- 1Doctoral Program in Design, College of Design, National Taipei University of Technology, Taipei, Taiwan
- 2Fuzhou University, Xiamen, China
- 3Department of Interaction Design, College of Design, National Taipei University of Technology, Taipei, Taiwan
Despite the widespread recognition of artificial intelligence’s advantages, it cannot replace human independent thinking and creativity, especially in fields such as artistic design that require creativity. Previous studies often examined its development trends from the perspective of technical advantages or application processes. This study explores the attitudes and acceptance of creative industry practitioners towards Artificial Intelligence Generated Content (AIGC) from the perspective of user behavior modification. Utilizing the Stimulus-Organism-Response Model (SOR) as the theoretical background, this research integrates the Technology Acceptance Model, Theory of Planned Behavior, and Self-Efficacy to form the research framework. By employing a mixed-method approach combining quantitative and qualitative analyses, data from 226 designers were explored, and structural equation modeling was used to verify the correlations between endogenous factors. The results indicate that users’ facilitating conditions significantly influence self-efficacy, which in turn determines their intention to adopt AIGC. Additionally, semi-structured interviews revealed that factors hindering the widespread application of AIGC mainly encompass legal security, ethical risks, and fairness. This study extends the application scope of the Stimulus-Organism-Response Model (SOR), enriches the Technology Acceptance Model, and provides a new research framework for the application of AIGC in the creative industry, detailing the responsibilities, processes, and content of designers in the Artificial Intelligence Generated Design (AIGD) process.
1 Introduction
With the widespread application of artificial intelligence generated content (AIGC) in academic research and commercial practice, governments and international organizations are actively introducing policies and regulations to standardize and promote its development. For instance, the State Council of China released the “New Generation Artificial Intelligence Development Plan” in 2017, which emphasizes AI ethics and safety (Chinese State Council. New Generation Artificial Intelligence Development Plan [EB/OL], 2017); the U.S. government issued the “AI Risk Management Framework” in 2024, aimed at ensuring the reliability and safety of AI systems (National Institute of Standards and Technology, 2024). Additionally, the European Union is expected to release a comprehensive AI regulation draft, the “Artificial Intelligence Act,” in 2024, which seeks to rigorously regulate high-risk AI systems to ensure their safety, transparency, and accountability (European Union, 2021). This demonstrates that various countries recognize the significance of AIGC. It can be seen that AIGC represents the future advanced productivity, and the development of artificial intelligence and communication technology has also promoted the intelligence of human–computer interaction devices, which has research value and application potential (Choudrie et al., 2020). AIGC is extensively applied in technology, education, and healthcare. For example, AIGC provides new pathways for constructing the metaverse through Digital Twin Networks (DTNs) and more natural user experiences (Chen et al., 2024); it enables more equitable smart education through multimodal human–computer interactions (Ren et al., 2023); and it achieves personalized healthcare through Human Digital Twins (HDTs) (Chen et al., 2023). Thus, AIGC leverages its technological advantages to offer personalized services to users through efficient working methods. In the field of social media, it shapes the work processes and social relationships of users in the new era (Wei and Tyson, 2024). In the field of medical care, it improves treatment efficiency and reduces moral hazard (Fraser, 2023; Shao et al., 2024). In the construction of the metaverse, it greatly improves the efficiency of information storage and display (Qin and Hui, 2023). In the field of user experience design, it adds application scenarios and tools for human–computer interaction (Yu et al., 2023). As technology advances, understanding the relationship between humans and artificial intelligence will become increasingly important. Economist Schumpeter believed that the iteration of social technology takes 50 years as a cycle, and new technologies will bring about a revolution in work methods during the process of social transformation (Mallick et al., 2023). Now that we have entered the era of artificial intelligence, AIGC improves the efficiency of social operations by introducing technologies such as big data analysis, graphical language, and rapid prototyping. It can be seen that AIGC represents advanced productivity and future lifestyles.
AIGC, a term originating from the field of computer science, utilizes technologies such as Generative Adversarial Networks (GANs), CLIP, Diffusion, Transformers, multimodal technologies, pre-trained models, and generative algorithms to offer new forms of digital content generation and interaction (Cao et al., 2023). AIGC is widely applied in various fields, including healthcare (Tao et al., 2023), intangible cultural heritage preservation (Cong, 2024), and artistic creation (Li and Liu, 2024). Characterized by efficiency, openness, and personalized training, AIGC integrates text, images, audio, and video through big data to generate solutions tailored to specific tasks, representing a new era of productivity.
In 2022, the OpenAI research laboratory introduced the text generator ChatGPT and the image generator DALL-E2(Harrison, 2023). The objective of AIGC is to streamline and enhance the content creation process, enabling the rapid production of high-quality content (Cao et al., 2023). It can be widely applied to various input formats such as text, images, videos, and 3D content (Liao et al., 2023). However, the limitations of traditional software systems, including poor interactivity and interpretability, have hindered their widespread adoption in practical production scenarios (Tao et al., 2023). In contrast, AIGC technology based on diffusion models meets users’ demands for efficiency, standardization, and intelligence across different output formats (Du et al., 2023). By January 2023, the user base of ChatGPT had exceeded 100 million, making it one of the fastest-growing human–computer interaction applications in history (Du et al., 2023). This underscores the strong demand for artificial intelligence products among users. In summary, AIGC has emerged as a significant research direction in various fields, greatly enhancing work efficiency and user experience. To explore the research progress of AIGC in the academic domain, we analyzed 500 documents selected from the Web of Science database. Figures 1–4 depict the graphs generated based on different keywords.
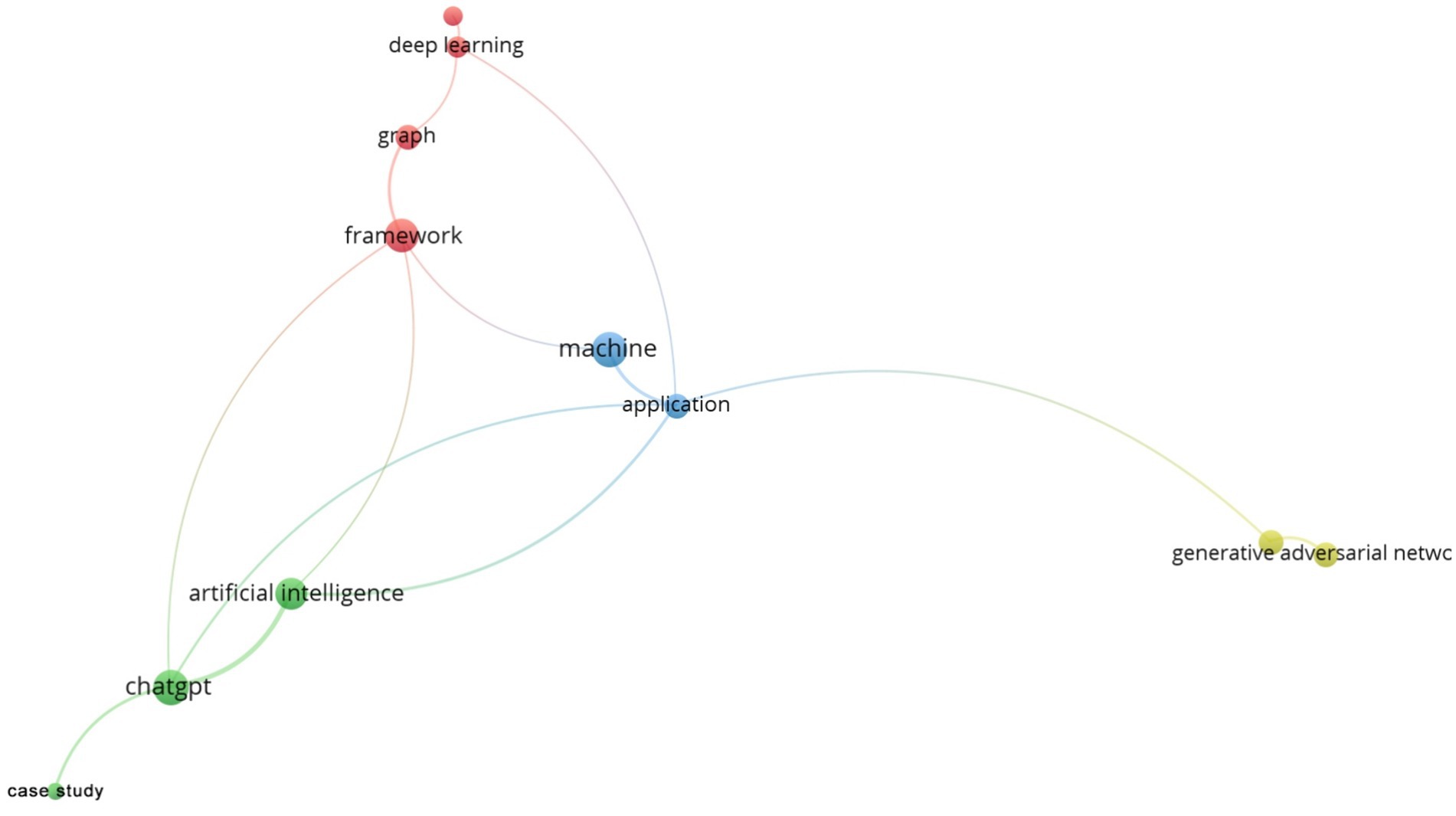
Figure 1. Keyword co-occurrence network under the theme of “Artificial Intelligence Generated Content”(Sort by: Relevance).
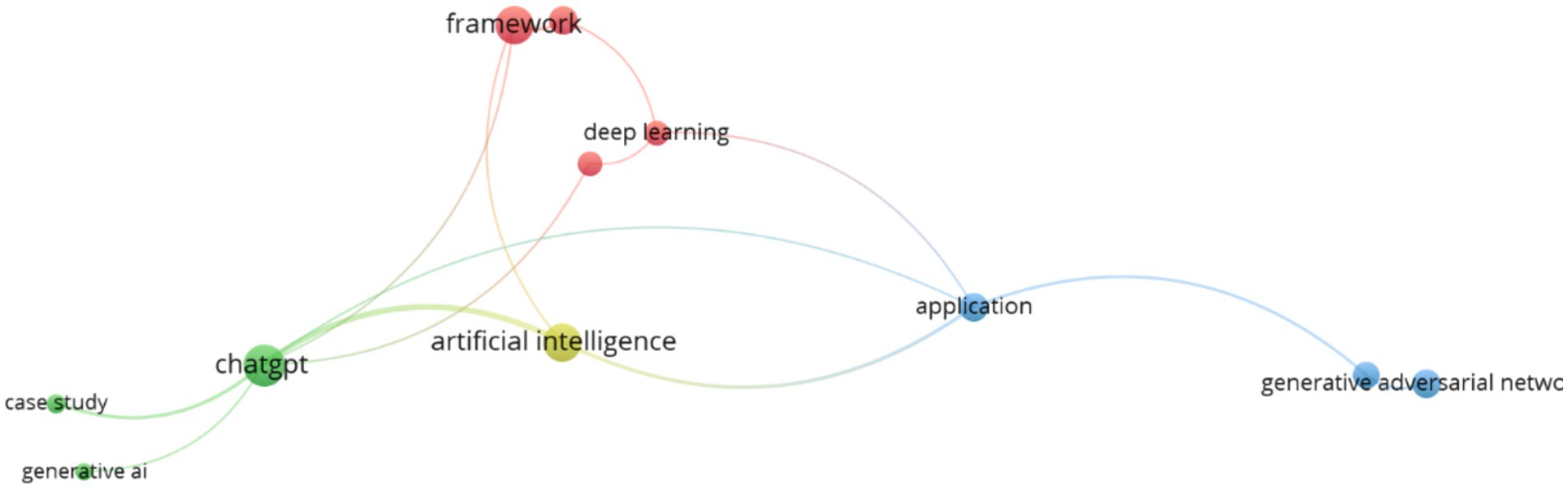
Figure 2. Keyword co-occurrence network under the theme of “Artificial Intelligence Generated Content”(Sort by: Date: newest first).
