- Department of Linguistics, University of California, Davis, Davis, CA, United States
The current study had two goals: First, we aimed to conduct a conceptual replication and extension of a classic study by Nass et al. in 1997 who found that participants display voice-gender bias when completing a tutoring session with a computer. In the present study, we used a more modern paradigm (i.e., app-based tutoring) and commercially-available TTS voices. Second, we asked whether participants provided different social evaluations of non-native-accented and native-accented American English-speaking machines. In the present study, 85 American participants completed a tutoring session with a system designed to look like a device application (we called it a “TutorBot”). Participants were presented with facts related to two topics: ‘love and relationships’ and ‘computers and technology’. Tutoring was provided either by a female or male TTS voice. Participants heard either native-English accented voices or non-native-English accented (here, Castilian Spanish-accented) voices. Overall, we find no effect of voice gender on any of the dependent measures: listeners recalled facts and rated female and male voices equivalently across topics and conditions. Yet, participants rated non-native accented TTS voices as less competent, less knowledgeable, and less helpful after completing the tutoring session. Finally, when participants were tutored on facts related to ‘love and relationships’, they showed better accuracy at recall and provided higher ratings for app competency, likeability, and helpfulness (and knowledgeable, but only for native-accented voices). These results are relevant for theoretical understandings of human-computer interaction, particularly the extent to which human-based social biases are transferred to machines, as well as for applications to voice-AI system design and usage.
1 Introduction
Voice-enabled Artificial Intelligence (AI) is a rapidly developing technology that allows users to interact with machines through natural spoken language to complete a variety of activities and tasks. The ease and efficiency in using speech to interact with AI to complete everyday tasks explains why people find using functions—such as getting a weather report, searching for information, creating and sending text messages, making shopping lists or calendar events, and playing music or news reports—to be effortlessly integrated into our spoken interactions (De Renesse, 2017; Ammari et al., 2019). Voice-enabled devices are growing in popularity worldwide (Sener, 2023), which means that many more people will be spending a lot of time talking to machines in the future. The huge adoption and daily use of voice-enabled devices by millions of people raises important scientific questions about how people perceive the speech and language generated by these devices (Zellou and Holliday, 2024).
Voice AI can be customized by the user to have different characteristics, such as selecting voices that have different apparent genders and accents (Bilal and Barfield, 2021). The voice characteristics of a voice-enabled device have a significant impact on how the listener perceives and evaluates the machine. For instance, in a classic study investigating this topic, Nass et al. (1997) had participants complete a tutoring session with a computer program. The tutoring was administered via spoken language, with facts and information presented to the listener through the computer with a voice. They manipulated voice gender: participants were tutored by a female-presenting and a male-presenting voice; then, they were tested on their retention of the material, and evaluated the computer based on traits like competence and friendliness. They found that participants’ responses reflected gender bias, based on the voice alone: female-voiced computers were rated as more knowledgeable about “feminine” topics like ‘love and relationships’, whereas male voiced computers were rated as more knowledgeable for “masculine” topics such as “computers and technology” (Nass et al., 1997). Findings like this lead Nass and colleagues to posit that people view computers as social actors and argued that humans unconsciously apply human-based social biases and expectations to computers—even though they know computers do not have feelings, intentions, or human experiences (Nass et al., 1994).
Like gender stereotypes, there are also powerful stereotypes about accents that shape how people evaluate non-native speakers with major societal consequences. For instance, Rubin (2011) investigated how listener expectations affect perception of speech. Linguistic stereotyping is the process of attributing traits to speakers based on pronunciation and reverse linguistic stereotyping is attributing pronunciation characteristics to speakers based on their social identity. Rubin (2011) revealed that native American English-speaking listeners expect non-native speakers to have less intelligible accents than native speakers, and thus give them lower performance ratings or show that they misunderstood them—even when the speech signal is produced by a native speaker. Subsequent work by Rubin and Smith (1990) investigated this further. Rubin and Smith (1990) measured undergraduates’ listening comprehension, teacher ratings, and perceptions of the speaker’s personality and attractiveness for two levels of accentedness—moderate or high, two ethnic identities—Chinese or Caucasian, and two topics—humanities or science. They found that instructor ethnicity and lecture topic were determinants of student attitudes (Rubin and Smith, 1990). When students perceived high levels of foreign accentedness, they judged speakers to be poorer teachers. Similar findings are reported by Rubin and Heintzman (1991) and Rubin (1992).
