- 1Research Center in Information and Communication Technologies, University of Costa Rica, San José, Costa Rica
- 2Department of Computer Technology and Computation, University of Alicante, Alicante, Spain
In the dynamic landscape of modern agriculture, integrating technology holds immense potential to enhance efficiency and productivity for small-scale farmers. This study presents a user-centric evaluation of an intelligent context-aware alert system, tailored for small-scale greenhouse farming. We employed standardized questionnaires, including the NASA Task Load Index and the User Experience Questionnaire, to assess the system's perceived utility, mental workload, and overall user experience. Our findings reveal the high perceived utility of the system among farmers. Farmers participating in the assessment indicated a strong intention to utilize the system for crop monitoring. Moreover, the system demonstrated a moderate mental workload, suggesting ease of use and potential acceptance by users. Our evaluation highlighted an excellent user experience, with scores ranging from very good to extremely good across all dimensions. Furthermore, user preferences for alert mechanisms underscored the importance of adaptable notifications, with voice and text alerts favored for comprehensive information dissemination. Light and voice alerts were preferred during manual tasks. This study highlights the significance of user-centered design in agricultural technology, offering insights to enhance the usability and the adoption of alert systems in small-scale farming environments. The positive reception of the system's utility and the moderate mental workload suggest that such technology can be readily adopted by farmers, thereby improving monitoring and management practices in greenhouse farming. The preference for adaptable alert mechanisms further emphasizes the need for flexible and context-sensitive solutions in agricultural technology.
1 Introduction
In the domain of agricultural technology, the importance of designing solutions tailored for farmers cannot be overstated. While technological solutions that automate farming activities have primarily been developed to assist large-scale farming installations, it is essential to acknowledge the unique needs of small-scale farming operations. These smaller installations often require more targeted technological development to address their specific challenges and enhance efficiency (Norman and Hays, 1979; Dhillon and Moncur, 2023). Therefore, focusing on user-centric design principles from the outset becomes even more crucial for ensuring that technological solutions effectively meet the needs of small-scale farmers.
The agricultural workforce faces significant demographic shifts characterized by an increasing proportion of aging individuals. Studies indicate a notable trend toward an older farming population, with a substantial portion of agricultural labor being carried out by individuals over the age of 50 (Satola, 2019; Akdemir et al., 2021; Liu et al., 2021). This aged workforce also suffers from low scholarship and limited technological capabilities. For these reasons, it is imperative to develop user-friendly and accessible technological innovations that meet the evolving demands of modern agricultural practices and resonate with the unique needs and capabilities of this aging demographic, becoming usable for them.
This paper focuses on the usability assessment of a greenhouse context-aware alert system specifically designed for small-scale farmers. The overarching objective is twofold: first, to ensure that technological advancements are not only technologically sophisticated but also practical and user-friendly for individuals engaged in agricultural activities, including those in small-scale farming operations; and second, to address the needs of an elderly farming population with limited technological proficiency, by developing solutions that are intuitive and accessible to individuals of varying levels of technological skills.
The evaluated greenhouse alert system forms part of an innovative smart farming platform, representing a tangible step toward fulfilling these objectives. Leveraging advancements in sensor technology, data analytics, and artificial intelligence-based decision-making, the system is equipped with real-time monitoring capabilities to detect environmental changes and potential risks to crops within greenhouse settings. To achieve this, the smart farming solution also incorporates expert knowledge provided by agronomists. By delivering timely alerts and notifications using distinct delivery mechanisms, the system aims to provide farmers with the information they need to make informed decisions and mitigate potential risks to crop yields and quality.
To gauge the system's effectiveness and suitability for small-scale farming operations, we employ a rigorous evaluation process, drawing upon established instruments such as the User Experience Questionnaire (UEQ)1 and the NASA Task Load Index (NASA-TLX).2 These standardized questionnaires enable us to assess key metrics such as perceived utility, mental workload, and overall user experience, providing valuable insights into the system's usability and practicality from the perspective of end-users.
In the next section, we delve into research conducted by other authors, clearing their contributions in creating and evaluating monitoring and alert solutions for smart agriculture.
2 Related work
The enhancement of technological solutions to bolster farming activities has become increasingly vital in recent years. Leveraging emerging technologies such as the Internet of Things (IoT), Wireless Sensor Networks (WSN), Artificial Intelligence (AI), and Big Data Analysis has paved the way for the creation of innovative, intelligent, and robust solutions designed specifically to solve agricultural needs (Khan et al., 2021).
Within the expansive domain of agricultural innovation, numerous research endeavors have delved into developing monitoring, control, and alerting systems designed for smart farming. However, it is noteworthy that a significant portion of these proposed solutions lack rigorous evaluation processes. Table 1 shows some literature reviews in which authors analyzed numerous proposals of Decision Support Systems (DSS) for smart farming. Table 1 also states some remarks about the reported evaluation.