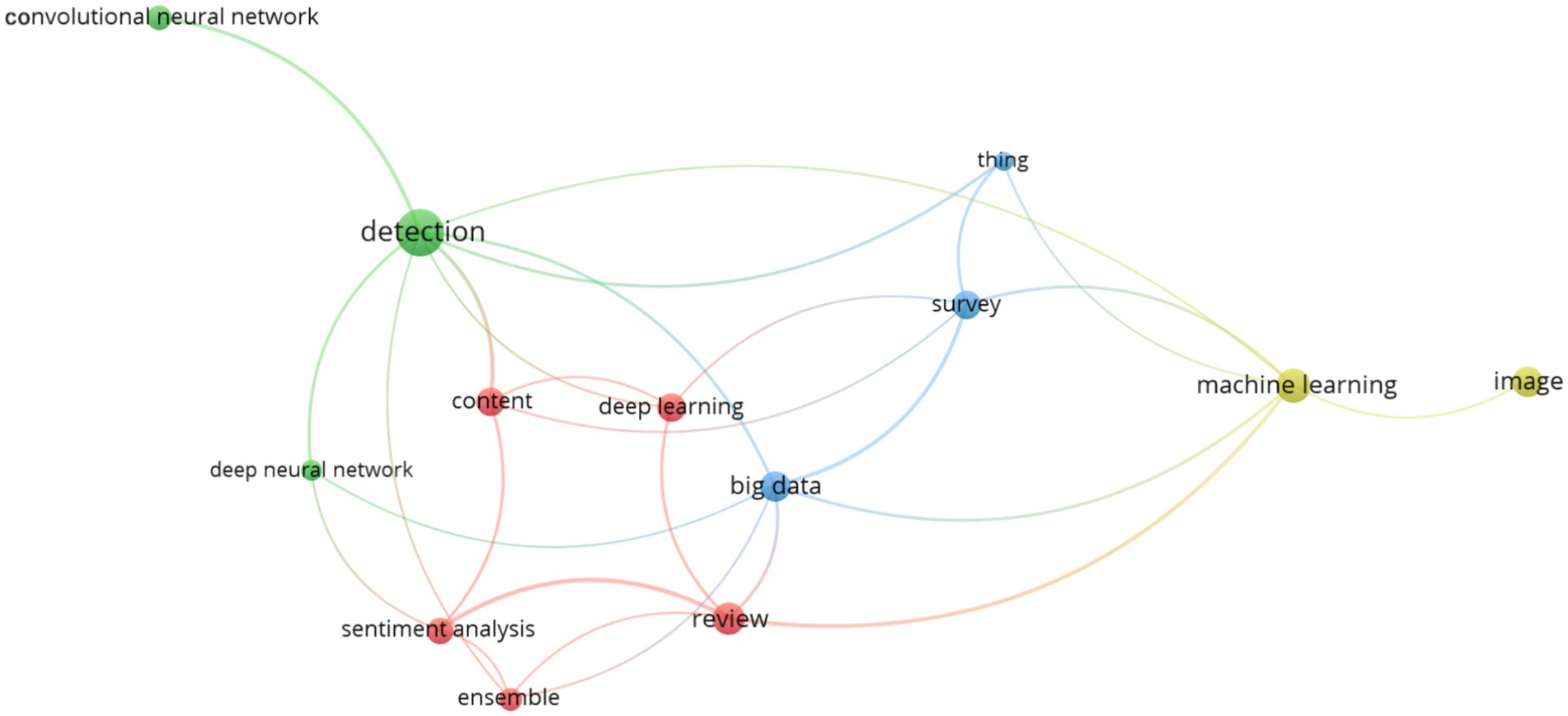
Figure 3. Keyword co-occurrence network under the theme of “Artificial Intelligence Generated Content”(Sort by: Citations: highest first).
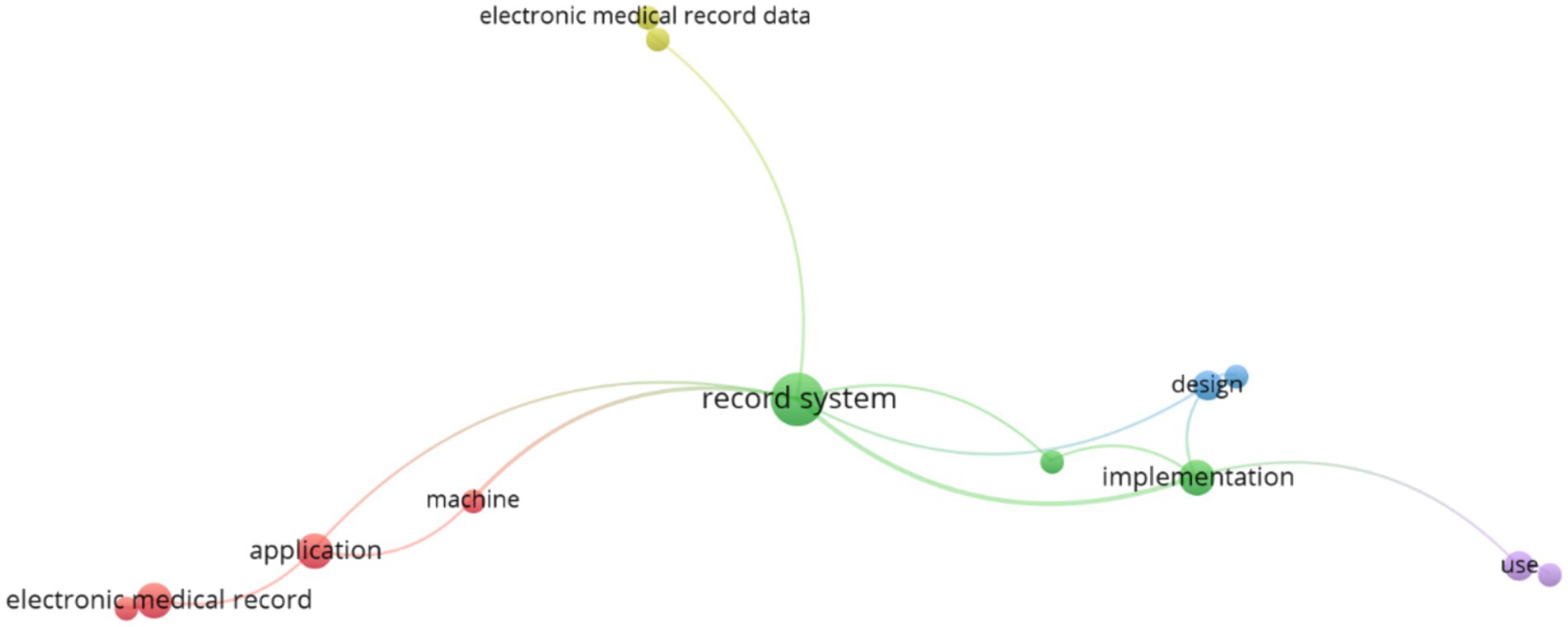
Figure 4. Keyword co-occurrence network under the theme of “Artificial Intelligence Generated Content”&“Systems of Record” (Sort by: Relevance).
Despite the widespread application of AIGC across various platforms, its integration into the creative design industry still faces a prolonged period of adaptation. This is because traditional quantifiable work scenarios are conducive to AI intervention, whereas innovative design relies heavily on human creativity, which involves greater uncertainty and depends more on the personal experience and inspiration of designers. The realm of creative design encompasses industries such as industrial design, visual communication design, environmental art design, digital media art, and environmental art design (Ingtyas et al., 2019). It is a dynamic and complex field driven by creativity and strategic management to foster the generation of new ideas, characterized by its unquantifiable nature (Lindwall et al., 2022; Yuan, 2020). Hence, this study endeavors to explore the work experiences and driving factors of designers in the creative design industry using AIGC.
Although there are numerous studies on artificial intelligence technologies in the field of creativity, they often focus on two extremes: on one hand, they extol the superiority of the technology, such as Stephanopoulos’s view that artificial intelligence has transformed the computing industry over the past 30 years and will become the primary work partner in the future (Stephanopoulos, 1990). In 1955, the scholar John McCarthy introduced the concept of artificial intelligence at the Dartmouth Conference, marking the beginning of the exploration from algorithm evolution to deep learning technology (McCarthy et al., 2006). For example, in his work 《On Artificial Intelligence》, scholar Schönemann expanded the connotation and denotation of “artificial intelligence (Schönemann, 1985).” Scholar Warburton theoretically introduced the significant roles of computer language, natural language processing technology, and speech recognition technology in the development of artificial intelligence. Additionally (Warburton, 2003), scholar Nie proposed that electroencephalogram (EEG) emotion recognition technology could effectively understand human emotional fluctuations (Habash, 2010). Furthermore, researchers have been dedicated to optimizing technological performance and exploring how AI can be integrated into existing systems to drive innovation and improve efficiency across various fields (Brunette et al., 2009).
On the other hand, they highlight the user’s subjective initiative, as Yongqi Lou argues that human creativity’s unique advantages must be preserved through cultural revival (Lou, 2023). Previous research has typically focused on technological development or application processes. Research indicates that artificial intelligence can effectively enhance the quality of teaching and students’ learning experiences in higher education (Nelson Laird et al., 2008). Additionally, in the field of public health, AI has demonstrated a positive impact on prevention mechanisms and treatment outcomes (Yadav, 2020). Of course, the most widespread applications are in the business sector, where AI is employed through mediums such as robots and user interfaces to expand its application scope, significantly improving customer purchasing behavior and shopping experiences (Lv et al., 2021). It is evident that artificial intelligence technology has become widely integrated into our daily lives.
AIGC is reshaping the work methods and processes of the creative industry in unprecedented ways. Its impact is evident in three key areas: (1) AIGC expands the avenues and methods for acquiring design knowledge, giving rise to new models of design knowledge generation; (2) AIGC breaks through the limitations of designers in terms of knowledge accumulation and creative thinking, reducing repetitive tasks while enhancing workflow efficiency and flexibility; (3) the accessibility of AIGC lowers the employment threshold in the creative industry, attracting more talent into the field, which, in turn, presents more challenges and professional anxiety for designers. In conclusion, possessing systematic AIGC theoretical knowledge and design skills has become an essential competence for designers (Yin et al., 2023). Of course, as AIGC continues to permeate various aspects of the creative industry, many scholars have begun to explore its implications on ethics, morality, law, and fairness. In contrast, this study examines the impact of artificial intelligence on the art and design industry, focusing on the relationship between individual designers’ capabilities and their tools. In this research, creativity represents the designer’s capabilities, and AIGC represents the tools. In summary, the novelty of this study lies in its attempt to explore the relationship between AIGC and creativity, aiming to deconstruct the advantages and disadvantages of AIGC from the designer’s perspective.
To further understand the research history and key focus areas of “artificial intelligence, “this study uses the visualization software VOSviewer to conduct clustering of literature keywords, word frequency statistics, and generate a knowledge graph of co-occurrence networks. First, an exact search for the keyword “artificial intelligence” was conducted on the “Web of Science” platform, with the results sorted by topic relevance to obtain the top 1,000 articles. The bibliometric data indicate that recent research hotspots primarily focus on two trends. One trend involves the exploration of technology, with research keywords such as “generative artificial intelligence,” “use,” “analysis,” “future,” “implementation,” and “perspective” outlining technological exploration paths. The other research hotspot is reflected in the application of technology, with keywords such as “medicine,” “editorial,” “healthcare,” and “role” highlighting related fields. The specific clustering results of “artificial intelligence” keywords are shown in Figure 5.
The aim of this research is to investigate the attitudes and willingness of practitioners in the creative industry towards AIGC. It employs the Stimulus-Organism-Response Model (SOR) theoretical framework to analyze the driving factors influencing the usage behavior of practitioners in the creative industry. Ultimately, it aims to provide an empirical analysis report to enhance work efficiency in the creative industry and the compatibility of AIGC technology. The novelty of this study lies in its attempt to examine the relationship between AIGC and creativity, seeking to deconstruct the advantages and disadvantages of AIGC from the perspective of designers. The research findings offer valuable insights for expanding the scope of AIGC applications and enhancing the technological compatibility of AIGC in the creative industry.
2 Materials and methods
To explore the relationship between artificial intelligence and creativity, we utilize the Stimulus-Organism-Response Model (SOR) theoretical framework (Jacoby, 2002; Russell and Mehrabian, 1974) to analyze the impact of the external environment on designers’ innovation capabilities. The SOR theory posits that external environmental stimuli can influence individuals’ perceptual states and drive positive feedback (Ullah et al., 2021), providing a scientifically ordered mechanism for understanding the complexity of designers’ creative abilities (Mariani et al., 2022). It is evident that the SOR theory adequately explains the influencing factors of individual behavioral changes and has numerous applications in the field of user behavior research (Ding et al., 2020).