Since the original Nass et al. (1997) study, technology advancements, like voice recognition and voice assistants, have improved rapidly. These voice AI systems have made spoken interactions with computers more natural, intuitive, and inclusive. Many users utilize voice AI to communicate with machines, learn about new topics, control devices around their home, and even when driving vehicles. While these advancements are helpful and important, they also pose new challenges related to ethics, bias, and inclusion. Present day, the computer tutor would be akin to voice AI tools that search and provide information to users—a common functionality for voice-enabled devices (Ammari et al., 2019). In recent studies, the ethical implications of AI systems and chatbots have been a focal point of inquiry, highlighting their transformative potential in many domains, like education, branding, and e-commerce (Cheng et al., 2021; Kirkby et al., 2023; Kooli, 2023). Kirkby et al. (2023) showed that text disclosed as AI generated is perceived as equally authentic as human-written, suggesting that transparency can enhance consumer trust. Additionally, ethical implications of AI in education necessitate robust guidelines and innovative assessment methods to adapt to the changing technological landscape (Kooli, 2023). Furthermore, consumers’ trust in chatbots is significantly influenced by their perception of its empathy and friendliness (Cheng et al., 2021). These insights underscore the importance of ethical considerations, transparency, and trust in using AI systems effectively.
As mentioned previously, Nass et al. (1997) only investigated gender-based factors and did not look at non-native English accentedness. In addition, due to advancements in AI technology, paired with the changing ways society uses this technology, it is necessary to have a contemporary update of the original study to explore whether gender-differentiated responses continue to be applied to voice AI. Thus, the main question is to what extent do users exhibit gender and non-native English accent bias in responses to voice AI? The aim of the current study is to adapt and extend the work of Nass et al. (1997) by examining how gender and accentedness of modern voice AI affect users’ social evaluations of an app-based tutor. We designed an experiment to present participants with a variety of facts on two topics— “love and relationships” and “computers and technology” —under the guise of an app-based tutor program (we called it a “TutorBot”).
Will the same gender bias found by Nass et al. (1997) be observed in our TutorBot paradigm? On the one hand, we hypothesize that we will validate the original finding. Even though there have been huge advancements in the quality of voice-enabled technologies available, recent work that has explored gender biases for contemporary voice-AI finds consistent effects as that found by Nass et al. (1997). For instance, Ernst and Herm-Stapelberg (2020) investigated whether gender bias influences the perceived likability of modern-day virtual voice assistants. They had participants interact with a virtual assistant, assigned either to a female-voiced condition or a male-voiced condition. Post-interaction surveys revealed that participants who interacted with the female-voiced assistant rated the system as more likable, yet less competent, than the male-voiced assistant. On the other hand, recent research on gender bias in voice assistants raises awareness about harmful unconscious stereotypes in technologies, and how they could be mitigated through workshops (Schumacher, 2022). In her study, Schumacher (2022) completed a trial workshop with Computer Science students aimed to expose them to different gendered AI voices, including a genderless voice, and challenge them to reflect on their own prejudices. The findings of her workshop reveal that this is an effective way to make students more aware and conscious of their own gender biases when using computers. Overall, there is evidence from previous research that gender bias can extend to voice AI, but it is uncertain how changing technologies and possibly changing gender norms would affect the results.
We also ask whether participants will provide different social evaluations of non-native-accented and native-accented machines. Do people’s accent bias apply to machines as well? We hypothesize that we will find lower evaluations for non-native-accented speech, consistent with the original Rubin studies (e.g., Rubin and Smith, 1990; Rubin and Heintzman, 1991; Rubin, 1992). This would indicate that non-native-accent-based biases will transfer to machines. There is some more recent research that looks at accent bias and Intelligent Virtual Agents (IVAs) (Obremski et al., 2022), but this is still a relatively understudied topic. For instance, Obremski et al. (2022) had native English speakers watch a video of an IVA with either a Spanish, Hindi, or Mandarin accent and it was either natural speech or synthetically generated. They found that there was a significant impact of natural speech on the perceived warmth of the IVAs, and a significant interaction of accent and naturalness on perceived competence. Overall, then, there is some evidence that people are biased against accented speech and that this bias extends to speech-enabled devices. Thus, we predict we will find similar results in the present study.
2 Methodology
The design of the present experiment, which was roughly based on the Nass et al. (1997) experiment, involves participants completing a tutoring session with a machine, under the guise of a “TutorBot” application, that produces facts via spoken language. Participants are then assessed on their retention of facts from the session as well as asked to provide various social evaluations of the tutoring application.
2.1 Stimuli: materials and recordings
The original Nass et al. (1997) paper does not provide all of the facts they presented to participants, and the ones that are presented are somewhat outdated (e.g., “The more wire a computer has, the more slowly it runs.”). Therefore, we constructed 15 statements to reflect contemporary facts related to each of the topics ‘love and relationships’ and ‘computers and technology’. The statements were generated from trusted news and information sources on the internet (such as Encyclopedia Britannica, NPR, and Pew Research). One example of a fact from the ‘love and relationships’ topic is “Eye contact can enhance empathy, trust, and social connection.” One example of a fact from the ‘computers and technology’ topic is “Keyboards work by closing an open circuit every time a key is pressed, which allows a tiny amount of electrical current to travel through.”
