, M. (2016). Spray-dried powder of Bacillus megaterium for control of rice sheath blight disease: formulation protocol and efficacy testing in laboratory and greenhouse. Cereal Res. Commun. 44, 131–140. doi: 10.1556/0806.43.2015.034
Cubero, S., Lee, W. S., Aleixos, N., Albert, F., and Blasco, J. (2016). Automated systems based on machine vision for inspecting citrus fruits from the field to postharvest—a review. Food Bioprocess Technol. 9, 1623–1639. doi: 10.1007/s11947-016-1767-1
Daniya, T., and Vigneshwari, S. J. T. C. J. (2022). Deep neural network for disease detection in rice plant using the texture and deep features. Comput. J. 65, 1812–1825. doi: 10.1093/comjnl/bxab022
Daniya, T., and Vigneshwari, S. (2023). Rice plant leaf disease detection and classification using optimization enabled deep learning. J. Environ. Inf. 42, 25–38. doi: 10.3808/jei.202300492
Dhiman, P. (2014). Empirical validation of website quality using statistical and machine learning methods. 2014 5th international conference-confluence the next generation information technology Summit (confluence).
Dhiman, P., Kaur, A., Balasaraswathi, V. R., Gulzar, Y., Alwan, A. A., and Hamid, Y. (2023b). Image acquisition, preprocessing and classification of citrus fruit diseases: a systematic literature review. Sustain. For. 15:9643. doi: 10.3390/su15129643
Dhiman, P., Kaur, A., and Kukreja, V. (2023a). Citrus fruit disease detection techniques: a survey and comparative analysis of relevant approaches. Int. J. Comput. Digit. Syst. 14, 10127–10148. doi: 10.12785/ijcds/140187
Ding, Z., Li, H., Zhou, D., Liu, Y., and Hou, R. (2023). Multi-spectral colour vision fusion jointly with two-stream feature interaction and colour transformation network. Digit. Signal Process. 133:103875. doi: 10.1016/j.dsp.2022.103875
Ding, B., Zhang, L., He, Y., and Qin, J. (2023). 3D shape classification based on global and local features extraction with collaborative learning. Vis. Comput. 40, 4539–4551. doi: 10.1007/s00371-023-03098-0
Faizal Azizi, M. M., and Lau, H. Y. (2022). Advanced diagnostic approaches developed for the global menace of rice diseases: a review. Can. J. Plant Pathol. 44, 627–651. doi: 10.1080/07060661.2022.2053588
Food Summit, F. A. O. (2009). Declaration of the world summit on food security. Rome: Food and Agriculture Organization.
Ganatra, N., and Patel, A. (2020). A multiclass plant leaf disease detection using image processing and machine learning techniques. Int. J. Emerg. Eng. Res. Technol. 11, 1082–1086.
Gayathri Devi, T., and Neelamegam, P. J. C. C. (2019). Image processing based rice plant leaves diseases in Thanjavur, Tamilnadu. Cluster Comput. 22, 13415–13428. doi: 10.1007/s10586-018-1949-x
Ghosal, S., and Sarkar, K. (2020), Rice leaf diseases classification using CNN with transfer learning. 2020 IEEE Calcutta Conference (Calcon).
Ghyar, B. S., and Birajdar, G. K. (2017). Computer vision based approach to detect rice leaf diseases using texture and colour descriptors. 2017 international conference on inventive computing and informatics (ICICI).
Goluguri, N. R. R., Devi, K. S., and Srinivasan, P. (2021). Rice-net: an efficient artificial fish swarm optimization applied deep convolutional neural network model for identifying the Oryza sativa diseases. Neural Comput. & Applic. 33, 5869–5884. doi: 10.1007/s00521-020-05364-x
Gulzar, Y. (2024). Enhancing soybean classification with modified inception model: a transfer learning approach. Emir. J. Food Agric. 36, 1–9. doi: 10.3897/ejfa.2024.122928
Gulzar, Y., Alwan, A. A., Abdullah, R. M., Abualkishik, A. Z., and Oumrani, M. (2023). OCA: ordered clustering-based algorithm for E-commerce recommendation system. Sustain. For. 15:2947. doi: 10.3390/su15042947
Haridasan, A., Thomas, J., and Raj, E. D. (2023). Deep learning system for paddy plant disease detection and classification. Environ. Monit. Assess. 195:120. doi: 10.1007/s10661-022-10656-x
Hasan, M. J., Mahbub, S., Alom, M. S., and Nasim, M. A. (2019). Rice disease identification and classification by integrating support vector machine with deep convolutional neural network. 2019 1st international conference on advances in science, engineering and robotics technology (ICASERT).