Scholar Pavlov introduced the classic stimulus-response theory through the “Pavlov’s Dogs Experiment” to discuss the correlation between stimuli and responses, but this theory overlooks the personal traits and internal states of the subjects (Organism). Therefore, scholar Woodworth proposed the SOR model in 1929. This theory suggests that any factor in the experimental setting (including physical and non-physical factors) can affect the organismic experience and internal states of the subjects, especially external physiological feedback and internal cognitive perception (Woodworth, 1929). Since subjects do not immediately respond behaviorally after receiving stimuli but rather influence behavior change through external physiological and internal psychological intermediary states, scholars Albert Mehrabian and James A. Russel further refined the SOR model in 1974 by expanding the scope and objects of experiments. They proposed that various stimuli in the external environment would affect individuals’ emotional experiences, thereby altering their behavioral intentions. Subjects undergo three processes: external environmental stimuli, internal psychological state changes, and eventual behavioral feedback (Russell and Mehrabian, 1974).
In 1992, scholar Bitner further expanded the scope and implications of the SOR model and proposed the user relationship model theory in the service organization context, which comprises three factors: antecedent variables (stimulus), mediating variables (organism), and outcome variables (response) (BITNER, Mary Jo Servicescapes, 1992). With technological iterations, the application domains of the SOR model have extended to various emerging fields such as e-commerce (Kim and Lennon, 2010), online social behavior (Koo and Seon-Hee, 2010), tourism economics (Qiu et al., 2023), and digital media (Baker and Wakefield, 2012). Additionally, researchers have identified two outcomes of user responses to stimuli: (1) positive feedback characterized by affinity, which entails exploration and engagement behaviors (Beatty and Elizabeth Ferrell, 1998) and (2) negative feedback characterized by avoidance, which involves resistance and escape behaviors (Verhagen and Van Dolen, 2011).
To explore the impact of new technologies on user behavior, the technology acceptance model (TAM) requires expansion to predict users’ acceptance of technology (Kazlauskaite et al., 2015). In 1989, scholar Davis proposed the Technology Acceptance Model (TAM) based on the Theory of Reasoned Action to analyze users’ acceptance of new technologies or innovations. The TAM comprises two primary determinants: (1) perceived usefulness, which refers to the extent to which new technology is perceived to enhance efficiency in work, and (2) perceived ease of use, which reflects users’ self-efficacy in mastering new technology. Initially developed to measure the acceptability of computer technology, the model has gradually expanded to various new technology application scenarios. In the realm of online education, it evaluates students’ acceptance of artificial intelligence (Salloum et al., 2019); in electronic consumption, it explains factors influencing consumers’ online shopping behaviors (Sohn and Kwon, 2020); in the field of artificial intelligence, it enhances public acceptance of online libraries (Rafique et al., 2020); in virtual reality, it proposes effective human–computer interaction principles based on immersive technology features (Sagnier et al., 2020). In conclusion, the technology acceptance model (TAM) has been widely applied, with a substantial research foundation, providing valuable reference data for research in the field of artificial intelligence.
In summary, given the rapidly evolving technological landscape, the creative design industry urgently needs to establish a theoretical model tailored to designers’ acceptance of AIGC technology to explore factors influencing designers’ willingness to use it. Therefore, this study adopts the SOR theory as the research framework, integrating the Technology Acceptance Model, Self-Efficacy Theory, and Theory of Planned Behavior to construct a model of designers’ acceptance of AIGC, predicting designers’ acceptance levels of AIGC. This research employs a mixed-method approach combining qualitative interviews and structural equation modeling to consider various dimensions such as perceived usefulness, perceived ease of use, self-efficacy, technological anxiety, and subjective norms affecting designers’ use of AIGC. It predicts user behavior changes through the stimulation of the external environment, organismic changes, and responses. The model proposed in this study transforms important variables from the Theory of Planned Behavior, Technology Acceptance Model, and Self-Efficacy Theory into relevant stimuli and organic states for designers, ultimately driving their responses to use AIGC. By empirically analyzing, this study extends the applicability of the SOR framework and provides a theoretical framework for designers’ usage behavior. The results of this study enrich research on designers’ behavior changes and provide insights for the sustainable development of AIGC.
3 Theory and model building
The Stimulus-Organism-Response (SOR) theory (Jacoby, 2002; Russell and Mehrabian, 1974) is a psychological model comprising three factors: stimulus, organism, and response. This model posits that external stimuli from the environment affect the internal state of organisms, leading to changes in user behavior, thus providing a theoretical framework for understanding behavioral changes (Huang, 2023; Zhang et al., 2021). In the SOR theory, external environmental stimuli typically serve as independent variables, organismic state changes act as mediating variables, and user responses serve as dependent variables. When external stimuli prompt psychological responses in organisms, it spontaneously leads to changes or guides user behavior (Mehrabian, 1974). The SOR theory has been widely applied across various fields. In the realm of public services, it offers solutions for coordinating social crises (Verma, 2020); in healthcare, it provides guidance strategies for students’ mental health during the COVID-19 pandemic (Zhang et al., 2021). In the social domain, it is utilized to explain individual learning responses to external stimuli (Hsiao and Tang, 2021). Additionally, scholars Sampat and Raj proposed in 2022 that the SOR theory can effectively assess or predict the thought processes of users during behavioral changes (Sampat et al., 2022). Furthermore, the theory is extensively applied in online shopping, instant messaging software, e-commerce, and mobile applications to understand the impact of external stimuli on consumer behavior (Jiatong et al., 2021). In conclusion, the SOR theory offers a comprehensive understanding of how stimuli, organisms, and responses interact to influence behavior in various environments. This study posits that designers’ willingness to use AIGC software is influenced by external factors and corresponding emotions. Therefore, the SOR theory framework provides a rational theoretical framework for this study to delve into designers’ decision-making behavior regarding the use of AIGC tools.
The reasons for adopting the SOR theory framework in this study are as follows:
Firstly, the SOR theory framework has a broad application scope, with successful precedents in consumer behavior, online electronic shopping, and offline retail research (Huang, 2017), particularly in studies on the acceptance of computer software (Wu et al., 2021). Additionally, there are mature application precedents in the fields of Augmented Reality (AR), Internet of Things (IoT), and Brain-Computer Interface (BCI) (Park et al., 2019). In other words, the applicability of the SOR theory in explaining the diversity and complexity of user behavior has been validated in various academic research outcomes. Therefore, its application to the willingness and behavior of innovative industry professionals towards AIGC tools is appropriate.
Secondly, the SOR theory possesses high scalability and flexibility, with a wide research background (Talwar et al., 2023). It describes the unidirectional causal relationships between components (What drives brand love for natural products? The moderating role of household size, n.d.) and can adequately explain users’ positive or negative psychological state changes towards AIGC products.
Lastly, and most importantly, the SOR theory provides a good architectural perspective for understanding the impact of antecedents related to AIGC on designers’ psychological and cognitive states, and subsequently on their intentions to use AIGC. These changes in behavior are the focus of this study, as they are suitable for understanding the decision-making process of designers when facing new technologies.
3.1 Stimulus (S)
A stimulus (S) refers to external environmental factors that can influence internal psychological changes in individuals (Fu et al., 2021). It is ubiquitous in various aspects of life (Talwar et al., 2021). External environmental stimuli (S) serve as the independent variable in the system, triggering internal psychological cognition and emotional organisms (O) in users, and ultimately eliciting user behavioral responses (R) (Russell and Mehrabian, 1974). In addition to this, another theory that studies the relationship between user behavior and psychology and explains changes in user behavior is the theory of planned behavior (Douglass, 1977). This theory has been widely applied in fields such as social behavior research. As an important variable in the theory of planned behavior, subjective norms are defined as the degree to which users accept specific behaviors under pressure from individuals or social groups. Social factors such as subjective norms can influence user behavioral intentions, and designers’ choices of AIGC as a creative tool are also influenced by subjective norms (Kim and Ho, 2021). Therefore, this study considers subjective norms as one of the stimulating factors. Thus, the hypotheses of this study are as follows:
H1: Subjective norms have a positive impact on technological anxiety.
H2: Subjective norms positively influence designers’ perception of the usefulness of AIGC.
H3: Subjective norms have a significant positive impact on the intention to use AIGC.
3.2 Facilitating conditions
When users perceive a technology as helpful in improving work efficiency, they are more likely to have a higher intention to use it (Wang et al., 2016). Among the primary factors influencing their adoption of new technology is convenience (Cimperman et al., 2016). Convenience refers to the degree to which users can freely access and use the technology, significantly influencing perceived ease of use (Ma et al., 2016), and indirectly impacting users’ intention to use the new technology (Chen et al., 2018). Therefore, in this study, convenience when using new technology will be considered as the second stimulating factor. Hence, we propose the following hypotheses:
H4: Convenience conditions have a positive impact on designers’ perceived ease of use of AIGC.
H5: Convenience conditions positively influence designers’ intention to use AIGC.
H6: Convenience conditions have a positive impact on designers’ self-efficacy.
3.3 Organism (O)
The organism refers to the user’s perception of the external environment (Tandon et al., 2021), which includes the user’s psychological state, emotional state, and cognitive changes (Duong, 2023). When users face complex external environments, they can utilize their cognitive experiences to distinguish different stimuli and make behavior feedback that is advantageous to themselves (Sun et al., 2021). This study posits that external environmental factors stimulate designers’ cognition and psychological feelings toward AIGC. While the organism encompasses factors such as attitudes, motivations, beliefs, and cognitive awareness (Jacoby, 2002) this research focuses on the influence of self-efficacy, technological anxiety, perceived usefulness, and perceived ease of use on user behavior.
3.4 Self-efficacy
Self-efficacy theory refers to the user’s confidence in their ability to accomplish goal tasks (Bandura, 1977), and it is commonly used to measure the perceived difficulty of users in using new technologies (Purnomo and Lee, 2013). In assessing user behavior change, it is one of the primary predictive factors (Bandura, 2006). Studies have found that increasing self-efficacy can significantly reduce technological anxiety, and it has a significant impact on users’ vocational adaptability, self-monitoring, and work effort (Beatty and Elizabeth Ferrell, 1998). Conversely, lower self-efficacy can make users feel lacking in confidence (Awofala et al., 2019). Additionally, self-efficacy is the best predictor of perceived usefulness and perceived ease of use, and users’ self-efficacy has a positive correlation with perceived usefulness and perceived ease of use (DOAN, Thuy Thanh Thi, 2021). Therefore, this study actively considers self-efficacy as an important influencing factor in designing behavioral changes. The specific hypotheses are as follows:
H7: High self-efficacy of designers has a direct and significant impact on technological anxiety.
H8: Self-efficacy of designers has a direct positive effect on their perceived usefulness of AIGC.
H9: Self-efficacy of designers has a direct positive effect on their intention to use AIGC.
H10: Self-efficacy of designers has a direct positive effect on their perceived ease of use of AIGC.
3.5 Technology anxiety
Technology anxiety refers to users’ concerns that technological changes will bring uncertainty and crises to them (Freeman and Leaf, 1989). Technology anxiety is suitable for studying users’ ability and psychological state when using new technologies, and it provides theoretical support for the birth of the Technology Acceptance Model (TAM) (Abdullah and Ward, 2016). It is often combined with the Technology Acceptance Model (TAM) to explore users’ psychological changes. For example, it has explored factors influencing consumers’ acceptance of smartphones with built-in NFC functionality (Chen and Chang, 2013), analyzed the skills of students learning mathematics through virtual reality (Sampat et al., 2022), and explained patients’ perceived intention to resist remote healthcare (Baccarella et al., 2021). Particularly when users are faced with the large amount of data presented by AIGC, it is important to understand their unstable factors when dealing with new technologies. Therefore, this study proposes the following hypotheses:
H11: Technology anxiety has a negative significant effect on the usefulness of AIGC.
H12: Technology anxiety has a significant negative impact on designers’ intention to use AIGC.
H13: Technology anxiety has a significant negative impact on the perceived ease of use of AIGC.
