- 1Computing + Mathematical Sciences, California Institute of Technology, Pasadena, CA, United States
- 2Division of Humanities and Social Science, California Institute of Technology, Pasadena, CA, United States
- 3Activision-Blizzard-King, Santa Monica, CA, United States
- 4Center for Science, Society, and Public Policy, California Institute of Technology, Pasadena, CA, United States
Online competitive action games are a very popular form of entertainment. While most are respectfully enjoyed by millions of players, a small group of players engages in disruptive behavior, such as cheating and hate speech. Identifying and subsequently moderating these toxic players is a challenging task. Previous research has only studied specific aspects of this problem using curated data and with limited access to real-world moderation practices. In contrast, our work offers a unique and holistic view of the universal challenges of moderating disruptive behavior in online systems. We combine an analysis of a large dataset from a popular online competitive first-person action title (Call of Duty®: Modern Warfare®II) with insights from stakeholders involved in moderation. We identify six universal challenges related to handling disruptive behaviors in such games. We discuss challenges omitted by prior work, such as handling high-volume imbalanced data or ensuring the comfort of human moderators. We also offer a discussion of possible technical, design, and policy approaches to mitigating these challenges.
1 Introduction
In 2020, U.S. consumers spent approximately $57 billion on video games1. One of the popular types of video games are online competitive action games, and their proliferation has led to a significant increase in the number of people present online, often immersing themselves in virtual worlds for extended periods of time (Ng and Wiemer-Hastings, 2005). For many, this has proven to be a positive experience, as online games have been shown to improve wellbeing (Kriz, 2020), provide entertainment (Bourgonjon et al., 2016), and foster social interactions (Kriz, 2020). Unfortunately, a small number of players leveraged such platforms to engage in disruptive behavior, including cheating, trolling, and offensive speech (Cook et al., 2019). This disruptive behavior may be due to a misunderstanding of the proper code of conduct (ABK, 2023a), players' mismatched expectations, or the game's generally competitive nature (Kou, 2020). Given the large size of the player base, which for high selling titles could number in the millions, a low incidence of disruptive behavior can translate into thousands of players misbehaving on a daily basis, which can negatively affect the experience of a much larger number of players (Steinkuehler, 2023). In turn, this might lead to a normalization of toxic behaviors across platforms (Beres et al., 2021). Identifying toxic players and deciding on the right mitigation actions is a challenging problem (Kou, 2020; Wijkstra et al., 2023). This is due to the sheer volume of data on players and gameplay (ABK, 2022), difficulty in recognizing what is disruptive in different social contexts (Wijkstra et al., 2023), psychological phenomena leading to under-reporting [e.g., the bystander effect (Barlińska et al., 2013)] or over-reporting [e.g., in-group favoritism and out-group hostility (Turner, 1975)], and because disruptive players may try to avoid having their misbehavior observed and detected (Srikanth et al., 2021). Well-trained human moderators can provide high-quality labels and interpret ambiguous and challenging cases in light of current policies. Nevertheless, human moderators can also face challenges when exposed to large amounts of toxic content (Levkovitz, 2023).
Previous research has studied disruptive behavior in online platforms using well-labeled datasets (Canossa et al., 2021), with a focus on applying classification approaches in isolation (Märtens et al., 2015), testing theory-informed hypotheses about social interactions (Kwak et al., 2015), understanding how players become toxic (Cook et al., 2019), investigating cultural bias in player behavior (Sengün et al., 2019), or running small-scale proof-of-concept studies (Stoop et al., 2019). While informative, these offer only a narrow view of a vast socio-technical challenge. No holistic portrait of the challenges of detecting and moderating disruptive behavior in online competitive action games exists (Wijkstra et al., 2023).
In this paper, we comprehensively examine the challenges of moderating disruptive behavior in online competitive action games as presented in Figure 1. Our work is informed by a review of the existing literature, statistical analysis of real-world data (Call of Duty: Modern Warfare II), and insights from stakeholders directly involved in moderation. We offer the first holistic overview of pertinent challenges and provide analyses of evidence and impact. We also identify previously unreported challenges, such as the need for protecting human moderators' comfort and the impact of delay in moderation on player engagement and the propensity of repeating the offense. Our work identifies the important scientific challenges in this area, and sets the agenda for comprehensive analysis and mitigation opportunities in the gaming domain affecting millions of people daily.
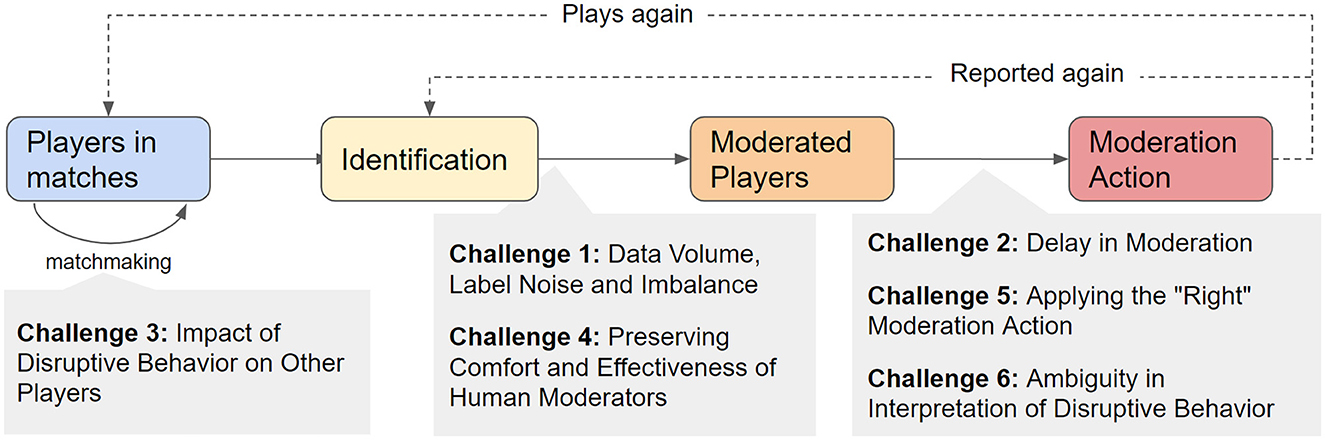
Figure 1. Overview of the general moderation process in Modern Warfare II and similar titles. This process is similar to other gaming platforms. We highlight a set of 6 pertinent challenges described in this paper.
2 Gameplay, reporting and moderation practices
2.1 Call of Duty: Modern Warfare II
A 2022 first-person action videogame developed by Infinity Ward and published by Activision (McWhertor, 2022). The game takes place in a realistic and modern combat setting on multiple continents (ABK, 2023c). The game offers single-player, story-driven campaign mode and several online multiplayer modes: Gunfight, Ground War, Team Deathmatch, and Domination, for example. Individual online matches can involve between 4 and 64 players at a time, depending on the game mode, and team sizes varying between 2 and 32 players. The primary goal of each match also varies between modes, but generally, the last team standing or the first team to capture/complete an objective will win the match.
