- 1School of Computer Science and Engineering, Vellore Institute of Technology, Vellore, Tamil Nadu, India
- 2VIT School of Design, Vellore Institute of Technology, Vellore, Tamil Nadu, India
Alzheimer's disease (AD) is a type of brain disease that makes it hard for someone to perform daily tasks. Early diagnosis and classification of the condition are thought to be essential study areas due to the speedy progression of the disease in people living with dementia and the absence of precise diagnostic procedures. One of the main aims of the researchers is to correctly identify the early stages of AD so that the disease can be prevented or significantly reduced. The main objective of the current review is to thoroughly examine the most recent work on early AD detection and classification using the deep learning (DL) approach. This paper examined the purpose of an early diagnosis of AD, the various neuroimaging modalities, the pre-processing methods that were employed, the maintenance of data, the deep learning used in classifying AD from magnetic resonance imaging (MRI) images, the publicly available datasets, and the data that were fed into the deep models. A comparative analysis of different classification methods using DL techniques is performed. Further, the paper discussed the challenges involved in AD detection.
1 Introduction
Alzheimer's disease is a neurodegenerative condition that impairs brain function and destroys brain cells, altering memory and causing instability in human existence (Shankar and Walsh, 2009). Overproduction of amyloid-ß (Aß) and hyperphosphorylation of abnormal proteins are believed to be the two main contributors to AD pathogenesis. As a result, A-plaques and tau neurofibrillary entanglements accumulate and alter nucleo-cellular cytoplasmic transfer between neurons, leading to cell death and impaired memory and learning (Ramzan et al., 2020). The urgency of swift and precise disease identification and detection cannot be overstated. This is necessary because of economic expansion, the growth of information technology, and the advent of clinical information processing technologies. Early detection is not just a medical necessity but a societal responsibility, and role in this process is crucial.
Although genetic, environmental, and behavioral variables are thought to cause AD (Akkus et al., 2017; Albawi et al., 2017), the exact causes of this dementia are uncertain (Aldweesh et al., 2020). Conventional wisdom suggests starting an effective care plan as soon as cognitive impairment is noticed and basing it on an early diagnosis to prevent AD (Al-Naami et al., 2013; Alom et al., 2019). The current diagnosis relies on diagnostic methods that are positive when the sickness is practically irreversible (Al-Qatf et al., 2018). Most methods for diagnosing AD are arduous and involve a physician (Chen et al., 2018), making travel to clinical sites difficult for the elderly. Additionally, disease progression monitoring is costly (Chen et al., 2020). There is hope, though. Non-invasive, quick, inexpensive, and accurate AD diagnostic techniques are being developed (Forouzannezhad et al., 2019), promising early identification and effective treatment.
Alzheimer's disease is a gradual, degenerative condition that affects the brain's neurons, as shown in Figure 1. Cognitive and verbal skills change, and memory lapses follow (Amen, 2016). Age increases the occurrence of anomalies and loss in this neurodevelopmental condition, which disproportionately affects those over 65 (An et al., 2020). Clinical assessment, cognitive testing, and excluding other potential causes have traditionally been the three main components of the clinical diagnosis of dementia (Panza et al., 2010). To rule out other neurological illnesses, imaging investigations, clinical evaluations, and psychological testing must all be used to support the clinical diagnosis of AD (Atri, 2019).
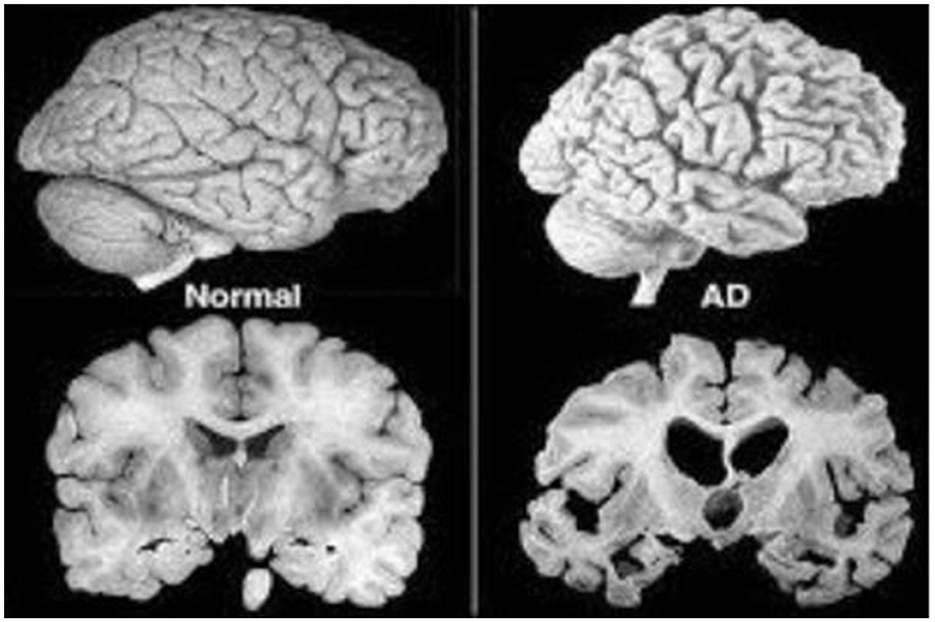
Figure 1. Normal vs. AD brain MRI (Huang et al., 2020).
Numerous non-invasive neuroimaging techniques, including diffusion tensor imaging (DTI), positron emission tomography (PET), and magnetic resonance imaging (MRI), have been studied in order to identify AD (Douaud et al., 2011), shown in Figure 2. The DTI examines water diffusion at the brain's microstructure to find the AD's abnormal diffusion pattern. By displaying data and images, PET allows us to statistically examine abnormal alterations in the human body and its normal metabolism. MRI can show the brain's structure and structural composition, which helps researchers examine AD brain shrinkage. MRI, one of many neuroimaging technologies (Lerch et al., 2008), is used to image brain tissue and classify illnesses. Before a diagnosis is made, patients go through multiple steps, although due to the diagnostic phases, this can happen later, and patients go into a late stage (Pierce et al., 2017). The different stages and phases of AD are discussed in the following subsection.
1.1 Various stages of AD
Most studies now focus on early AD diagnosis to find treatments and enhance patients' quality of life by studying the pathogenic mechanisms of different phases of AD (Basaia et al., 2019), shown in Figure 3. Preclinical AD is the first stage, where blood, brain, and CSF abnormalities occur without symptoms. Thus, contemporary life's beginning is no longer clear. The typical test struggles to detect AD early on, when the changes are too minor. Early AD detection is hindered by stigma, a lack of dementia care training, and the importance of early diagnosis (Dubois et al., 2009). Confusing dementia-like disorders with cognitive impairment (delirium, melancholy, vitamin deficiencies). This stage may begin 20 years before symptoms appear. An earlier AD experience study found significantly stronger connections between early linguistic skills and AD later in life (Bhandare et al., 2016). Stage two is modest cognitive impairment. Memory loss or other mental disability in those who can do most of their daily chores alone is called MCI (Sarraf et al., 2016). The patient's family and close friends may detect cognitive ability issues at this time, but they don't influence their everyday lives (Dubois et al., 2009). Only 10%−15% of MCI patients develop AD (Baskar et al., 2019; Bi et al., 2020), and it's unclear why certain people get dementia. After an MCI diagnosis, a particular diagnostic process must begin to identify the disease or condition that causes the impairment (Borghesani et al., 2008). The former describes memory-impaired patients. However, the latter identifies patients with cognitive problems in areas other than memory, such as attention or language processing. MCI increases AD risk, according to theory (Brown, 2015). Other stages of AD include late moderate cognitive impairment (LMCI), early mild cognitive impairment (EMCI), and cognitive normal (CN). AD's last stage, dementia, impairs daily life due to memory, cognitive, and behavioral issues. Table 1 lists AD phase symptoms. Early AD diagnosis helps patients take preventive measures, helps physicians identify AD progression risk, informs patients about the severity of the condition, and motivates them to use medications and lifestyle changes (Liu et al., 2014b). Therefore, the importance of an early AD diagnosis is as follows in the next subsection.
1.2 Purpose of an early diagnosis
The issue is that AD symptoms are frequently misdiagnosed as an effect of aging, delaying medical consultation until it is too late and leading to a delayed diagnosis (Brown et al., 2020). In the 2008 study (Busche et al., 2008), 64% of carriers admitted that before diagnosis, they believed changes in patients' behaviors were signs of aging. Sixty-seven percent of respondents agree that the diagnosis has been delayed. Moreover, once in the hands of experts, a precise diagnosis of AD is still tricky. Even the most skilled practitioners cannot correctly diagnose AD in 10%−15% of cases (Çayir et al., 2018). The only way to accurately diagnose the illness is through a postmortem examination of the brain. A clinical diagnosis of AD may currently be made with an accuracy of ~90% using a patient's medical history, neurological and physical examinations, laboratory tests, brain imaging, and neuropsychological assessment (Chakraborty et al., 2017).
Early diagnosis allows patients to access programs and support services and medications that can postpone some symptoms and work better in the early stages (Hennig et al., 2003). To diagnose AD early, even small symptoms must be recognized. Given the multimodal character of AD symptomatology, the best early AD detection method cannot simply rely on measurements of a single domain (Chitradevi and Prabha, 2020), such as physiological or behavioral symptoms, but rather on the combination of many modalities, which may allow to identify all subtle alterations of all domains from the start and contrast them with other symptoms for an appropriate diagnosis (Choi et al., 2020). Several research groups use neuroimaging techniques like MRI, fMRI, and PET to classify AD and MCI.
Numerous researchers are studying early AD diagnosis using machine learning (ML) and DL methods to discover a treatment. Even though ML models are more effective than ever in many domains, they still need human specialists to solve some problems. A flexible DL model algorithm learns from automatic feature extraction approaches regardless of prediction accuracy (Mahrishi et al., 2020). The DL model learns and predicts features. ML cannot automatically extract features. The following section discusses ML and DL AD diagnoses.
1.3 Machine learning vs. deep learning approaches
Over the past 20 years, AI and ML have gained popularity and relevance. ML is semi-automated data knowledge retrieval (Bardis et al., 2020). ML feeds an algorithm that understands input-output relationships. After training, the computer can estimate a new data point's value or class (Islam and Manivannan, 2017). Figure 4 shows ML vs. DL, a framework that can be learned without design. AI can run machines and act like people. The ANN is utilized in DL learning (Ayodele, 2010). ANN architecture is layered. DL types include RNNs, auto-encoders, and CNNs (Hosseini et al., 2020). DL frameworks let programmers build and test DL apps. PyTorch, TensorFlow, Keras, Caffe, Theano, and more open-source DL frameworks are available online. Many notable open-source frameworks are ranked by developer's group, supported languages, and useful programs. It compares ML with DL based on data, training time, and interpretability. The fundamental difference between ML and DL is in Table 2. A suitable function is manually extracted from the data by ML. The extracted function is used to update model parameters for accurate prediction (Wei et al., 2019). This is irrelevant since DL automatically retrieves data functions. DL also uses end-to-end learning, where the network delivers data and tasks (Chen et al., 2018).
High-performing DL models require 1,000 records (Guo et al., 2019). Recent years have seen DL's speech recognition (Deng and Platt, 2014; Noda et al., 2015), image recognition (Pak and Kim, 2017; Wu and Chen, 2015), and speech analysis applications assist healthcare (Li et al., 2017), pharmacy (Wang F. et al., 2019), natural language processing (Otter et al., 2020), and other sectors (Young et al., 2018). Recently, many DL recommendations (Alom et al., 2019) have emerged, which may be categorized into algorithms, which will be explored in Section 3. To retrieve the original image and meet feature extraction, selection, and classification criteria, several pre-processing steps are needed. Most machine learning studies involve preprocessing before data manipulations, whereas deep learning surveys may not. Most research preprocesses raw data with intensity normalization, recording, skull dissection, and motion correction (Zheng et al., 2017).
The structure of this survey is as follows: Section 2 discusses the materials and method of this review, and Section 3 discusses the detailed investigation of AD detection. Section 4 mentions the various datasets for diagnosing AD. Performance measures for detecting AD are discussed in Section 5. In Section 6, the different challenges of the AD diagnosis are presented, and Section 7 concludes with the substantive work of the survey.
2 Materials and methods
This review aims to systematically assess studies investigating the association between AD biomarkers and affective symptoms in MCI and AD dementia, adhering to the recent research framework (Jack et al., 2018). The search query was composed of several combinations of the following keywords: Alzheimer's disease, challenges, MRI, deep learning, pre-processing, feature extraction, and classification.
Based on the following criteria, titles and abstracts were independently evaluated for broad appropriateness in the review. In addition to this electronic search, a lateral search was undertaken: reference lists of retrieved publications and secondary literature (review articles, editorials, etc.) were screened to identify possible additional studies, as recommended (Greenhalgh and Peacock, 2005). Research protocols and conference abstracts or posters were excluded. After this first screen, a full-text review was conducted to assess eligibility. The selection process followed the Preferred Reporting Items for Systematic Reviews and Meta-Analyses (PRISMA) guidelines (Liberati et al., 2009) as shown in Figure 5.
3 Literature review on the detection of AD
According to recent literature, numerous studies (Esmaeilzadeh et al., 2018; Yanase and Triantaphyllou, 2019) emphasize the importance of diagnosis and classification as key areas of computational research in Alzheimer's disease. The several stages of the process are illustrated in Figure 6, which includes data acquisition, preprocessing, data splitting, classification and learning evaluation. Each of these stages is considered crucial in developing accurate and reliable models. The following subsections provide more insight on each of these stages.