3.6 Perceived ease of use
Perceived ease of use is a crucial measurement in the technology acceptance model, referring to the learning cost users incur when adopting new technologies (Davis, 1989). Users’ perceptions of ease of use and usefulness of electronic learning systems influence their attitudes and behaviors (Chatterjee and Kar, 2020). It has been utilized in educational research to explore students’ acceptance of e-learning (Salloum et al., 2019), and in virtual reality studies to investigate the negative impact of perceived usefulness on internet addiction (Al-Emran et al., 2020). This study posits that, when designers perceive ease of use in AIGC technologies, their perception of usefulness and willingness to use AIGC will increase. Therefore, this study proposes the following hypothesis:
H14: Perceived ease of use positively influences perceived usefulness.
H15: Perceived ease of use positively influences behavioral intention.
H16: Perceived usefulness positively influences behavioral intention.
3.7 Response (R)
Response refers to the behavioral changes made by individual users based on cognitive experience and emotional responses (Sultan et al., 2021). It is the avoidance or approach feedback exhibited by users to maintain their own interests, which can explain users’ real experiences, cognitive and emotional responses, and behavioral intentions (Sherman et al., 1997) In this study, the intention to use refers to the willingness of designers to use AIGC. Therefore, this study proposes the following hypotheses:
In summary, based on AIGC, this study proposes a conceptual model for designers’ usage behavior, as shown in Figure 6. This model consists of 16 research hypotheses and 7 influencing variables. Moreover, the model proposed in this study integrates key variables from the Theory of Planned Behavior, the Technology Acceptance Model, and self-efficacy theory, and translates them into relevant stimuli and organic states when designers use AIGC, ultimately driving designers’ intention to use AIGC.
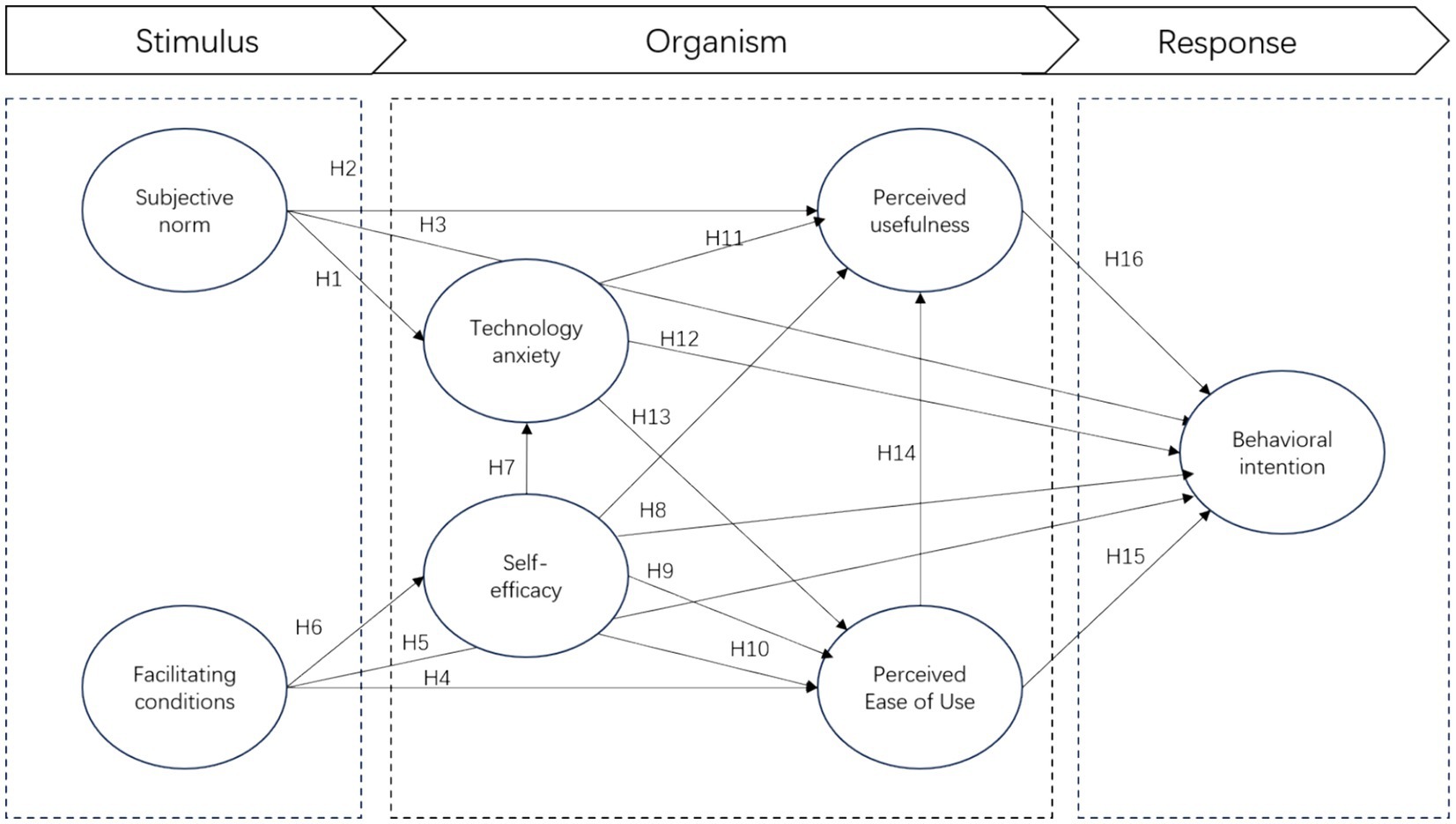
Figure 6. The proposed conceptual model. (This model providing a theoretical framework for industrial designers’ AIGC use behavior).
4 Research methods
4.1 Research subjects
Given that I teach a course related to “Artificial Intelligence Assisted Design,” the students enrolled have undergone systematic design theory education, are proficient in traditional design tools, and have some understanding of AIGC. Therefore, they are highly suitable as subjects for this study. Prior to the questionnaire, verbal consent and approval of the experimental design were obtained from them.
To ensure the representativeness of the subjects and the rationality of the experimental process, the first stage involved recruiting respondents from students who had previously taken the course, using a sampling method. Respondents were informed about the purpose of the study and relevant AIGC knowledge. The participants are design students or designers with design experience and innovation capabilities, either currently enrolled or recently graduated. A total of 250 questionnaires were collected. After excluding those with overly brief response times, identical answers to key variables, and missing values, 226 valid online questionnaires were retained, resulting in a validity rate of 90.4%.
The second stage employed semi-structured interviews to further investigate respondents’ subjective attitudes. Paper questionnaires were distributed only after obtaining verbal consent from the respondents. The information of the respondents is shown in Table 1. Among them, 46.9% are male and 53.1% are female; all respondents are under 30 years old, either current students or recent graduates. There are 132 design students (80.7%) and 94 graduated designers (19.3%). 196 respondents (86.8%) have experience with AIGC, while 30 (13.2%) do not. Despite differences in gender, grade, and work experience, all respondents are proficient with traditional design tools and show a high level of interest and learning ability regarding new design tools represented by AIGC. Additionally, 30 respondents who completed the questionnaire participated in semi-structured interviews, including 13 males and 17 females. They all have experience with AIGC and can articulate their views and experiences with it.
4.2 Research procedures and methods
To obtain more comprehensive information, the validation of factors driving designers’ use of AIGC proposed in this study employed a mixed research method of questionnaire and scale surveys. The first stage of data collection involved semi-structured interviews. Before undergoing testing, the interviewees were informed about the background and purpose of this study after learning about the characteristics of AIGC technology from various channels. The measurement items of this study’s scale are shown in Table 2, with items for perceived ease of use and perceived usefulness drawn from (Huang, 2023; Davis, 1989), subjective norm from (Douglass, 1977), technology anxiety from (Hsu and Peng, 2022), behavioral intention and convenience conditions from (Venkatesh et al., 2012), and self-efficacy from (Huang, 2023; Compeau and Higgins, 1995). All items for variables utilized a 5-point Likert scale, with 1 representing strongly disagree and 5 representing strongly agree.
The second stage of the survey questionnaire delved deeper based on the previous scale items. After obtaining consent from the respondents, they were invited to continue participating in semi-structured interviews. The focus of this interview was to explore designers’ intentions of use based on three questions: (1) What do you perceive as the challenges of AIGC in the creative industries? (2) What motivates your interest in using AI products? (3) What prevents you from increasing the frequency of using AIGC products? A total of 226 valid questionnaires were collected in this interview phase. The specifics are outlined in Table 2.
4.3 Analytical methods
4.3.1 Loading
In statistics, the loading value refers to the strength of the association between observed variables and latent variables in factor analysis or structural equation modeling (Fornell and Larcker, 1981a). Loadings reflect how well an observed variable represents the characteristics of a latent variable, and therefore play a crucial role in assessing model quality. This study uses the following thresholds for loading values: (1) Loading ≥0.7: indicates a very good indicator, demonstrating that the observed variable has strong explanatory power for the latent variable. (2) 0.5 ≤ Loading <0.7: acceptable value, particularly in the early stages of research or exploratory studies. (3) Loading <0.5: typically considered less ideal, suggesting that the observed variable has weak explanatory power for the latent variable and may need to be reconsidered or excluded. The results of this study show that the loadings in Table 2 range from 0.706 to 0.876, all exceeding the conventional threshold of 0.7 (Fornell and Larcker, 1981b), indicating good reliability in the measurement range.
4.3.2 Cronbach’s alpha
Cronbach’s alpha (α) is an indicator of internal consistency among items in a questionnaire, commonly used to assess the reliability of a scale (Anderson and Gerbing, 1988). Its value ranges from 0 to 1, with higher values indicating better internal consistency of the scale. This study uses the following thresholds for Cronbach’s Alpha: (1) α ≥ 0.9: very high internal consistency, with possible item redundancy; (2) 0.8 ≤ α < 0.9: good internal consistency; (3) 0.7 ≤ α < 0.8: acceptable internal consistency; (4) 0.6 ≤ α < 0.7: questionable internal consistency, potentially needing improvement; (5) α < 0.6: poor internal consistency, requiring significant improvement of the scale. The Cronbach’s alpha values for all variables in Table 3 range from 0.722 to 0.924, all exceeding the conventional threshold of 0.7 (Anderson and Gerbing, 1988).
4.3.3 Composite reliability
Composite reliability (CR) is an indicator used in Structural Equation Modeling (SEM) to measure the internal consistency of latent constructs. It provides a more precise measurement than Cronbach’s alpha, particularly when factor loadings are not entirely equal. CR values range from 0 to 1, with higher values indicating better internal consistency of the scale. This study adopts the following thresholds for CR: (1) CR ≥ 0.7: indicates good internal consistency; (2) 0.6 ≤ CR < 0.7: acceptable, especially in exploratory research; (3) CR < 0.6: indicates poor internal consistency, potentially requiring reassessment or improvement of the measurement tool. The Composite Reliability (CR) values in this study range from 0.75 to 0.86, all exceeding the conventional threshold of 0.7 (Nunnally and Berstein, 1994).
4.3.4 Average variance extracted
Average variance extracted (AVE) is an indicator used in structural equation modeling (SEM) and factor analysis to assess the convergent validity of latent constructs. It represents the average amount of variance that a latent variable explains in its observed variables. Higher AVE values indicate that the latent variable explains the variance in observed variables well, reflecting strong convergent validity. AVE values range from 0 to 1, with higher values indicating greater explanatory power of the latent variable. This study uses the following thresholds for AVE: (1) AVE ≥ 0.5: indicates good convergent validity, where observed variables effectively reflect the characteristics of the latent variable; (2) AVE < 0.5: indicates lower convergent validity, suggesting the need to improve the model or scale. The AVE values extracted in this study range from 0.5 to 0.67, all exceeding the conventional threshold of 0.5 (Nunnally and Berstein, 1994), demonstrating good convergent validity and effective reflection of latent variables by observed variables.
Based on the definitions and measurement data above, the survey used in this study aligns with the research objectives, showing good convergent validity and reliable measurement models. It meets the validity standards recommended by scholars Fornell and Larcker (1981a), making the scale suitable for further exploring designers’ willingness to use AIGC.
4.4 Analytical tools
To enhance the predictive power and explanatory strength of the model, structural equation modeling (SEM) often involves repeated cross-validation processes to ensure the reliability and consistency of endogenous variable measurements. This approach helps to ensure model stability, prevent overfitting, and provide a comprehensive assessment of model performance. In SEM, the structural model refers to the component that describes the relationships between latent variables, while endogenous variables are those predicted or explained by other variables in the model. In the structural model, endogenous variables serve as dependent variables, with their values influenced by other variables in the model (exogenous variables or other endogenous variables).