2.2 Player interactions and reporting
Players can interact with one another in the game environment by exchanging items, coordinating movement, and using in-game gestures (e.g., pings, game-derived voice narration). Players can also communicate using text-based and audio-based voice chats. These player-player interactions can occur both within and across teams. Players are able to report other players for behavior they consider disruptive. Such reporting is available during or after a match. The reporting player can indicate one of 5 categories of disruptive behaviors (e.g., offensive username, or cheating) and include additional context such as offensive text chat quotes.
3 Methodology and data
3.1 Semi-structured interactions with domain experts
Over the course of 6 months, we engaged in regular meetings with different stakeholders involved in logging, analysis, and moderation of player behavior. These bi-weekly meetings involved one-hour interactions in which we (1) developed our understanding of the players' in-game experiences, the architecture of the current moderation workflow, and instrumentation of the data on the platform, (2) discussed and verified discoveries around player and moderation behavior as logged in the dataset, and (3) coordinated on the selection of pertinent challenges in handling disruptive behavior. These meetings resulted in the characterization of moderation workflow presented in Figure 1 and informed the selection of the most important long-term challenges.
3.2 Logs of player and moderation behavior
During game play in COD:MWII, many aspects of the game and associated events are summarized and stored in SQL databases. These data are distributed over several SQL tables containing match statistics for players, player reports about potentially disruptive behavior of other players, as well as moderation events. Table 1 summarizes our anonymized dataset of players and matches for one week from Feb 06, 2023 to Feb 12, 2023. We present a breakdown of the reports about disruptive behavior. We report a ratio of reports per player “Reports/Player” and a ratio of reports per match “Reports/Match”. We note that these are many-to-many relations where the same player and the same match can appear multiple times. We also report the % of unique players that were reported for disruptive behavior by other players during the period of our study (“Reported Players”). Finally, we present the % of reports that the moderation team was able to review in “Moderated Reports” as well as the % of reports that resulted in a moderation action, indicating a possibly genuine report and a need for intervention (“% Act”). The precise data extraction and processing details for constructing this table are presented in Appendix 1.1.
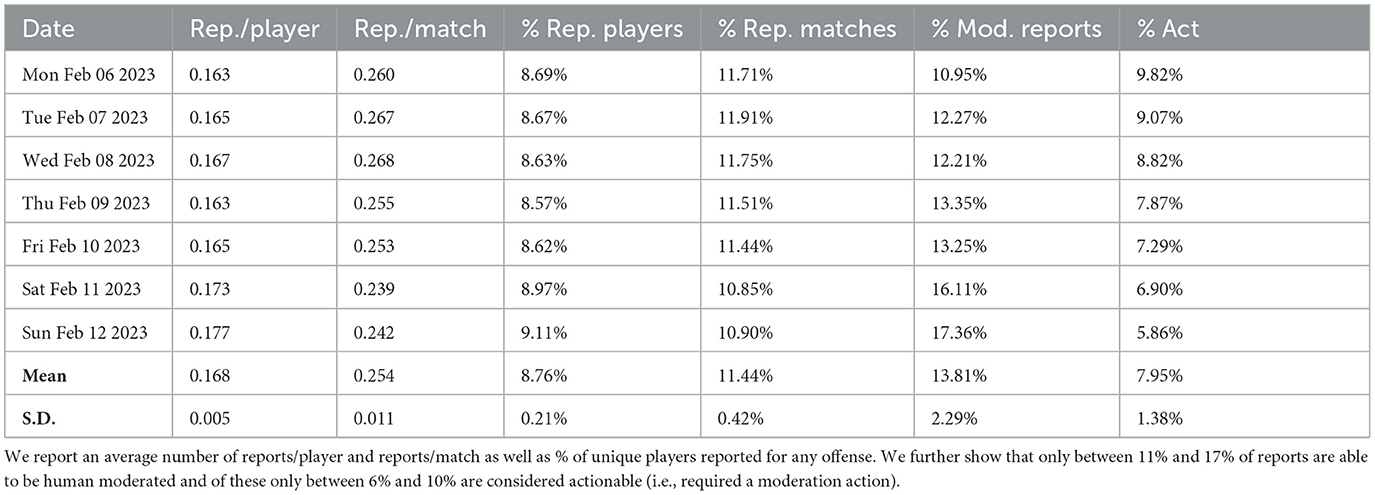
Table 1. Statistics of the dataset obtained for the Call of Duty: Modern Warfare II between Feb 06, 2023 and Feb 12, 2023.
3.3 Analysis methods
Data is stored in an SQL database and distributed over several tables containing timestamps, anonymized player ids, matches, reports, and moderation entries. For obtaining aggregate information we connected several tables over a given time period between Feb 06, 2023 and Feb 12, 2023. As the moderation of player reports may take additional time over the inspected period, we collected moderation entries within 30 days from our start date. As real-world data can contain missing or inaccurate data, we employed additional pre-processing checks in our queries, specifically: (1) we ensured that both reporter and reported players were in the same match or in the same lobby on a given day, (2) we discarded entries for which join keys were empty. We used Python packages PySpark, Pandas for data querying as well as the R package ggplot for plotting. Statistical analysis was performed using the Python package statsmodels. For each of the challenges reported in the subsequent sections of the paper, we performed specific data extraction and analysis. We report the details of this analysis in Appendix 1. We further refer to the specific subsection of this Appendix when providing the evidence for each challenge in the subsequent sections of the paper.
4 Identified challenges
To identify the crucial challenges in the moderation of disruptive behavior, we leveraged triangulation, which is a mixed-methods technique of using multiple data sources and analysis methods in order to increase the confidence in our findings (Fielding, 2012). We use a combination of 3 sources: (1) moderation workflow, (2) exploratory analysis of our dataset, and (3) stakeholder conversations. The combination of these three sources allowed us to ground the challenges in the practical needs of both the players and the moderation team, as well as supporting our results with concrete measurable evidence present in the data logs.
4.1 Challenge 1: data volume, label noise and imbalance
A general challenge in moderation is the large volume of data (Ghani et al., 2019). We discuss the severity of this challenge in our dataset and the practical impact it has on player experience and moderation efforts.
Evidence: Nearly 400 million players worldwide engaged with ABK games in 2022 (ABK, 2022). There are an average of 100k+ players in COD:MWII alone at any given moment according to Steam Charts (Charts, 2023). Furthermore, the number of unique active players in a 30-day period frequently reaches between 7M and 10M with average daily active players estimated at between 500k and 900k according to an estimate from online sources as the official player counts are not released (ActivePlayer, 2023). We estimate the breakdown of reported players and matches as well as the moderation percentages and actionable reports (Table 1) using the procedure described in Appendix 1.1. Although only 9% of players are reported for various forms of disruptive behaviors, this still translates to large absolute numbers. Due to such a high volume, only around 14% of reports are reviewed by human moderation. Furthermore, only about 8% of moderated reports are deemed to necessitate a moderation action, suggesting a high ratio of noisy reporting.