To make the auditory stimuli, a total of 4 “TutorBot” voices were generated using Amazon Polly, Amazon’s text-to-speech service. We selected one Native Female (US-Salli), Native Male (US-Matthew), Non-Native Female (SPA-Lucia), and Non-Native Male (SPA-Sergio) voice. The native voices were standard American English because the study was completed in America, and the non-native accented voices were Castilian Spanish. We generated audio of each of the facts on the topic ‘love and relationships’ and the same facts on the topic ‘computers and technology’ in each of the voices. After each file was downloaded from Amazon Polly, a.wav file was created in Praat for each audio clip, 30 sound files per voice. All sound files were amplitude-normalized to 65 dB.
2.2 Participants and procedure
Participants were 85 undergraduate students from the University of California, Davis (61 female, 0 non-binary/gender non-conforming, 24 male; mean age = 19.6 years old). All participants were recruited through SONA, and all reported that they have no known hearing difficulty and were native speakers of American English. This study was approved by the UC Davis Institutional Review Board and all participants completed informed consent.
The experiment was conducted online through a Qualtrics survey, where participants were told to complete it in a quiet room with no distractions. The study began with a pre-test of their audio: participants heard one sentence presented auditorily (“She asked about the host”) and were asked to identify the sentence from three multiple choice options, each containing a phonologically close target word (host, toast, coast). All participants passed this audio check.
In the experiment, each participant completed two complete blocks that consisted of three tasks in each: a tutoring session, a test phase, and a ratings task.
In the tutoring session task, participants completed two tutoring sessions, one on each topic described above, with a testing session after each topic. In each tutoring session, participants listened to 10 different facts, each played one time, with the TutorBot audio. There was text on the screen of what the audio said.
After the tutoring phase, participants completed the test phase by identifying whether they had heard each of the 10 statements in the tutoring session or not. Of the 10 statements, 5 had indeed been presented to the participants in the tutoring session (a random selection of the original 10 tutoring statements heard) and 5 had not been presented to the participants in the tutoring session. Each question on the test presented a fact written out (no audio) and asked participants to respond whether or not they heard that fact from the TutorBot in the tutoring session. Participants selected “yes” if they believed they heard the fact in the tutoring session before the test, and “no” if they believed they did not hear the fact from the TutorBot from the previous block.
Finally, participants completed the ratings task where they filled out a questionnaire in which they assessed the TutorBot’s competence, knowledge, helpfulness, and likability on a scale from 0 to 100.
Each participant completed two blocks where one voice and one topic was presented only. Across participants, the order of the two topics was counterbalanced, so half of the participants completed the ‘love and relationships’ block first, then the ‘computers and technology’ block second, and the other half were presented the reverse order of blocks.
This experiment design was a 2 (TutorBot voice: female, male) x 2 (TutorBot accent: native American English accented, non-native American English accented) x 2 (topic: computers and technology, love and relationships) mixed design. Both Gender and Topic were within-subjects variables: all participants heard both topics and one male and one female voice, counterbalanced in topic-assignment across listeners. Nativeness was a between-subjects factor: participants were assigned either to the native-accented voices condition or they were assigned to non-native accented voices condition.
3 Results
3.1 Accuracy results
Responses to the recall task were coded for whether the participant correctly identified hearing a fact from tutoring/correctly identified unheard facts (=1) or not (=0). The effects of gender, accent, and topic on statement recall were modeled using a generalized linear mixed model and p-values were calculated using the lmer() function in the lme4 package in R. The model included fixed effects of Gender (Female, Male), Accent (Native, Non-Native), and Topic (Love and Relationships, Computers and Technology). Effects were sum-coded. All two-and three-way interactions between gender, accent, and topic were also included. The model also included by-participant random intercepts and by-participant random slopes for gender and topic.
Table 1 shows the output of the statistical model for accuracy and Figure 1 shows the aggregated results. The model revealed a significant effect for Topic: participants were more accurate at remembering the facts they heard or did not hear for the topic ‘love and relationships’ compared to the topic “computers and technology”.
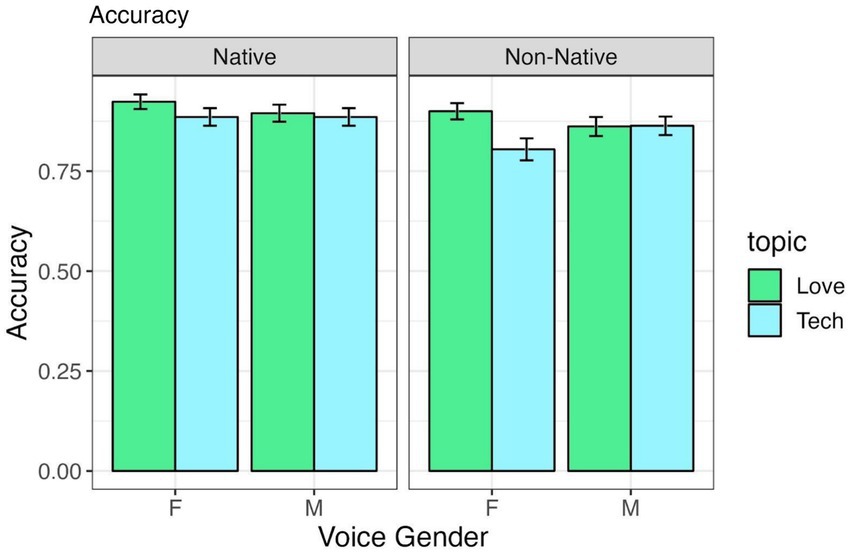
Figure 1. Accuracy in identifying statements heard in the tutoring session, by voice gender and accentedness, across “Love and Relationship” and “Computer and Technology” topics.