3.1 Data acquisition for Alzheimer's disease detection
The first and foremost step in the diagnostic pipeline is data acquisition, which involves collecting necessary datasets, including imaging data or other biomarkers for diagnosis. The quality and diversity of the data have a direct impact on the model's overall performance. Errors created here can spread across the system and disturb clinical operations. Generally, the following factors can help a digital imaging department integrate image acquisition equipment (Andriole, 2006).
• The simplicity of integrating the gadget into the clinical environment's established daily workflow routine.
• High device fault tolerance and dependability.
• User interface simplicity and intuitiveness.
• Device performance.
When it comes to acquiring images from intrinsically digital modalities like computed tomography (CT) and MRI, the industry standard for digital imaging and communication in medicine is called DICOM capture (Paul et al., 2024). The DICOM method ensures the acquisition and transfer of images from the modality at its full bit depth and spatial resolution. However, the DICOM method only allows for 8 bits of image quality or 256 gray levels, but the direct recording of digital data from essentially digital modalities is the preferred acquisition method, providing the highest quality images for clinical needs.
Images contain information that can be gained through a variety of modalities or procedures and describe the internal characteristics of the body. This process can produce neurological data and combine multiple physical principles (Theodore et al., 1986). The task determines the choice of these modalities, the model being employed, and the researcher's preference. Numerous organizations, such as clinics, hospitals, X-ray facilities, and online platforms, provide datasets (Wu et al., 2019).
An early diagnosis of AD is more likely if representative causes, such as blood, urine, saliva, and nasal mucosa, are thoroughly analyzed (Sakatani et al., 2020). However, this method cannot be utilized as a routine detection tool because of the difficulties in gathering samples. Furthermore, the subject's body will inevitably move slightly because of the extended acquisition period. Building DL models cannot directly use real-world data since it contains noise and missing values in an unprocessed state.
Pre-processing procedures for data are required to transform such noisy data into a format that a machine can read. The original image must undergo several preprocessing steps to meet feature extraction, classification, and selection requirements. Most studies require preprocessing before data operations, particularly in ML. Some investigations might need something other than preprocessing with the aid of DL. However, most research continues preprocessing raw data using motion correction, registration, skull dissection, and intensity normalization (Tong et al., 2014).
3.2 Pre-processing
This section focuses solely on the preprocessing of MRI for AD identification. Nonetheless, if essential for an improved result, this procedure is vital for all imaging data. Building DL models cannot directly use real-world data since it contains noise and missing values in an unprocessed state. Pre-processing procedures for data are required to transform such noisy data into a format that a machine can read. Figure 7 shows various preprocessing techniques for AD detection using DL approaches.
• Correction: one method of aligning image geometry is through AC-PC correction, which places the anterior commissure (AC) and posterior commissure (PC) in the same axial plane (Jiao et al., 2016). These two critical anatomical landmarks in the brain are the centers of alignment for the AC and PC. Gradwarp is another preprocessing step that uses gradient non-linearity to rectify geometric distortions (Weavers et al., 2017).
• Tissue segmentation: it is sometimes necessary to consider the state of particular discrete portions while examining MRI images. The brain's anatomical structure removes the tissue from the target area (Lazli and Boukadoum, 2018). Measuring the tissue volume in each region is the aim of tissue segmentation. MRI was divided into three parts, and depending on the pre-treatment architecture of the CSF, white matter, and gray matter, each segment was examined alone or in combination. These three brain structures have different roles and undergo different morphological changes due to AD or MCI. All of them require the extraction of features. Hence, it has to employ an image segmentation technique.
• Heterologous registration: this pre-processing method completes the registration of T1 and T2 echo time images from PET and MRI and the registration of differential imaging data (Gao and Lima, 2022). Significant variances exist since the images that must be stored originate from various data formats. As a result, the least squares approach is no longer suitable, and this operation is finished using a more precise and reliable registration method based on shared information.
• Normalization: direct analysis is impossible due to the significant variations among individual images. Normalization is registering the images from the earlier pre-processing step in the standard brain template space established by the Montreal Neurological Institute (MNI) to standardize the imaging coordinate system. MRI images of numerous healthy individuals were analyzed for a new brain reference, MNI space (Tufail et al., 2022). It offers a standard model for brain image normalization.
• Smoothing: smoothing reduces functional differences between images, suppresses noise in functional images, and increases the signal-to-noise ratio. For straightening, the gaussian core function is typically used. Based upon practical efforts and experience (Zhang et al., 2019), gray matter density images and PET images are commonly used in 64 × 64 × 64 pixel cube-down samples; this can cut down on operating and memory requirements without sacrificing categorization precision. Format conversion, head motion correction, heterologous registration, normalizing, smoothing, and other preprocessing techniques are applied to PET images (Hamdi et al., 2022). Different preprocessing methods for MRI images also have differences. For MRI images, skull removal, time slice correction, head movement, format conversion, heterologous registration, standardization, brain region segmentation, and smoothing are examples of pre-processing steps (Aderghal, 2021).
Preprocessing is essential when the quantity of high-quality labeled data is constrained and acquiring additional examples is costly and labor-intensive. Consequently, it should be regarded as a significant concern in medical analysis, especially in the early diagnosis of Alzheimer's disease.
3.3 Data extraction and maintenance
Maintenance of data can be used for tasks like testing and training data. Extraction of data is needed for the two steps above. Taking away the attributes is an easy way to make the data less multidimensional. Direct classification is complicated due to the enormous size and noise of the raw image. Depending on the types of extracted features, input data management solutions may be categorized into four groups: slice-based, voxel-based, regions of interest (ROI)-based, and patch-based (Ahmed et al., 2020; Nguyen and Chu, 2020; Healy and McMahon, 2018). The four primary categories into which it can be separated are shown in Table 3.
(a) Slice-based: the slice is a section of a complex 3-D image that has been simplified to a 2-D image. However, the information from brain scans cannot be fully represented in 2D image slices, and the spatial link between adjacent slices is lost. Slice-based techniques typically focus on the brain center while ignoring other regions. Some searches extract sections of 2D images using exclusive techniques. The axial or horizontal, frontal, medial, and coronal planes are some examples of standard projections used in neuroimaging studies. A slice-based iron buildup model (Ren et al., 2019) is used to develop novel therapies for long-term diseases like AD. Simple broad plain CNNs with a specific slice, multi-slice CNNs, and simple assembled CNNs with combinations of three classifier slices are suggested.
Additionally, they recommended monitoring the model using fewer slice regions, which improved AD and CN task performance by over 90%. In 2021, a DL architecture based on MRI brain carving was proposed (Zhang et al., 2022). By fusing the attention mechanism with the slicing region, this framework has successfully increased intelligence, increasing precision from 1% to 8% compared to more sophisticated techniques. The slices were then adjusted using wavelet entropy, multi-layer perceptron, and biogeography after being selected as single slices using 3D volume data using a cutting-edge AD detection technique (Wang et al., 2018b). Reduced computing costs by employing fewer fluorodeoxyglucose PET and CT images, the posterior single cortex, and the hippocampus are two regions with selective learning affected by neuropathological AD deficits (Kim et al., 2020).
(b) Voxel-based: Using statistical analysis, the extraction of voxel-based features approach removes the active value of a voxel as a feature from the preprocessed image. For evaluating the volume or density of the three tissue components, CSF, white matter, and gray matter. It is the most precise voxel-based analysis technique. To use this technology, registration is usually necessary, which involves normalizing each spatial image of the midbrain into a three-dimensional space. Small changes in individual brain areas in brain tissue can be quantitatively analyzed using the voxel-based feature extraction method. To assess the validity of long-term variations in the volume of gray matter, a voxel-based longitudinal morphological measure was used (Takao et al., 2021). The authors proposed a voxel-based morphometry technique (Zhang F. et al., 2019) to identify AD sufferers from the healthcare control group (HCG). Although texture parameter modeling was the best method for the model, voxel-based longitudinal morphological parameter modeling was faster. However, each brain MRI scan has hundreds of thousands of voxel units. They will lead to excessive complexity if they are used directly as characteristics, resulting in the need for adequate solutions to lessen the number of characteristics. Choosing an example voxel will reduce the number of fields that need to be filled in. The authors (Ortiz et al., 2016) employed the t-algorithm to select the optimal voxel for the study in order to increase and decrease the amount of calculations and the efficacy of the experimental setup.
(c) RoI-based: forming voxels into the distortion-specified atlas yields the ROI, and part of image classification entails figuring out the region's measurement value. The RoI technique focuses on particular brain areas, which are known to be affected early in AD, rather than the whole brain. DL networks are also used to classify advertisements and extract the prospective characteristics of RoI measurements from various imaging modes. To identify hierarchical nonlinear interactions between areas (Karwath et al., 2017), for each RoI, a DL model was trained and a deep auto-encoder was created. Based on the RoI of the MRI, principal component analysis (PCA) was applied to PET scans to segregate 93 volumetric features (Suk et al., 2016). In Zheng et al. (2017), the authors coupled the strain amplitude of MRI scans with patch-based specifications to extract the RoI. DL models were used by Li et al. (2015) to extract the informational RoI from PET scans. To prevent the subdivision of RoIs from being influenced by researchers and other factors, RoIs must be established in RoI-based approaches using the long-term empirical accumulation of pertinent researchers (Spreng et al., 2020; Verfaillie et al., 2018). Consequently, the application of this method does not produce steady performance.
(d) Patch-based: early on in AD, structural changes are localized rather than affecting the whole brain or particular brain areas. The patch-based method looks for patterns associated with brain illness by extracting a feature from small picture patches. The main problem with the patch-based strategy is choosing the best image patch to capture both local and global information. This method has been applied in research on AD detection. In order to gather patterns associated to disease in the brain, patch-based techniques (Platero and Tobar, 2017) may obtain features from tiny portions of images that may identify alterations in specific regions carried on by disease. Its main goal is to select patches of information and filter out areas with less information to gather both local and global characteristics. A multimodal-like deep belief model (DBM) is used to represent common features from paired MRI and PET patches (Suk et al., 2014). Complex underlying models can be found in MRI and PET by incorporating graders for hierarchically merging information at the patch level. A multi-instance learning model (Paul et al., 2024) was developed using local fixes as attributes to predict MCI transition and classify AD. Patch-level sub-networks were created to boost training efficiency (Lian et al., 2018).
(e) Summary based: the objective method of analysis is based on voxels. It treats the brain consistently without modifying its anatomical structure (Takao et al., 2021). Due to its large number of voxels and disregard for local information, using voxel preselection is crucial. By employing 2D slices as input, the network can be made simpler with fewer formation parameters; however, the relationship between neighboring slices will fail. Slice approaches are more easily recognized in coronal and sagittal views; however, axial views are the most typical. The sound while scanning will more or less impact the original brain (Vinutha et al., 2021). This noise is typically brought on by the patient's mental activity, the environment around the equipment, the operators, etc. The ROI-based method is easy to teach and use in clinical practice, and it can represent the complete brain with fewer functions (Suk et al., 2014). Due to this, the functionality dimension is lower compared to approaches using slices and voxels. According to the study, RoI can exploit the hippocampus, cortical thickness, and other brain parts effectively. There are a lot of unexplained regions in the brain's distribution of outlier areas, which might cause information to be lost and make it difficult to extrapolate traits. Despite the functionality extraction approach being more precise, the extraction per patch does not require RoI detection.
The above Section 3 points out that the literature discussing the benefits of diagnosing AD early is based on the opinions of experts rather than research evidence. Not many studies have been published that look at the pros and cons of diagnosing early AD. This may be because AD is only recently defined as a condition that includes both the pre-dementia and dementia stages and the way they were conducted. It's possible that some studies were missed because of the search terms that were used, even though the literature review was thorough and the terms should have been broad enough to include most publications important to a timely diagnosis of AD. Also, some of the studies looked at the possible benefits of noticing cognitive loss early without saying what caused the dementia syndromes. These studies could have included people with other types of dementia or mixed pathology. A different problem with this review is that it didn't rate the scientific quality of the studies. This means that some of them may have had a high risk of bias. Also, because the studies were so different, a full systematic review with meta-analysis could not be done, as required by the PRISMA recommendations.
Several studies looked at the possible economic benefits of diagnosing and treating Alzheimer's disease early. Early identification and treatment are likely to cost more up front, but economic modeling shows that these costs may be balanced out by savings in the long run, mostly from fewer patients having to stay in hospitals and better quality of life for both patients and caregivers. But longitudinal studies are still needed to find out how much it really costs to stop the disease from progressing from predementia to mild to serious AD and to keep people living in their own homes for longer. Because of this, more research needs to be done on the health and social care costs that come with diagnosing AD early, during the prodromal stage.
3.4 Classification methods using deep learning
As previously stated, DL is a branch of ML. Due to its automatic feature extraction, DL is more efficient than previous ML methods. Additionally, DL carries out “end-to-end learning,” in which the network is given tasks and raw data. The important classifiers of DL methods are shown in Figure 8. Compared to other DL techniques, including recurrent neural network (RNN), deep neural network (DNN) autoencoder (AE), and deep belief network (DBN), most studies relied on convolutional neural network (CNN) methods for diagnosing AD using MRI scans. Table 4 lists the advantages and disadvantages of learning and classifying AD diagnostics. The given section discusses AD classification using deep learning methods in detail.
3.4.1 Autoencoder
Feature-encoded data can be produced by compressing unlabeled datasets using unsupervised learning techniques such as AE. It reduces dimensionality and consists of two primary parts: an encoder and a decoder. The encoder converts the input data into code, or compressed data, which the decoder then rebuilds to create an output corresponding to the input. Convolution layers, or dense layers, are present in the encoder section. The encoding and decoding phases ought to be at comparable stages. The encoder increases the size of the data, but it also reduces it. The intermediary layer that reduces the representation of the incoming data is known as the bottleneck layer.