Impact: The large volume of data makes it challenging to effectively investigate all reports using human-based moderation alone (Levkovitz, 2023). Unfortunately, automation via domain-knowledge-based heuristics which are relied on in many production systems is hard to adapt to new player behavior and complex in-game contexts (Srikanth et al., 2021). Furthermore, unlike toxicity or misinformation in social media platforms, which is often concentrated around few individuals (Stewart et al., 2018), disruptive behavior in games has been shown to be much less concentrated around particular individuals (Stoop et al., 2019). Quality and label scarcity also impact machine-learning-based solutions on a fundamental level. The large difference in the volume of “disruptive” vs. “regular” players introduces the fundamental problem of extreme class imbalance (Abd Elrahman and Abraham, 2013). This makes it very hard to effectively identify all the disruptive behaviors (recall) with a high level of accuracy (precision). While several technical approaches exist (Tyagi and Mittal, 2020), fundamentally optimizing for either metric results in a high volume of false reports or risks missing a large volume of genuinely disruptive behaviors. Different perceptions of toxicity (Lin and Sun, 2005), unfamiliarity with the code of conduct (ABK, 2023b), as well as social phenomenons such in-group favoritism and out-group hostility (Turner, 1975), the bystander-effect (Barlińska et al., 2013), and attribution theory (Kwak et al., 2015) suggest both over-reporting and under-reporting in certain settings, which introduces a fundamental challenge of label noise. While such noise can be identified to some extent (Sharma et al., 2020) it also introduces additional verification effort.
4.2 Challenge 2: delay in moderation actions
Disruptive player behavior can be moderated in various ways, however, such moderation, especially in high-volume production systems may come at a delay. Such delay introduces a period of time during which a player's disruptive behavior was not met with any reaction.
Evidence: In Figure 2 we report the delay in moderation by different disruptive player behavior categories. This figure has four panels, in the first we show the distribution of the delay in the moderation of a reported cheating behavior. Subsequent panels report delays for reports of offensive text chat, offensive user identification and other offenses respectively. The x-axis represents the number of days that have passed since the report submission. The y-axis on the left reports the percentage of reports reviewed by moderation on a given day. The black line depicts the cumulative distribution function (CDF) reporting the percentage of all the reports that have been reviewed. The corresponding y-axis scale for the CDF is presented on the right side of the graphs. This only includes the reports that have eventually been reviewed by the moderation team and hence adds up to 100%. This delay is measured as the number of days from when the first report about a player was submitted until the time the moderation examined the reported case. We report the precise data extraction and analysis involved in producing this figure in Appendix 1.2. The reason for existence of a delay can be due to the need for manual inspection, or even in an automated system due to the need for accumulation of sufficient evidence to justify taking an action (e.g., threshold of reports).
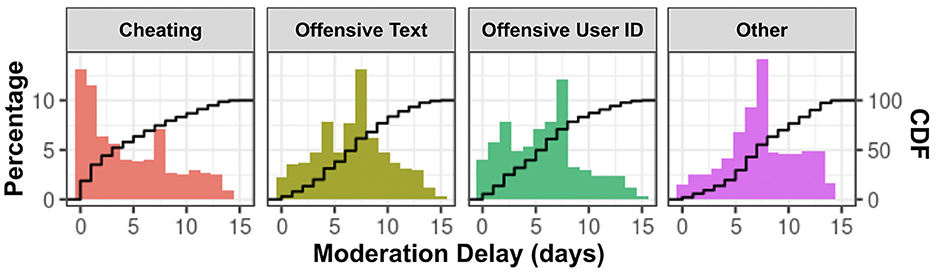
Figure 2. Delay in human moderation of the reported players by type of disruptive behavior. We can see that the offensive text chat incurs a significant delay in the case handling.
Impact: Such delay in moderation can have many effects. Experiments in education settings suggest that delayed punishment can reduce its effectiveness (Abramowitz and O'Leary, 1990). Similarly, in the social media context, delayed moderation action (in the form of content removal) to posting inappropriate content has been found to be less effective (Srinivasan et al., 2019). Furthermore, temporal delay has been shown to affect the motivational structures of human decision-making (Suh and Hsieh, 2016). Specifically, temporal delay tends to be associated with increased uncertainty of whether the behavior will result in any consequences (Luhmann et al., 2008). If the reaction to disruptive behavior comes “too late”, players may be less likely to comply. Finally, from a learning theory perspective, timing of feedback is considered a key component affecting feedback effectiveness (Thurlings et al., 2013).
4.3 Challenge 3: impact of disruptive behavior on other players
The social nature of gaming means players are connected together. This can be via automated matchmaking or, in some game modes, explicit selection of teammates. In some game modes, they also form ephemeral teams. This means that players exhibiting disruptive behavior may affect others.
Evidence: We quantify the number of players exposed to disruptive behavior. We identify the players who are reported and moderated on a specific day–March 26th, 2023. We then count the number of matches they engaged in and the number of players they interacted with during one week before that day. We treat players who they play with on the same team as potentially “exposed” players. The detailed data processing and analysis steps involved are reported in Appendix 1.3. In Figure 3 we show the distribution of exposed matches and exposed players (left and middle respectively). In the left graphs, for example, we can see that there were ~300 disruptive players that participated in ~100 matches in a week prior to being reported. In the middle graph, we can also see that there were ~200 disruptive players who played with more than 500 other players during the week before being reported. These exposure statistics show an opportunity for affecting other players but do not, by themselves, indicate that disruptive players meaningfully affect others. We hence perform further analysis focused on the change in the number of reports about players before and after exposure to a toxic player. In the right-hand chart, we show that exposure to a toxic player increases the average number of reports per exposed player from 0.42 to 0.65 (t-test p-value < 0.001), an increase of about 0.224 per player.
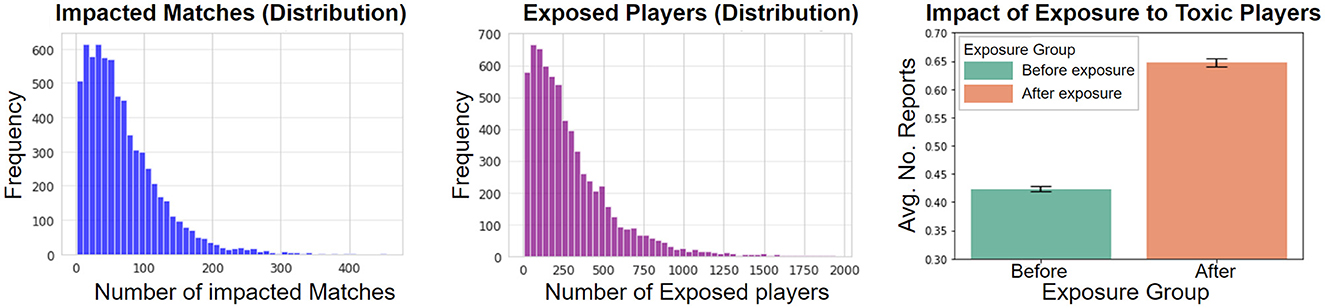
Figure 3. Estimation of the exposure to disruptive behavior from a subsequently reported player. Left: distribution of exposed matches, Middle: exposed players based on matches, Right: impact on the report count pre- and post-exposure to the disruptive player.