Of note, the lack of a significant effect for Accent indicates that listeners in the present study were not overall worse at remembering facts heard in a non-native accent (cf. Rubin, 1992). No other effects or interactions were significant.
3.2 Ratings results
3.2.1 Statistical analysis: ratings
The effects of voice gender, voice accent, and topic on competence, knowledgeable, likable, and helpfulness ratings were modeled using separate linear mixed effects models for each rating. The t and p-values were calculated using the lmer() function in the lme4 package in R (Bates et al., 2014). Each model included fixed effects of Gender (Female, Male), Accent (Native, Non-Native), and Topic (Love and Relationships, Computers and Technology). All two-and three-way interactions between gender, accent, and topic were also included in each model. Effects were sum-coded. The models also included by-participant random intercepts and by-participant random slopes for gender and topic.
3.2.2 Competence ratings
Table 2 shows the output for the statistical model for ratings on competence, and means are provided in Figure 2. There is an effect of accentedness, whereby native accents are rated as more competent than non-native accents. There is also an effect of the topic, which reveals that participants found the TutorBots to be more competent when teaching about love and relationships than computers and technology. No other effects or interactions were significant for the competence model.
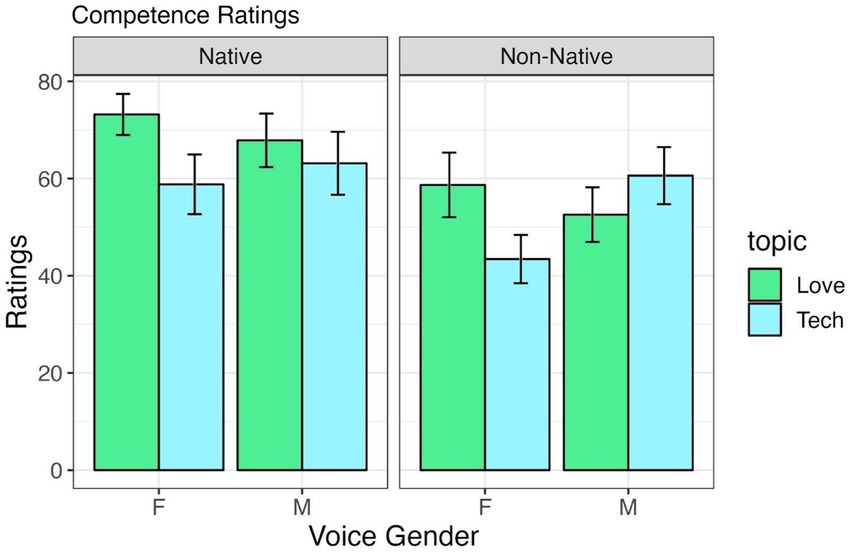
Figure 2. Competence ratings by voice gender and accentedness, across “Love and Relationship” and “Computer and Technology” tutoring conditions.
3.2.3 Knowledgeable ratings
Figure 3 provides the means for ratings on how ‘knowledgeable’ the TutorBots were across conditions and Table 3 presents the output for the statistical model. There is an effect of accentedness: native accents are rated as more knowledgeable than non-native accents. In addition, there is an interaction between accentedness and the topic, revealing that the TutorBots were rated as even more knowledgeable when they had a native American English accent and were tutoring the topic “love and relationships.” No other effects or interactions were significant.
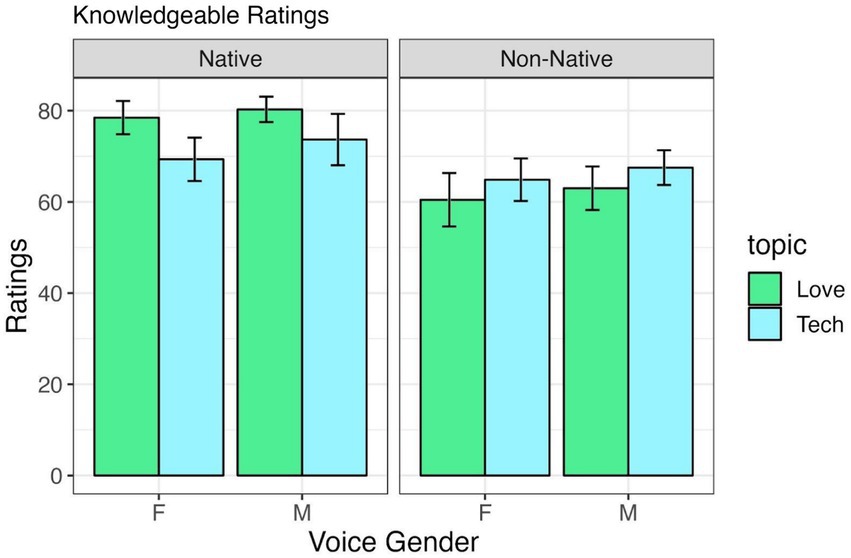
Figure 3. Knowledgeable ratings by voice gender and accentedness, across “Love and Relationship” and “Computer and Technology” tutoring conditions.