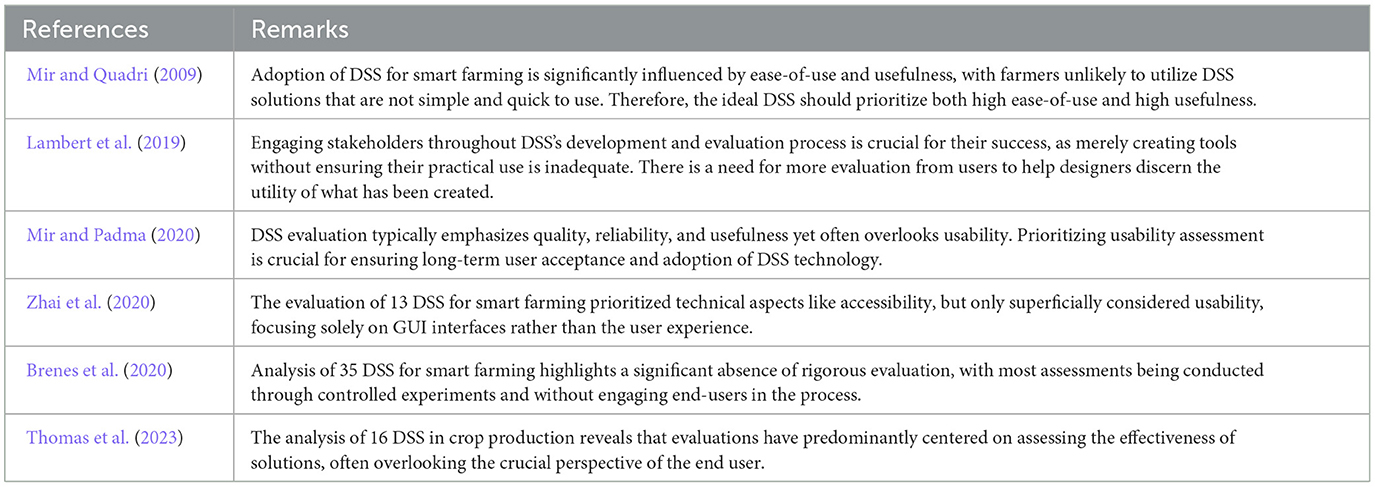
Table 1. Literature reviews that unveil a lack of evaluation of technological solutions for smart farming.
As illustrated in Table 1, there is a conspicuous absence of evaluations centered on end-users, raising concerns about these systems' practical applicability and effectiveness in real-world farming scenarios. This gap highlights the critical need for comprehensive assessments involving end-users to ensure that innovations not only meet theoretical standards but also address the practical needs and challenges faced by the farming community. Nevertheless, some studies do address usability assessments.
Authors in Santoso et al. (2018) present an evaluation centered on the usability of the smart farming system. In this study, 58 participants, including system administrators, farmers, and general users, analyzed the usability of system functionality for each user profile. The researchers gathered a set of recommendations from the participants for enhancing the system. Moreover, the results indicate that the various assessments carried out achieved high percentages of usability. However, the assessment conducted in this case primarily focused solely on the interface's usability, overlooking users' perceptions. Finding studies where researchers analyze tools from the user's perspective is challenging. Few studies prioritize this type of evaluation, focusing on aspects that directly impact user experience. A review conducted in 2023 highlighted technological barriers hindering technology adoption in the agricultural sector. These barriers include the complexity of use, lack of suitability to farmers' needs, increased workload, and perceptions of technology (Thomas et al., 2023). All of these barriers are intricately linked to the user experience. Therefore, conducting user-centric assessments, such as the one presented in this paper, may be crucial for addressing these challenges and ensuring the effective adoption of agricultural technologies.
Prioritizing user experience from the initial stages of solution development can yield significant benefits. In a study by Novák et al. (2019), the authors introduce a series of tools that can effectively evaluate systems at various stages of development. They argue that adopting a user-centric design approach for technological solutions can address many potential challenges. Moreover, while these tools are particularly useful for assessing systems during development, they can also be valuable for evaluating developed and deployed smart farming systems. These tools include questionnaire surveys, remote usability testing, deep-dive interviews, and laboratory usability testing.
Furthermore, given that previous evaluations of the alert greenhouse system have primarily concentrated on the effectiveness of the alerts delivered to farmers. This study focuses on evaluating the system from the user's perspective. This includes assessing the perceived utility, mental workload, and user experience when utilizing the alert system using some of the tools suggested by Novák et al. (2019).
Next, we present all the details of the conducted assessment on the greenhouse alert system for small-scale farmers.
3 Materials and methods
In this section, we present the greenhouse alert system that we are evaluating. First, we contextualize the scenario in which the proposed system can be deployed. We present the system modules and functionalities. Next, we detail the evaluation methodology followed to carry out the user-centric assessment of the system.
3.1 Context of use of the smart farming solution
A low-cost smart farming solution was developed. The solution empowers small-scale farmers by offering a comprehensive system that integrates sensors placed strategically throughout the crop field while controlling distinct actuators. Figure 1 shows the deployment of intelligent monitoring stations in crop fields. The figure also shows the visualization dashboards provided in a web application that enables farmers to check data collected from crops. The right section of Figure 1 shows the alert delivery mechanisms and devices used in the smart farming solution, detailed below. The architecture of the smart farming solution is presented in Brenes and Marín-Raventós (2024).