Impact: Such exposure to disruptive and toxic behavior can have many adverse effects. It can lead to the normalization of toxicity (Beres et al., 2021). Players unwilling to follow such “new normal” can be driven away from the game altogether. Recent work reports that roughly half (49.3%) of players report avoiding some game titles due to toxicity (Steinkuehler, 2023). This can have a real-world monetary impact as players' average monthly spending on games deemed “non-toxic” was reported to be 54% higher than on games deemed “toxic” (Steinkuehler, 2023). Potential mitigation strategies could involve engaging the player community in effective self-moderation akin to the tribunal system (Kwak et al., 2015), or lowering propensity for toxic behavior via “prosocial nudges” (Caraban et al., 2019), or limiting the perception of anonymity shown conducive to offensive behavior (Reicher et al., 1995).
4.4 Challenge 4: preserving comfort and effectiveness of human moderators
Prior work tends to focus on the impact exposure to toxic content has on the user base of various social platforms (Malmasi and Zampieri, 2017). Often omitted is the impact that exposure has on human moderators.
Evidence: Given the active daily player estimates of 500k+ (ActivePlayer, 2023) and that ~9% of players are reported for offensive behavior (see Table 1), we can see that the moderation team is exposed to high volume of reports about disruptive behavior in one week. We further aggregate different moderation reasons and moderation actions taken in Table 2. The details of how this table is generated are provided in Appendix 1.4, looking at the breakdown of the reasons for player reports in Table 2, we can see that a large proportion is related to various types of toxic content via text, or offensive user identification (offensive username or clan tags). Such high exposure to toxic content is not uncommon for moderators, as evidenced by testimonials from individuals across the moderation landscape (Verge, 2019).
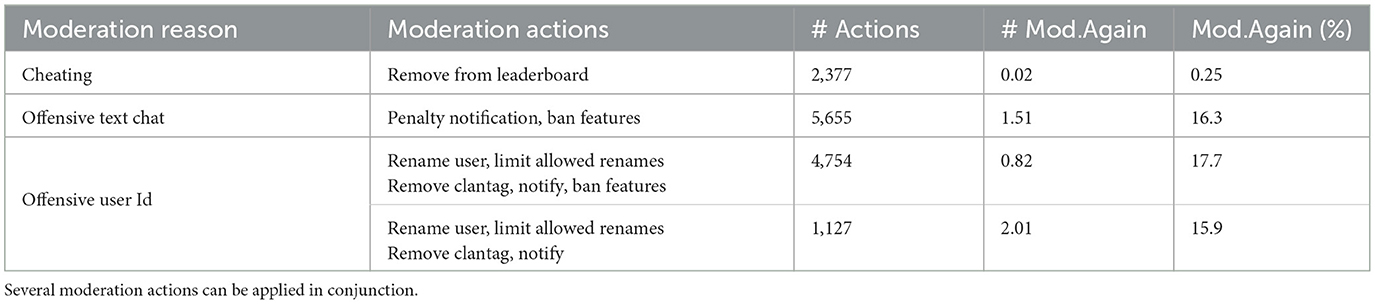
Table 2. Moderation reason, associated actions, and the mean as well as the % of players reported again for the same reason in the following month (an indicator of the effectiveness of action in preventing future offenses).
Impact: Repeated exposure to toxicity, hate-speech, offensive or disruptive content, or even racism (Sengün et al., 2019; Lakomy et al., 2023) can have many adverse effects on the work of human moderators. First of all, it can impact their comfort leading to the need for relaxation breaks and even recovery (Levkovitz, 2023). Furthermore, constant exposure to offensive content can produce desensitization and exhaustion leading to impaired judgment and higher error rates (Soral et al., 2018). Such desensitization can further lead to higher disagreement rates with other moderators and drive up the costs of any rigorous moderation workflow (Chen et al., 2018). Finally, high exposure to hate speech has been linked to lowering empathy (Pluta et al., 2023). Potential mitigation opportunities lie in variations of human-in-the-loop automation via active learning approaches (Link et al., 2016). In such an approach, only ambiguous examples require human inspection and the obviously toxic and non-toxic ones are handled fully automatically. Emotional management techniques such as mutual supervision, working in teams, and emotional reset methods can also be included in the moderation workflow (Lakomy et al., 2023).
4.5 Challenge 5: applying the “right” moderation action
Even if detected, effective moderation of disruptive behavior is challenging (Ma et al., 2023). Approaches relying on bans and retributive actions have been criticized in favor of positive socialization and restorative forms of justice (Steinkuehler, 2023).
Evidence: In Table 2 we present a breakdown of different types of moderation reasons and associated moderation actions. We take players who were reported between March 1 and 7, 2023 and receive a moderation action. We then look at the average times/percent the player is reported again for the same reason in the following month. Exact details of how this analysis is performed are provided in Appendix 1.4. Several actions can be applied in combination in response to a report. Also, different combinations of actions can be associated with the same reason. Moderators use their best judgment to apply the most appropriate actions in a given context. We can see that action “Remove From leaderboard” applied in response to cheating is quite effective with only 0.25% of players being reported and verified by moderation as cheaters again. The moderation actions related to toxic or offensive content such as “Rename User” or “Ban Features” seem less effective, with 16% to 18% of players being reported again and verified by moderation to be disruptive for the same offense in a subsequent month.
Impact: Recent work reports the lack of effective ways to combat toxicity as one of the major obstacles (Wijkstra et al., 2023). Increasingly the traditionally punitive moderation actions are being criticized (Steinkuehler, 2023). A reactance phenomenon known in persuasion literature suggests that imposing forceful rules and regulations can actually lead to players being more toxic to fight regulation or trigger more covert behaviors to avoid detection (Quick et al., 2013). Prior work calls for more transparency with the player base, overhauling existing in-game reporting practices, and reinforcing positive behaviors (Lapolla, 2020). Recent work also points to the importance of explanations for increasing perceptions of moderation fairness (Ma et al., 2023).
4.6 Challenge 6: ambiguity in interpretation of what is toxic/non-toxic
The subjectivity in the assessment of what is considered toxic is a known challenge in social media (Sheth et al., 2022). Recent work has also shown severe heterogeneity among gamers in the perception of what is appropriate, tolerable, or harmful (Beres et al., 2021).
Evidence: To find evidence for potential ambiguity in the interpretation of the same reports among human moderation, we look at cases where reports about toxic player behavior were considered non-actionable (i.e., moderation checked these and decided not to take action) at one time, but they were deemed actionable upon subsequent reporting. We provide the detailed processing steps in Appendix 1.5. Among all players whose usernames were reported, but ultimately determined non-toxic between March 1 and 7, 2023, 2.5% were ultimately moderated because of a toxic username in the same month. Similarly, among all of the players whose text chat posts were determined to be not actionable, 14.7% are eventually moderated because of toxic text chat in the same month. These alterations may indicate disagreement between subsequent moderators. We acknowledge that some of these changes could have been due to simple omission or the player engaging in more toxic actions over time.
Impact: A consistent definition of toxicity is challenging in itself. Märtens et al. (2015) define toxicity as the use of profane language by one player to insult or humiliate a different player in his own team. This definition is naturally limited as it focuses on toxicity expressed in language and is also limited to particular recipients. The ambiguity in the perception of toxicity can be linked to fundamental differences in players' experiences and expectations. The matching of players, the familiarity between them, and the context of the game have all been linked to heterogeneity of toxicity judgments (Wijkstra et al., 2023). Additionally, the toxicity can be targeted at the aspects of the game itself, or even at aspects outside of the game. The impact is usually the most harmful when it becomes personal and relates to the game skills of other players (Sengün et al., 2019). Additionally, sequences of fairly usual in-game events can lead to the emergence of toxicity (Kwak et al., 2015).