This study employed IBM SPSS 25.0 for data statistical analysis. We conducted reliability tests, validity tests, descriptive statistics, and internal consistency checks using this software. The specific analysis results are outlined in Table 3.
In this study, the main factors were measured using scales, so it is crucial to inspect the quality of the measurement results to ensure the meaningfulness of subsequent analyses. Firstly, internal consistency was analyzed using Cronbach’s alpha coefficient reliability test method. Cronbach’s alpha coefficient ranges from 0 to 1, and the obtained value is 0.837, exceeding the standard of 0.7. In this analysis, the results of reliability analysis are presented in Table 3, indicating that the reliability coefficients of each secondary dimension fall within the range of 0.7 to 1. Therefore, it can be concluded that the scales used in this study exhibit good internal consistency and reliability. Refer to Table 4 for specific details.
To enhance the predictive capability and explanatory power of the model, structural equation modeling (SEM) often employs repeated cross-validation processes to ensure the reliability and consistency of measurements for endogenous variables. This method helps to ensure model stability, prevent overfitting, and provide a comprehensive assessment of model performance. In SEM, the structural model refers to the component that describes the relationships between latent variables, while endogenous variables are those predicted or explained by other variables in the model. In the structural model, endogenous variables act as dependent variables, with their values influenced by other variables in the model (exogenous variables or other endogenous variables).
The structural model’s endogenous variables are measured by cross-validation of redundancy. When the redundancy of the endogenous variables is greater than 0, it indicates that the endogenous variables of the structural model are correlated (Chin, 1998). According to the model fit test results in Table 5, CMIN/DF (chi-square degrees of freedom ratio) = 1.696, falling within the range of 1–3, and RMSEA (root mean square error of approximation) = 0.079, within the excellent range of <0.08. Additionally, the results of ITI, TL, and CF1 tests all exceed the excellent level of 0.9. Therefore, based on the comprehensive analysis results, it can be concluded that the model used in this study exhibits good fit.
5 Research result
“In this study, the significance of various coefficients was examined using the bootstrapping method. As shown in Table 5, of the 16 proposed hypotheses, only 5 were validated, while 11 were not. The specific results are as follows: subjective norms significantly affect perceived usefulness (β = 0.277, t = 3.289, p < 0.05), thus H2 is supported, indicating that perceived usefulness remains a key factor in determining users’ willingness to use technology; facilitating conditions significantly impact perceived ease of use (β = 1.049, t = 4.158, p < 0.05), thus H4 is supported, suggesting that convenient usage conditions enhance users’ perceptions of technology; facilitating conditions also significantly affect self-efficacy (β = 0.433, t = 5.353, p < 0.05), thus H6 is supported, indicating that favorable conditions boost users’ confidence; self-efficacy significantly impacts perceived usefulness (β = 0.595, t = 2.901, p < 0.05), thus H8 is supported, showing that users’ confidence influences their judgments of technology quality; self-efficacy significantly affects intention to use (β = 0.963, t = 3.316, p < 0.05), thus H9 is supported, demonstrating that users’ confidence is a crucial factor in adopting new technology. Among the validated hypotheses, the complete behavioral path diagram is illustrated in Figure 7, which shows that, in the context of AIGC, facilitating conditions can positively influence self-efficacy, thereby affecting the intention to use AIGC.”
The remaining hypotheses were not supported, as follows: subjective norms did not significantly impact technology anxiety (β = 0.034, t = 0.267, p > 0.05), thus H1 was not validated; subjective norms did not significantly affect behavioral intention (β = 0.097, t = 1.353, p > 0.05), thus H3 was not validated. These results indicate that subjective norms do not directly influence behavioral change in designers. Facilitating conditions did not significantly impact behavioral intention (β = 0.045, t = 0.216, p > 0.05), thus H5 was not validated, suggesting that facilitating conditions affect usage behavior only through the mediation of self-efficacy. In other words, facilitating conditions represent the objective conditions of AIGC, while self-efficacy represents the subjective conditions; both must be integrated to influence designers’ usage behavior. Self-efficacy did not significantly impact technology anxiety (β = 0.433, t = 5.353, p < 0.05), thus H7 was not validated; self-efficacy did not significantly affect perceived ease of use (β = −0.335, t = −0.832, p > 0.05), thus H10 was not validated. This suggests that self-efficacy does not directly influence external technological changes. Technology anxiety did not significantly impact perceived usefulness (β = −0.021, t = −0.291, p > 0.05), thus H11 was not validated; technology anxiety did not significantly affect behavioral intention (β = −0.017, t = −0.328, p > 0.05), thus H12 was not validated; technology anxiety did not significantly impact perceived ease of use (β = 0.006, t = 0.071, p > 0.05), thus H13 was not validated. This indicates that technology anxiety does not directly influence external technological changes. Perceived ease of use did not significantly impact perceived usefulness (β = 0.058, t = 0.669, p > 0.05), thus H14 was not validated; perceived ease of use did not significantly affect behavioral intention (β = −0.066, t = −0.634, p > 0.05), thus H15 was not validated. This suggests that perceived ease of use does not directly influence users’ behavioral change. Perceived usefulness did not significantly impact behavioral intention (β = 0.134, t = 1.280, p > 0.05), thus H16 was not validated, indicating that users are unlikely to adopt new technology without clear applications and prospects for AIGC.
Thus, there is still a significant adjustment period from AIGC to artificial intelligence generated design (AIGD). The reasons can be summarized as both subjective and objective factors. Subjectively, many designers’ distrust of new things, reluctance to try, career anxiety, and high learning costs hinder their curiosity about AIGC. Objectively, some paid AI products, such as ChatGPT 4.0 and Midjourney, raise the usage threshold for designers, and the lack of systematic training channels also prevents AIGC from being quickly applied in practical work. Detailed data is shown in Table 5, and the structural equation hypothesis diagram is illustrated in Figure 8.
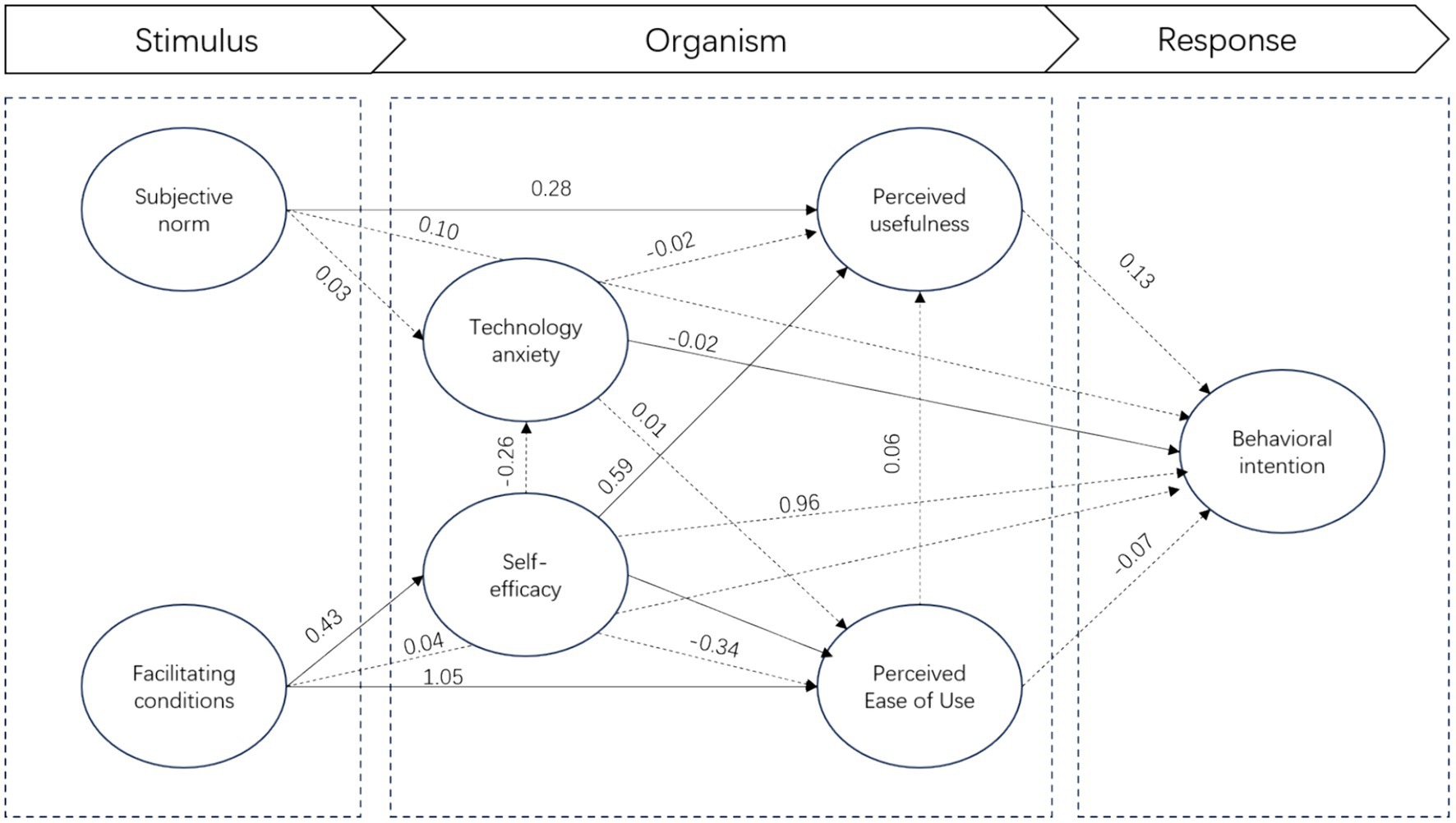
Figure 8. Results of model analysis. It explored the effects of subjective norms, facilitating conditions, technology anxiety, self-efficacy, perceived usefulness, and perceived ease of use on industrial designers’ intention to use AIGC.
Following the questionnaire survey, we sought to explore designers’ views on AIGC and understand the attitudes behind their behaviors through in-depth interviews. This study considers three representative questions (Table 6) to examine the impact of AIGC on their learning and work, as well as to understand their coping strategies. For example, respondent 7, when answering “Question 1: What challenges could AIGC bring?” stated, “AIGC systems are often trained based on known data or experiences, so they may be limited by existing frameworks and unable to create materials they have never seen before.” This viewpoint also reflects the concerns of creative industry practitioners about the creativity of AIGC. Additionally, respondent 3 raised concerns about the risks posed by AIGC, stating, “The extensive use of AIGC can create legal and ethical issues.” This is a topic worthy of further exploration. While AIGC’s potential has been recognized in commercial applications and academic research, it still faces ethical risks and technical compatibility issues. On one hand, AIGC challenges existing legal rules in regulations involving human public interests (Zhang et al., 2023). On the other hand, the distribution of individual human interests, produces different results based on users’ varying learning backgrounds and cognitive levels, thereby inadvertently widening the gap in users’ abilities (Huang et al., 2024). Additionally, AIGC poses varying degrees of risks to all users’ privacy and data security (Zhang et al., 2023). These factors all influence users’ acceptance and user experience of AIGC. Representative interview content is shown in Table 6.
AI has promoted social progress and optimized industrial structure on a macro level, while improving individual work efficiency and reducing repetitive labor on a micro level. Particularly for the creative industry, AI is like a double-edged sword. On one hand, creative industry practitioners hope to improve work efficiency with AI. On the other hand, they are concerned that AI may lower industry entry barriers and reduce job opportunities and salaries. Its main impacts on the creative industry are reflected in several aspects.
The advantages that AIGC brings to designers and the creative industry are as follows: (1) it reduces repetitive labor and improves work efficiency. AIGC technology can generate a large number of design proposals in a short time, saving designers time and energy; (2) it has strong learning and induction capabilities, enhancing design creativity expression and implementation. AIGC technology can explore novel points and potential inspirations that designers may overlook through different algorithms and data analysis methods, enhancing design creativity; (3) AI can achieve customized services based on big data background user experiences. AIGC technology can optimize user experience through analysis of user behavior data, enhancing market competitiveness.
The threats posed by AIGC to designers and the creative industry are as follows: (1) it cannot replace human aesthetics. Although AIGC technology can generate design proposals, it cannot replace human aesthetics and personalized demands; (2) lack of design thinking and leadership. AIGC technology can generate a large number of design proposals, but it cannot understand the intent and purpose of design, and cannot fundamentally solve design problems; (3) possibility of repetitive design. Due to the training of AIGC technology algorithms based on existing data, there may be similar or repetitive design proposals.