Huang, X., Chen, A., Zhou, G., Zhang, X., Wang, J., Peng, N., et al. (2023). Tomato leaf disease detection system based on FC-SNDPN. Multimed. Tools Appl. 82, 2121–2144. doi: 10.1007/s11042-021-11790-3
Islam, A., Islam, R., Haque, S. R., Islam, S. M., and Khan, M. A. I. (2021). Rice leaf disease recognition using local threshold based segmentation and deep CNN. Int. J. Intell. Syst. Appl 13, 35–45. doi: 10.5815/ijisa.2021.05.04
Islam, S. T., and Mazumder, B. (2019). Wavelet based feature extraction for rice plant disease detection and classification. 2019 3rd international conference on electrical, Computer & Telecommunication Engineering (ICECTE).
Islam, T., Sah, M., Baral, S., and Choudhury, R. R. (2018). A faster technique on rice disease detectionusing image processing of affected area in agro-field. 2018 second international conference on inventive communication and computational technologies (ICICCT).
Jiang, Z., Dong, Z., Jiang, W., and Yang, Y. (2021). Recognition of rice leaf diseases and wheat leaf diseases based on multi-task deep transfer learning. Comput. Electron. Agric. 186:106184. doi: 10.1016/j.compag.2021.106184
Jiang, F., Lu, Y., Chen, Y., Cai, D., and Li, G. (2020). Image recognition of four rice leaf diseases based on deep learning and support vector machine. Comput. Electron. Agric. 179:105824. doi: 10.1016/j.compag.2020.105824
Jordan, M. I., and Mitchell, T. M. (2015). Machine learning: trends, perspectives, and prospects. Science 349, 255–260. doi: 10.1126/science.aaa8415
Joshi, A. A., and Jadhav, B. D. (2016). Monitoring and controlling rice diseases using image processing techniques. 2016 international conference on computing, analytics and security trends (CAST).
Khairnar, K., and Dagade, R. (2014). Disease detection and diagnosis on plant using image processing—a review. Int. J. Comput. Appl. 108, 36–38. doi: 10.5120/18973-0445
Khan, F., Ayoub, S., Gulzar, Y., Majid, M., Reegu, F. A., Mir, M. S., et al. (2023). MRI-based effective ensemble frameworks for predicting human brain tumor. J. Imaging 9:163. doi: 10.3390/jimaging9080163
Kirtphaiboon, S., Humphries, U., Khan, A., and Yusuf, A. (2021). Model of rice blast disease under tropical climate conditions. Chaos, Solitons Fractals 143:110530. doi: 10.1016/j.chaos.2020.110530
Kitpo, N., and Inoue, M. (2018). Early rice disease detection and position mapping system using drone and IoT architecture. 2018 12th south east Asian technical university consortium (SEATUC).
Kukreja, V., and Kadyan, V. (2021). Rice diseases detection using convolutional neural networks: A survey. 2021 International Conference on Advance Computing and Innovative Technologies in Engineering (ICACITE)
Liang, W. J., Zhang, H., Zhang, G. F., and Cao, H. X. (2019). Rice blast disease recognition using a deep convolutional neural network. Sci. Rep. 9:2869. doi: 10.1038/s41598-019-38966-0
Liu, Z. Y., Shi, J. J., Zhang, L. W., and Huang, J. F. (2010). Discrimination of rice panicles by hyperspectral reflectance data based on principal component analysis and support vector classification. J Zhejiang Univ Sci B 11, 71–78. doi: 10.1631/jzus.B0900193
Lu, Y., Tao, X., Jiang, F., Du, J., Li, G., and Liu, Y. (2023). Image recognition of rice leaf diseases using atrous convolutional neural network and improved transfer learning algorithm. Multimed. Tools Appl. 83, 12799–12817. doi: 10.1007/s11042-023-16047-9
Lu, Y., Yi, S., Zeng, N., Liu, Y., and Zhang, Y. (2017). Identification of rice diseases using deep convolutional neural networks. Neurocomputing 267, 378–384. doi: 10.1016/j.neucom.2017.06.023
Malik, I., Ahmed, M., Gulzar, Y., Baba, S. H., Mir, M. S., Soomro, A. B., et al. (2023). Estimation of the extent of the vulnerability of agriculture to climate change using analytical and deep-learning methods: a case study in Jammu, Kashmir, and Ladakh. Sustain. For. 15:11465. doi: 10.3390/su151411465
Mehmood, A., Gulzar, Y., Ilyas, Q. M., Jabbari, A., Ahmad, M., and Iqbal, S. (2023). SBXception: a shallower and broader Xception architecture for efficient classification of skin lesions. Cancers 15:3604. doi: 10.3390/cancers15143604
Mekha, P., and Teeyasuksaet, N. (2021). Image classification of rice leaf diseases using random forest algorithm. 2021 joint international conference on digital arts, media and technology with ECTI northern section conference on electrical, electronics, computer and telecommunication engineering.