5 Discussion
For each challenge, we briefly discussed possible mitigation strategies. As game platforms are socio-technical systems, we see the solutions as involving three prongs: (1) technical innovations, (2) player experience designs, and (3) policies.
5.1 Technology
We identify several important areas related to technical challenges and innovation.
Contextual modeling: The nuances in the interpretation of toxicity (Challenge 6) call for modeling approaches incorporating longer interaction context (Lei et al., 2022) as well as multi-modal input (e.g., game-play, text, audio) (Velioglu and Rose, 2020). Furthermore, disambiguation of what is considered toxic or acceptable may require more complex modeling of social relations between players in different gameplay contexts. Approaches such as social network analysis (Schlauch and Zweig, 2015), in combination with contextual modeling via graph neural networks, might prove especially beneficial in these efforts (Fan et al., 2019; Hamid et al., 2020).
Resource-efficiency: Use of contextual deep-learning models can be slow and resource intensive for large-scale production systems (Borgeaud et al., 2022), this calls for techniques improving training (Rasley et al., 2020) and inference efficiency [e.g., in-context learning (Min et al., 2022) or prompting (Cao et al., 2023)]. Given the unique context of language use, involving game-specific terms, techniques for light-weight fine-tuning of existing general-purpose large language models such as Low-Rank Adaptation (Zhao et al., 2023) and adapters (Pfeiffer et al., 2020) can prove especially beneficial.
Human-in-the-loop: Evolution in the expression of disruptive behavior, policy changes, as well as the need for considering the impact on human moderators (Challenge 4) calls for techniques efficiently incorporating human labeling such as active learning (Link et al., 2016), semi-supervised learning (Zhu, 2005), as well as efficient quality verification techniques via labeling noise identification (Sharma et al., 2020). Impact of exposure to toxic or disruptive content can further be mitigated by techniques such as mutual supervision and emotional reset methods, along with coping strategies like humor and breaks (Lakomy et al., 2023). Furthermore, techniques around technology-facilitated self-moderating crowds can offer an effective approach to supplement dedicated moderation teams (Seering, 2020). These can be combined with automated techniques for better exploration of vast player-behavior space using techniques such as cluster-based active learning for concept drifts in online data streams (Halder et al., 2023).
Sampling techniques: The large volume of data, imbalance in the labels, as well as distributional shifts present in social media (Challenge 1) call for cost-sensitive training (Ling and Sheng, 2008), adaptive representations (Srikanth et al., 2021), and anomaly detection techniques (Zhou and Paffenroth, 2017). Supporting effective automated and data-driven interventions requires modeling techniques capturing complex causal interactions, which motivates the application of Causal Machine Learning (Causal ML) techniques in this context (Zhao and Liu, 2023).
5.2 Player experience design
Mitigation through design similarly involves several areas.
Rethinking moderation actions: Recent work increasingly suggests avoiding punitive actions and considering prosocial interventions informed by behavioral economics (Angner and Loewenstein, 2007). For example, addressing Challenge 5 could involve interventions promoting prosocial behavior such as providing a social comparison to “most prosocial player” of the match or day (Colusso et al., 2016). The behavior change and learning research literature suggest higher long-term effectiveness of reflection-driven nudges such as “reminding of consequences” and “providing multiple viewpoints” (Caraban et al., 2019).
Intervention transparency: Recent work also suggests the importance of explanations in increasing players' perceptions of fairness of moderation in games (Ma et al., 2023). This suggests adapting solutions from AI transparency (Kocielnik et al., 2019) and explanation strategies in recommendation systems (Kang et al., 2022). Such transparency efforts, however, need to be carefully designed to prevent potential abuse by malicious players (Blauth et al., 2022).
Preventive design: Prior work identified contexts conducive to the emergence of disruptive behaviors (Challenge 3) (Kou, 2020). The nudging literature (Caraban et al., 2019) provides several approaches for affecting such context including promotion of “reciprocity” (Cialdini, 2007) and “raising the visibility of user actions” (Hansen and Jespersen, 2013). Passive techniques such as “subliminal priming” via desired imagery for promoting pro-social behaviors can also create a more prosocial environment (Strahan et al., 2002).
5.3 Policy
An important aspect of handling disruptive behavior involves internal and external policies.
Transparency around player data and moderation: Policies such as GDPR (Voigt and Von dem Bussche, 2017) regulate player data storage practices and algorithmic transparency. Similar policies in relation to transparency in content moderation of hate speech, disinformation, and extremism are only just beginning to emerge (Zakrzewski, 2022; League, 2023). These efforts are just in their infancy and collaboration with gaming companies is needed. Of course, caution is necessary regarding the details of how disruptive behavior is detected to prevent players from trying to avoid detection.
Clarity of guidelines: Despite regulations around reporting, very little is universally agreed on in relation to the definition of disruptive behavior and its proper moderation. Various gaming companies proposed their own sets of corporate rules formulated as players' codes of conduct (ABK, 2023b; Ubisoft, 2023). These offer initial attempts at addressing the mitigation challenge, but further steps are clearly needed (Busch et al., 2015).
Industry standards: The development of codes of conduct for gaming more generally, better player education at the industry level about appropriate conduct, and clearer industry-wide information about how players who violate codes of conduct will be dealt with may help alleviate some of the problems across all of the challenges we have discussed in this paper.
6 Future work
Based on the identified challenges, we see several important areas of future work along the prongs identified in the discussion.
6.1 Enhanced technological development and optimization
Multimodal interaction analysis: Building on our theme of contextual modeling, future work should explore creating more robust models that combine various modalities such as gameplay, text, and audio to better interpret toxicity and disruptive behavior as it is expressed across the gaming platform. The integration of different data sources could enable more nuanced understanding and detection.
Optimizing resource efficiency: Future research should focus on creating more efficient models for large-scale production systems. This may involve developing new algorithms and methodologies to reduce the computational requirements of deep learning models, both in terms of training and inference.
Advanced sampling techniques: Exploring novel sampling techniques to handle large volumes of data, label imbalances, and distributional shifts should be a significant future direction. This includes investigating adaptive representations, cost-sensitive training, and causal machine learning approaches.
6.2 Player experience design and prosocial interventions
Promotion of prosocial behavior: Design interventions that encourage prosocial behavior, rather than simply punish toxicity. Such approaches could include utilizing behavioral economics concepts or nudging literature to create incentives and motivators for positive player interactions. Furthermore, game design and mechanics should be designed with the promotion of prosocial behavior in mind.
Intervention transparency: Future work should look into incorporating explanations and transparency into the moderation process, building upon AI transparency principles and explanation strategies to improve fairness perceptions.
Preventing toxicity and promoting prosocial behaviors: Research into how passive techniques like subliminal priming or preventive design could foster a more prosocial environment should be explored. This may involve the development and testing of new design patterns that help prevent the emergence of disruptive behaviors as well as limit the conditions leading to the emergence of toxicity.