In Liu et al. (2014a), the author has implemented a Softmax regression layer and sparse auto-encoders used in CNN's deep learning architecture to classify AD detection. Payan and Montana (2015) suggests a pre-formed 3D CNN for classification-based AD detection that uses sparse auto-encoders. Chen et al. (2017) suggested an auto-encoder (DSAE) that classifies data using support vector machines (SVM). SVM was outperformed by applying a DBN, a layered RBM model, and structural AD detection. An expert illustration of low-latitude information (Faturrahman et al., 2017) is obtained using DL techniques as an auto-encoder of changes in hippocampal morphology.
3.4.2 DBN
The unsupervised features taken out of the stacked layer can be connected using DBN, a supervised learning method. This generative graphical framework comes from an array of restricted Boltzmann machines (RBM) that can extract information and replicate the input. The top two levels of DBN are connected in an undirected manner. DBN uses RBM to lower weight initialization, aiding the model's overfitting mitigation.
Ortiz et al. (2016) put out four distinct voting methods and two deep learning-based structures. They are put into practice and contrasted, yielding a robust classification architecture that computes discriminative features in an unsupervised manner. Generally speaking, a combination of weak classifiers is more accurate than a single classifier. The suggested approach exhibits strong performance in testing for more complex cases of MCI subject classification due to the integration of two techniques. It is valid for distinguishing between NC and AD images. In Ortiz et al. (2017), the authors proposed automated anatomy labeling based on brain regions' Deep Belief Network (DBN) architecture to develop classification techniques. This approach is effective at categorizing HC and AD as well as MCI. In Hon and Khan (2017), the author developed classification-based AD detection using the Inception V4 deep model.
3.4.3 DNN
A DNN consists of an input, an output, and several hidden layers. It is notable for its capacity to manage complex problems, understand the connection between input and output data, and simulate complex non-linear interactions (Ebrahimighahnavieh et al., 2020). It considers supervised learning techniques and is used in many academic disciplines to look for input patterns that haven't been found before (JayaLakshmi and Kishore, 2022). The features of the tagged images must be extracted using a substantial amount of data for training (Noor et al., 2020).
Qiu et al. (2020) predicted the subjects' cognitive function using a deep neural network (DNN) based on the subjects' age and blood test items. To train the DNN model, they recruited 202 individuals with a range of systemic metabolic diseases. The Mini-Mental State Examination (MMSE) was administered to the participants. The authors also proposed that using the DNN model for younger patients may predict future cognitive impairment after the development of atherosclerosis and that the discrepancy between the predicted and ground truth MMSE scores was due to changes in atherosclerosis with aging.
In Park et al. (2020), the authors have suggested a DL-based approach that uses extensive gene expression and DNA methylation data to predict AD. The most difficult problem when developing a framework for diagnosing AD based on the multi-omics dataset is combining different omics data and dealing with highly dimensional and low-sample-size data. Furthermore, the author developed a prediction model based on neural networks with deep layers that perform better than conventional machine learning methods. The prediction model and feature selection approach presented in this study (Park et al., 2020) perform better than traditional algorithms for machine learning that use conventional dimension reduction methods. The author also demonstrated how integrating gene expression and DNA methylation data could improve prediction accuracy.
In Basher et al. (2021), the authors have developed a technique to detect AD using structural magnetic resonance imaging (MRI) data that has been slice-wise volumetrically extracted from the left and right hippocampi. The suggested approach combines a convolutional neural network (CNN) model and a deep neural network (DNN) model. A two-stage ensemble, Hough-CNN, has been used to automatically locate the left and right hippocampi. Three-dimensional patches with 80 × 80 × 80 voxels are extracted using the localized hippocampus positions. Then, the 2-D slices are divided from the 3-D patches using axial, sagittal, and coronal perspectives. Volumetric information is extracted from each slice using the preprocessed 2-D patches and a discrete volume estimation convolutional neural network (DVE-CNN) model. The classification network has been trained and tested using the derived volumetric features. Based on the derived volumetric features ascribed to the left and right hippocampi, the suggested method has obtained average weighted classification accuracies of 94:82% and 94:02%, respectively. Furthermore, the area under the curve (AUC) values of 92:54% and 90:62%, respectively, were obtained for the left and right hippocampi.
In Wang et al. (2023), the author examines three popular heatmap techniques: guided gradient CAM (GGC), integrated gradients (IG), and layer-wise relevance propagation (LRP). By computing overlap with a ground-truth map from a sizable meta-analysis that merged 77 voxel-based morphometry (VBM) investigations conducted independently from ADNI, the authors could derive exact quantitative measurements. By creating heatmaps using convolutional neural networks (CNNs) trained on T1 MRI scans from the ADNI data set and contrasting them with brain maps that matched support vector machine (SVM) activation patterns, the authors investigated this work (Wang et al., 2023).
3.4.4 RNN
RNN addresses time-series or sequence-based challenges. Utilized storage and hidden states are the two main advantages. An example of an RNN design comprising an input, a hidden layer, and a layer for output is displayed. Recalling reliable knowledge regarding the problem sequence is facilitated by the secret state. Unlike feedforward networks, RNNs share identical parameters throughout all network layers, another trait that sets them apart. There are many parameters in the later networks, such as the feedforward networks, because each network node has a different set of parameters (DiPietro and Hager, 2020).
Common RNN architectures include gated recurrent units (GRUs) and long short-term memory (LSTM). Maintaining any inaccuracy that arises throughout the many layers and timeframes is the primary goal of the LSTM (Nicholson and Gibson, 2018). The hidden layer has cells with three gates: an input, an output, and a forget gate. These gates store data and control information flow to predict the network's production (Chen et al., 2018). This one cell helps the model decide what to store and when to change information through the gates. GRUs have two gates: an update gate and a reset gate. They operate in a concealed state. They decide what data is kept and for how long (Ruiz et al., 2020). In numerous jobs, its performance surpasses LSTM's (Alom et al., 2019; Akkus et al., 2017).
In Hong et al. (2019), the author suggests a prediction model based on LSTM. Consequently, an LSTM network with fully connected layers and activated layers is built in order to encode the temporal association between attributes and the ensuing stage of Alzheimer's disease. The model considers the temporal data gathered from the instances. The authors (Liu et al., 2020) have described a technique that combines an LSTM network with a multi-time sparse smooth network to identify early and late MCIs using multi-time points of resting-state fMRI. Specifically, resting-state fMRI data obtained across various time points is used to construct a sparse, smooth brain network. Features are then extracted and longitudinally analyzed using an attention-based stacking bidirectional LSTM.
The authors (Rajasree and Brintha Rajakumari, 2024) developed a four-phase method for early diagnosis of Alzheimer's disease: pre-processing, feature extraction, feature selection, and classification. Preprocessing involves data normalization and cleansing. The preprocessed data is then used to recover characteristics such as “Weighted Geometric Mean Principal Component Analysis (WGM-PCA), statistical features, higher-order statistical features, and weighted modified correlation-based features.” The most pertinent attributes are selected using the Improved Attribute Ranker (IAR). Additionally, a deep learning model based on an ensemble of classifiers that includes optimized “Bi-GRU, Multi-Layer Perceptron (MLP), and Quantum Neural Network (QDNN)” represents the disease categorization phase.
3.4.5 CNN
A convolutional neural network is one of the most effective methods for classifying and recognizing images in neural networks. It comprises a classifier layer, layers for pooling, activation layers, convolution layers, and fully linked layers. An essential layer that retrieves the feature maps for a given input image size without requiring a learned filter (or kernel) is the convolution layer. The activation function, which decides whether or not to stimulate the cell, is then followed. It transforms the input nonlinearly to learn and carry out increasingly tricky tasks. Activation functions come in many varieties to construct feature maps, including sigmoid, Tanh, and ReLU. Layers of pooling retain the most significant features while reducing dimensionality. They fit the definition of downscalers. Every neuron in the current layer is connected to every other neuron in the preceding layer via a fully connected layer. The classifier layer then chooses the label or class with the highest probability. A few papers for AD detection using CNN are discussed below.
In Andersen et al. (2021), the authors presented an interpretable deep learning technique that uses multimodal inputs such as age, gender, MRI, and Mini-Mental State Examination score to identify distinct Alzheimer's disease signs. The framework connected a fully convolutional network, which creates precise, understandable visualizations of individual Alzheimer's disease risk en route to accurate diagnosis, to a multilayer perceptron. This network develops high-resolution maps of illness likelihood from local brain anatomy.
To recognize AD (Aderghal et al., 2016), the author has applied an eight-layered 2D CNN to MRI data in the sagittal view. The activation mechanisms sigmoid, leaky ReLU, and ReLU were tested along with three distinct pooling functions (average, max, and stochastic). The most significant outcomes for image classification came from the leaky activation function of ReLU with max-pooling. In 2D CNNs, convolutions are only used to analyze features from the spatial dimensions of the 2D feature maps. A longstanding issue in the medical industry is the scanning of image samples.
In Wang et al. (2018a), the author, with the help of the ReLU leak and experiment, constructs an 8-layer CNN with 97.65% accuracy. The pre-formed VGG-16 was used as a functionality extractor by Janghel and Rathore (2021) to categorize AD, CN, and MCI. The VGG-inspired network was introduced as a backbone in the paper to increase the dataset (Jain et al., 2019). Additionally, the VGG-inspired network has the convolution block attention module. The accuracy of the migration technique employed in the research (Wang S. H. et al., 2021) to locate AD within the ResNet network was 98.37%. Thanks to neuroimaging's ability to offer spatial correlations between the pictures, 3D CNN technology is also frequently used in AD diagnosis.
In Ding et al. (2019), the author employed an Inception v3 network tested at 10% and formed on 90% of the ADNI data. Processing 18 forms of non-fluoro PET images is done using the grid method, which is extracted from the ADNI dataset. To locate brain voxels, researchers employed Otsu's threshold approach. Using an 8-batch size and a 0.0001 learning rate, the Adam Optimizer trained the training model. It comprises three classes: AD, MCI, and CN. The suggested idea achieves 82.5% specificity and 100% sensitivity. The paper (Liu et al., 2018) discusses CNN 3D architecture for deep, multi-instance learning based on landmarks for diagnosing brain diseases. Performance is significantly better than the conventional Alex Net system.
An ensemble of 3D densely connected convolutional networks (3D-DenseNets) for diagnosing AD and MCI has been proposed by the authors in the paper (Wang H. et al., 2019). The authors have arbitrarily altered hyperparameters around the ideal value to create base networks with various topologies. To optimize the flow of information, dense connections—where each layer is closely coupled with every other layer—were first introduced. Then, 3D-DenseNets with various designs were combined using a weighted-based fusion technique. By altering the hyperparameter initializations and architecture, a base 3D-DenseNet was built. A weighted-based fusion technique was employed to integrate the basis classifiers. Accuracy was gradually increased using the ensemble model instead of merely taking the network's forecast average.
In Chitradevi and Prabha (2020), the authors divided the brain into smaller sections, including the gray matter, white matter, and hippocampus, using various optimization methods, including the Cuckoo Search, Gray Wolf Optimization, Particle Swarm Optimization, and Genetic Algorithm. Two-hundred images were received from the hospital and processed using various methods, including head stripping, quality improvement, and contrast amplification. After segmentation, an area is validated against a field truth image to determine segmentation performance. The Jaccard Index, Tanimoto, similarity of volumes, structure similarity index metrics, and feature similarity index metrics are all assessed as part of the validation process. CNN has specifically used feature extraction and classification tasks using the AlexNet model. Compared to other optimization techniques, Gray Wolf Optimization offers the best performance, reaching a high precision of 95%.
In Nawaz et al. (2021), the authors have implemented three models to determine their accuracy. The first model involved retrieving handmade features from the images during pre-processing, then classification using the closest k-neighbor and Random Forest. The second model was constructed using CNN deep learning based on the preprocessed data set. Classifiers such as random forest, nearest k-neighbors, and vector support machines were evaluated. The third model, AlexNet, was used to derive profound characteristics. Upon examination, the model with deep features demonstrated the highest classification accuracy using a carrier vector machine. Notably, vector support machines showed the highest accuracy, at 99.21%, followed by k-nearest neighbors with 57.32% accuracy and random forests with 93.97%. Liu et al. (2018) used a real-time method based on deep features to identify the phases of Alzheimer's disease.
In Kundaram and Pathak (2021), data from the ADNI were obtained, and then the images were pre-processed and scaled. Disease classification and formation take place using CNN models. The template used 9,540 photos from the three image categorization classes (AD, MCI, and CN). The CNN model produced three maximum pooling layers, three layers of convolution, four ReLU activation layers, and numerous optimizers, such as Adam, Adagrad, Nadam, Adadelta, and Rmsprop. Adagrad achieves the best precision with the slightest loss by contrasting several optimizers with the suggested framework. On the ADNI dataset, the proposed model is 98.57% accurate.
Lee et al. (2019) employed AlexNet to increase the overall accuracy of categorizing AD with success. Despite outperforming the bulk of conventional characteristic extraction techniques, CNN's extended training period necessitates substantial visual data. In Huang et al. (2020), the authors used fast and dense connections to accurately classify medical images, feeding the densenet's input the feature map generated by the improved PCANet.
In Marzban et al. (2020), the author used convoluted neuron networks and diffusion tensor images to diagnose AD detection. To expand the dataset, the authors presented the VGG-inspired network as a backbone (Wang J. J. et al., 2021). They also incorporated the convolution block attention module into a VGG-inspired network.
In ResNet-50, the spatial transformer networks (STN) and the nonlocal attention mechanism were added to address the issue of CNN losing local information (Sun et al., 2021). The most relevant attention modules may be quickly implemented into the current network architecture. Liang and Gu (2020) proposed a method that yielded excellent results in classifying and recognizing AD through a few annotations using a core network with a focus strategy. In Katabathula et al. (2021), the author used the hippocampus MRI data, a 3D light array model with visual and overall form representations that can be used to predict AD detection.