Figure 2 illustrates the various subsystems comprising the smart farming solution. Each subsystem leverages the capabilities of the Internet of Things, Artificial Intelligence, and Data Analytics to deliver valuable information to farmers. Since greenhouse environments demand meticulous monitoring and control to ensure the wellbeing and productivity of crops, the smart farming solution uses monitoring stations deployed in the field for real-time data acquisition. To do that, monitoring stations are equipped with sensors that measure parameters such as temperature, humidity, soil moisture, and light intensity (Brenes et al., 2019). These parameters are then processed in the intelligent farming solution to provide actionable insights, allowing farmers to make informed decisions about resource allocation, irrigation, and climate control.
Moreover, the system does not solely focus on data collection but extends to activating actuators, such as irrigation systems, ventilation fans, and shading mechanisms. This level of control empowers farmers to respond promptly to changing environmental conditions and enact preventative measures, enhancing crop yield and minimizing resource wastage. The system strives to streamline the farming process and maximize resource efficiency by offering enhanced control, automation, and data-driven decision support (Ferrández-Pastor et al., 2023). In addition, the platform can also get information from external sources related to situations that could affect the crops, like weather forecasts and disease-plagues warnings from government services and in-site AI-guided disease-plagues detection.
Recognizing the criticality of monitoring greenhouse activities in real-time, the system is equipped with a context-aware alert subsystem (Brenes et al., 2023). This sophisticated alert mechanism ensures that farmers receive timely notifications when events occur within the greenhouse. By providing instantaneous alerts, farmers gain immediate insights into the status of their crops, facilitating prompt action and informed decision-making.
As an innovative feature, the alert system employs a variety of notification mechanisms, including voice, light, sound, and text, to disseminate alerts to users. This diverse array of devices ensures users receive real-time alerts tailored to their locations, enhancing their awareness of crop conditions. Moreover, users can customize their notification preferences within the smart farming system. This includes configuring the notification mechanisms they prefer, establishing the schedule for receiving alerts and selecting the alerts they wish to trigger. Such customization options empower users to seamlessly integrate the alert system into their workflows, optimizing its utility and effectiveness in supporting their agricultural endeavors.
In developing the alert system, agronomists have contributed their expertise to identify the key variables and agronomic indicators essential for triggering alerts. This collaborative effort ensures the system is finely tuned to suit farmers' unique requirements, enabling even those without technical expertise to utilize it effectively. Moreover, farmers have the autonomy to configure their alerts within the system, tailoring it to their specific needs and preferences. This flexibility empowers farmers to obtain maximum benefit from using the system, enhancing its utility and relevance in agricultural practices. Furthermore, this collaborative approach extends to the customization options available to users, ensuring seamless integration into their daily activities.
In this paper, our primary focus lies in evaluating the alert delivery component of the smart farming solution. Employing a user-centric approach, we assess the perceived utility, cognitive load, and overall user experience associated with utilizing the system. We present the methodology used to evaluate the greenhouse alert system.
3.2 Evaluation of the greenhouse alert system
We structured the evaluation of the greenhouse alert system into two distinct phases. Initially, our focus was on understanding farmers' anecdotal experiences and gauging the perceived utility of the alert system. Subsequently, we analyzed the user task load and overall user experience during system usage. In the following sections, we delineate the methodology employed for these evaluations.
3.2.1 Anecdotal situations and perceived utility evaluation
Recognizing the significance of understanding farmers' real-world scenarios where an alert system could prove beneficial, we developed a custom expert-validated survey to gather anecdotal insights. We aimed to compile a comprehensive list of these scenarios by engaging with farmers. Participants were introduced to the system during this survey, enabling us to gather their impressions of its features and perceived utility. In addition to assessing perceived utility, we sought to ascertain participants' intentions regarding system usage and whether they would recommend it to others. We used a ten-score scale for these three items and asked for justifications. Table 2 shows the items of the survey.
To apply the survey, we visited a farmer's fair, a weekend street market where farmers sell their products directly to consumers. There, we voluntarily included farmers in the survey. The application was done by interviewing farmers while they were attending to clients. The instrument was applied on paper and was tabulated afterward for analysis.
The methodology for the second evaluation phase is explained in the next section.
3.2.2 Mental workload and user experience evaluation
Regarding the usability of the alert system, we conducted a second evaluation in which we asked potential users to evaluate the system. To select participants, we enlisted the assistance of individuals walking throughout our university campus. To carry out the assessment, we prepared an evaluation setup in which we put the devices that deliver the alerts. To collect data, we used standardized questionnaires widely employed in the industry to evaluate usability and user experience. Additionally, the collected data were validated and analyzed according to the predetermined procedures specified in the questionnaires.
We also used the Think-Aloud protocol (van Someren, 1994). In this technique, the participant had to speak and tell everything s/he was thinking and doing while the researcher took notes.