6.3 Policy formulation and standardization across the industry
Transparency and regulation compliance: Collaborative efforts between gaming companies, legislators, and regulators to craft transparent policies around player data and moderation are an essential step forward. Ensuring compliance with regulations like GDPR and exploring emerging guidelines related to content moderation would be a vital aspect of this.
Creation of universal guidelines: Building on the need for clarity in defining disruptive behavior, future work should focus on creating universal guidelines and codes of conduct that transcend individual companies' policies. This would help in defining common standards for disruptive behavior and its proper moderation.
Industry standards and education: The development of broader industry standards, coupled with education initiatives for players, can form a concerted effort to improve overall conduct within the gaming community. This should include collaborative efforts among different stakeholders in the gaming industry to create consistent standards and educational materials.
These directions highlight the multidisciplinary nature of challenges within game platforms, bridging technological innovation, design considerations, and policy interventions. The collaborative effort across these domains could contribute to a more effective and player-friendly gaming ecosystem.
7 Conclusion
In this work, we posited six challenges related to the moderation of disruptive behavior in online competitive action games. These challenges are informed by our interactions with various stakeholders, indications from prior work, and evidence from analysis of a real-world dataset from one of the popular first-person action gaming titles—Call of Duty: Modern Warfare II. We discuss the inherent difficulties in addressing these challenges, their impact, and potential mitigation approaches. Our work offers the first comprehensive organization of this space and sets the agenda for much-needed progress around moderation.
Data availability statement
The data analyzed in this study is subject to the following licenses/restrictions: the dataset is from proprietary commercial company–Activision Blizzard King. Requests to access these datasets should be directed to cm1hQGhzcy5jYWx0ZWNoLmVkdQ==.
Ethics statement
The studies involving humans were approved by Caltech Institutional Review Board. The studies were conducted in accordance with the local legislation and institutional requirements. Written informed consent for participation was not required from the participants or the participants' legal guardians/next of kin in accordance with the national legislation and institutional requirements.
Author contributions
RK: Conceptualization, Methodology, Project administration, Supervision, Writing—original draft, Writing—review & editing, Validation. ZL: Data curation, Formal analysis, Methodology, Visualization, Writing—original draft. CK: Conceptualization, Data curation, Writing—review & editing. DS: Writing—original draft, Writing—review & editing. JM: Writing—original draft, Writing—review & editing. ML: Writing—review & editing. CT: Writing—review & editing. MK: Software, Writing—review & editing. NN: Software, Writing—review & editing. FS: Writing—review & editing. AD: Writing—review & editing. GC: Project administration, Resources, Writing—review & editing. AA: Funding acquisition, Project administration, Writing—review & editing. RA: Conceptualization, Funding acquisition, Project administration, Resources, Supervision, Writing—original draft, Writing—review & editing.
Funding
The author(s) declare that no financial support was received for the research, authorship, and/or publication of this article.
Acknowledgments
We thank Sabrina Hameister and Gary Quan for their assistance with this project.
Conflict of interest
CT, MK, NN, FS, AD, and GC were employed by Activision-Blizzard-King. The research was sponsored by Activision Blizzard King under a sponsored research grant.
The remaining authors declare that the research was conducted in the absence of any commercial or financial relationships that could be construed as a potential conflict of interest.
Publisher's note
All claims expressed in this article are solely those of the authors and do not necessarily represent those of their affiliated organizations, or those of the publisher, the editors and the reviewers. Any product that may be evaluated in this article, or claim that may be made by its manufacturer, is not guaranteed or endorsed by the publisher.
Supplementary material
The Supplementary Material for this article can be found online at: https://www.frontiersin.org/articles/10.3389/fcomp.2024.1283735/full#supplementary-material
Footnotes
1. ^Data from the Entertainment Software Association,
(https://www.theesa.com/news/u-s-consumer-video-game-spending-totaled-56-6-billion-in-2022/).
References
Abd Elrahman, S. M., and Abraham, A. (2013). A review of class imbalance problem. J. Netw. Innov. Comput. 1, 332–340.
ABK (2022). 2022_esg_report.pdf. Available online at: https://ourcommitments.activisionblizzard.com/content/dam/atvi/activisionblizzard/ab-touchui/our-commitments/docs/2022_ESG_Report.pdf (accessed July 20, 2023).
ABK (2023a). Activision Announces New Code of Conduct in an Effort to “Combat Toxicity” Across Various Titles in the ‘Call of Duty' Series. Available online at: https://www.callofduty.com/blog/2024/01/call-of-dutyricochet-modern-warfare-iii-warzone-anti-cheatprogress-report (accessed April 24, 2023).
ABK (2023b). Call of Duty Code of Conduct|FPS Game Terms. Available online at: https://www.callofduty.com/values (accessed April 19, 2023).
ABK (2023c). Call of Duty: Modern Warfare 2|Call of Duty Wiki|Fandom. Available online at: https://callofduty.fandom.com/wiki/Call_of_Duty:_Modern_Warfare_2 (accessed April 28, 2023).
Abramowitz, A. J., and O'Leary, S. G. (1990). Effectiveness of delayed punishment in an applied setting. Behav. Ther. 21, 231–239. doi: 10.1016/S0005-7894(05)80279-5
ActivePlayer (2023). Call of Duty: Modern Warfare 2 Live Player Count and Statistics. Available online at: https://activeplayer.io/call-of-duty-modern-warfare/ (accessed June 16, 2023).
Angner, E., and Loewenstein, G. (2007). “Behavioral economics,” in Handbook of the Philosophy of Science: Philosophy of Economic 641–690. doi: 10.1016/B978-0-444-51676-3.50022-1
Barlińska, J., Szuster, A., and Winiewski, M. (2013). Cyberbullying among adolescent bystanders: Role of the communication medium, form of violence, and empathy. J. Commun. Appl. Soc. Psychol. 23, 37–51. doi: 10.1002/casp.2137
Beres, N. A., Frommel, J., Reid, E., Mandryk, R. L., and Klarkowski, M. (2021). “Don't you know that you're toxic: Normalization of toxicity in online gaming,” in Proceedings of the 2021 CHI Conference on Human Factors in Computing Systems 1–15. doi: 10.1145/3411764.3445157
Blauth, T. F., Gstrein, O. J., and Zwitter, A. (2022). Artificial intelligence crime: an overview of malicious use and abuse of ai. IEEE Access 10, 77110–77122. doi: 10.1109/ACCESS.2022.3191790
Borgeaud, S., Mensch, A., Hoffmann, J., Cai, T., Rutherford, E., Millican, K., et al. (2022). “Improving language models by retrieving from trillions of tokens,” in International Conference on Machine Learning (PMLR), 2206–2240.