A model is proposed (Pei et al., 2022) to use long-range addiction mechanisms based on a network of convolutional neurons to diagnose Alzheimer's disease. Ajagbe et al. (2021) proposes deep CNN (DCNN) methods for multiple AD classifications using MRI. Many developed CNN models are capable of accurate AD detection, including VGG Net, Alex Net (Zhang Y. D. et al., 2019; Lu et al., 2019; Wang S. H. et al., 2019), ResNet (Alotaibi and Alotaibi, 2020; Firdaus et al., 2018), DenseNet, and Inception (Satapathy, 2020). Co-investigators can establish a proprietary architecture based on Puttagunta and Ravi (2021); Yang and Mohammed (2020). Recently, several authors have developed different techniques for detecting AD. Faisal and Kwon (2022) used automated mild cognitive problems and whole-brain MRI to identify AD based on numerous investigations; CNN is the most efficient deep learning model.
Meng and Zhang (2023) suggested the Dual Fusion Cluster Graph Convolution Network (DFCGCN) model, which integrates the Cluster GCN in series with one adjacency matrix, two feature extraction channels, and one. The decomposition-fusion framework (Zuo et al., 2023) decomposes the feature space into the union of uniform and unique spaces for each modality, then adaptively fuses the decomposed features to learn MCI representation. The flattened, sparse vectors can retrieve advanced information from brain imaging by downsampling them via graph pooling. Specifically, two feature extraction channels, one adjacency matrix, and one cluster GCN in series are combined in the Dual Fusion Cluster Graph Convolution Network (DFCGCN) model (Prasath and Sumathi, 2024). The flattened sparse vectors can retrieve sophisticated features by sampling brain imaging data with graph pooling. The Alzheimer's Disease Neuroimaging Initiative (ADNI2) sMRI image collection was utilized by the authors of this work (Ravi et al., 2024) to quantify and categorize the AD stage. The author concentrated on using various DL algorithms to classify AD MRI images into multiple classes. The author suggested the most effective pre-trained model to forecast the patient's stage in this work reliably. Visualization methods like Saliency Map and Grad-CAM are applied to the model that best explains the image's region of focus, allowing for the prediction of the image's class.
To characterize brain patterns seen in 2D slices of MRI images, Avots et al. (2024) implemented the performance of 16 histogram-based image texture descriptors and features taken from 18 pre-trained CNN. The main goal is to find this assignment's most helpful feature types. The initiative known as the Alzheimer's Disease Neuroimaging Initiative (ADNI) provided the MRI dataset that was utilized to derive the features. This paper used five binary machine learning approaches to classify the data after computing characteristics on 2D axial, coronal, and sagittal slices. Data collection should be done using an autonomous, distinct, and efficient AD detection framework that directs the different AD detection techniques. This would facilitate enhancing the models' capacity to generalize the subsequent datasets.
Zuo et al. (2024) introduce a PALH model for predicting faulty brain connections using triple-modality medical images. An adversarial technique estimates anatomical knowledge's previous distribution to assist multimodal representation learning. An additional pairwise collaborative discriminator structure is used to narrow representation distribution differences. To fuse learned representations and establish high-order interactions inside and between multimodal images, the hypergraph perceptual network is designed. Zong et al. (2024) developed a diffusion-based brain network pipeline for end-to-end brain network building. Using diffusion, the brain region-aware module (BRAM) correctly locates brain regions without subjective parameter selection. DGCL then uses graph contrastive learning to improve brain connections by reducing individual differences in redundant connections unrelated to diseases, improving brain network consistency within a group.
In Pan et al. (2024), the authors implemented a decoupling module that divides a brain network into two parts: sparse graphs representing neuronal circuits influencing AD progression and a supplement graph with minimal impact on AD. Additionally, the adversarial technique guides the decoupling module to extract AD-related features. Table 5 provides a comparison of a variety of studies that diagnose AD using a variety of AD approaches.
Upon reviewing the latest literature on the early diagnosis of Alzheimer's disease, it was found that to enhance overall performance and increase diagnostic accuracy through a computer program, the following considerations must be addressed: A significant problem is the acquisition of comprehensive and balanced data pertaining to Alzheimer's disease. Data augmentation and preprocessing methods can enhance the overall state-of-the-art performance. Many recently implemented approaches and techniques connected to deep learning are striving to address the previously described issues. All studies possess both advantages and disadvantages. This section addressed only five strategies of deep learning, despite the existence of numerous approaches, focusing on the most prevalent one for diagnosing Alzheimer's disease. This section examines five datasets and the existing literature from prior years of research.
4 Data set
Even though AD detection is challenging, researchers can use a variety of datasets and software packages to aid in their work. The DL model can be implemented using software packages like PyTorch, Keras, TensorFlow, Torch, and others. Publicly accessible biomarkers are provided via datasets (Popuri et al., 2020), like the ADNI, the open access series of imaging studies (OASIS), the Australian imaging biomarkers and lifestyle study of aging (AIBL), and MICAAI stands for medical image computing and computerized assistance intervention, and the Internet brain segmentation repository (IBSR). Table 6 shows the several datasets that DL techniques were utilized to identify AD.
4.1 ADNI
Nearly 90% of studies use ADNI, the most often used dataset in research, alone or in conjunction with other studies. The current go-to data source for studies on AD is ADNI. It was established in 2004 by the National Institutes of Health and the National Institute on Aging with the goal of compiling and organizing data on AD patients, tracking their progression, detecting pathogenesis-related alterations and causes, and finally discovering the origins of the disease and developing a therapy. The four stages of ADNI data are now separated into ADNI-GO, ADNI-1, ADNI-2, and ADNI-3, according to the authors of Dimitriadis et al. (2018). In comparison with ADNI-GO and ADNI-1, which are baseline data, the majority of the information in ADNI-2 and ADNI-3 is monitoring and newly added modal data. The basis of AD is primarily genetic.
To help researchers better understand the genetics behind illness causation, enabling the linking of genomes with neuroimaging and medical evidence is one of ADNI's main objectives. To monitor the onset of MCI and early AD, the main objective of ADNI is to ascertain whether ongoing MRI, PET, and biological samples may be used. Through the Laboratory on Neuroimaging (LONI), Image and Data Archive (IDA), a safe research data repository, all ADNI data are accessible without restriction. To conduct research, disseminate knowledge, or organize clinical research trials, interested scientists may have access to ADNI imaging, clinical, genomic, and biomarker data. Access is subject to compliance with the ADNI data use agreement and the policies indicated for the publications. Through the IDA, data from several dementia studies that supplement ADNI are also accessible. Among them are the ADNI project and the AIBL study, which examine the impact of traumatic brain injury and post-traumatic stress disorder on AD in veterans.
4.2 OASIS
OASIS-3 and OASIS-4 were recently provided by OASIS, an organization that aims to give the scientific community open access to neuroimaging datasets. Pre-published data for OASIS-cross-sectional (Marcus et al., 2007) and OASIS-longitudinal (Marcus et al., 2010) have been utilized in hypothesis-driven data analysis, neuroanatomical atlases, and segmentation algorithm development. The OASIS-3 dataset for normal aging and AD is a longitudinal multimodal neuroimaging, clinical, cognitive, and biomarker dataset. OASIS-4 contains MR, clinical, cognitive, and biomarker data for people who complained about their memory (Khagi et al., 2019).
The OASIS initiative, which comprises two large datasets, aims to provide open brain MRI data, according to the authors of Prasath and Sumathi (2024). The cross-sectional data collection comprised 416 people aged 18–96 with MRI results (people with dementia who are young, middle-aged, and old). For each MRI scan, a minimum of three to four high-resolution-to-noise ratio T1-weighted images were obtained. Intracranial volume measurements and total brain capacity were employed to investigate AD and normal aging (Bachman et al., 2014). The collection also contained information on 20 individuals who had dementia. The xnat.org central The OASIS datasets provide the public with free access to a substantial neuroimaging and processed imaging data source spanning a broad range of demographic, cognitive, and genetic characteristics, together with a user-friendly platform for usage in.
4.3 AIBL
The largest study, the AIBL initiative, uses PET scans. Participants also undergo a variety of other scans, such as a PET to evaluate brain function, a series of MRI contrasts for anatomical characterization (T1w, PDw, and T2w), the structural integrity of the white matter, and pathological imaging, in addition to the PET. MRI is a potent technique for capturing various brain tissues with excellent anatomical detail. Because MRI can evaluate the cortical atrophy linked to neurodegeneration, it has emerged as a crucial method for diagnosing AD. The accurate delineation of the outer cortical mantel is made possible, particularly by relatively quick T1w scans that provide excellent contrast between white and gray matter.
AIBL aims to pinpoint the biomarkers, cognitive characteristics, and lifestyle and health variables that impact the development of symptomatic AD. This includes biopsies, PET, MRI, clinical and cognitive tests, and lifestyle assessment (Martins et al., 2018). In the dataset, about 2,000 people's personal details are contained. The data was gathered using a variety of clinical procedures and surveys. Over a 10-year period, the entire data set was obtained, which included patient records for 582 normal individuals, 142 AD, and 220 MCI. Furthermore, the baseline cohort includes data on 786 healthy individuals, 133 MCI, and 211 AD. The recruitment times, gender, and age were used as proxy data.
4.4 IBSR
Initial funding for the IBSR came from the National Institute of Neurological Disorders and Stroke. Researchers from Boston University, Draper Laboratory, North Eastern University, Massachusetts Institute of Technology, Massachusetts General Hospital and Harvard Medical School use this award to research MR brain segmentation. Since the old website is no longer fully functional (Martins et al., 2018), all data will be made available at the respective website address.
After positional adjustment, the three-dimensional coronal T1-weighted medical examination is the MRI data. Often referred to as “outlines,” the segmentation files result from labor-intensive, semi-automated processes that require many hours of expertise from trained individuals. While manual segmentations aren't quite “ground truth,” they are a good place to start when contrasting different automated segmentation methods.
The IBSR offers carefully guided findings for expert segmentation and data from magnetic resonance brain images (Fatima et al., 2022). Its objective is to further segmentation technique analysis and progress. The ground truth is made up of 20 real T1-weighted (T1w) MRI pictures with carefully focused expert segmentation findings. Additionally, each MRI volume includes ~60 3.1 mm resolution coronal T1w slices and 18 1.5 mm resolution cortical T1w scans (slice gap between succeeding slices). With voxels separated at frequencies of 0.84 × 0.84 × 1.5 mm3 to 0.94 × 0.94 × 1.5 mm3 to 1.0 × 1.0 × 1.5 mm3, the topic volumes in this dataset have a size of 256 × 256 × 128 pixels. A manual division of 32 noncritical structures has also been performed.
4.5 MICCAI
The main objective of the MICCAI BraTS'21 challenge is to segment intrinsically heterogeneous brain glioma sub-regions in MRI scans using multi-institutional foundation multi-parametric magnetic resonance imaging (mpMRI) data. A significant pool of routine multi-institutional clinically acquired mpMRI scans of gliomas with a pathologically verified diagnosis and accessible O(6)-methylguanine-DNA methyltransferase promoter methylation status was used to generate the testing, training, and validation. Specifically, the data set used for this year's challenge has received a significant addition of routinely collected mpMRI images since BraTS'20. The validation, training, and testing datasets contain information about a single subject; experienced neuroradiologists produce and approve ground truth annotations of the tumor sub-regions to quantitatively evaluate the predicted tumor segmentations of Task 1. Clinical data is used to assess Task 2.
The MICCAI-2012 dataset (Landman and Warfield, 2012) includes 134 manually segmented structures from 35 T1w MRI volumes received from Scotts Valley, California-based Neuro-Metrics, Inc. The segmentation of tissue, tumors, and structures is its principal application. In 2012, this dataset began with 80 authentic and artificial examples. The amount of training and testing data has grown over time. Subcortical structure segmentation is accomplished using the MICCAI 2012 challenge in multi-atlas labeling. The study simulated various methods for accurately recognizing AD using brain MRI data. The ablation study considers how each contribution affects the effectiveness of the measures. The performance metrics of the suggested methods are contrasted with those of alternative strategies in the following section.
5 Performance measures
DL classification problems are frequently validated by sensitivity, specificity, precision, accuracy, Matthews Correlation Coefficient (MCC), F1 Score, Fine Motor Impairment (FMI), and comparing several models using receiver operating characteristics (ROC) and area under the curve (AUC) in particular to assess the effectiveness of recommendation systems or to comprehend generalizability (Luque et al., 2019).
Sensitivity: The classifier's ability to identify positive samples is enhanced by false negatives (Fn), the proportion of total positive samples the model interprets as true positives (Tp).
Specificity: The classifier's ability to identify negative samples is represented by the percentage of false positives (Fp) samples that the model determines to be true negatives (Tn).
Precision is the percentage of all findings that are deemed to be positive research.
MCC: The MCC indicator is more accurate because it considers Tp, Tn, Fp, and Fn. It is frequently used to assess categorization performance while working with dichotomies.
Accuracy: This represents the proportion of correct predictions to all other predictions.
Loss: For binary classification, apply binary cross-entropy loss, where y is the actual value and p is the predicted value.
where l is the loss value and M is the number of classes.
F1 score: This represents the harmonic mean of recall and precision. Its range is between 0 and 1. The model's performance improves with increasing the F1 score.
FMI refers to the geometric mean of the recall and precision rates. It functions as an external indicator for measuring the performance of clustering. Higher values lead to better clustering outcomes.