Two different assessments were part of this evaluation phase. The first assessment was a Task Load Evaluation. We used the NASA Task Load Index (NASA-TLX) instrument to execute it. After completing a task, we collected data from the participants to analyze the cognitive charge and general workload when using the system. The second assessment was a User Experience Evaluation. In this case, we used the User Experience Questionnaire (UEQ) to collect data about the interaction of the participants with the alert system.
We designed a factorial experiment compounded by two factors with three levels, each one. The first factor was the alert delivery mechanism; the levels were light+sound, voice, or text. The second factor was the user's location when the alert was received, which had three levels: at home, at the greenhouse, or another place (away).
Using the use scenarios collected from the first evaluation with farmers, we designed nine scenarios in which the alert system could be used. These scenarios reflect potential real-life situations. The scenarios ask participants to do actions such as reading a newspaper, simulating an inventory at the greenhouse, or visiting a relative, among others. Participants were provided with materials to execute the activities requested in each scenario.
We introduced the smart farming solution and the greenhouse alert system to the participants at the beginning of the experiment. Then, we ask participants to complete the weighing phase of the NASA-TLX instrument. We randomly selected four to five scenarios for each participant in the evaluation. Next, for each of the selected scenarios, we asked the participant to complete the detailed actions, activating the alerts and recording the times of each activity.
After completing the task load evaluation for the four or five scenarios, we present the UEQ questionnaire to the participants and ask them to complete it. After completing the UEQ instrument, we ask participants to characterize the system globally using three distinct words or phrases.
We executed the experiment with 22 participants. This way, we obtained 108 results, where each scenario was evaluated 12 times, and each alert delivery mechanism and the location were evaluated 36 times individually.
It is important to note that, according to national regulations in the country where the study was conducted, the involvement of a bioethics committee in the creation and execution of data collection instruments is not required in this type of study, as its focus is on the interaction with technological solutions rather than on the individuals themselves. Similarly, signing an informed consent form for participation is regarded as not necessary. However, all participants were provided with a detailed description of the research objectives and the handling of the collected data. Furthermore, participation in this study was entirely voluntary.
We present the results from the executed evaluations in the next section.
4 Results
This section unveils the outcomes derived from evaluating the alert system. We organize the results according to the two distinct evaluation phases.
4.1 Anecdotal situations and perceived utility evaluation results
In the first evaluation phase, we surveyed the perceived utility of the alert system for farmers. Also, we measured the intention of use and recommendation probability of the system by the participants. We applied the survey to 30 farmers, where seven were females, and 23 were males. Figure 3 shows the age distribution of the survey participants. As can be seen in the figure, most participants are between 37 and 69 years old, which aligns with the age range for farmers worldwide according to a study carried out by the International Fund for Agricultural Development (IFAD, 2019).
Figure 4 shows the distribution of the answers received from farmers regarding perceived utility, the intention of use, and the probability of recommending the greenhouse alert system. About the first metric, fifteen farmers stated that they perceived the alert system to have a high utility, while nine said they should use it first for a clearer viewpoint. Two farmers considered the system a new technological tool, highlighting the relevance and current need for innovation in the productive sector. Meanwhile, two of them did not see benefits for their activities. Finally, two farmers did not provide more details to this first question.
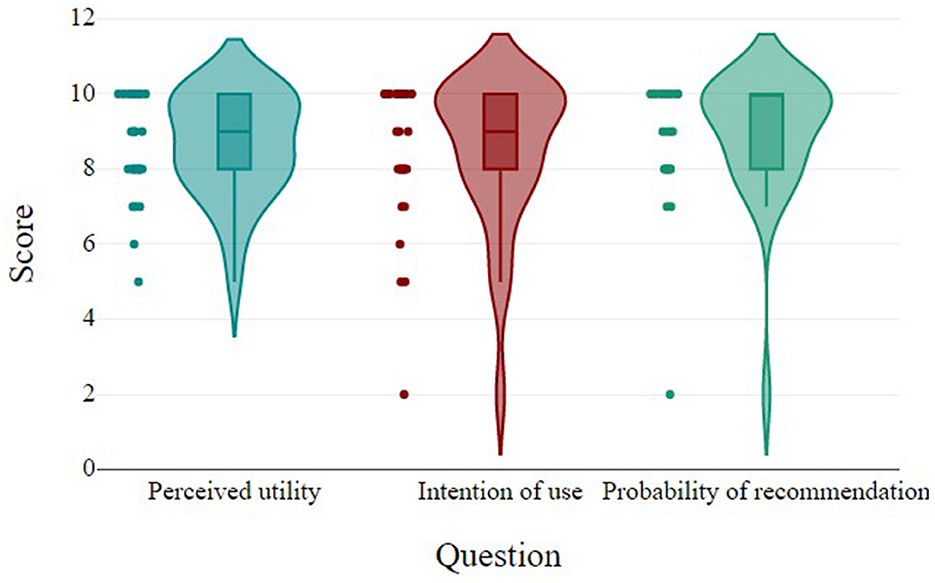
Figure 4. First evaluation scores distribution for perceived utility, intention of use, and probability of recommendation.