Bourgonjon, J., Vandermeersche, G., De Wever, B., Soetaert, R., and Valcke, M. (2016). Players' perspectives on the positive impact of video games: A qualitative content analysis of online forum discussions. New Media Soc. 18, 1732–1749. doi: 10.1177/1461444815569723
Busch, T., Boudreau, K., and Consalvo, M. (2015). “Toxic gamer culture, corporate regulation, and standards of behavior among players of online games,” in Video Game Policy: Production, Distribution, and Consumption 176–190. doi: 10.4324/9781315748825-13
Canossa, A., Salimov, D., Azadvar, A., Harteveld, C., and Yannakakis, G. (2021). For honor, for toxicity: Detecting toxic behavior through gameplay. Proc. ACM Hum. Comput. Inter. 5, 1–29. doi: 10.1145/3474680
Cao, J., Li, M., Wen, M., and Cheung, S.-C. (2023). A study on prompt design, advantages and limitations of chatgpt for deep learning program repair. arXiv preprint arXiv:2304.08191.
Caraban, A., Karapanos, E., Gonçalves, D., and Campos, P. (2019). “23 ways to nudge: a review of technology-mediated nudging in human-computer interaction,” in Proceedings of the 2019 CHI Conference on Human Factors in Computing Systems 1–15. doi: 10.1145/3290605.3300733
Charts, S. (2023). Call of Duty: Modern Warfare II|Warzone 2.0 - Steam Charts. Available online at: https://steamcharts.com/app/1938090 (accessed April 18, 2023).
Chen, N.-C., Drouhard, M., Kocielnik, R., Suh, J., and Aragon, C. R. (2018). Using machine learning to support qualitative coding in social science: Shifting the focus to ambiguity. ACM Trans. Inter. Intell. Syst. 8, 1–20. doi: 10.1145/3185515
Colusso, L., Hsieh, G., and Munson, S. A. (2016). “Designing closeness to increase gamers' performance,” in Proceedings of the 2016 CHI Conference on Human Factors in Computing Systems 3020–3024. doi: 10.1145/2858036.2858206
Cook, C., Conijn, R., Schaafsma, J., and Antheunis, M. (2019). For whom the gamer trolls: a study of trolling interactions in the online gaming context. J. Comput. Med. Commun. 24, 293–318. doi: 10.1093/jcmc/zmz014
Fan, W., Ma, Y., Li, Q., He, Y., Zhao, E., Tang, J., et al. (2019). “Graph neural networks for social recommendation,” in The World Wide Web Conference 417–426. doi: 10.1145/3308558.3313488
Fielding, N. G. (2012). Triangulation and mixed methods designs: data integration with new research technologies. J. Mixed Methods Res. 6, 124–136. doi: 10.1177/1558689812437101
Ghani, N. A., Hamid, S., Hashem, I. A. T., and Ahmed, E. (2019). Social media big data analytics: a survey. Comput. Hum. Behav. 101, 417–428. doi: 10.1016/j.chb.2018.08.039
Halder, B., Hasan, K. A., Amagasa, T., and Ahmed, M. M. (2023). Autonomic active learning strategy using cluster-based ensemble classifier for concept drifts in imbalanced data stream. Expert Syst. Applic. 231:120578. doi: 10.1016/j.eswa.2023.120578
Hamid, A., Shiekh, N., Said, N., Ahmad, K., Gul, A., Hassan, L., et al. (2020). Fake news detection in social media using graph neural networks and NLP techniques: a COVID-19 use-case. arXiv preprint arXiv:2012.07517.
Hansen, P. G., and Jespersen, A. M. (2013). Nudge and the manipulation of choice: a framework for the responsible use of the nudge approach to behaviour change in public policy. Eur. J. Risk Regul. 4, 3–28. doi: 10.1017/S1867299X00002762
Kang, H. B., Kocielnik, R., Head, A., Yang, J., Latzke, M., Kittur, A., et al. (2022). “From who you know to what you read: augmenting scientific recommendations with implicit social networks,” in Proceedings of the 2022 CHI Conference on Human Factors in Computing Systems 1–23. doi: 10.1145/3491102.3517470
Kocielnik, R., Amershi, S., and Bennett, P. N. (2019). “Will you accept an imperfect AI? Exploring designs for adjusting end-user expectations of ai systems,” in Proceedings of the 2019 CHI Conference on Human Factors in Computing Systems 1–14. doi: 10.1145/3290605.3300641
Kou, Y. (2020). “Toxic behaviors in team-based competitive gaming: The case of league of legends,” in Proceedings of the Annual Symposium on Computer-Human Interaction in Play 81–92. doi: 10.1145/3410404.3414243
Kriz, W. C. (2020). Gaming in the time of COVID-19. Simul. Gam. 51, 403–410. doi: 10.1177/1046878120931602
Kwak, H., Blackburn, J., and Han, S. (2015). “Exploring cyberbullying and other toxic behavior in team competition online games,” in Proceedings of the 33rd Annual ACM Conference on Human Factors in Computing Systems 3739–3748. doi: 10.1145/2702123.2702529
Lakomy, M., and Bożek, M. (2023). “Understanding the trauma-related effects of terrorist propaganda on researchers,” in Global Network on Extremism and Technology.
Lapolla, M. (2020). Tackling toxicity: Identifying and addressing toxic behavior in online video games. Seton Hall University Dissertations and Theses (ETDs). 2798.
League, A. D. (2023). Anti Defamation League Homepage Org|ADL. Available online at: https://www.adl.org/ (accessed April 29, 2023).
Lei, Y., Huang, R., Wang, L., and Beauchamp, N. (2022). “Sentence-level media bias analysis informed by discourse structures,” in Proceedings of the 2022 Conference on Empirical Methods in Natural Language Processing 10040–10050. doi: 10.18653/v1/2022.emnlp-main.682
Levkovitz, Z. (2023). Content moderators alone can't clean up our toxic internet. Available online at: https://www.fastcompany.com/90515733/content-moderators-alone-cant-clean-up-our-toxic-internet (accessed April 20, 2023).
Lin, H., and Sun, C.-T. (2005). “The ‘white-eyed' player culture: Grief play and construction of deviance in MMORPGs,” in DiGRA Conference.
Ling, C. X., and Sheng, V. S. (2008). Cost-sensitive learning and the class imbalance problem. Encyclop. Mach. Lear. 2011, 231–235. doi: 10.1007/978-0-387-30164-8_181
Link, D., Hellingrath, B., and Ling, J. (2016). “A human-is-the-loop approach for semi-automated content moderation,” in ISCRAM.
Luhmann, C. C., Chun, M. M., Yi, D.-J., Lee, D., and Wang, X.-J. (2008). Neural dissociation of delay and uncertainty in intertemporal choice. J. Neurosci. 28, 14459–14466. doi: 10.1523/JNEUROSCI.5058-08.2008
Ma, R., Li, Y., and Kou, Y. (2023). “Transparency, fairness, and coping: How players experience moderation in multiplayer online games,” in Proceedings of the 2023 CHI Conference on Human Factors in Computing Systems 1–21. doi: 10.1145/3544548.3581097
Malmasi, S., and Zampieri, M. (2017). Detecting hate speech in social media. arXiv preprint arXiv:1712.06427. doi: 10.26615/978-954-452-049-6_062
Märtens, M., Shen, S., Iosup, A., and Kuipers, F. (2015). “Toxicity detection in multiplayer online games,” in 2015 International Workshop on Network and Systems Support for Games (NetGames) (IEEE), 1–6. doi: 10.1109/NetGames.2015.7382991
McWhertor, M. (2022). Call of Duty 2022: Modern Warfare 2 Is This Year's Call of Duty. Available online at: https://www.polygon.com/23040804/call-of-duty-2022-modern-warfare-2 (accessed April 16, 2023).