ROC: The curve for the operating characteristic of the receiver. The capacity of a classifier to differentiate between samples can be easily determined at a certain threshold. It needs to be more responsive to changes in the distribution of classes, simple, and intuitive. A comprehensive representation of the performance of a diagnostic approach is provided by the ROC curve, which precisely illustrates the relationship among the specificity and sensitivity of a particular analytical method and combines the two. The Tp vs. Fp plot for various thresholds in the interval [0, 1] provides an appropriate limit threshold for the predictive model.
The confusion matrix provides a matrix as output and serves as the foundation for other metrics that depend on the Tp, Tn, Fp, and Fn metrics. It is a comprehensive representation of the model's performance. The following section discusses the various challenges of an early diagnosis of AD.
6 Discussions
Predicting the transition from MCI to AD and identifying the initial stage of patients with MCI are more beneficial for the diagnostic work of AD. When it comes to efficiency, DL is superior to traditional ML. The efficacy of the AD detection technique is highly dependent on the accuracy of the neuroimaging. RoIs and patch-based strategies are critical. Combining genetic data, cognitive test scores, CSF biomarkers, and traits extracted from neuroimaging models can also lead to accurate classification. The research analyzed in this paper demonstrates that DL has been heavily used in AD detection. According to medical imaging studies, CNN end-to-end training has emerged as the favored method for medical imaging in recent years (AbdulAzeem et al., 2021). Even though DL techniques have produced encouraging findings, AD diagnosis is still quite difficult. While the proliferation of learning methodologies and the expansion of data have limited harmonization in survey and data gathering, insufficient data samples may still cause issues with generalization. This issue can be remedied by using data creation techniques to generate new images from the current methodology (Arbabyazd et al., 2021). The investigation in this field will continue despite the expansion of the dataset, as its efficacy remains uncertain.
In addition to this, image annotation (Choi et al., 2020) is another problem. Radiologists should annotate the image data provided for certain tasks when tagging medical images. The classification process is very time-consuming. This problem has been handled through supervised learning, and the requirement for expert experience has diminished; nevertheless, further research is still needed. Though algorithms for DL have produced amazing achievements, the area of AD detection still faces a number of challenges and limitations. A detailed description of the DL approach, benchmarking platform, and additional components is necessary in order to identify the optimal combination of several biomarkers (Meyer, 2018). Whether it can improve medical efficacy and reduce medical expenses is still debated. The primary limitation is the lack of research on image analysis of AD histology (Irankhah, 2020). Enhancing the DL approach is one of the most significant advances in improving these systems (Fu'Adah et al., 2021; Helaly et al., 2022). It summarizes the basic principles and information related to the provided survey.
6.1 Research gaps
This research study examines diverse methodologies to tackle the identified research issues in order to combat this global sickness. Investigation into effective and precise early detection of Alzheimer's disease is essential to facilitate timely treatment alternatives. Automated methods are necessary to manage the substantial volume of patients' medical imaging data. The fundamental etiology of the disease remains unidentified, with the exception of a limited number of family instances attributable to genetic mutation. At present, there is an absence of therapeutic interventions for the disease, necessitating a solution for the substantial amounts of imaging data required to manage a significant number of patients. A crucial viewpoint is the examination of longitudinal data. Alzheimer's disease is a degenerative condition that develops gradually, and documenting the dynamic alterations is essential for comprehending its progression.
6.2 Challenges
Moreover, it is essential to tackle the obstacles related to restricted data accessibility in Alzheimer's disease research. Deep learning methodologies frequently necessitate substantial quantities of labeled data for peak efficacy. Nevertheless, AD datasets are generally constrained by the challenges and expenses associated with data acquisition.
6.3 Future directions
Future research should concentrate on advancing strategies to improve the transparency and explainability of deep learning models in Alzheimer's disease diagnosis and prediction. Interpretability in medical applications is essential for fostering clinician trust and acceptance, as well as ensuring the ethical implementation of AI technologies. It is essential to include interpretable elements, such as attention mechanisms or saliency maps, into deep learning frameworks for Alzheimer's disease analysis. It is advisable to establish uniform benchmarks and evaluation criteria for deep learning activities linked to Alzheimer's disease to foster collaboration and expedite advancements in the field. This would enable equitable comparisons among various models and promote the repeatability of research results. Moreover, the dissemination of meticulously curated and annotated datasets helps mitigate the challenges posed by data scarcity and promote the advancement of innovative algorithms and approaches.
To address this difficulty, researchers may investigate transfer learning methodologies, wherein pre-trained models on analogous tasks or datasets are refined for Alzheimer's disease analysis. Furthermore, data augmentation techniques can artificially enhance the volume and diversity of accessible data, facilitating the development of more robust and generalizable models. Deep learning models ought to persist in investigating the integration of diverse data sources, including neuroimaging, genetics, and clinical information. Utilizing the complimentary information from different modalities can improve the precision of Alzheimer's disease diagnosis, prognosis, and treatment response prediction. The integration of multimodal data can yield a more holistic understanding of the condition and facilitate the formulation of individualized treatment approaches. Deep learning architectures possess significant potential for enhancing our comprehension of Alzheimer's disease and refining diagnosis, prognosis, and treatment. Integrating various modalities, evaluating longitudinal data, resolving data restrictions, improving interpretability, and promoting collaboration can facilitate the development of more accurate, efficient, and interpretable deep learning models in Alzheimer's disease research. These initiatives possess the capacity to revolutionize clinical practice and aid in the formulation of individualized and targeted interventions for those at risk of or impacted by Alzheimer's disease.
7 Conclusion
Because AD is hard to diagnose in its early stages, researchers are always searching for new approaches. This survey's primary topics are the pre-treatment approach, the technique for identifying traits associated with AD, and the application of deep models to AD diagnosis. This evaluation examined CNN's use in classifying AD, the datasets that were made publicly available, the neuroimaging modalities that were available, the pre-processing methods that were employed, and the data that were fed into the CNN. MRI is the modality most frequently used to classify AD. When complementary data from various modalities is used, multiple modalities' AD categorization results are more accurate than those of a single modality. Compared to slice-based and voxel-based technologies, the methods for managing patch and RoI data are significantly more efficient. Compared to other DL models, CNN is the most widely used and significant classification technique in this industry. The overfitting problem with the data set remains to be resolved, though. Study regions in medical images evolve due to self-monitoring and unsupervised operations brought on by a lack of medical information. Even though there are still many unanswered problems about how to classify AD, DL technology is still quite successful. There are situations when a person can detect AD better than a medical practitioner. A scientific study has also focused on using DL algorithms to identify MCI signs in MRI brain scans to halt the disease's growth and protect human life for a better, more peaceful future.
Author contributions
IN: Conceptualization, Investigation, Software, Methodology, Resources, Writing – original draft, Writing – review & editing. GL: Conceptualization, Supervision, Validation, Writing – original draft, Writing – review & editing.
Funding
The author(s) declare that no financial support was received for the research, authorship, and/or publication of this article.
Acknowledgments
The authors thank VIT for providing support and infrastructure for carrying out this research work.
Conflict of interest
The authors declare that the research was conducted in the absence of any commercial or financial relationships that could be construed as a potential conflict of interest.
Publisher's note
All claims expressed in this article are solely those of the authors and do not necessarily represent those of their affiliated organizations, or those of the publisher, the editors and the reviewers. Any product that may be evaluated in this article, or claim that may be made by its manufacturer, is not guaranteed or endorsed by the publisher.
Abbreviations
AD, Alzheimer's disease; DL, deep learning; ML, machine learning; MRI, magnetic resonance imaging; DTI, diffusion tensor imaging; CT, computed tomography; PET, positron emission tomography; CSF, cerebrospinal fluid; MCI, mild cognitive impairment; EMCI, early mild cognitive impairment; LMCI, late mild cognitive impairment; CN, cognitive normal; fMRI, functional MRI; sMRI, structural MRI; AI, artificial intelligence; ANN, artificial neural network; AE, Autoencoder; DBN, Deep belief network; DNN, deep neural network; CNN, convolutional neural network; RNN, recurrent neural network; DICOM, Digital Imaging and Communication in Medicine; AC, anterior commissure; PC, posterior commissure; MNI, Montreal Neurological Institute; GRU, gated recurrent units; LSTM, long short-term memory; ADNI, Alzheimer's disease neuroimaging initiative; OASIS, open access series of imaging studies; AIBL, Australian imaging biomarkers and lifestyle study of aging; IBSR, Internet Brain Segmentation Repository; MICCAI, medical image computing and computer-assisted intervention; IDA, image and data archive; MCC, Matthews Correlation Coefficient; FMI, fine motor impairment; ROC, receiver operating characteristics.
References
AbdulAzeem, Y., Bahgat, W. M., and Badawy, M. (2021). A CNN-based framework for classification of Alzheimer's disease. Neural Comput. Appl. 33, 10415–10428. doi: 10.1007/s00521-021-05799-w
Aderghal, K. (2021). Classification of multimodal MRI images using Deep Learning: Application to the diagnosis of Alzheimer's disease (Doctoral dissertation). Université de Bordeaux; Université Ibn Zohr, Agadir.
Aderghal, K., Boissenin, M., Benois-Pineau, J., Catheline, G., and Afdel, K. (2016). “Classification of sMRI for AD diagnosis with convolutional neuronal networks: a pilot 2-D+ study on ADNI,” in International Conference on Multimedia Modeling (Cham: Springer International Publishing), 690–701. doi: 10.1007/978-3-319-51811-4_56
Ahmed, S., Kim, B. C., Lee, K. H., Jung, H. Y., and Alzheimer's Disease Neuroimaging Initiative (2020). Ensemble of ROI-based convolutional neural network classifiers for staging the Alzheimer disease spectrum from magnetic resonance imaging. PLoS ONE 15:e0242712. doi: 10.1371/journal.pone.0242712
Ajagbe, S. A., Amuda, K. A., Oladipupo, M. A., Oluwaseyi, F. A., and Okesola, K. I. (2021). Multi-classification of Alzheimer disease on magnetic resonance images (MRI) using deep convolutional neural network (DCNN) approaches. Int. J. Adv. Comput. Res. 11:51. doi: 10.19101/IJACR.2021.1152001
Akkus, Z., Galimzianova, A., Hoogi, A., Rubin, D. L., and Erickson, B. J. (2017). Deep learning for brain MRI segmentation: state of the art and future directions. J. Digit. Imaging 30, 449–459. doi: 10.1007/s10278-017-9983-4
Albawi, S., Mohammed, T. A., and Al-Zawi, S. (2017). “Understanding of a convolutional neural network,” in 2017 international conference on engineering and technology (ICET) (Antalya: IEEE), 1–6. doi: 10.1109/ICEngTechnol.2017.8308186
Aldweesh, A., Derhab, A., and Emam, A. Z. (2020). Deep learning approaches for anomaly-based intrusion detection systems: a survey, taxonomy, and open issues. Knowl.-Based Syst. 189:105124. doi: 10.1016/j.knosys.2019.105124
Al-Naami, B., Gharaibeh, N., and Kheshman, A. A. (2013). Automated detection of Alzheimer disease using region growing technique and artificial neural network. World Acad. Sci. Eng. Technol. Int. J. Biomed. Biol. Eng. 7, 13–17. doi: 10.1145/334499
Alom, M. Z., Taha, T. M., Yakopcic, C., Westberg, S., Sidike, P., Nasrin, M. S., et al. (2019). A state-of-the-art survey on deep learning theory and architectures. Electronics 8:292. doi: 10.3390/electronics8030292
Alotaibi, B., and Alotaibi, M. (2020). A hybrid deep ResNet and inception model for hyperspectral image classification. PFG - J. Photogramm. Remote Sens. Geoinf. Sci. 88, 463–476. doi: 10.1007/s41064-020-00124-x
Al-Qatf, M., Lasheng, Y., Al-Habib, M., and Al-Sabahi, K. (2018). Deep learning approach combining sparse autoencoder with SVM for network intrusion detection. IEEE Access 6, 52843–52856. doi: 10.1109/ACCESS.2018.2869577
Amen, D. G. (2016). Change Your Brain, Change Your Life: Revised and Expanded Edition: The breakthrough program for conquering anxiety, depression, anger and obsessiveness. Hachette.