However, we can balance the advantages and disadvantages of AIGC technology through some methods. Specific measures include: (1) making the generation process and creative thinking of AIGC algorithms transparent and interpretable, allowing humans to better understand the generation process and results of AIGC technology; (2) guiding AI training through enhanced human–computer interaction to achieve consistency in thinking and skills; (3) increasing affinity through humanized interfaces or forms. Designing more friendly and user-friendly interfaces can make AIGC technology more in line with human needs and creativity.
6 Discussion and conclusion
6.1 Implications
AIGC is rapidly transforming the creative design industry by enhancing design quality through increased efficiency, reduced costs, and personalized services, while also driving interdisciplinary integration and transforming design education. This presents unprecedented development opportunities for the creative industry (Huang et al., 2024). After exploring the key factors influencing designers’ adoption of AIGC, from objective conditions to subjective intentions, this study concludes that the main barriers to the rapid adoption of AIGC in the creative industry are concerns regarding intellectual property and ethical challenges.
Undoubtedly, mastering AIGC has become an indispensable skill for future designers. However, transitioning from AIGC to AIGD, the application of AIGC technology in the field of design continues to expand and deepen, providing designers with more tools and possibilities, while also promoting innovation and development in design. Its impact on the creative industry can be seen in several aspects: (1) providing designers with more intelligent design tools. With the continuous development of AIGC technology, more and more intelligent design tools will be developed. These tools can help designers complete design tasks more quickly and accurately, while also being able to intelligently optimize based on user feedback. (2) Facilitating interdisciplinary collaboration. AIGC requires expertise from multiple fields, thus promoting interdisciplinary collaboration to achieve better design outcomes. (3) Designers also need to bear design ethics and legal responsibilities. Designers must ensure the fairness and transparency of AIGC algorithms, avoiding bias and discrimination in AIGC technology. These are issues that future designers need to pay attention to and consider.
In the creative industry, the legal risks associated with AIGC primarily include issues related to copyright and intellectual property ownership and infringement liability, the dissemination of false information and fraud, data privacy and ethical concerns, as well as contract and licensing agreements. First, although there have been widespread calls for the government to establish effective punitive measures against unlawful AI activities, the progress of policy implementation has been relatively slow (Gaifutdinov et al., 2020). The main reason lies in the complexity of AIGC application scenarios and the diversity of evaluation criteria. The specific legal risks can be analyzed from the following three perspectives: (a) Copyright and intellectual property ownership: public policy must clearly protect these rights, particularly patent rights. AIGC-generated content may infringe on others’ copyrights or privacy, leading to legal disputes. Additionally, the generation of false content by AIGC could be used for fraudulent purposes. (b) Data privacy: this must be ensured by the technology platforms. Designers require vast amounts of data for AIGC model training, and the collection and use of this data might violate privacy protection laws. The inherent technical characteristics of AIGC could inadvertently lead to personal data breaches, further complicating the determination of infringement. (c) Data authenticit: ensuring the authenticity of data is a prerequisite for its commercial application. Since AIGC can generate highly realistic false content (e.g., fake news, images, videos), such content could be used for fraud, misleading the public, or infringing on others’ rights. Therefore, the authenticity and validity of AIGC content must be verified before its use. In conclusion, to regulate the risks brought by artificial intelligence technology, designers must operate within the framework of policy regulations, ensure data security, and uphold the principles of authenticity and validity when applying AIGC commercially.
Technological change brings new challenges and opportunities to societal production models. In the face of AIGC’s transformation of the creative industry, designers must first assess its feasibility from the perspectives of external legal risks, internal ethical risks, and the fairness that balances both. Only then can AIGC be broadly integrated into creative work. In summary, the widespread application of AIGC in the creative industry needs to meet the following three conditions:
The moral risks brought by AIGC in the creative industry mainly cover content authenticity, trustworthiness of works, weakening of subjective values of creators, unclear attribution of moral responsibility, and spreading of prejudice and discrimination, which can weaken the output of cultural diversity and individualized viewpoints. This can be analyzed from the following three perspectives: (a) designers need to ensure the content’s authenticity and the work’s trustworthiness before using AIGC, and the destruction of social trust by false content must be avoided; (b) designers must ensure that AIGC complies with social moral codes and ethical standards to avoid negative social impacts. Due to the autonomy-generating nature of AIGC, generating content may lead to unethical or harmful outcomes, such as spreading hate speech, violent content, and so on. How to pursue responsibility and attribution is a complex ethical issue; (c) designers need to actively maintain cultural diversity and individualized creative perspectives. As AIGC is based on integrating big data and innovation, its design proposals tend to generate content that conforms to mainstream culture and aesthetics, which will lead to homogenization of cultural expression and weaken the space for the expression of cultural diversity and minority cultures. In conclusion, to ensure that AIGC is applied ethically, its reasonableness should be assessed from the perspective of individual moral constraints and social expectations.
The inequality that AIGC may bring in the creative industry is mainly reflected in the imbalance in access to resources, the application of technology, and the distribution of opportunities. In addition, AIGC may weaken the subjective status of human creators and amplify the bias in the data, leading to the restriction of cultural expression of minority groups. AIGC will enhance the level of creativity of social groups on the one hand, and exacerbate the differences in the level of individual creativity on the other hand. These phenomena and pitfalls can be discussed from the following three perspectives: (a) inequitable access to knowledge and resources. Individuals’ acceptance of and proficiency in new technologies will magnify the differences between them, thus reinforcing the inequality of access to knowledge. (b) Inequity in access to cultural expression and creativity will be exacerbated. As AIGC models are often trained based on large-scale models, these data are more biased towards the mainstream culture, which will weaken the uniqueness of human creativity and intensify the bias of cultural expression. (c) Inequity in income and opportunity distribution. As AIGC undermines the market demand for human creators and lowers the employment threshold for different industries, this will lead to a reduction in income and employment opportunities for junior people, which in turn exacerbates economic inequality. The above analyses the challenges brought by AIGC to the creative industry from the path of access, application, and distribution of resources, and these hidden dangers of technological development need to be taken seriously by designers and actively guided by policies in order to achieve healthy and sustainable social innovation. In conclusion, the opportunities brought by AIGC to the creative industry are more efficient, high-quality, and high-experience work models, while the resulting legal, ethical, and unfair pitfalls will accompany the technological changes and the development of the industry (Bertoncini, 2023).
The research results show that in the creative industry, designers’ perceptions of the usefulness of AIGC, perceived ease of use, technology anxiety, self-efficacy, and convenience conditions all influence their willingness to use and behavior. Among the 16 hypotheses in the structural equation, only five hypotheses were validated: subjective norms significantly influence perceived usefulness, technology anxiety significantly influences perceived ease of use, technology anxiety significantly influences self-efficacy, self-efficacy significantly influences perceived ease of use, and self-efficacy significantly influences intention to use. The reasons behind these findings lie in the subjective barriers to usage, technological development and compatibility, as well as objective factors such as self-learning channels, learning costs, and rapid technological advancements.
6.2 Limitations and future directions
This study has several limitations. Firstly, the experiment was conducted in August 2023, when the participants had limited experience with AIGC and were in the early stages of learning. Secondly, many designers self-learned AIGC through online resources, leading to a lack of systematic understanding of its usage methods, which in turn may have affected their willingness to use the technology. Lastly, the rapid iteration of AI technology poses challenges to future research hypotheses and expected results. Despite these uncertainties, updating designers’ design tools through technology remains an effective means of improving productivity and creativity. Future designers can combine intuitive creativity with rational induction from AIGC to create a collaborative working mode between humans and machines.
The application of artificial intelligence in the creative industry emphasizes the subjective initiative of designers. Artificial Intelligence Generated Design (AIGD) is an innovative process guided by designers, based on the extensive data provided by AIGC. Studies have shown that human-machine collaborative work models can effectively enhance work quality, especially in the creative industry (Bogucka et al., 2024). AIGC offers more channels for knowledge acquisition, shortens the design research process, and expands the scope of design proposals, bringing transformative changes to the traditional roles, content, imitation, procedures, and methods of designers (Meron, 2022). Thus, the ability to utilize AIGC will become a fundamental competency for future designers. It redefines the processes and methods of innovation and has already achieved numerous successful precedents (Wang and Chen, 2024).
For example, in the field of graphic design, the division and collaboration between AIGC and designers have improved the quality of creative thinking (Lin and Liu, 2024). In industrial design, AIGC optimizes the process of product innovation and the details of product coloring in later stages, enhancing product competitiveness (Wu et al., 2024). In fashion design, AIGC meets market demands for cultural diversity and personalization (Wu et al., 2024). In digital media art, AIGC can provide higher quality and more diverse characters in game scene design, further improving the entertainment experience and commercialization of games (Li and Liu, 2024). In conclusion, the collaboration between designers and AIGC will stimulate the innovative capabilities of AIGD, thereby increasing the efficiency and quality of work in the creative industry.
Artificial Intelligence Generated Design (AIGD) refers to a collaborative innovation work model where designers leverage the advantages of AIGC. In this process, the responsibilities and roles of designers will undergo significant changes:
(1) Designers will transition from being “creators” to “supervisors.” As AIGC utilizes the advantages of big data to quickly, automatically, and extensively complete specific tasks, the designer’s role will shift from executing creative tasks to making creative decisions. Designers will need to develop AIGC work methods and processes and review and adjust the output results.
(2) Designers will need stronger innovative capabilities to scientifically guide AIGC creation. Given AIGC’s advantage in data integration, designers must possess a stronger sense of innovation to create forward-thinking design solutions that differ from traditional big data approaches.
(3) Designers must have interdisciplinary and team collaboration skills. Since AIGC involves expertise from multiple fields, designers will need to focus more on team collaboration and communication to better integrate knowledge and resources from various areas, achieving superior design outcomes.
In conclusion, this study constructed a model of designers’ acceptance of AIGC, integrating the theory of planned behavior (TPB), technology acceptance model (TAM), and self-efficacy, with a good fit of the model. Empirical analysis using structural equation modeling demonstrated that perceived usefulness, perceived ease of use, technology anxiety, self-efficacy, subjective norms, and convenience conditions are effective measurement factors for designers’ use of AIGC. This study further expands the application scope of AIGC and the measurement range of the technology acceptance model, providing references for both practical application and academic research in the field of AIGC.
Data availability statement
The original contributions presented in the study are included in the article/supplementary material, further inquiries can be directed to the corresponding author.
Ethics statement
Ethical review and approval was not required for the study on human participants in accordance with the local legislation and institutional requirements. Written informed consent from the patients/participants or patients/participants’ legal guardian/next of kin was not required to participate in this study in accordance with the national legislation and the institutional requirements.
Author contributions
S-FW: Conceptualization, Data curation, Formal analysis, Funding acquisition, Methodology, Project administration, Resources, Software, Validation, Visualization, Writing – original draft, Writing – review & editing. C-CC: Investigation, Supervision, Writing – review & editing.
Funding
The author(s) declare that no financial support was received for the research, authorship, and/or publication of this article.
Conflict of interest
The authors declare that the research was conducted in the absence of any commercial or financial relationships that could be construed as a potential conflict of interest.
Publisher’s note
All claims expressed in this article are solely those of the authors and do not necessarily represent those of their affiliated organizations, or those of the publisher, the editors and the reviewers. Any product that may be evaluated in this article, or claim that may be made by its manufacturer, is not guaranteed or endorsed by the publisher.
References
Abdullah, F., and Ward, R. (2016). Developing a general extended technology acceptance model for E-learning (GETAMEL) by analysing commonly used external factors. Comput. Hum. Behav. 56, 238–256. doi: 10.1016/j.chb.2015.11.036
Al-Emran, M., Mezhuyev, V., and Kamaludin, A. (2020). Towards a conceptual model for examining the impact of knowledge management factors on mobile learning acceptance. Technol. Soc. 61:101247.