Regarding the intention of use, scores were high in most cases. However, some farmers took a more reserved position. While ten farmers stated they would use the proposed system, another seven indicated that costs would determine their real possible use. Interestingly, three farmers indicated that they would use the proposed system, but they would require help to do it. In this case, two farmers noted that they would not use the system because they did not see it useful for their activities. Eight farmers did not provide more information for this question.
Similarly to the intention of use, regarding recommendation probability, eighteen farmers established, as a condition, that they must use the solution before recommending it to other people. Meanwhile, five farmers said they would recommend the proposed system since they see it useful. In this case, one farmer indicated that s/he would not recommend the system. A total of six farmers did not provide details for this question.
We also sought feedback from farmers regarding potential improvements and suggestions for enhancing the alert system. Interestingly, one recurring suggestion was the need for specialized training, particularly for elderly users, to optimize their system usage. We found this insight valuable, especially as we conducted our assessment with the aim of enhancing the system's usability for all users. Additionally, farmers expressed the desire for the system to offer technical information adapted to the specific crops being cultivated, indicating a need for more detailed insights. Another noteworthy suggestion pertained to the nighttime usage of the system. Farmers highlighted the potential usefulness of receiving alerts about crop pest detection during nighttime. They stated that visibility is limited at night, and identifying crop problems becomes challenging.
In general, the alert system was highly valued by farmers. The results related to the second evaluation phase are described next.
4.2 Mental workload and user experience results
As stated in the methodology description section, we evaluated several aspects simultaneously in this evaluation phase. First, we conduct a mental workload evaluation to measure the users' mental workload when they use the system. Next, we conducted a user experience evaluation to measure the user's interaction with the system.
We executed this evaluation phase with a total of 22 participants. Most of the participants were under 32 years old. Meanwhile, only five are over that age (33, 34, 36, 45, and 71 specifically). This occurred because, despite having selected the participants randomly, we executed the study on the university campus, where potential participants are typically younger. This limitation was outweighed by the possibility of using a controlled lab setting.
Regarding gender, 14 males (64%) and eight females (36%) participated in this evaluation. To evaluate the system, four to five distinct scenarios were randomly assigned to participants to execute the assessments. We enforce a similar number of evaluations per scenario to ease the comparison of the obtained data. To do that, we registered the scenarios' IDs for each participant and controlled their distribution between them. This way, each scenario was evaluated 12 times by distinct participants.
Figure 5 shows the randomized distribution of scenarios. The last two participants only evaluated four scenarios to keep the number of assessments per scenario at twelve. The randomization made to the scenarios assigned to the participants can be seen in the Figure 5.
We applied the NASA-TLX standardized questionnaire (Hart and Staveland, 1988) for the metal workload evaluation. This instrument can be used to measure the mental workload associated with the execution of a task. The measurement is made based on six dimensions: mental demands, physical demands, temporal demands, performance, effort, and frustration level. The mental demands refer to the cognitive and perceptive activity required to execute a task. Physical demands are associated with the physical activity needed to complete a task. The temporal demands refer to the temporary pressure level perceived during task execution. The performance is related to the degree of satisfaction with the achieved performance. The effort corresponds to the combination of physical and mental effort required to complete a task, earning the degree of performance perceived. Finally, the frustration level refers to the degree of insecurity, stress, and irritation felt during the task execution.
We used the assigned fixed number of scenarios per participant to evaluate the system. Participants had to execute the instructions detailed in the scenario. We provided them with the materials to complete the distinct sub-tasks detailed in the scenarios. At the end of the scenario execution, we asked participants to fill out a form containing the six dimensions of the assessments and a scale from 1 to 20 to evaluate the mental workload of each scenario.
Following the instructions provided with the evaluation instrument (Hart and Staveland, 1988), we asked participants to weigh the dimensions of the NASA-TLX to determine which dimension was more relevant for them. The results reveal that, in general, the most relevant dimension was Frustration level (mean = 4), followed by Effort (mean = 3). This is interesting, considering the system's objective is to provide information about the alerts even when the user is doing other activities. Therefore, mental and temporal demands are the two most important dimensions to measure the proposed system's mental workload.
After the weighing and score phase, we calculated the mental workload scores. These scores were obtained by applying the formula in Equation (1).
It is important to consider that there are several ways to calculate the mental workload score for a task based on the NASA-TLX instrument. So, we decided to follow the specifications detailed by the Ministry of Labor and Social Welfare of the Chilean government (Ministerio del Trabajo y Previsión Social, 2016). In this specification, the scores get values from 0 to 1,200, which can be classified into three levels of mental workload: low, medium, and high. According to the Chilean specification of the NASA-TLX, a score below 500 points represents a low level of mental workload. Meanwhile, a score between 500 and 1,000 points indicates that the task presents a medium mental workload. Scores above 1,000 points refer to tasks with a high mental workload. Figure 6 shows the averages of the mental workload scores for each scenario.
It can be seen in Figure 6 that all the average workload falls into the medium level of mental workload (500–1,000) category, except the scenario light-away (1,040).