An, N., Jin, L., Ding, H., Yang, J., and Yuan, J. (2020). A deep belief network-based method to identify proteomic risk markers for Alzheimer disease. arXiv [Preprint]. arXiv:2003.05776. doi: 10.48550/arXiv.2003.05776
Andersen, E., Casteigne, B., Chapman, W. D., Creed, A., Foster, F., Lapins, A., et al. (2021). Diagnostic biomarkers in Alzheimer's disease. Biomark. Neuropsychiatry 5:100041. doi: 10.1016/j.bionps.2021.100041
Andriole, K. P. (2006). “Image acquisition,” in PACS: A Guide to the Digital Revolution (New York, NY: Springer New York), 189–227. doi: 10.1007/0-387-31070-3_11
Arbabyazd, L., Shen, K., Wang, Z., Hofmann-Apitius, M., Ritter, P., McIntosh, A. R., et al. (2021). Virtual connectomic datasets in Alzheimer's disease and aging using whole-brain network dynamics modelling. Eneuro 8, 1–33. doi: 10.1523/ENEURO.0475-20.2021
Atri, A. (2019). The Alzheimer's disease clinical spectrum: diagnosis and management. Med. Clinics 103, 263–293. doi: 10.1016/j.mcna.2018.10.009
Avots, E., Jafari, A. A., Ozcinar, C., Anbarjafari, G., and Alzheimer's Disease Neuroimaging Initiative (2024). Comparative efficacy of histogram-based local descriptors and CNNs in the MRI-based multidimensional feature space for the differential diagnosis of Alzheimer's disease: a computational neuroimaging approach. Signal Image Video Process. 18, 1–13. doi: 10.1007/s11760-023-02942-z
Ayodele, T. O. (2010). Types of machine learning algorithms. New Adv. Mach. Learn. 3, 5–1. doi: 10.5772/9385
Bachman, A. H., Lee, S. H., Sidtis, J. J., and Ardekani, B. A. (2014). Corpus callosum shape and size changes in early Alzheimer's disease: a longitudinal MRI study using the OASIS brain database. J. Alzheimers Dis. 39, 71–78. doi: 10.3233/JAD-131526
Bardis, M. D., Houshyar, R., Chang, P. D., Ushinsky, A., Glavis-Bloom, J., Chahine, C. S., et al. (2020). Applications of artificial intelligence to prostate multiparametric MRI (mpMRI): current and emerging trends. Cancers 12:1204. doi: 10.3390/cancers12051204
Basaia, S., Agosta, F., Wagner, L., Canu, E., Magnani, G., Santangelo, R., et al. (2019). Automated classification of Alzheimer's disease and mild cognitive impairment using a single MRI and deep neural networks. NeuroImage: Clin. 21:101645. doi: 10.1016/j.nicl.2018.101645
Basher, A., Kim, B. C., Lee, K. H., and Jung, H. Y. (2021). Volumetric feature-based Alzheimer's disease diagnosis from sMRI data using a convolutional neural network and a deep neural network. IEEE Access 9, 29870–29882. doi: 10.1109/ACCESS.2021.3059658
Baskar, D., Jayanthi, V. S., and Jayanthi, A. N. (2019). An efficient classification approach for detection of Alzheimer's disease from biomedical imaging modalities. Multimed. Tools Appl. 78, 12883–12915. doi: 10.1007/s11042-018-6287-8
Bhandare, A., Bhide, M., Gokhale, P., and Chandavarkar, R. (2016). Applications of convolutional neural networks. Int. J. Comput. Sci. Inf. Technol. 7, 2206–2215. doi: 10.25079/ukhjse.v3n2y2019.pp31-40
Bi, X., Zhao, X., Huang, H., Chen, D., and Ma, Y. (2020). Functional brain network classification for Alzheimer's disease detection with deep features and an extreme learning machine. Cognit. Comput. 12, 513–527. doi: 10.1007/s12559-019-09688-2
Borghesani, P. R., Johnson, L. C., Shelton, A. L., Peskind, E. R., Aylward, E. H., Schellenberg, G. D., et al. (2008). Altered medial temporal lobe responses during visuospatial encoding in healthy APOE* 4 carriers. Neurobiol. Aging 29, 981–991. doi: 10.1016/j.neurobiolaging.2007.01.012
Brown, E. M., Pierce, M. E., Clark, D. C., Fischl, B. R., Iglesias, J. E., Milberg, W. P., et al. (2020). Test-retest reliability of FreeSurfer automated hippocampal subfield segmentation within and across scanners. Neuroimage 210:116563. doi: 10.1016/j.neuroimage.2020.116563
Brown, J. (2015). The use and misuse of short cognitive tests in the diagnosis of dementia. J. Neurol. Neurosurg. Psychiatry 86, 680–685. doi: 10.1136/jnnp-2014-309086
Busche, M. A., Eichhoff, G., Adelsberger, H., Abramowski, D., Wiederhold, K. H., Haass, C., et al. (2008). Clusters of hyperactive neurons near amyloid plaques in a mouse model of Alzheimer's disease. Science 321, 1686–1689. doi: 10.1126/science.1162844
Çayir, A., Yenidogan, I., and Dag, H. (2018). “Feature extraction based on deep learning for some traditional machine learning methods,” in 2018 3rd International conference on computer science and engineering (UBMK) (Sarajevo: IEEE), 494–497. doi: 10.1109/UBMK.2018.8566383
Chakraborty, S., Tomsett, R., Raghavendra, R., Harborne, D., Alzantot, M., Cerutti, F., et al. (2017). “Interpretability of deep learning models: a survey of results,” in 2017 IEEE smartworld, ubiquitous intelligence and computing, advanced and trusted computed, scalable computing and communications, cloud and big data computing, Internet of people and smart city innovation (smartworld/SCALCOM/UIC/ATC/CBDcom/IOP/SCI) (San Francisco, CA: IEEE), 1–6. doi: 10.1109/UIC-ATC.2017.8397411
Chen, J., Yan, S., and Wong, K. C. (2020). Verbal aggression detection on Twitter comments: convolutional neural network for short-text sentiment analysis. Neural Comput. Appl. 32, 10809–10818. doi: 10.1007/s00521-018-3442-0
Chen, M., Shi, X., Zhang, Y., Wu, D., and Guizani, M. (2017). Deep feature learning for medical image analysis with convolutional autoencoder neural networks. IEEE Trans. Big Data 7, 750–758. doi: 10.1109/TBDATA.2017.2717439
Chen, Z., Zhang, T., and Ouyang, C. (2018). End-to-end airplane detection using transfer learning in remote sensing images. Remote Sens. 10:139. doi: 10.3390/rs10010139
Chitradevi, D., and Prabha, S. (2020). Analysis of brain subregions using optimization techniques and deep learning methods in Alzheimer disease. Appl. Soft Comput. 86:105857. doi: 10.1016/j.asoc.2019.105857
Choi, B. K., Madusanka, N., Choi, H. K., So, J. H., Kim, C. H., Park, H. G., et al. (2020). Convolutional neural network-based MR image analysis for Alzheimer's disease classification. Curr. Med. Imaging 16, 27–35. doi: 10.2174/1573405615666191021123854
Deng, L., and Platt, J. (2014). “Ensemble deep learning for speech recognition,” in Proc. interspeech (Redmond, WA: Microsoft Research, One Microsoft Way). doi: 10.21437/Interspeech.2014-433
Dimitriadis, S. I., Liparas, D., Tsolaki, M. N., and Alzheimer's Disease Neuroimaging Initiative. (2018). Random forest feature selection, fusion and ensemble strategy: Combining multiple morphological MRI measures to discriminate among healthy elderly, MCI, cMCI and Alzheimer's disease patients: from the Alzheimer's disease neuroimaging initiative (ADNI) database. J. Neurosci. Methods 302, 14–23. doi: 10.1016/j.jneumeth.2017.12.010
Ding, Y., Sohn, J. H., Kawczynski, M. G., Trivedi, H., Harnish, R., Jenkins, N. W., et al. (2019). A deep learning model to predict a diagnosis of Alzheimer disease by using 18F-FDG PET of the brain. Radiology 290, 456–464. doi: 10.1148/radiol.2018180958
DiPietro, R., and Hager, G. D. (2020). “Deep learning: RNNs and LSTM,” in The Handbook of Medical Image Computing and Computer-Assisted Intervention, eds. S. K. Zhou, D. Rueckert, and G. Fichtinger (New York, Academic PRess), 503–519. doi: 10.1016/B978-0-12-816176-0.00026-0
Douaud, G., Jbabdi, S., Behrens, T. E., Menke, R. A., Gass, A., Monsch, A. U., et al. (2011). DTI measures in crossing-fibre areas: increased diffusion anisotropy reveals early white matter alteration in MCI and mild Alzheimer's disease. Neuroimage 55, 880–890. doi: 10.1016/j.neuroimage.2010.12.008
Dubois, B., Picard, G., and Sarazin, M. (2009). Early detection of Alzheimer's disease: new diagnostic criteria. Dialogues Clin. Neurosci. 11, 135–139. doi: 10.31887/DCNS.2009.11.2/bdubois
Ebrahimighahnavieh, M. A., Luo, S., and Chiong, R. (2020). Deep learning to detect Alzheimer's disease from neuroimaging: a systematic literature review. Comput. Methods Programs Biomed. 187:105242. doi: 10.1016/j.cmpb.2019.105242
Esmaeilzadeh, S., Belivanis, D. I., Pohl, K. M., and Adeli, E. (2018). “End-to-end Alzheimer's disease diagnosis and biomarker identification,” in Machine Learning in Medical Imaging: 9th International Workshop, MLMI 2018, Held in Conjunction with MICCAI 2018, Granada, Spain, September 16, 2018, Proceedings 9 (Cham: Springer International Publishing), 337–345. doi: 10.1007/978-3-030-00919-9_39
Faisal, F. U. R., and Kwon, G. R. (2022). Automated detection of Alzheimer's disease and mild cognitive impairment using whole-brain MRI. IEEE Access 10, 65055–65066. doi: 10.1109/ACCESS.2022.3180073
Fatima, A., Madni, T. M., Anwar, F., Janjua, U. I., and Sultana, N. (2022). Automated 2D slice-based skull stripping multi-view ensemble model on NFBS and IBSR datasets. J. Digit. Imaging 35, 374–384. doi: 10.1007/s10278-021-00560-0
Faturrahman, M., Wasito, I., Hanifah, N., and Mufidah, R. (2017). “Structural MRI classification for Alzheimer's disease detection using deep belief networks,” in 2017 11th International Conference on Information and Communication Technology and System (ICTS) (Surabaya: IEEE), 37–42. doi: 10.1109/ICTS.2017.8265643
Firdaus, N. M., Chahyati, D., and Fanany, M. I. (2018). “Tourist attractions classification using ResNet,” in 2018 International Conference on Advanced Computer Science and Information Systems (ICACSIS) (Indonesia: IEEE), 429–433. doi: 10.1109/ICACSIS.2018.8618235
Forouzannezhad, P., Abbaspour, A., Fang, C., Cabrerizo, M., Loewenstein, D., Duara, R., et al. (2019). A survey on applications and analysis methods of functional magnetic resonance imaging for Alzheimer's disease. J. Neurosci. Methods 317, 121–140. doi: 10.1016/j.jneumeth.2018.12.012
Fu'Adah, Y. N., Wijayanto, I., Pratiwi, N. K. C., Taliningsih, F. F., Rizal, S., and Pramudito, M. A. (2021). Automated classification of Alzheimer's disease based on MRI image processing using convolutional neural network (CNN) with AlexNet architecture. J. Phys. Conf. Ser. 1844:012020. doi: 10.1088/1742-6596/1844/1/012020
Gao, S., and Lima, D. (2022). A review of the application of deep learning in the detection of Alzheimer's disease. Int. J. Cogn. Comput. Eng. 3, 1–8. doi: 10.1016/j.ijcce.2021.12.002
Greenhalgh, T., and Peacock, R. (2005). Effectiveness and efficiency of search methods in systematic reviews of complex evidence: audit of primary sources. BMJ 331, 1064–1065. doi: 10.1136/bmj.38636.593461.68
Guo, K., Xu, T., Kui, X., Zhang, R., and Chi, T. (2019). iFusion: towards efficient intelligence fusion for deep learning from real-time and heterogeneous data. Inf. Fusion 51, 215–223. doi: 10.1016/j.inffus.2019.02.008
Hamdi, M., Bourouis, S., Rastislav, K., and Mohmed, F. (2022). Evaluation of neuroimages for the diagnosis of Alzheimer's disease using deep learning neural networks. Front Public Health 10:834032. doi: 10.3389/fpubh.2022.834032
Healy, S., McMahon, J., and FitzGerald, U. (2018). UPR induction prevents iron accumulation and oligodendrocyte loss in ex vivo cultured hippocampal slices. Front. Neurosci. 12:424726. doi: 10.3389/fnins.2018.00969
Helaly, H. A., Badawy, M., and Haikal, A. Y. (2022). Deep learning approach for early detection of Alzheimer's disease. Cognit. Comput. 14, 1711–1727. doi: 10.1007/s12559-021-09946-2
Hennig, J., Speck, O., Koch, M. A., and Weiller, C. (2003). Functional magnetic resonance imaging: a review of methodological aspects and clinical applications. J. Magn. Reson. Imaging 18, 1–15. doi: 10.1002/jmri.10330
Hon, M., and Khan, N. M. (2017). “Towards Alzheimer's disease classification through transfer learning,” in 2017 IEEE International conference on bioinformatics and biomedicine (BIBM) (Kansas City, MO: IEEE), 1166–1169. doi: 10.1109/BIBM.2017.8217822
Hong, X., Lin, R., Yang, C., Zeng, N., Cai, C., Gou, J., et al. (2019). Predicting Alzheimer's disease using LSTM. IEEE Access 7, 80893–80901. doi: 10.1109/ACCESS.2019.2919385
Hosseini, M. P., Lu, S., Kamaraj, K., Slowikowski, A., and Venkatesh, H. C. (2020). “Deep learning architectures,” in Deep Learning: Concepts and Architectures, eds. W. Pedrycz, and S. M. Chen (Cham: Springer), 1–24. doi: 10.1007/978-3-030-31756-0_1
Huang, Z., Zhu, X., Ding, M., and Zhang, X. (2020). Medical image classification using a lightweight hybrid neural network based on PCANet and DenseNet. IEEE Access 8, 24697–24712. doi: 10.1109/ACCESS.2020.2971225
Irankhah, E. (2020). Evaluation of early detection methods for Alzheimer's. Bioprocess Eng. 4, 17–22. doi: 10.11648/j.be.20200401.13
Islam, T., and Manivannan, D. (2017). “Predicting application failure in the cloud: a machine learning approach,” in 2017 IEEE International Conference on Cognitive Computing (ICCC) (Honolulu, HI: IEEE), 24–31. doi: 10.1109/IEEE.ICCC.2017.11
Jack Jr, C. R., Bennett, D. A., Blennow, K., Carrillo, M. C., Dunn, B., Haeberlein, S. B., et al. (2018). NIA-AA research framework: toward a biological definition of Alzheimer's disease. Alzheimers Dement. 14, 535–562. doi: 10.1016/j.jalz.2018.02.018
Jain, R., Jain, N., Aggarwal, A., and Hemanth, D. J. (2019). Convolutional neural network-based Alzheimer's disease classification from magnetic resonance brain images. Cogn. Syst. Res. 57, 147–159. doi: 10.1016/j.cogsys.2018.12.015
Janghel, R. R., and Rathore, Y. K. (2021). Deep convolution neural network-based system for early diagnosis of Alzheimer's disease. Irbm 42, 258–267. doi: 10.1016/j.irbm.2020.06.006
JayaLakshmi, A. N. M., and Kishore, K. K. (2022). Performance evaluation of DNN with other machine learning techniques in a cluster using Apache Spark and MLlib. J. King Saud Univ. - Comput. Inf. Sci. 34, 1311–1319. doi: 10.1016/j.jksuci.2018.09.022
Jiao, J., Bousse, A., Thielemans, K., Burgos, N., Weston, P. S., Schott, J. M., et al. (2016). Direct parametric reconstruction with joint motion estimation and correction for dynamic brain PET data. IEEE Trans. Med. Imaging 36, 203–213. doi: 10.1109/TMI.2016.2594150
Karwath, A., Hubrich, M., Kramer, S., and Alzheimer's Disease Neuroimaging Initiative (2017). “Convolutional neural networks for the identification of regions of interest in PET scans: a study of representation learning for diagnosing Alzheimer's disease,” in Conference on Artificial Intelligence in Medicine in Europe (Cham: Springer International Publishing), 316–321. doi: 10.1007/978-3-319-59758-4_36
Katabathula, S., Wang, Q., and Xu, R. (2021). Predict Alzheimer's disease using hippocampus MRI data: a lightweight 3D deep convolutional network model with visual and global shape representations. Alzheimers Res. Ther. 13:104. doi: 10.1186/s13195-021-00837-0
Khagi, B., Lee, B., Pyun, J. Y., and Kwon, G. R. (2019). “CNN Models performance analysis on MRI images of the OASIS dataset for distinction between healthy and Alzheimer's patients,” in 2019 International Conference on Electronics, Information, and Communication (ICEIC) (Auckland: IEEE), 1–4. doi: 10.23919/ELINFOCOM.2019.8706339
Kim, H. W., Lee, H. E., Oh, K., Lee, S., Yun, M., Yoo, S. K., et al. (2020). Multi-slice representational learning of convolutional neural networks for Alzheimer's disease classification using positron emission tomography. Biomed. Eng. Online 19, 1–15. doi: 10.1186/s12938-020-00813-z
Kundaram, S. S., and Pathak, K. C. (2021). “Deep learning-based Alzheimer disease detection,” in Proceedings of the Fourth International Conference on Microelectronics, Computing and Communication Systems: MCCS 2019 (Cham: Springer Singapore), 587–597. doi: 10.1007/978-981-15-5546-6_50
Landman, B. A., and Warfield, S. (2012). “MICCAI 2012: grand challenge and workshop on multi-atlas labeling,” in Proc. international conference on medical image computing and computer assisted intervention, MICCAI (Vol. 2012) (Nice).