Anderson, J. C., and Gerbing, D. W. (1988). Structural equation modeling in practice: a review and recommended two-step approach. Psychol. Bull. 103:411. doi: 10.1037/0033-2909.103.3.411
Awofala, A. O. A., Olabiyi, O. S., Awofala, A. A., Arigbabu, A. A., Fatade, A. O., and Udeani, U. N. (2019). Attitudes toward computer, computer anxiety and gender as determinants of pre-service science, technology, and mathematics teachers' computer self-efficacy. Digital Educ. Rev. 36, 51–67. doi: 10.1344/der.2019.36.51-67
Baccarella, C. V., Wagner, T. F., Scheiner, C. W., Maier, L., and Voigt, K.-I. (2021). Investigating consumer acceptance of autonomous technologies: the case of self-driving automobiles. Eur. J. Innov. Manag. 24, 1210–1232. doi: 10.1108/EJIM-09-2019-0245
Baker, J., and Wakefield, K. L. (2012). How consumer shopping orientation influences perceived crowding, excitement, and stress at the mall. J. Acad. Mark. Sci. 40, 791–806. doi: 10.1007/s11747-011-0284-z
Bandura, A. (1977). Self-efficacy: toward a unifying theory of behavioral change. Psychol. Rev. 84:191.
Bandura, Albert . Guide for constructing self-efficacy scales, Self-efficacy beliefs of adolescents 5, (2006): 307–337
Beatty, S. E., and Elizabeth Ferrell, M. (1998). Impulse buying: modeling its precursors. J. Retail. 74, 169–191. doi: 10.1016/S0022-4359(99)80092-X
Bertoncini, A. L. C., and Serafim, M. C. (2023). Ethical content in artificial intelligence systems: a demand explained in three critical points. Front. Psychol. 14:1074787. doi: 10.3389/fpsyg.2023.1074787
BITNER, Mary Jo Servicescapes (1992). The impact of physical surroundings on customers and employees. J. Mark. 56:57. doi: 10.2307/1252042
Bogucka, E., Constantinides, M., Šćepanović, S., and Quercia, D. Co-designing an AI impact assessment report template with AI practitioners and AI compliance experts, arXiv preprint arXiv:2407.17374 (2024)
Brunette, Emma S., Flemmer, Rory C., and Flemmer, Claire L.. A review of artificial intelligence, 2009 4th international conference on autonomous robots and agents. IEEE, (2009)
Cao, Y., Li, S., Liu, Y., Yan, Z., Dai, Y., Yu, P. S., et al. A comprehensive survey of ai-generated content (aigc): A history of generative AI from Gan to chatgpt. arXiv 2023, arXiv preprint arXiv:2303.04226 (2023)
Chatterjee, S., and Kar, A. K. (2020). Why do small and medium enterprises use social media marketing and what is the impact: empirical insights from India. Int. J. Inf. Manag. 53:102103.
Chen, K.-Y., and Chang, M.-L. (2013). User acceptance of ‘near field communication’ mobile phone service: an investigation based on the ‘unified theory of acceptance and use of technology’ model. Serv. Ind. J. 33, 609–623. doi: 10.1080/02642069.2011.622369
Chen, X., Luo, L., Tang, F., Zhao, M., and Kato, N. (2024). AIGC-based evolvable digital twin networks: a road to the intelligent Metaverse. IEEE Netw. :1. doi: 10.1109/MNET.2024.3411008
Chen, A. N., McGaughey, R. E., Zeltmann, S. M., Lu, H. K., and Lee, M. R. (2018). How seniors in Taiwan use information technology: computer and cell phones. Int. J. Human–Comput. Interact. 34, 166–176. doi: 10.1080/10447318.2017.1335445
Chen, J., Yi, C., Du, H., Niyato, D., Kang, J., Shen, J. C. X., et al. A revolution of personalized healthcare: enabling human digital twin with mobile AIGC, (2023). arXiv preprint arXiv: 2307.12115
Chin, W. W. (1998). The partial least squares approach to structural equation modeling : Modern Methods for Business Research/Lawrence Erlbaum Associate.
Chinese State Council. New Generation Artificial Intelligence Development Plan [EB/OL] . (2017). Available at: https://www.gov.cn/zhengce/zhengceku/2017-07/20/content_5211996.htm (Accessed on 07 August 2024).
Choudrie, J., Pheeraphuttranghkoon, S., and Davari, S. (2020). The digital divide and older adult population adoption, use and diffusion of mobile phones: a quantitative study. Inf. Syst. Front. 22, 673–695. doi: 10.1007/s10796-018-9875-2
Cimperman, M., Brenčič, M. M., and Trkman, P. (2016). Analyzing older users’ home telehealth services acceptance behavior—applying an extended UTAUT model. Int. J. Med. Inform. 90, 22–31. doi: 10.1016/j.ijmedinf.2016.03.002
Compeau, D. R., and Higgins, C. A. (1995). Computer self-efficacy: development of a measure and initial test. MIS Q. 19, 189–211. doi: 10.2307/249688
Cong, L. (2024). A framework study on the application of AIGC technology in the digital reconstruction of cultural heritage. Appl. Math. Nonlinear Sci. 9:1. doi: 10.2478/amns-2024-2190
Davis, F. D. (1989). Perceived usefulness, perceived ease of use, and user acceptance of information technology. MIS Q. 13, 319–340. doi: 10.2307/249008
Ding, S., Qu, S., Xi, Y., and Wan, S. (2020). Stimulus-driven and concept-driven analysis for image caption generation. Neurocomputing 398, 520–530. doi: 10.1016/j.neucom.2019.04.095
DOAN, Thuy Thanh Thi (2021). The effect of perceived risk and technology self-efficacy on online learning intention: an empirical study in Vietnam. J. Asian Finance Econ. Business 8, 385–393. doi: 10.13106/jafeb.2021.vol8.no10.0385
Douglass, R. B. (1977). Belief, attitude, intention, and behavior: An introduction to theory and research, 130–132.
Du, D, Zhang, Y, and Ge, J. Effect of AI Generated Content Advertising on Consumer Engagement. In International conference on human-computer interaction. Springer Nature Switzerland: Cham (2023).
Du, Hongyang, et al., Enabling AI-generated content (AIGC) services in wireless edge networks. arXiv preprint arXiv:2301.03220 (2023).
Duong, C. D. (2023). Applying the stimulus-organism-response theory to investigate determinants of students’ social entrepreneurship: moderation role of perceived university support. Soc. Enterp. J. 19, 167–192. doi: 10.1108/SEJ-10-2022-0091
European Union . Europe fit for the digital age: Commission proposes new rules and actions for excellence and trust in artificial intelligence [EB/OL]. (2021). Available at: https://ec.europa.eu/commission/presscorner/detail/en/ip_21_1682 (Accessed on 07 August 2024).
Fornell, C., and Larcker, D. F. (1981a). Evaluating structural equation models with unobservable variables and measurement error. J. Mark. Res. 18, 39–50. doi: 10.1177/002224378101800104
Fornell, C., and Larcker, D. F. (1981b). Structural equation models with unobservable variables and measurement error: Algebra and statistics, 382–388.
Fraser, D. (2023). Who wrote this editorial? The use of artificial intelligence-generated content in nursing literature. Neonatal Netw. 42, 115–117. doi: 10.1891/NN-2023-0027
Freeman, Arthur, and Leaf, Russell C.. Cognitive therapy applied to personality disorders, Comprehensive Handbook of Cognitive Therapy (1989): 403–433
Fu, S., Chen, X., and Zheng, H. (2021). Exploring an adverse impact of smartphone overuse on academic performance via health issues: a stimulus-organism-response perspective. Behav. Inform. Technol. 40, 663–675. doi: 10.1080/0144929X.2020.1716848
Gaifutdinov, R. R., Khisamova, Z. I., Sidorenko, E. L., Efremova, M. A., Lopatina, T. M., and Kirpichnikov, D. V. (2020). Theoretical and legal bases of artificial intelligence punishment system development. Revista san gregorio 41, 159–164. doi: 10.36097/rsan.v1i41.1496
Habash, N. Y. (2010). Introduction to Arabic natural language processing : Morgan and Claypool Publishers. Available at: https://futurism.com/the-byte/chatgpts-fastest-growing-app-human-history
Harrison, M. (2023). ChatGPT’s explosive popularity makes it the fastest-growing app in human history. Byte. Available at: https://futurism.com/the-byte/chatgpts-fastest-growing-app-human-history
Hsiao, C.-H., and Tang, K.-Y. (2021). Who captures whom–Pokémon or tourists? A perspective of the stimulus-organism-response model. Int. J. Inf. Manag. 61:102312. doi: 10.1016/j.ijinfomgt.2021.102312
Hsu, C.-W., and Peng, C.-C. (2022). What drives older adults’ use of mobile registration apps in Taiwan? An investigation using the extended UTAUT model. Inform. Health Soc. Care 47, 258–273. doi: 10.1080/17538157.2021.1990299
Huang, Li-Ting . Exploring consumers’ intention to urge to buy in mobile commerce: The perspective of pleasure-arousal-dominance. (2017). doi: 10.1016/j.tsc.2024.101508
Huang, T. (2023). Using SOR framework to explore the driving factors of older adults smartphone use behavior. Human. Soc. Sci. Commun. 10:1.
Huang, K.-L., Liu, Y.-c., and Dong, M.-Q. (2024). Incorporating AIGC into design ideation: a study on self-efficacy and learning experience acceptance under higher-order thinking. Think. Skills Creat. 52:101508.
Huang, K.-L., Liu, Y.-C., Dong, M.-Q., and Lu, C.-C. (2024). Integrating AIGC into product design ideation teaching: an empirical study on self-efficacy and learning outcomes. Learn. Instr. 92:101929. doi: 10.1016/j.learninstruc.2024.101929
Ingtyas, Fatma Tresno, Hilda, Nuwairi, and Handayani, Nila. Development of a “product design” guidelines book based on Indonesia National Curriculum Framework (KKNI) to develop creative industries students of education Department of Family Welfare State University of Medan. ACEIVE 2018: Proceedings of the 2nd annual conference of engineering and implementation on vocational education (ACEIVE 2018), 3rd November 2018, North Sumatra, Indonesia. European Alliance for Innovation, (2019)
Jacoby, J. (2002). Stimulus-organism-response reconsidered: an evolutionary step in modeling (consumer) behavior. J. Consum. Psychol. 12, 51–57. doi: 10.1207/S15327663JCP1201_05
Jiatong, W., Murad, M., Li, C., Gill, S. A., and Ashraf, S. F. (2021). Linking cognitive flexibility to entrepreneurial alertness and entrepreneurial intention among medical students with the moderating role of entrepreneurial self-efficacy: a second-order moderated mediation model. PLoS One 16:e0256420. doi: 10.1371/journal.pone.0256420
Kazlauskaite, A., Martínez-Torres, R. J., Wilkie, S., Kumar, A., Peltier, J., Gonzalez, A., et al. (2015). Binding to serine 65-phosphorylated ubiquitin primes Parkin for optimal PINK 1-dependent phosphorylation and activation. EMBO Rep. 16, 939–954. doi: 10.15252/embr.201540352
Kim, T. B., and Ho, C.-T. B. (2021). Validating the moderating role of age in multi-perspective acceptance model of wearable healthcare technology. Telematics Inform. 61:101603.