Mique, E. L., and Palaoag, T. D. (2018). Rice pest and disease detection using convolutional neural network. Proceedings of the 1st international conference on information science and systems.
Nayak, A., Chakraborty, S., and Swain, D. K. (2023). Application of smartphone-image processing and transfer learning for rice disease and nutrient deficiency detection. Smart Agr. Technol. 4:100195. doi: 10.1016/j.atech.2023.100195
Nuttakarn, K., and Inoue, M. (2018). Early Rice disease detection and position mapping system using drone and IoT architecture Nuttakarn. 12th south east Asian technical university consortium Sysmposium (SEATUC), Yogyakarta, Indonesia Early.
Pan, J., Wang, T., and Wu, Q. (2023). RiceNet: a two stage machine learning method for rice disease identification. Biosyst. Eng. 225, 25–40. doi: 10.1016/j.biosystemseng.2022.11.007
Patil, R. R., and Kumar, S. (2022). Rice transformer: a novel integrated management system for controlling Rice diseases. IEEE Access 10, 87698–87714. doi: 10.1109/ACCESS.2022.3200688
Phadikar, S., Sil, J., and Das, A. K. (2012). Classification of rice leaf diseases based on morphological changes. IJIEEB 2, 460–463. doi: 10.7763/IJIEE.2012.V2.137
Phadikar, S., Sil, J., and Das, A. K. (2013). Rice diseases classification using feature selection and rule generation techniques. Comput. Electron. Agric. 90, 76–85. doi: 10.1016/j.compag.2012.11.001
Pothen, M. E., and Pai, M. L. (2020). Detection of rice leaf diseases using image processing. 2020 fourth international conference on computing methodologies and communication (ICCMC).
Prajapati, H. B., Shah, J. P., and Dabhi, V. K. (2017). Detection and classification of rice plant diseases. Intell. Decis. Technol. 11, 357–373. doi: 10.3233/IDT-170301
Prashar, V., Sood, D., and Kumar, S. (2022). An efficient recognition and classification system for Paddy leaf disease using Naïve Bayes with optimization algorithm. The international conference on recent innovations in computing.
Rafeed Rahman, C., Saha Arko, P., Eunus Ali, M., Khan, M. A. I., Hasan Apon, S., Nowrin, F., et al. (2018). Identification and recognition of Rice diseases and pests using convolutional neural networks. Biosys. Eng. 194, 112–120. doi: 10.1016/j.biosystemseng.2020.03.020
Ramesh, S., and Vydeki, D. (2018). Rice blast disease detection and classification using machine learning algorithm. 2018 2nd international conference on Micro-electronics and telecommunication engineering (ICMETE).
Ramesh, S., and Vydeki, D. (2019). Application of machine learning in detection of blast disease in south Indian rice crops. J. Phytology 11, 31–37.
Ramesh, S., and Vydeki, D. (2020). Rice disease detection and classification using deep neural network algorithm. Micro-electronics and telecommunication engineering: Proceedings of 3rd ICMETE 2019.
Rumy, S. S. H., Hossain, M. I. A., Jahan, F., and Tanvin, T. (2021). An IoT based system with edge intelligence for rice leaf disease detection using machine learning. 2021 IEEE international IOT, electronics and mechatronics conference (IEMTRONICS).