Lazli, L., and Boukadoum, M. (2018). “Tissue segmentation by fuzzy clustering technique: case study on Alzheimer's disease,” in Medical Imaging 2018: Imaging Informatics for Healthcare, Research, and Applications, Vol. 10579 (Houston, TX: SPIE), 399–409. doi: 10.1117/12.2294545
Lee, B., Ellahi, W., and Choi, J. Y. (2019). Using deep CNN with a data permutation scheme for classification of Alzheimer's disease in structural magnetic resonance imaging (sMRI). IEICE Trans. Inf. Syst. 102, 1384–1395. doi: 10.1587/transinf.2018EDP7393
Lerch, J. P., Pruessner, J., Zijdenbos, A. P., Collins, D. L., Teipel, S. J., Hampel, H., et al. (2008). Automated cortical thickness measurements from MRI can accurately separate Alzheimer's patients from normal elderly controls. Neurobiol. Aging 29, 23–30. doi: 10.1016/j.neurobiolaging.2006.09.013
Li, F., Tran, L., Thung, K. H., Ji, S., Shen, D., Li, J., et al. (2015). A robust deep model for improved classification of AD/MCI patients. IEEE J. Biom. Health Inf. 19, 1610–1616. doi: 10.1109/JBHI.2015.2429556
Li, H., Yu, L., Tian, S., Li, L., Wang, M., Lu, X., et al. (2017). Deep learning in pharmacy: the prediction of aqueous solubility based on deep belief networks. Autom. Control Compu. Sci. 51, 97–107. doi: 10.3103/S0146411617020043
Lian, C., Liu, M., Zhang, J., and Shen, D. (2018). Hierarchical, fully convolutional network for joint atrophy localization and Alzheimer's disease diagnosis using structural MRI. IEEE Trans. Pattern Anal. Mach. Intell. 42, 880–893. doi: 10.1109/TPAMI.2018.2889096
Liang, S., and Gu, Y. (2020). Computer-aided diagnosis of Alzheimer's disease through weak supervision and a deep learning framework with attention mechanisms. Sensors 21:220. doi: 10.3390/s21010220
Liberati, A., Altman, D. G., Tetzlaff, J., Mulrow, C., Gøtzsche, P. C., Ioannidis, J. P., et al. (2009). The PRISMA statement for reporting systematic reviews and meta-analyses of studies that evaluate health care interventions: explanation and elaboration. Ann. Intern. Med. 151, W65–W94. doi: 10.7326/0003-4819-151-4-200908180-00136
Liu, D., Xu, Y., Elazab, A., Yang, P., Wang, W., Wang, T., et al. (2020). “Longitudinal analysis of mild cognitive impairment via sparse smooth network and attention-based stacked bi-directional long-short term memory,” in 2020, IEEE 17th International Symposium on Biomedical Imaging (ISBI) (Iowa City, IA: IEEE), 1–4. doi: 10.1109/ISBI45749.2020.9098727
Liu, M., Zhang, J., Adeli, E., and Shen, D. (2018). Landmark-based deep multi-instance learning for brain disease diagnosis. Med. Image Anal. 43, 157–168. doi: 10.1016/j.media.2017.10.005
Liu, S., Cai, W., Pujol, S., Kikinis, R., and Feng, D. (2014b). “Early diagnosis of Alzheimer's disease with deep learning,” in 2014 IEEE 11th international symposium on biomedical imaging (ISBI) (Beijing: IEEE), 1015–1018. doi: 10.1109/ISBI.2014.6868045
Liu, S., Liu, S., Cai, W., Che, H., Pujol, S., and Kikinis, R. (2014a). Multimodal neuroimaging feature learning for multiclass diagnosis of Alzheimer's disease. IEEE Trans. Biomed. Eng. 62, 1132–1140. doi: 10.1109/TBME.2014.2372011
Lu, S., Lu, Z., and Zhang, Y. D. (2019). Pathological brain detection based on AlexNet and transfer learning. J. Comput. Sci. 30, 41–47. doi: 10.1016/j.jocs.2018.11.008
Luque, A., Carrasco, A., Martín, A., and de Las Heras, A. (2019). The impact of class imbalance in classification performance metrics based on the binary confusion matrix. Pattern Recognit. 91, 216–231. doi: 10.1016/j.patcog.2019.02.023
Mahrishi, M., Hiran, K. K., Meena, G., and Sharma, P. (Eds.) (2020). Machine Learning and Deep Learning in Real-time Applications. London: IGI Global. doi: 10.4018/978-1-7998-3095-5
Marcus, D. S., Fotenos, A. F., Csernansky, J. G., Morris, J. C., and Buckner, R. L. (2010). Open access series of imaging studies: longitudinal MRI data in nondemented and demented older adults. J. Cogn. Neurosci. 22, 2677–2684. doi: 10.1162/jocn.2009.21407
Marcus, D. S., Wang, T. H., Parker, J., Csernansky, J. G., Morris, J. C., Buckner, R. L., et al. (2007). Open Access Series of Imaging Studies (OASIS): cross-sectional MRI data in young, middle-aged, nondemented, and demented older adults. J. Cogn. Neurosci. 19, 1498–1507. doi: 10.1162/jocn.2007.19.9.1498
Martins, R. N., Villemagne, V., Sohrabi, H. R., Chatterjee, P., Shah, T. M., Verdile, G., et al. (2018). Alzheimer's disease: a journey from amyloid peptides and oxidative stress to biomarker technologies and disease prevention strategies—gains from AIBL and DIAN cohort studies. J. Alzheimers Dis. 62, 965–992. doi: 10.3233/JAD-171145
Marzban, E. N., Eldeib, A. M., Yassine, I. A., Kadah, Y. M., and Alzheimer's Disease Neurodegenerative Initiative. (2020). Alzheimer's disease diagnosis from diffusion tensor images using convolutional neural networks. PLoS ONE 15:e0230409. doi: 10.1371/journal.pone.0230409
Meng, L., and Zhang, Q. (2023). Research on early diagnosis of Alzheimer's disease based on a dual fusion cluster graph convolutional network. Biomed. Signal Process. Control 86:105212. doi: 10.1016/j.bspc.2023.105212
Meyer, F. (2018). “Toward a biological definition of Alzheimer's disease,” in Datablitz des neurosciences cliniques-ULiège.
Nawaz, H., Maqsood, M., Afzal, S., Aadil, F., Mehmood, I., Rho, S., et al. (2021). A deep feature-based real-time system for Alzheimer disease stage detection. Multimed. Tools Appl. 80, 35789–35807. doi: 10.1007/s11042-020-09087-y
Nguyen, H., and Chu, N. N. (2020). An introduction to deep learning research for Alzheimer's disease. IEEE Consum. Electron. Mag. 10, 72–75. doi: 10.1109/MCE.2020.3048254
Nicholson, C., and Gibson, A. (2018). A Beginner's Guide to LSTMs and Recurrent Neural Networks. San Francisco, CA: Skymind.
Noda, K., Yamaguchi, Y., Nakadai, K., Okuno, H. G., and Ogata, T. (2015). Audio-visual speech recognition using deep learning. App. Intell. 42, 722–737. doi: 10.1007/s10489-014-0629-7
Noor, M. B. T., Zenia, N. Z., Kaiser, M. S., Mamun, S. A., and Mahmud, M. (2020). Application of deep learning in detecting neurological disorders from magnetic resonance images: a survey on the detection of Alzheimer's disease, Parkinson's disease, and schizophrenia. Brain Inf. 7, 1–21. doi: 10.1186/s40708-020-00112-2
Ortiz, A., Munilla, J., Gorriz, J. M., and Ramirez, J. (2016). Ensembles of deep learning architectures for the early diagnosis of Alzheimer's disease. Int. J. Neural Syst. 26:1650025. doi: 10.1142/S0129065716500258
Ortiz, A., Munilla, J., Martínez-Murcia, F. J., Górriz, J. M., Ramírez, J., and Alzheimer's Disease Neuroimaging Initiative (2017). “Learning longitudinal MRI patterns by SICE and deep learning: Assessing the Alzheimer's disease progression,” in Medical Image Understanding and Analysis: 21st Annual Conference, MIUA 2017, Edinburgh, UK, July 11–13, 2017, Proceedings 21 (Cham: Springer International Publishing), 413–424. doi: 10.1007/978-3-319-60964-5_36
Otter, D. W., Medina, J. R., and Kalita, J. K. (2020). A survey of the usages of deep learning for natural language processing. IEEE Trans. Neural Netw. Learn. Syst. 32, 604–624. doi: 10.1109/TNNLS.2020.2979670
Pak, M., and Kim, S. (2017). “A review of deep learning in image recognition,” in 2017, 4th international conference on computer applications and information processing technology (CAIPT) (Kuta Bali: IEEE), 1–3. doi: 10.1109/CAIPT.2017.8320684
Pan, J., Zuo, Q., Wang, B., Chen, C. P., Lei, B., Wang, S., et al. (2024). Decgan: decoupling generative adversarial networks for detecting abnormal neural circuits in Alzheimer's disease. IEEE Trans. Artif. Intell. 5, 5050–5063. doi: 10.1109/TAI.2024.3416420
Panza, F., Frisardi, V., Capurso, C., D'Introno, A., Colacicco, A. M., Imbimbo, B. P., et al. (2010). Late-life depression, mild cognitive impairment, and dementia: possible continuum? Am. J. Geriatr. Psychiatry 18, 98–116. doi: 10.1097/JGP.0b013e3181b0fa13
Park, C., Ha, J., and Park, S. (2020). Prediction of Alzheimer's disease based on a deep neural network by integrating gene expression and DNA methylation datasets. Expert Syst. Appl. 140:112873. doi: 10.1016/j.eswa.2019.112873
Paul, S., Pachipulusu, B., Kumar, T., and Chandra, P. (2024). DICOM: a revolution in facet of maxillofacial imaging. J. Clin. Diagn. Res. 18:18921. doi: 10.7860/JCDR/2024/66403.18921
Payan, A., and Montana, G. (2015). Predicting Alzheimer's disease: a neuroimaging study with 3D convolutional neural networks. arXiv [Preprint]. arXiv:1502.02506. doi: 10.48550/arXiv.1502.02506
Pei, Z., Gou, Y., Ma, M., Guo, M., Leng, C., Chen, Y., et al. (2022). Alzheimer's disease diagnosis based on long-range dependency mechanisms using convolutional neural networks. Multimed. Tools Appl. 81, 1–16. doi: 10.1007/s11042-021-11279-z
Pierce, A. L., Bullain, S. S., and Kawas, C. H. (2017). Late-onset Alzheimer disease. Neurol. Clin. 35, 283–293. doi: 10.1016/j.ncl.2017.01.006
Platero, C., and Tobar, M. C. (2017). Combining a patch-based approach with a non-rigid registration-based label fusion method for the hippocampal segmentation in Alzheimer's disease. Neuroinformatics 15, 165–183. doi: 10.1007/s12021-017-9323-3
Popuri, K., Ma, D., Wang, L., and Beg, M. F. (2020). Using machine learning to quantify structural MRI neurodegeneration patterns of Alzheimer's disease into dementia score: independent validation on 8,834 images from ADNI, AIBL, OASIS, and MIRIAD databases. Hum. Brain Mapp. 41, 4127–4147. doi: 10.1002/hbm.25115
Prasath, T., and Sumathi, V. (2024). Pipelined deep learning architecture for the detection of Alzheimer's disease. Biomed. Signal Process. Control 87:105442. doi: 10.1016/j.bspc.2023.105442
Puttagunta, M., and Ravi, S. (2021). Medical image analysis is based on a deep learning approach. Multimed. Tools Appl. 80, 24365–24398. doi: 10.1007/s11042-021-10707-4
Qiu, S., Joshi, P. S., Miller, M. I., Xue, C., Zhou, X., Karjadi, C., et al. (2020). Development and validation of an interpretable deep learning framework for Alzheimer's disease classification. Brain 143, 1920–1933. doi: 10.1093/brain/awaa137
Rajasree, R. S., and Brintha Rajakumari, S. (2024). Ensemble-of-classifiers-based approach for early Alzheimer's disease detection. Multimed. Tools Appl. 83, 16067–16095. doi: 10.1007/s11042-023-16023-3
Ramzan, F., Khan, M. U. G., Rehmat, A., Iqbal, S., Saba, T., Rehman, A., et al. (2020). A deep learning approach for automated diagnosis and multi-class classification of Alzheimer's disease stages using resting-state fMRI and residual neural networks. J. Med. Syst. 44, 1–16. doi: 10.1007/s10916-019-1475-2