3.2.4 Likable ratings
Table 4 shows the output for the statistical model for ratings on how ‘likable’ the TutorBots were and Figure 4 presents the aggregated data. There was only an effect for the topic ‘love and relationships’, showing that the TutorBots were rated more likable when teaching this topic, regardless of the voice. No other effects or interactions were significant.
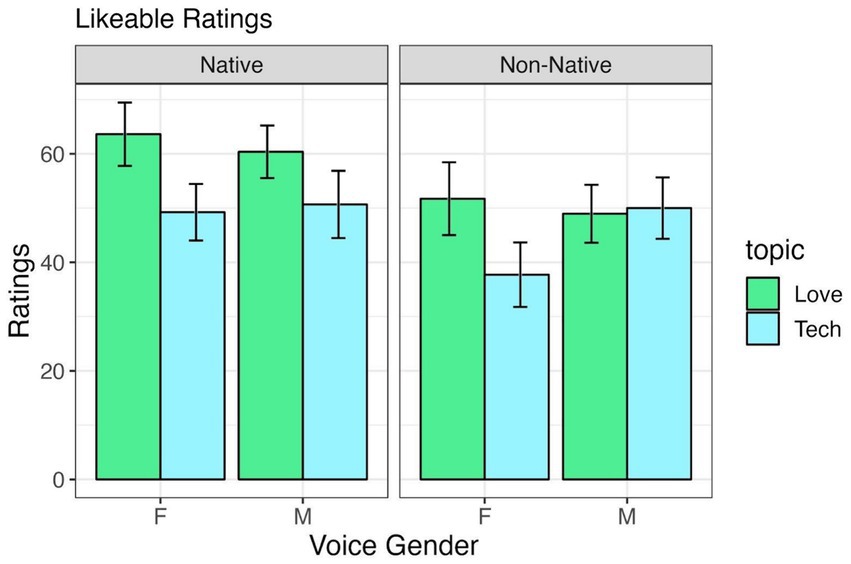
Figure 4. Likable ratings by voice gender and accentedness, across “Love and Relationship” and “Computer and Technology” tutoring conditions.
3.2.5 Helpfulness ratings
Table 5 shows the output for the statistical model for ratings on how ‘helpful’ the TutorBots were and the means are provided in Figure 5. Like the competence and knowledgeable results, we find an effect for accent, whereby native English accent TutorBots were rated significantly more helpful. In addition, similarly to the likable ratings, there is an effect for the topic ‘love and relationships’, meaning the TutorBots were rated most helpful when teaching this topic, compared to the topic ‘computers and technology’. While not statistically significant, we do see a hint towards the Nass study results in the effect for Female sounding voice and the topic love and relationships, but overall, we are not finding the results are corroborated. No other effects or interactions were significant.
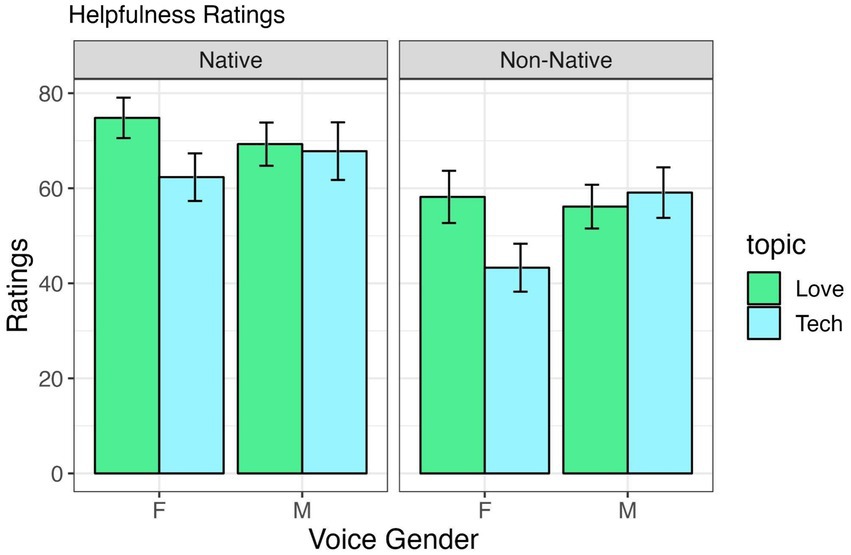
Figure 5. Helpfulness ratings by voice gender and accentedness, across “Love and Relationship” and “Computer and Technology” tutoring conditions.