Saha, S., and Ahsan, S. M. M. (2021), Rice disease detection using intensity moments and random forest. 2021 international conference on information and communication Technology for Sustainable Development (ICICT4SD).
Salaba, A., and Chan, L. M. (2023). Cataloging and classification: An introduction. Lanham: Rowman & Littlefield.
Sankareshwaran, S. P., Jayaraman, G., Muthukumar, P., and Krishnan, A. (2023). Optimizing rice plant disease detection with crossover boosted artificial hummingbird algorithm based AX-RetinaNet. Environ. Monit. Assess. 195:1070. doi: 10.1007/s10661-023-11612-z
Sanyal, P., and Patel, S. C. (2008). Pattern recognition method to detect two diseases in rice plants. J. Imaging Sci. 56, 319–325. doi: 10.1179/174313108X319397
Sethy, P. K., Barpanda, N. K., Rath, A. K., and Behera, S. K. (2020a). Deep feature based rice leaf disease identification using support vector machine. Comput. Electron. Agric. 175:105527. doi: 10.1016/j.compag.2020.105527
Sethy, P. K., Barpanda, N. K., Rath, A. K., and Behera, S. K. (2020b). Image processing techniques for diagnosing rice plant disease: a survey. Procedia Comput. Sci. 167, 516–530. doi: 10.1016/j.procs.2020.03.308
Sharma, R., Kukreja, V., and Kadyan, V. (2021). Hispa rice disease classification using convolutional neural network. 2021 3rd international conference on signal processing and communication (ICPSC).
Shrivastava, V. K., and Pradhan, M. K. (2021). Rice plant disease classification using colour features: a machine learning paradigm. J. Plant Pathol. 103, 17–26. doi: 10.1007/s42161-020-00683-3
Shrivastava, V. K., Pradhan, M. K., Minz, S., and Thakur, M. P. (2019). Rice plant disease classification using transfer learning of deep convolution neural network. Int. Arch. Photogramm. Remote. Sens. Spat. Inf. Sci. 42, 631–635. doi: 10.5194/isprs-archives-XLII-3-W6-631-2019
Shrivastava, V. K., Pradhan, M. K., and Thakur, M. P. (2021). Application of pre-trained deep convolutional neural networks for rice plant disease classification. 2021 international conference on artificial intelligence and smart systems (ICAIS).
Simhadri, C. G., and Kondaveeti, H. K. (2023). Automatic recognition of Rice leaf diseases using transfer learning. Agronomy 13:961. doi: 10.3390/agronomy13040961
Singh, P. K., Nag, A., Arya, P., Kapoor, R., Singh, A., Jaswal, R., et al. (2018). Prospects of understanding the molecular biology of disease resistance in rice. Int. J. Mol. Sci. 19:1141. doi: 10.3390/ijms19041141
Singh, V., Sharma, N., and Singh, S. (2020). A review of imaging techniques for plant disease detection. Artif. Intell. Agric. 4, 229–242. doi: 10.1016/j.aiia.2020.10.002
Singh, A. K., Sreenivasu, S. V. N., Mahalaxmi, U. S. B. K., Sharma, H., Patil, D. D., and Asenso, E. (2022). Hybrid feature-based disease detection in plant leaf using convolutional neural network, bayesian optimized SVM, and random forest classifier. J. Food Qual. 2022, 1–16. doi: 10.1155/2022/2845320
Singla, S., Garg, S., Garg, I., Jha, T. K., Singh, B., and Arya, H. (2023). Disease detection in Bombyx Mori silkworm using deep learning algorithm CNN. 2023 international conference on Advanced Computing & Communication Technologies (ICACCTech).
Snilstveit, B., Oliver, S., and Vojtkova, M. (2012). Narrative approaches to systematic review and synthesis of evidence for international development policy and practice. J. Dev. Eff. 4, 409–429. doi: 10.1080/19439342.2012.710641
Stephen, A., Punitha, A., and Chandrasekar, A. (2023). Designing self attention-based ResNet architecture for rice leaf disease classification. Neural Comput. & Applic. 35, 6737–6751. doi: 10.1007/s00521-022-07793-2
Sudhesh, K. M., Sowmya, V., Kurian, S., and Sikha, O. K. (2023). AI based rice leaf disease identification enhanced by dynamic mode decomposition. Eng. Appl. Artif. Intell. 120:105836. doi: 10.1016/j.engappai.2023.10583
Tete, T. N., and Kamlu, S. (2017). Plant disease detection using different algorithms. Proceedings of the Second International Conference on Research in Engineering.