The text and voice mechanisms show similar mental workloads in the same location. The scores between mechanisms in each location are nearly identical, obtaining a difference only in the case of text-delivered alerts in the greenhouse against voice-delivered alerts in the same location. Nevertheless, the light-delivered alerts in all the locations got higher mental workload scores. This can be caused by the light-delivered alerts only producing a stimulus and not providing additional information about the related problem, forcing the user to search for more details about the alert.
Besides, light-delivered alerts generate the highest mental workload when users are away, i.e., outside the greenhouse and the house. This phenomenon arises from users' challenges in receiving a light stimulus when absent. Considering that the alert device also produces sound, the alert remains difficult to perceive due to possible environmental noise. Some participants suggested changing the device's sound, making the light brighter, and changing the color to ease the alert's recognition.
Considering the user's location, text and voice-delivered alerts are better for delivering information when the users are away from their houses and the greenhouse. Meanwhile, when the user is at the greenhouse, the better delivery mechanism is the voice. In this case, participants pointed out that the voice mechanism is better because they can interact with the device and request more information about the problem, reducing the mental workload. Even they can ask for a possible solution, making dealing with the related issue easier. Moreover, considering they could be doing manual tasks, voice-delivered alerts remain better than those delivered by text despite the more comprehensive information that can be delivered in the text messages. With the text-delivered alerts, farmers would have to stop their current activities to check the messages on the device.
Interestingly, all the delivery mechanisms generate a similar mental workload when the user is at the greenhouse. At the greenhouse, users are commonly occupied and focused on tasks that require their complete attention, and when they receive the alerts, they must change their mental context to respond to them. These context changes significantly increase the mental workload. Therefore, participants emphasized that when they are engaged in manual tasks, they prefer the voice mechanism due to its interactive nature.
In addition, an interesting finding is related to the general mental workload level achieved. If we compare all the scenarios and mechanisms, it can be seen that all of them generate a medium mental workload (score between 500 and 1,000). This is important because even when some users feel a high mental workload in an individual scenario, the mental workload generated by the proposed system remains medium. We consider this an acceptable level of mental workload, considering that it was the first time participants used the system, and the proposed experiment (scenario design) and alert system were designed to be obtrusive in all contexts to guarantee user awareness of the alerts.
Finally, in the User Experience Evaluation, we utilized the User Experience Questionnaire (UEQ), which includes 26 items of semantic differentials to assess the user experience comprehensively. The UEQ questionnaire measures the interaction between the user and the system based on six dimensions: attractiveness, perspicuity, efficiency, dependability, stimulation, and novelty. As stated in Díaz-Oreiro et al. (2019), the common sample size to measure User Experience using the UEQ instrument is about 20 participants. We reached the typical sample size since we had 22 participants completing the assessments. Nevertheless, it is important to consider that we must remove one of the evaluations because it presented inconsistencies in the answers. Thus, we kept 21 completed answers for the analysis.
Regarding the results, the evaluated alert system obtained excellent outcomes. All the dimensions got values in the range of very good to extremely good, with the next individual means Attractiveness = 2.119, Perspicuity = 2.036, Efficiency = 1.976, Dependability = 1.690, Stimulation = 2.048, and Novelty = 2.024. The dimensions with lower scores were Efficiency and Dependability. Nevertheless, these dimensions got metrics in the very good range, which is excellent for us.
UEQ questionnaire also enables the grouping of the scales into Pragmatic Quality (Perspicuity, Efficiency, and Dependability) and Hedonic Quality (Stimulation and Originality). In this case, the proposed alert system obtained very good results. The system received a score of 1.90 for Pragmatic Quality, indicating that users perceive it as highly practical, efficient, and conducive to effectively achieving their goals. Additionally, the system scored 2.04 for Hedonic Quality, signifying that users derive pleasure, enjoyment, and aesthetic appeal from interacting with it. This suggests a positive emotional response and overall satisfaction with the user experience.
UEQ instrument also provides a benchmark compounded by a database with data from 452 software product evaluations (with a total of 20,190 participants in all evaluations). The benchmark classifies a product based on five categories: Excellent, Good, Above average, Below average, and Bad.
Regarding the proposed alert system evaluation, Figure 7 shows the scores for each dimension compared to the benchmark data. Here, the proposed system was evaluated well, achieving an Excellent score for almost all dimensions (Attractiveness, Perspicuity, Efficiency, Stimulation, and Novelty). In the case of the dimension Dependability, the obtained score is situated in the category of Good. This dimension measures how in control the user feels with the interaction. In this case, our proposed alert system is an innovative proposal to inform users of the distinct situations occurring in the crop field in a disruptive way. Several participants pointed out that training or explanations could be required to use the system better. This suggestion matches the remarks provided by the farmers in the perceived utility evaluation.
Finally, we asked participants to describe the proposed alert system using three words or short phrases. Figure 8 shows a word cloud containing the characteristics obtained from the participants.
As seen in the word cloud, the most common characteristics obtained were usefulness, novelty, fastness, ease of use, and efficiency. The word cloud results hold significant relevance as they provide a tangible manifestation of users' perspectives on the system. Moreover, they encapsulate the system's desired characteristics that were pursued. The word cloud not only unveils the prominent themes and concepts that users associate with the system but also serves as a valuable tool in gauging the system's effectiveness in meeting its intended goals. Furthermore, none of the participants qualified the proposed system negatively.