Kim, H., and Lennon, S. J. (2010). E-atmosphere, emotional, cognitive, and behavioral responses. J. Fashion Market. Manag. Int. J. 14, 412–428. doi: 10.1108/13612021011061861
Koo, D.-M., and Seon-Hee, J. (2010). The interactional effects of atmospherics and perceptual curiosity on emotions and online shopping intention. Comput. Hum. Behav. 26, 377–388. doi: 10.1016/j.chb.2009.11.009
Nelson Laird, T. F., Shoup, R., Kuh, G. D., and Schwarz, M. J.. (2008). The effects of discipline on deep approaches to student learning and college outcomes. Res. High. Educ. 49, 469–494. doi: 10.1007/s11162-008-9088-5
Li, J., and Liu, Q. (2024). Application of generative artificial intelligence AIGC technology under neural network algorithm in game character art design. J. Knowl. Econ., 14702, 1–32. doi: 10.1007/s13132-024-02152-z
Liao, W., Liu, Z., Dai, H., Xu, S., Wu, Z., Zhang, Y., et al. Differentiate ChatGPT-generated and human-written medical texts. arXiv, (2023), arXiv preprint arXiv:2304.11567
Lin, Yonghui, and Liu, Hailin. The impact of artificial intelligence generated content driven graphic design tools on creative thinking of designers. International Conference on Human-Computer Interaction. Cham: Springer Nature Switzerland, (2024)
Lindwall, A., Dordlofva, C., Öhrwall Rönnbäck, A., and Törlind, P. (2022). Innovation in a box: exploring creativity in design for additive manufacturing in a regulated industry. J. Eng. Des. 33, 567–586. doi: 10.1080/09544828.2022.2139967
Lou, Y. (2023). Human creativity in the AIGC era. She Ji J. Design Econ. Innov. 9, 541–552. doi: 10.1016/j.sheji.2024.02.002
Lv, X., Liu, Y., Luo, J., Liu, Y., and Li, C. (2021). Does a cute artificial intelligence assistant soften the blow? The impact of cuteness on customer tolerance of assistant service failure. Ann. Tour. Res. 87:103114. doi: 10.1016/j.annals.2020.103114
Ma, Q., Chan, A. H. S., and Chen, K. (2016). Personal and other factors affecting acceptance of smartphone technology by older Chinese adults. Appl. Ergon. 54, 62–71. doi: 10.1016/j.apergo.2015.11.015
Mallick, R., Flathmann, C., Lancaster, C., Hauptman, A., McNeese, N., and Freeman, G. (2023). The pursuit of happiness: the power and influence of AI teammate emotion in human-AI teamwork. Behav. Inform. Technol., 1–25. doi: 10.1080/0144929X.2023.2277909
Mariani, M. M., Perez-Vega, R., and Wirtz, J. (2022). AI in marketing, consumer research and psychology: a systematic literature review and research agenda. Psychol. Mark. 39, 755–776. doi: 10.1002/mar.21619
McCarthy, J., Minsky, M. L., Rochester, N., and Shannon, C. E. (2006). A proposal for the Dartmouth summer research project on artificial intelligence, August 31, 1955. AI Mag. 27:12.
Mehrabian, A. (1974). An approach to environmental psychology : Massachusetts Institute of Technology.
Meron, Yaron , Graphic design and artificial intelligence: Interdisciplinary challenges for designers in the search for research collaboration, (2022)
National Institute of Standards and Technology . AI Risk Management Framework [EB/OL]. (2024). Available at: https://www.nist.gov/itl/ai-risk-management-framework (Accessed on 07 August 2024).
Park, S., Cha, H.-S., and Im, C.-H. (2019). Development of an online home appliance control system using augmented reality and an SSVEP-based brain–computer interface. IEEE Access 7, 163604–163614. doi: 10.1109/ACCESS.2019.2952613
Purnomo, S. H., and Lee, Y.-H. (2013). E-learning adoption in the banking workplace in Indonesia: an empirical study. Inf. Dev. 29, 138–153. doi: 10.1177/0266666912448258
Qin, Hua Xuan, and Hui, Pan, Empowering the metaverse with generative AI: survey and future directions. 2023 IEEE 43rd international conference on distributed computing systems workshops (ICDCSW). HongKong: IEEE, (2023).
Qiu, H., Wang, X., Wu, M.-Y., Wei, W., Morrison, A. M., and Kelly, C. (2023). The effect of destination source credibility on tourist environmentally responsible behavior: an application of stimulus-organism-response theory. J. Sustain. Tour. 31, 1797–1817. doi: 10.1080/09669582.2022.2067167
Rafique, H., Almagrabi, A. O., Shamim, A., Anwar, F., and Bashir, A. K. (2020). Investigating the acceptance of mobile library applications with an extended technology acceptance model (TAM). Comput. Educ. 145:103732. doi: 10.1016/j.compedu.2019.103732
Ren, X., Tong, L., Zeng, J., and Zhang, C. (2023). AIGC scenario analysis and research on technology roadmap of internet industry application. China Commun. 20, 292–304. doi: 10.23919/JCC.fa.2023-0359.202310
Russell, J. A., and Mehrabian, A. (1974). Distinguishing anger and anxiety in terms of emotional response factors. J. Consult. Clin. Psychol. 42:79.
Sagnier, C., Loup-Escande, E, Lourdeaux, D., Thouvenin, I., and Valléry, G. (2020). User acceptance of virtual reality: an extended technology acceptance model. Int. J. Human–Comput. Inter. 36, 993–1007. doi: 10.1080/10447318.2019.1708612
Salloum, S. A., al-Emran, M., Shaalan, K., and Tarhini, A. (2019). Factors affecting the E-learning acceptance: a case study from UAE. Educ. Inf. Technol. 24, 509–530. doi: 10.1007/s10639-018-9786-3
Salloum, S. A., Qasim Mohammad Alhamad, A., al-Emran, M., Abdel Monem, A., and Shaalan, K. (2019). Exploring students’ acceptance of e-learning through the development of a comprehensive technology acceptance model. IEEE access 7, 128445–128462. doi: 10.1109/ACCESS.2019.2939467
Sampat, B., Raj, S., Behl, A., and Schöbel, S. (2022). An empirical analysis of facilitators and barriers to the hybrid work model: a cross-cultural and multi-theoretical approach. Pers. Rev. 51, 1990–2020. doi: 10.1108/PR-02-2022-0176
Schönemann, P. H. (1985). On artificial intelligence. Behav. Brain Sci. 8, 241–242. doi: 10.1017/S0140525X0002063X
Shao, L., Chen, B., Zhang, Z., Zhang, Z., and Chen, X. (2024). Artificial intelligence generated content (AIGC) in medicine: a narrative review. Math. Biosci. Eng. 21, 1672–1711. doi: 10.3934/mbe.2024073
Sherman, E., Mathur, A., and Smith, R. B. (1997). Store environment and consumer purchase behavior: mediating role of consumer emotions. Psychol. Mark. 14, 361–378. doi: 10.1002/(SICI)1520-6793(199707)14:4<361
Sohn, K., and Kwon, O. (2020). Technology acceptance theories and factors influencing artificial intelligence-based intelligent products. Telematics Inform. 47:101324. doi: 10.1016/j.tele.2019.101324
Stephanopoulos, G. (1990). Artificial intelligence in process engineering—current state and future trends. Comput. Chem. Eng. 14, 1259–1270. doi: 10.1016/0098-1354(90)80006-W
Sultan, P., Wong, H. Y., and Azam, M. S. (2021). How perceived communication source and food value stimulate purchase intention of organic food: an examination of the stimulus-organism-response (SOR) model. J. Clean. Prod. 312:127807. doi: 10.1016/j.jclepro.2021.127807
Sun, J., Chen, P.-J., Ren, L., Shih, E. H.-W., Ma, C., Wang, H., et al. (2021). Place attachment to pseudo establishments: an application of the stimulus-organism-response paradigm to themed hotels. J. Bus. Res. 129, 484–494. doi: 10.1016/j.jbusres.2020.10.005
Talwar, S., Kaur, P., Nunkoo, R., and Dhir, A. (2023). Digitalization and sustainability: virtual reality tourism in a post pandemic world. J. Sustain. Tour. 31, 2564–2591. doi: 10.1080/09669582.2022.2029870
Talwar, M., Talwar, S., Kaur, P., Islam, A. K. M. N., and Dhir, A. (2021). Positive and negative word of mouth (WOM) are not necessarily opposites: a reappraisal using the dual factor theory. J. Retail. Consum. Serv. 63:102396. doi: 10.1016/j.jretconser.2020.102396
Tandon, A., Jabeen, F., Talwar, S., Sakashita, M., and Dhir, A. (2021). Facilitators and inhibitors of organic food buying behavior. Food Qual. Prefer. 88:104077. doi: 10.1016/j.foodqual.2020.104077
Tao, W., Gao, S., and Yuan, Y. (2023). Boundary crossing: an experimental study of individual perceptions toward AIGC. Front. Psychol. 14:1185880. doi: 10.3389/fpsyg.2023.1185880
Ullah, N., Mugahed al-Rahmi, W., Alzahrani, A. I., Alfarraj, O., and Alblehai, F. M. (2021). Blockchain technology adoption in smart learning environments. Sustain. For. 13:1801. doi: 10.3390/su13041801
Venkatesh, V., Thong, J. Y. L., and Xin, X. (2012). Consumer acceptance and use of information technology: extending the unified theory of acceptance and use of technology. MIS Q. 36, 157–178. doi: 10.2307/41410412
Verhagen, T., and Van Dolen, W. (2011). The influence of online store beliefs on consumer online impulse buying: a model and empirical application. Inf. Manag. 48, 320–327. doi: 10.1016/j.im.2011.08.001
Verma, P. (2020). The effect of presentation, product availability and ease upon transaction reliability for online food delivery aggregator applications—moderated mediated model. J. Foodserv. Bus. Res. 23, 285–304. doi: 10.1080/15378020.2020.1761586
Wang, S.-F., and Chen, C.-C. (2024). Exploring designer Trust in Artificial Intelligence-Generated Content: TAM/TPB model study. Appl. Sci. 14:6902. doi: 10.3390/app14166902
Wang, S., Fan, J., Zhao, D., Yang, S., and Fu, Y. (2016). Predicting consumers’ intention to adopt hybrid electric vehicles: using an extended version of the theory of planned behavior model. Transportation 43, 123–143. doi: 10.1007/s11116-014-9567-9
Warburton, K. (2003). Deep learning and education for sustainability. Int. J. Sustain. High. Educ. 4, 44–56. doi: 10.1108/14676370310455332
Wei, Yiluo, and Tyson, Gareth. Understanding the impact of AI generated content on social media: The Pixiv case. arXiv preprint arXiv:2402.18463 (2024)
What drives brand love for natural products? The moderating role of household size J. Retail Consum. Serv. (2021).
Woodworth, R. H. (1929). Cytological studies in the Betulaceae. I. Betula. Bot. Gaz. 87, 331–363. doi: 10.1086/333945
Wu, F., Hsiao, S.-W., and Peng, L. (2024). An AIGC-empowered methodology to product color matching design. Displays 81:102623. doi: 10.1016/j.displa.2023.102623
Wu, Zhuohao, Tang, Ritong, Wang, Ganyu, Li, Hailing, Yang, Sibo, and Shidujaman, Mohammad, The research and design of an AIGC empowered fashion design product, International Conference on Human-Computer Interaction. Cham: Springer Nature Switzerland, (2024)
Wu, S., Wong, I. K. A., and Lin, Z. C. J. (2021). Understanding the role of atmospheric cues of travel apps: a synthesis between media richness and stimulus–organism–response theory. J. Hosp. Tour. Manag. 49, 226–234. doi: 10.1016/j.jhtm.2021.09.014
Yadav, S. (2020). Deep learning based safe social distancing and face mask detection in public areas for covid-19 safety guidelines adherence. Int. J. Res. Appl. Sci. Eng. Technol. 8, 1368–1375. doi: 10.22214/ijraset.2020.30560
Yin, H., Zhang, Z., and Liu, Y. (2023). The exploration of integrating the midjourney artificial intelligence generated content tool into design systems to direct designers towards future-oriented innovation. Systems 11:566. doi: 10.3390/systems11120566
Yu, Hanjie, Dong, Yan, and Wu, Qiong. User-centric AIGC products: Explainable artificial intelligence and AIGC products. arXiv preprint arXiv:2308.09877 (2023).
Yuan, Lin . Research on cultural and creative design industry under the background of computer internet. J. Phys. Conf. Ser.. 1648. IOP Publishing, (2020) 566. doi: 10.1088/1742-6596/1648/2/022154
Zhang, G., Yue, X., Ye, Y., and Peng, M. Y.-P. (2021). Understanding the impact of the psychological cognitive process on student learning satisfaction: combination of the social cognitive career theory and SOR model. Front. Psychol. 12:712323. doi: 10.3389/fpsyg.2021.712323
Keywords: AIGC, SOR, TAM, TPB, designer, usage behavior
Citation: Wang S-F and Chen C-C (2024) Explore the driving factors of designers’ AIGC usage behavior based on SOR framework. Front. Comput. Sci. 6:1417016. doi: 10.3389/fcomp.2024.1417016
Edited by:
Xiang Li, Harvard Medical School, United StatesReviewed by:
Peng Shu, University of Georgia, United StatesJiqiao Lu, Hong Kong Polytechnic University, Hong Kong SAR, China
Copyright © 2024 Wang and Chen. This is an open-access article distributed under the terms of the Creative Commons Attribution License (CC BY). The use, distribution or reproduction in other forums is permitted, provided the original author(s) and the copyright owner(s) are credited and that the original publication in this journal is cited, in accordance with accepted academic practice. No use, distribution or reproduction is permitted which does not comply with these terms.
*Correspondence: Shao-Feng Wang, t09302@fzu.edu.cn