4 General discussion
The goal of the present work was twofold: first, to revisit the classic Nass et al. (1997) study looking at voice-gender bias when completing a tutoring session with a computer using a more contemporary task (app-based tutoring) and more modern TTS voices and, second, extend this line of work to non-native-accented (American English) speech. There are three key findings from the current study. First, we find no effect of voice gender on any of the dependent measures: listeners identified information from, and rated female and male voices equivalently overall and across topics and conditions. Secondly, participants rated non-native English accented (here: Castilian Spanish-accented) TTS voices as less competent, less knowledgeable, and less helpful after completing the tutoring session with the app. Finally, when participants were tutored on facts related to Love and Relationships, they showed better accuracy at recall and provided higher ratings for app competency, likeability, and helpfulness (and knowledgeable, but only for native-accented voices). We discuss each of these key findings in turn below, with respect to theoretical understandings of human-computer interaction, as well as applications to voice-AI system design.
4.1 Theoretical implications
Our observation that voice gender does not affect ratings of the TutorBot is contrary to several past studies that do find such an effect. As outlined in the Introduction, the original Nass et al. (1997) study reported that male-voiced computers are rated as more competent tutors on topics related to technology, while female-voiced computers are rated as more competent tutors on love-and-relationship topics. Why do we not find an interaction between gender and topic in the present study? This could reflect changing gender norms in the past 2+ decades; there are some indications that the way society views gender roles is evolving, for instance, as more women enter the workforce and (Pessin, 2018). Based on the large society shifts with respect to how society uses computers and indications that there have been changes in gender biases, we might have predicted a modulation of the original Nass et al. finding. However, the effect of voice gender on evaluation of machines has been observed in recent work. For instance, Ernst and Herm-Stapelberg (2020) found that users perceive male voice assistants as more competent than female voice assistants, yet female voice assistants are rated as more likable. Other researchers have also shown in recent work that participants do display distinctly gender-mediated patterns for other types of behavior (e.g., vocal shadowing in Zellou et al., 2021; Cohn et al., 2023, and gender-typicality of a task in Kuchenbrandt et al., 2014). Yet, the current study does not find an effect of voice gender on overall social evaluations. It is critical to note, then, that voice gender effects are not categorically observed across studies and it remains an open question of when and under what conditions voice gender does influence how people behave towards machines.
While we failed to observe the original gender bias effect, we extended the role of social factors influencing interactions with voice-AI to speaker accent. For human-human interactions, prior work has shown that listeners show distinct biases when interacting with someone who they believe to be a non-native speaker of the language (Rubin, 1992; McGowan, 2015). From a voice alone, listeners categorize a talker as a native or non-native speaker (Girard et al., 2008). With respect to learning specifically, many studies report a bias against “non-native speaker” teachers (Holliday, 2006; Llurda, 2005; Mahboob and Golden, 2013), even if recent work shows that “nativeness” was not the most important factor contributing to teaching effectiveness (Kiczkowiak, 2019). Our results indicate that social biases against non-native accented speakers is also applied to machine agents. In an era where generative voice technology is improving, there is push from society and companies towards diversity of voice (see discussion in Zellou and Holliday, 2024). With the customizability that modern technology affords, and a growing interest in users changing their default voice settings to be one with a non-local accent (Bilal and Barfield, 2021), understanding the role that accentedness has on evaluations of voice-AI is of growing importance.
Third, there is evidence for an effect of the topic ‘love and relationships’. Participants were more accurate at remembering the facts they heard or did not hear for this topic compared to facts from ‘computers and technology’, and they rated the TutorBot as more competent, knowledgeable, likable, and helpful when they taught the topic ‘love and relationships’ than ‘computers and technology’. These results show that there was an affinity towards this topic. One reason for this, perhaps, is because of the way voice AI is seen today-as entertainment rather than as a tool to learn about technical topics. There could be misalignment in users’ expectations and the topic ‘computers and technology’ because they were expecting to use voice AI for entertainment and social reasons, and instead were met with an unexpected use. This is also a direction for future work to explore. Lastly, future work could explore using different facts or topics, to see if they yield similar or different results, as there could also be something about the particular facts used in the present study.
Finally, because the present study only used Castilian Spanish as the non-native accent, it remains an open question for future investigation as to whether other non-native English accents are perceived as more competent in one topic over another. We acknowledge that within non-native accents, there are nuanced stereotypes and their interaction with different topics is not explored here. A ripe direction for future work is to explore how other accents are perceived in terms of competence across diverse topics. For example, certain accents may be perceived as more competent in scientific and technological contexts, but less so in artistic domains. In addition, the present study did not control for participants’ second language, so there were participants who spoke Spanish in addition to self-identifying as a native English speaker. Future work could compare performance across participants with different language backgrounds. Because this study recruited participants from a large university in California, it is likely that those who self-reported speaking Spanish spoke some variety of California Spanish. In the future, the potential influence of California Spanish can be explored. This line of inquiry is crucial for understanding broader implications of accent variation, user language experience, and expectations of competence for AI tutors.