Next, we delve into a discussion of the findings gleaned from the system evaluations.
5 Discussion
In this study, we have comprehensively evaluated a greenhouse alert system, an integral component of our smart farming solution, with a primary focus on gathering insights and feedback from end users, specifically farmers. User-centric evaluations play a pivotal role in ensuring the usability of technological tools, particularly in scenarios where users may have limited technological skills or are older individuals. By adopting a user-centric approach, we aimed to glean valuable insights into users' needs, preferences, and capabilities, particularly among farmers who represent the end users of the greenhouse alert system under study. This approach enables us to identify potential usability barriers and challenges faced by users and adapt the system accordingly to enhance user-friendliness and overall user experience.
Our findings highlight the effectiveness and user-friendliness of the alert system and its delivery mechanisms. The results indicate a high level of acceptance among the diverse user groups considered in the evaluations, emphasizing the perceived utility of the smart farming system. Farmers have expressed a clear demand for innovative solutions in the agricultural sector, underlining the significance of our smart farming solution in addressing their needs.
Furthermore, the mental workload evaluation revealed that the alert system maintains an acceptable cognitive burden, an essential consideration for users engaged in other tasks during their daily farming activities. Additionally, the user experience evaluation demonstrated that user interaction with the system was excellent, a critical finding considering that many users in the agriculture sector possess low technological skills and may feel apprehensive when using technology-driven solutions like the one evaluated.
In summary, our integrated smart farming solution has emerged as a valuable and user-friendly tool for small and medium-scale producers operating in greenhouse-controlled environments. The system's acceptance among farmers and its ability to empower users to monitor, control, and respond to crop-related challenges position it as a promising innovation in the agricultural sector. Moving forward, we will continue to refine the system, prioritize critical data, and collaborate closely with agronomists to ensure its usability for all interested stakeholders.
Data availability statement
The original contributions presented in the study are included in the article/supplementary material, further inquiries can be directed to the corresponding author.
Author contributions
JB: Conceptualization, Funding acquisition, Investigation, Methodology, Software, Writing – original draft. GL: Methodology, Validation, Writing – review & editing. FF-P: Conceptualization, Funding acquisition, Software, Supervision, Writing – review & editing. GM-R: Conceptualization, Methodology, Supervision, Writing – review & editing.
Funding
The author(s) declare financial support was received for the research, authorship, and/or publication of this article. This work was partially supported by the Research Center in Information and Communication Technologies (CITIC) and the School of Computer Science and Informatics (ECCI) at the University of Costa Rica, Research Project No. 834-B9-189. This study forms part of the AGROALNEXT program and was supported by MCIN with funding from European Union NextGenerationEU (PRTR-C17.I1) and Generalitat Valenciana (Spain).
Acknowledgments
We extend our sincere gratitude to all individuals who generously participated in the evaluation of the system and contributed their valuable insights. Additionally, we would like to thank the farmers who graciously spared their time to attend the interviews during their busy schedules at the farmer's fair. Their cooperation and feedback were integral to the success of this study. We are immensely thankful for their support and collaboration.
Conflict of interest
The authors declare that the research was conducted in the absence of any commercial or financial relationships that could be construed as a potential conflict of interest.
Publisher's note
All claims expressed in this article are solely those of the authors and do not necessarily represent those of their affiliated organizations, or those of the publisher, the editors and the reviewers. Any product that may be evaluated in this article, or claim that may be made by its manufacturer, is not guaranteed or endorsed by the publisher.
Footnotes
References
Akdemir, Ş., Kougnigan, E., Keskin, F., Akçaöz, H. V., Boz, İ., Kutlar, İ, et al. (2021). Aging population and agricultural sustainability issues: case of Turkey. New Medit 20:2104. doi: 10.30682/nm2104d
Brenes, J. A., Ferrández Pastor, F. J., López, G., and Marín-Raventós, G. (2023). “Designing—context-aware smart notifications system for precision agriculture,” in Proceedings of the International Conference on Ubiquitous Computing and Ambient Intelligence (UCAmI 2022), eds. J. Bravo, S. Ochoa, and J. Favela (Cham: Springer International Publishing), 821–826.
Brenes, J. A., and Marín-Raventós, G. (2024). Scalable technological architecture empowers small-scale smart farming solutions. Commun. ACM 2024:3653327. doi: 10.1145/3653327
Brenes, J. A., Martínez Porras, A., Quesada López, C. U., and Jenkins Coronas, M. (2020). Sistemas de apoyo a la toma de decisiones que usan inteligencia artificial en la agricultura de precisión. Revista Ibérica de Sistemas y Tecnologías de la Información 28, 217–229.
Brenes, J. A., Soto, F., and Marín-Raventós, G. (2019). “A cost-efficient smart solution for small-scale farmers: a multidisciplinary approach,” in Proceedings of the III International Conference on Agro BigData and Decision Support Systems in Agriculture, eds. V. M. Albornoz and A. M. Cawley (Valparaíso: Universidad Técnica Federico Santa María, Chile), 101–104.