Ravi, V., Gopalakrishnan, E. A., and Soman, K. P. (2024). Deep learning-based approach for multi-stage diagnosis of Alzheimer's disease. Multimed. Tools Appl. 83, 16799–16822. doi: 10.1007/s11042-023-16026-0
Ren, F., Yang, C., Qiu, Q., Zeng, N., Cai, C., Hou, C., et al. (2019). Exploiting discriminative regions of brain slices based on 2D CNNs for Alzheimer's disease classification. IEEE Access 7, 181423–181433. doi: 10.1109/ACCESS.2019.2920241
Ruiz, L., Gama, F., and Ribeiro, A. (2020). Gated graph recurrent neural networks. IEEE Trans. Signal Process. 68, 6303–6318. doi: 10.1109/TSP.2020.3033962
Sakatani, K., Oyama, K., and Hu, L. (2020). Deep learning-based screening test for cognitive impairment using basic blood test data for health examination. Front. Neurol. 11:588140. doi: 10.3389/fneur.2020.588140
Sarraf, S., DeSouza, D. D., Anderson, J., Tofighi, G., and Alzheimer's Disease Neuroimaging Initiative (2016). DeepAD: Alzheimer's disease classification via deep convolutional neural networks using MRI and fMRI. bioRxiv 070441. doi: 10.1101/070441
Satapathy, S. C. (2020). COVID-19 diagnosis via DenseNet and optimization of transfer learning settings. Cogn. Comput. 7, 1–17. doi: 10.1007/s12559-020-09776-8
Shankar, G. M., and Walsh, D. M. (2009). Alzheimer's disease: synaptic dysfunction and Aβ. Mol. Neurodegener. 4, 1–13. doi: 10.1186/1750-1326-4-48
Spreng, R. N., Dimas, E., Mwilambwe-Tshilobo, L., Dagher, A., Koellinger, P., Nave, G., et al. (2020). The default network of the human brain is associated with perceived social isolation. Nat. Commun. 11:6393. doi: 10.1038/s41467-020-20039-w
Suk, H. I., Lee, S. W., Shen, D, and Alzheimer's Disease Neuroimaging Initiative (2014). Hierarchical feature representation and multimodal fusion with deep learning for AD/MCI diagnosis. Neuroimage 101, 569–582. doi: 10.1016/j.neuroimage.2014.06.077
Suk, H. I., Wee, C. Y., Lee, S. W., and Shen, D. (2016). State-space model with deep learning for functional dynamics estimation in resting-state fMRI. Neuroimage 129, 292–307. doi: 10.1016/j.neuroimage.2016.01.005
Sun, H., Wang, A., Wang, W., and Liu, C. (2021). An improved deep residual network prediction model for the early diagnosis of Alzheimer's disease. Sensors 21:4182. doi: 10.3390/s21124182
Takao, H., Amemiya, S., Abe, O., and Alzheimer's Disease Neuroimaging Initiative (2021). Reliability of changes in brain volume determined by longitudinal voxel-based morphometry. J. Magn. Reson. Imaging 54, 609–616. doi: 10.1002/jmri.27568
Theodore, W. H., Dorwart, R., Holmes, M., Porter, R. J., and DiChiro, G. (1986). Neuroimaging in refractory partial seizures: comparison of PET, CT, and MRI. Neurology 36, 750–750. doi: 10.1212/WNL.36.6.750
Tong, T., Wolz, R., Gao, Q., Guerrero, R., Hajnal, J. V., Rueckert, D., et al. (2014). Multiple instance learning for classification of dementia in brain MRI. Med. Image Anal. 18, 808–818. doi: 10.1016/j.media.2014.04.006
Tufail, A. B., Ullah, I., Rehman, A. U., Khan, R. A., Khan, M. A., Ma, Y. K., et al. (2022). On disharmony in batch normalization and dropout methods for early categorization of Alzheimer's disease. Sustainability 14:14695. doi: 10.3390/su142214695
Verfaillie, S. C., Binette, A. P., Vachon-Presseau, E., Tabrizi, S., Savard, M., Bellec, P., et al. (2018). Subjective cognitive decline is associated with altered default mode network connectivity in individuals with a family history of Alzheimer's disease. Biol. Psychiatry Cogn. Neurosci. Neuroimaging 3, 463–472. doi: 10.1016/j.jalz.2017.06.2309
Vinutha, N., Pattar, S., Shenoy, P. D., and Venugopal, K. R. (2021). SliceNet-AD: slice selection-based convolution neural network model for classification of Alzheimer's disease. Int. J. Image Mining 4, 69–97. doi: 10.1504/IJIM.2021.115771
Wang, D., Honnorat, N., Fox, P. T., Ritter, K., Eickhoff, S. B., Seshadri, S., et al. (2023). Deep neural network heatmaps capture Alzheimer's disease patterns reported in a large meta-analysis of neuroimaging studies. Neuroimage 269:119929. doi: 10.1016/j.neuroimage.2023.119929
Wang, F., Casalino, L. P., and Khullar, D. (2019). Deep learning in medicine—promise, progress, and challenges. JAMA Intern. Med. 179, 293–294. doi: 10.1001/jamainternmed.2018.7117
Wang, H., Shen, Y., Wang, S., Xiao, T., Deng, L., Wang, X., et al. (2019). Ensemble of 3D densely connected convolutional network for diagnosis of mild cognitive impairment and Alzheimer's disease. Neurocomputing 333, 145–156. doi: 10.1016/j.neucom.2018.12.018
Wang, J. J., Chen, S. W., Shao, J. Q., Gu, X. W., and Zhu, H. S. (2021). “Medical 3D reconstruction based on deep learning for healthcare,” in Proceedings of the 14th IEEE/ACM International Conference on Utility and Cloud Computing Companion (New York, NY: ACM), 1–5. doi: 10.1145/3492323.3495618
Wang, S. H., Phillips, P., Sui, Y., Liu, B., Yang, M., Cheng, H., et al. (2018a). Classification of Alzheimer's disease based on an eight-layer convolutional neural network with leaky rectified linear units and max pooling. J. Med. Syst. 42, 1–11. doi: 10.1007/s10916-018-0932-7
Wang, S. H., Xie, S., Chen, X., Guttery, D. S., Tang, C., Sun, J., et al. (2019). Alcoholism identification based on an AlexNet transfer learning model. Front. Psychiatry 10:454348. doi: 10.3389/fpsyt.2019.00205
Wang, S. H., Zhang, Y., Li, Y. J., Jia, W. J., Liu, F. Y., Yang, M. M., et al. (2018b). Single slice based detection for Alzheimer's disease via wavelet entropy and multilayer perceptron trained by biogeography-based optimization. Multimed. Tools Appl. 77, 10393–10417. doi: 10.1007/s11042-016-4222-4
Wang, S. H., Zhou, Q., Yang, M., and Zhang, Y. D. (2021). ADVIAN: Alzheimer's disease VGG-inspired attention network based on convolutional block attention module and multiple-way data augmentation. Front. Aging Neurosci. 13:687456. doi: 10.3389/fnagi.2021.687456
Weavers, P. T., Tao, S., Trzasko, J. D., Shu, Y., Tryggestad, E. J., Gunter, J. L., et al. (2017). Image-based gradient non-linearity characterization to determine higher-order spherical harmonic coefficients for improved spatial position accuracy in magnetic resonance imaging. Magn. Reson. Imaging 38, 54–62. doi: 10.1016/j.mri.2016.12.020
Wei, J., Chu, X., Sun, X. Y., Xu, K., Deng, H. X., Chen, J., et al. (2019). Machine learning in materials science. InfoMat 1, 338–358. doi: 10.1002/inf2.12028
Wu, M., and Chen, L. (2015). “Image recognition based on deep learning,” in 2015 Chinese Automation Congress (CAC) (Wuhan: IEEE), 542–546. doi: 10.1109/CAC.2015.7382560
Wu, O., Winzeck, S., Giese, A. K., Hancock, B. L., Etherton, M. R., Bouts, M. J., et al. (2019). Big data approaches to phenotyping acute ischemic stroke using automated lesion segmentation of multi-center magnetic resonance imaging data. Stroke 50, 1734–1741. doi: 10.1161/STROKEAHA.119.025373
Yanase, J., and Triantaphyllou, E. (2019). A systematic survey of computer-aided diagnosis in medicine: past and present developments. Expert Syst. Appl. 138:112821. doi: 10.1016/j.eswa.2019.112821
Yang, K., and Mohammed, E. A. (2020). A review of artificial intelligence technologies for early prediction of Alzheimer's disease. arXiv [Preprint]. arXiv:2101.01781. doi: 10.48550/arXiv.2101.01781
Young, T., Hazarika, D., Poria, S., and Cambria, E. (2018). Recent trends in deep learning based natural language processing. IEEE Comput. Intell. Mag. 13, 55–75. doi: 10.1109/MCI.2018.2840738
Zhang, B., Wang, J., Lin, L., and Wu, S. (2019). “The effect of smoothing filters on CNN based AD classification,” in Proceedings of the 2019 8th International Conference on Bioinformatics and Biomedical Science (New York, NY: ACM), 54–58. doi: 10.1145/3369166.3369191
Zhang, F., Tian, S., Chen, S., Ma, Y., Li, X., Guo, X., et al. (2019). Voxel-based morphometry: improving the diagnosis of Alzheimer's disease based on an extreme learning machine method from the ADNI cohort. Neuroscience 414, 273–279. doi: 10.1016/j.neuroscience.2019.05.014
Zhang, Y., Teng, Q., Liu, Y., Liu, Y., and He, X. (2022). Diagnosis of Alzheimer's disease based on regional attention with sMRI gray matter slices. J. Neurosci. Methods 365:109376. doi: 10.1016/j.jneumeth.2021.109376
Zhang, Y. D., Govindaraj, V. V., Tang, C., Zhu, W., and Sun, J. (2019). High-performance multiple sclerosis classification by data augmentation and AlexNet transfer learning model. J. Med. Imaging Health Inf. 9, 2012–2021. doi: 10.1166/jmihi.2019.2692
Zheng, X., Shi, J., Zhang, Q., Ying, S., and Li, Y. (2017). “Improving MRI-based diagnosis of Alzheimer's disease via an ensemble privileged information learning algorithm,” in 2017 IEEE 14th International Symposium on Biomedical Imaging (ISBI 2017) (Melbourne, VIC: IEEE), 456–459. doi: 10.1109/ISBI.2017.7950559
Zong, Y., Zuo, Q., Ng, M. K. P., Lei, B., and Wang, S. (2024). A new brain network construction paradigm for brain disorders via diffusion-based graph contrastive learning. IEEE Trans. Pattern Anal. Mach. Intell. 46, 10389–10403. doi: 10.1109/TPAMI.2024.3442811
Zuo, Q., Wu, H., Chen, C. P., Lei, B., and Wang, S. (2024). Prior-guided adversarial learning with hypergraph for predicting abnormal connections in Alzheimer's disease. IEEE Trans. Cybern. 54, 3652–3665. doi: 10.1109/TCYB.2023.3344641
Keywords: Alzheimer's disease, challenges, MRI, deep learning, pre-processing, feature extraction and classification
Citation: Nagarajan I and Lakshmi Priya GG (2025) A comprehensive review on early detection of Alzheimer's disease using various deep learning techniques. Front. Comput. Sci. 6:1404494. doi: 10.3389/fcomp.2024.1404494
Received: 21 March 2024; Accepted: 20 December 2024;
Published: 10 January 2025.
Edited by:
Marcello Pelillo, Ca' Foscari University of Venice, ItalyReviewed by:
Shuqiang Wang, Chinese Academy of Sciences (CAS), ChinaHao Xu, Zhejiang Normal University, China
Copyright © 2025 Nagarajan and Lakshmi Priya. This is an open-access article distributed under the terms of the Creative Commons Attribution License (CC BY). The use, distribution or reproduction in other forums is permitted, provided the original author(s) and the copyright owner(s) are credited and that the original publication in this journal is cited, in accordance with accepted academic practice. No use, distribution or reproduction is permitted which does not comply with these terms.
*Correspondence: G. G. Lakshmi Priya, bGFrc2htaXByaXlhLmdnQHZpdC5hYy5pbg==
†ORCID: I. Nagarajan orcid.org/0000-0001-8191-8144