4.2 Practical implications and future directions
There are several other avenues for future research on this topic. First, this work can be expanded on to include other accents, such as accents of different levels of prestige, non-local, but native accents, and different American (English) accents. A future study on other accents would be beneficial to understand accent bias further. Second, this work can be expanded to other languages and cultures, not just English. It would be interesting to investigate whether the same patterns are found for different populations of participants. For example, gender stereotypes were not substantiated in this study, but if the population of participants came from another country with different societal gender norms, would participants extend stereotypes to computers? It is unknown if these results would apply to different languages or populations, thus future work is necessary.
Finally, there are implications of this research for voice AI design, specifically, on what designers can do to mitigate bias. This study showed that accent-based stereotypes seen in society are extended to voice AI. On the engineering side, one way to combat this is to intentionally design many voice AI with diverse accents, to expose users to similar diversity that is in society. Additional research is needed so that voice AI can be designed to not perpetuate accent bias, while promoting user adoption. On the user side, users can change the settings of voice assistants and other voice AI applications they use to a non-standard voice to expose themselves to a broader variety of accents.
5 Conclusion
This present study investigated whether the Nass et al. (1997) results that users apply voice-gender biases to computers still hold today, and it also extended it to accent-based biases. We did not observe the previously reported finding of voice gender biases when learning facts via a tutoring voice-AI application. However, we did find an effect of non-native accent. Native-accented TutorBots were rated as more competent, knowledgeable, likable, and helpful, compared to non-native accented TutorBots. This study shows that society’s relationship with technology might be shifting as new technologies are created and also as societal norms and attitudes change and evolve. Thus, designers and users alike must be cognizant of extending real-world biases on to computers. More research must be done to fully analyze the effects of these biases towards marginalized groups.
Data availability statement
The raw data supporting the conclusions of this article will be made available by the authors, without undue reservation.
Ethics statement
The studies involving humans were approved by UC Davis Institutional Review Board. The studies were conducted in accordance with the local legislation and institutional requirements. The participants provided their written informed consent to participate in this study.
Author contributions
AJ: Conceptualization, Formal analysis, Methodology, Visualization, Writing – original draft. GZ: Conceptualization, Methodology, Supervision, Writing – original draft.
Funding
The author(s) declare that no financial support was received for the research, authorship, and/or publication of this article.
Conflict of interest
The authors declare that the research was conducted in the absence of any commercial or financial relationships that could be construed as a potential conflict of interest.
Publisher’s note
All claims expressed in this article are solely those of the authors and do not necessarily represent those of their affiliated organizations, or those of the publisher, the editors and the reviewers. Any product that may be evaluated in this article, or claim that may be made by its manufacturer, is not guaranteed or endorsed by the publisher.
Supplementary material
The Supplementary material for this article can be found online at: https://www.frontiersin.org/articles/10.3389/fcomp.2024.1436341/full#supplementary-material
References
Ammari, T., Kaye, J., Tsai, J. Y., and Bentley, F. (2019). Music, search, and IoT: how people (really) use voice assistants. ACM TOCHI 26, 1–28. doi: 10.1145/3311956
Bates, D., Mächler, M., Bolker, B., and Walker, S. (2014). Fitting linear mixed-effects models using lme4. arXiv preprint arXiv:1406.5823
Bilal, D., and Barfield, J. (2021). Increasing racial and ethnic diversity in the design and use of voice digital assistants. Research Symposium on Sociotechnical Perspectives on Equity, Inclusion, and Justice.
Cheng, X., Bao, Y., Zarifis, A., Gong, W., and Mou, J. (2021). Exploring consumers' response to text-based chatbots in e-commerce: the moderating role of task complexity and chatbot disclosure. Internet Res. 32, 496–517. doi: 10.1108/INTR-08-2020-0460
Cohn, M., Keaton, A., Beskow, J., and Zellou, G. (2023). Vocal accommodation to technology: the role of physical form. Lang. Sci. 99:101567. doi: 10.1016/j.langsci.2023.101567
De Renesse, R. (2017). Virtual digital assistants to overtake world population by 2021. Ovum Media Center.
Ernst, C. P. H., and Herm-Stapelberg, N. (2020). “The Impact of Gender Stereotyping on the Perceived Likability of Virtual Assistants”. AMCIS 2020 Proceedings. 4. Available at: https://aisel.aisnet.org/amcis2020/cognitive_in_is/cognitive_in_is/4
Girard, F., Floccia, C., and Goslin, J. (2008). Perception and awareness of accents in young children. Br. J. Dev. Psychol. 26, 409–433. doi: 10.1348/026151007X251712
Kiczkowiak, M. (2019). Students’, teachers’ and recruiters’ perception of teaching effectiveness and the importance of nativeness in ELT. J. Sec. Lang. Teach. Res. 7, 1–25.