Dhillon, R., and Moncur, Q. (2023). Small-scale farming: a review of challenges and potential opportunities offered by technological advancements. Sustainability 15:2115478. doi: 10.3390/su152115478
Díaz-Oreiro, I., López, G., Quesada, L., and Guerrero, L. A. (2019). Standardized questionnaires for user experience evaluation: a systematic literature review. Proceedings 31:14. doi: 10.3390/proceedings2019031014
Ferrández-Pastor, F. J., Cámara-Zapata, J. M., Alcañiz-Lucas, S., Pardo, S., and Brenes, J. A. (2023). “Reinforcement learning model in automated greenhouse control,” in Proceedings of the 15th International Conference on Ubiquitous Computing and Ambient Intelligence (UCAmI 2023), eds. J. Bravo and G. Urzáiz (Cham: Springer Nature Switzerland), 3–13.
Hart, S. G., and Staveland, L. E. (1988). Development of nasa-tlx (task load index): results of empirical and theoretical research. Hum. Ment. Workl. 1, 139–183.
IFAD (2019). Creating Opportunities for Rural Youth. 2019 Rural Development Report. Technical Report. Rome: International Fund for Agricultural Development (IFAD).
Khan, N., Ray, R. L., Sargani, G. R., Ihtisham, M., Khayyam, M., and Ismail, S. (2021). Current progress and future prospects of agriculture technology: gateway to sustainable agriculture. Sustainability 13:94883. doi: 10.3390/su13094883
Lambert, J., Sekhar, N. U., Chatrchyan, A., and DeGaetano, A. (2019). Agricultural Decision Support Tools: A Comparative Perspective on These Climate Services (Cham: Springer International Publishing), 459–478.
Liu, J., Du, S., and Fu, Z. (2021). The impact of rural population aging on farmers' cleaner production behavior: Evidence from five provinces of the north china plain. Sustainability 13:12199. doi: 10.3390/su132112199
Ministerio del Trabajo y Previsión Social (2016). Método NASA TLX (Task Load Index). Available online at: https://ergomedia.isl.gob.cl/app_ergo/nasatlx/ (accessed February 22, 2024).
Mir, S. A., and Padma, T. (2020). Integrated technology acceptance model for the evaluation of agricultural decision support systems. J. Glob. Inform. Technol. Manag. 23, 138–164. doi: 10.1080/1097198X.2020.1752083
Mir, S. A., and Quadri, S. M. K. (2009). Decision Support Systems: Concepts, Progress and Issues—A Review (Berlin: Springer Netherlands), 373–399.
Norman, D. W., and Hays, H. M. (1979). Developing a Suitable Technology for Small Farmers, Technical Report. Modern Government/National Development, Kansas State University, United States.
Novák, J. t., Masner, J., Vaněk, J., Šimek, P., and Hennyeyová, K. (2019). User experience and usability in agriculture—selected aspects for design systems. Agris Onl. Pap. Econ. Informat. 11, 75–83. doi: 10.22004/ag.econ.303926
Santoso, H. B., Delima, R., Intan, E., and Wibowo, A. (2018). Usability testing for crop and farmer activity information system. Int. J. Adv. Comput. Sci. Appl. 9:1122. doi: 10.14569/IJACSA.2018.091122
Satola, Ł. (2019). Problems of the aging of the farmers' population in small farms in poland. Sci. Pap. Ser. Manag. Econ. Eng. Agri. Rural Dev. 19, 305–310.
Thomas, R. J., O'Hare, G., and Coyle, D. (2023). Understanding technology acceptance in smart agriculture: a systematic review of empirical research in crop production. Technol. Forecast. Soc. Change 189:122374. doi: 10.1016/j.techfore.2023.122374
van Someren, M. (1994). The Think Aloud Method. Knowledge-Based Systems. San Diego, CA: Academic Press.
Keywords: user-centric evaluation, user experience, mental workload, alert system, small-scale farming
Citation: Brenes JA, López G, Ferrández-Pastor FJ and Marín-Raventós G (2024) Usability assessment of a greenhouse context-aware alert system for small-scale farmers. Front. Comput. Sci. 6:1412913. doi: 10.3389/fcomp.2024.1412913
Received: 05 April 2024; Accepted: 31 May 2024;
Published: 19 June 2024.
Edited by:
Alicia García-Holgado, University of Salamanca, SpainReviewed by:
Maha Khemaja, University of Sousse, TunisiaVladimir Villarreal, Technological University of Panama, Panama
Copyright © 2024 Brenes, López, Ferrández-Pastor and Marín-Raventós. This is an open-access article distributed under the terms of the Creative Commons Attribution License (CC BY). The use, distribution or reproduction in other forums is permitted, provided the original author(s) and the copyright owner(s) are credited and that the original publication in this journal is cited, in accordance with accepted academic practice. No use, distribution or reproduction is permitted which does not comply with these terms.
*Correspondence: Jose A. Brenes, am9zZWFudG9uaW8uYnJlbmVzQHVjci5hYy5jcg==