Thakur, P. S., Sheorey, T., and Ojha, A. (2023). VGG-ICNN: a lightweight CNN model for crop disease identification. Multimed. Tools Appl. 82, 497–520. doi: 10.1007/s11042-022-13144-z
Tuncer, A. (2021). Cost-optimized hybrid convolutional neural networks for detection of plant leaf diseases. J. Ambient. Intell. Humaniz. Comput. 12, 8625–8636. doi: 10.1007/s12652-021-03289-4
Ullah, K., Jan, M. A., and Sayyed, A. (2021). Automatic diseases detection and classification in maize crop using convolution neural network. Int. J. Adv. Trends Comput. Sci. Eng. 10, 675–679. doi: 10.30534/ijatcse/2021/301022021
Verma, S., Kumar, P., and Singh, J. P. (2023). A unified lightweight CNN-based model for disease detection and identification in corn, Rice, and wheat. IETE J. Res., 70, 1–12. doi: 10.1080/03772063.2023.2251445
Verma, G., Taluja, C., and Saxena, A. K. (2019). Vision based detection and classification of disease on rice crops using convolutional neural network. 2019 international conference on cutting-edge Technologies in Engineering (ICon-CuTE).
Wang, Y., Wang, H., and Peng, Z. (2021). Rice diseases detection and classification using attention based neural network and bayesian optimization. Expert Syst. Appl. 178:114770. doi: 10.1016/j.eswa.2021.114770
Wang, X., Wang, Y., Zhao, J., and Niu, J. (2023). ECA-ConvNeXt: a Rice leaf disease identification model based on ConvNeXt. Proceedings of the IEEE/CVF conference on computer vision and pattern recognition.
Wani, J. A., Sharma, S., Muzamil, M., Ahmed, S., Sharma, S., and Singh, S. (2022). Machine learning and deep learning based computational techniques in automatic agricultural diseases detection: methodologies, applications, and challenges. Arch. Comput. Method Eng. 29, 641–677. doi: 10.1007/s11831-021-09588-5
Wen, J., Li, S., Lin, Z., Hu, Y., and Huang, C. (2012). Systematic literature review of machine learning based software development effort estimation models. Inf. Softw. Technol. 54, 41–59. doi: 10.1016/j.infsof.2011.09.002
Zhang, S., Wu, G., Gu, J., and Han, J. (2020). Pruning convolutional neural networks with an attention mechanism for remote sensing image classification. Electronics 9:1209. doi: 10.3390/electronics9081209
Keywords: rice disease, Oryza sativa L, review, pre-processing, systematic, recognition, deep learning, image classification
Citation: Seelwal P, Dhiman P, Gulzar Y, Kaur A, Wadhwa S and Onn CW (2024) A systematic review of deep learning applications for rice disease diagnosis: current trends and future directions. Front. Comput. Sci. 6:1452961. doi: 10.3389/fcomp.2024.1452961
Received: 21 June 2024; Accepted: 19 August 2024;
Published: 11 September 2024.
Edited by:
Nitin Goyal, Central University of Haryana, IndiaReviewed by:
Hariharan Shanmugasundaram, Vardhaman College of Engineering, IndiaCopyright © 2024 Seelwal, Dhiman, Gulzar, Kaur, Wadhwa and Onn. This is an open-access article distributed under the terms of the Creative Commons Attribution License (CC BY). The use, distribution or reproduction in other forums is permitted, provided the original author(s) and the copyright owner(s) are credited and that the original publication in this journal is cited, in accordance with accepted academic practice. No use, distribution or reproduction is permitted which does not comply with these terms.
*Correspondence: Yonis Gulzar, eWd1bHphckBrZnUuZWR1LnNh
Disclaimer: All claims expressed in this article are solely those of the authors and do not necessarily represent those of their affiliated organizations, or those of the publisher, the editors and the reviewers. Any product that may be evaluated in this article or claim that may be made by its manufacturer is not guaranteed or endorsed by the publisher.
Research integrity at Frontiers
Learn more about the work of our research integrity team to safeguard the quality of each article we publish.