Kirkby, A., Baumgarth, C., and Henseler, J. (2023). To disclose or not disclose, is no longer the question–effect of AI-disclosed brand voice on brand authenticity and attitude. J. Prod. Brand Manag. 32, 1108–1122. doi: 10.1108/JPBM-02-2022-3864
Kooli, C. (2023). Chatbots in education and research: a critical examination of ethical implications and solutions. Sustain. For. 15:5614. doi: 10.3390/su15075614
Kuchenbrandt, D., Häring, M., Eichberg, J., Eyssel, F., and André, E. (2014). Keep an eye on the task! How gender typicality of tasks influence human–robot interactions. Int. J. Soc. Robot. 6, 417–427. doi: 10.1007/s12369-014-0244-0
Llurda, E. (2005). “Non-native TESOL students as seen by practicum supervisors” in Non-native language teachers: Perceptions, challenges and contributions to the profession (Boston, MA: Springer US), 131–154.
Mahboob, A., and Golden, R. (2013). Looking for native speakers of English: discrimination in English language teaching job advertisements. Age 3:21.
McGowan, K. B. (2015). Social expectation improves speech perception in noise. Lang. Speech 58, 502–521. doi: 10.1177/0023830914565191
Nass, C., Moon, Y., and Green, N. (1997). Are machines gender neutral? Gender-stereotypic responses to computers with voices. J. Appl. Soc. Psychol. 27, 864–876.
Nass, C., Steuer, J., and Tauber, E. R. (1994). Computers are social actors. In Proceedings of the SIGCHI conference on human factors in computing systems (pp. 72–78).
Obremski, D., Hering, H. B., Friedrich, P., and Lugrin, B. (2022). Mixed-cultural speech for intelligent virtual agents-the impact of different non-native accents using natural or synthetic speech in the English language. In Proceedings of the 10th international conference on human-agent interaction HAI ‘22. (pp. 67–75).
Pessin, L. (2018). Changing gender norms and marriage dynamics in the United States. J. Marriage Fam. 80, 25–41. doi: 10.1111/jomf.12444
Rubin, D. L. (1992). Nonlanguage factors affecting undergraduates' judgments of nonnative English-speaking teaching assistants. Res. High. Educ. 33, 511–531.
Rubin, D. (2011). The power of prejudice in accent perception: reverse linguistic stereotyping and its impact on listener judgments and decisions. Pronunciation in Second Language Learning and Teaching Proceedings.
Rubin, D., and Heintzman, M. (1991). Effects of accented speech and culture-typical compliance- gaining style on subordinates' impressions of managers. Int. J. Intercult. Relat. 15, 267–283. doi: 10.1016/0147-1767(91)90002-X
Rubin, D. L., and Smith, K. A. (1990). Effects of accent, ethnicity, and lecture topic on undergraduates' perceptions of nonnative English-speaking teaching assistants. Int. J. Intercult. Relat. 14, 337–353. doi: 10.1016/0147-1767(90)90019-S
Schumacher, C. (2022). Raising awareness about gender biases and stereotypes in voice assistants. Linköping University I Department of Computer and Information Science Master Thesis I MSc Design ISRN: LIU-IDA/LITH-EX-A--22/018--SE.
Sener, C. (2023) What the impact of global voice recognition means for Today’s brands. Available at: https://www.forbes.com/sites/forbescommunicationscouncil/2023/07/06/what-the-impact-of-global-voice-recognition-means-for-todays-brands/?sh=3ab5c3c20c2c (Accessed March 29, 2024).
Zellou, G., Cohn, M., and Ferenc Segedin, B. (2021). Age-and gender-related differences in speech alignment toward humans and voice-AI. Front. Commun. 5:600361. doi: 10.3389/fcomm.2020.600361
Keywords: voice gender, accentedness, human-computer interaction, social evaluation, learning
Citation: Jones A and Zellou G (2024) Voice accentedness, but not gender, affects social responses to a computer tutor. Front. Comput. Sci. 6:1436341. doi: 10.3389/fcomp.2024.1436341
Edited by:
Carina Soledad González González, University of La Laguna, SpainReviewed by:
Florian Georg Jentsch, University of Central Florida, United StatesAlex Zarifis, University of Southampton, United Kingdom
Copyright © 2024 Jones and Zellou. This is an open-access article distributed under the terms of the Creative Commons Attribution License (CC BY). The use, distribution or reproduction in other forums is permitted, provided the original author(s) and the copyright owner(s) are credited and that the original publication in this journal is cited, in accordance with accepted academic practice. No use, distribution or reproduction is permitted which does not comply with these terms.
*Correspondence: Allison Jones, YWdqb25lc0B1Y2RhdmlzLmVkdQ==; YWxsaWVqMTQxNEBnbWFpbC5jb20=