Min, S., Lewis, M., Zettlemoyer, L., and Hajishirzi, H. (2022). “Metaicl: learning to learn in context,” in Proceedings of the 2022 Conference of the North American Chapter of the Association for Computational Linguistics: Human Language Technologies 2791–2809. doi: 10.18653/v1/2022.naacl-main.201
Ng, B. D., and Wiemer-Hastings, P. (2005). Addiction to the internet and online gaming. Cyberpsychol. Behav. 8, 110–113. doi: 10.1089/cpb.2005.8.110
Pfeiffer, J., Rücklé, A., Poth, C., Kamath, A., Vulić, I., Ruder, S., et al. (2020). “Adapterhub: a framework for adapting transformers,” in Proceedings of the 2020 Conference on Empirical Methods in Natural Language Processing (EMNLP 2020): Systems Demonstrations (Association for Computational Linguistics), 46–54. doi: 10.18653/v1/2020.emnlp-demos.7
Pluta, A., Mazurek, J., Wojciechowski, J., Wolak, T., Soral, W., and Bilewicz, M. (2023). Exposure to hate speech deteriorates neurocognitive mechanisms of the ability to understand others' pain. Sci. Rep. 13:4127. doi: 10.1038/s41598-023-31146-1
Quick, B. L., Shen, L., and Dillard, J. P. (2013). Reactance theory and persuasion. Dev. Theory Pract. 2, 167–183. doi: 10.4135/9781452218410.n11
Rasley, J., Rajbhandari, S., Ruwase, O., and He, Y. (2020). “Deepspeed: System optimizations enable training deep learning models with over 100 billion parameters,” in Proceedings of the 26th ACM SIGKDD International Conference on Knowledge Discovery &Data Mining 3505–3506. doi: 10.1145/3394486.3406703
Reicher, S. D., Spears, R., and Postmes, T. (1995). A social identity model of deindividuation phenomena. Eur. Rev. Soc. Psychol. 6, 161–198. doi: 10.1080/14792779443000049
Schlauch, W. E., and Zweig, K. A. (2015). “Social network analysis and gaming: survey of the current state of art,” in Serious Games: First Joint International Conference, JCSG 2015, Huddersfield, UK, June 3–4, 2015, Proceedings 1 (Springer), 158–169. doi: 10.1007/978-3-319-19126-3_14
Seering, J. (2020). Reconsidering self-moderation: the role of research in supporting community-based models for online content moderation. Proc. ACM Hum. Comput. Inter. 4, 1–28. doi: 10.1145/3415178
Sengün, S., Salminen, J., Jung, S.-G., Mawhorter, P., and Jansen, B. J. (2019). “Analyzing hate speech toward players from the MENA in league of legends,” in Extended Abstracts of the 2019 CHI Conference on Human Factors in Computing Systems 1–6.
Sharma, K., Donmez, P., Luo, E., Liu, Y., and Yalniz, I. Z. (2020). “Noiserank: unsupervised label noise reduction with dependence models,” in Computer Vision-ECCV 2020: 16th European Conference, Glasgow, UK, August 23–28, 2020, Proceedings, Part XXVII (Springer), 737–753. doi: 10.1007/978-3-030-58583-9_44
Sheth, A., Shalin, V. L., and Kursuncu, U. (2022). Defining and detecting toxicity on social media: context and knowledge are key. Neurocomputing 490, 312–318. doi: 10.1016/j.neucom.2021.11.095
Soral, W., Bilewicz, M., and Winiewski, M. (2018). Exposure to hate speech increases prejudice through desensitization. Aggr. Behav. 44, 136–146. doi: 10.1002/ab.21737
Srikanth, M., Liu, A., Adams-Cohen, N., Cao, J., Alvarez, R. M., and Anandkumar, A. (2021). “Dynamic social media monitoring for fast-evolving online discussions,” in Proceedings of the 27th ACM SIGKDD Conference on Knowledge Discovery &Data Mining 3576–3584. doi: 10.1145/3447548.3467171
Srinivasan, K. B., Danescu-Niculescu-Mizil, C., Lee, L., and Tan, C. (2019). Content removal as a moderation strategy: Compliance and other outcomes in the change my view community. Proc. ACM Hum. Comput. Inter. 3, 1–21. doi: 10.1145/3359265
Stewart, L. G., Arif, A., and Starbird, K. (2018). “Examining trolls and polarization with a retweet network,” in Proceedings of the ACM WSDM, Workshop on Misinformation and Misbehavior Mining on the Web 70.
Stoop, W., Kunneman, F., van den Bosch, A., and Miller, B. (2019). “Detecting harassment in real-time as conversations develop,” in Proceedings of the Third Workshop on Abusive Language Online 19–24. doi: 10.18653/v1/W19-3503
Strahan, E. J., Spencer, S. J., and Zanna, M. P. (2002). Subliminal priming and persuasion: striking while the iron is hot. J. Exper. Soc. Psychol. 38, 556–568. doi: 10.1016/S0022-1031(02)00502-4
Suh, M., and Hsieh, G. (2016). “Designing for future behaviors: understanding the effect of temporal distance on planned behaviors,” in Proceedings of the 2016 CHI Conference on Human Factors in Computing Systems 1084–1096. doi: 10.1145/2858036.2858591
Thurlings, M., Vermeulen, M., Bastiaens, T., and Stijnen, S. (2013). Understanding feedback: a learning theory perspective. Educ. Res. Rev. 9, 1–15. doi: 10.1016/j.edurev.2012.11.004
Turner, J. C. (1975). Social comparison and social identity: some prospects for intergroup behaviour. Eur. J. Soc. Psychol. 5, 1–34. doi: 10.1002/ejsp.2420050102
Tyagi, S., and Mittal, S. (2020). “Sampling approaches for imbalanced data classification problem in machine learning,” in Proceedings of ICRIC 2019: Recent Innovations in Computing (Springer), 209–221. doi: 10.1007/978-3-030-29407-6_17
Ubisoft. (2023). Player code of conduct. Available online at: https://www.ubisoft.com/en-us/company/about-us/codes-of-conduct/articles/code-of-conduct-the-way-we-play (accessed April 29, 2023).
Velioglu, R., and Rose, J. (2020). Detecting hate speech in memes using multimodal deep learning approaches: prize-winning solution to hateful memes challenge. arXiv preprint arXiv:2012.12975.
Verge, T. (2019). The secret lives of facebook moderators in america - the verge. Available online at: https://www.theverge.com/2019/2/25/18229714/cognizant-facebook-content-moderator-interviews-trauma-working-conditions-arizona (accessed June 22, 2023).
Voigt, P., and Von dem Bussche, A. (2017). The EU General Data Protection Regulation (GDPR). A Practical Guide, 1st Ed. Cham: Springer International Publishing 10–5555. doi: 10.1007/978-3-319-57959-7
Wijkstra, M., Rogers, K., Mandryk, R. L., Veltkamp, R. C., and Frommel, J. (2023). “Help, My Game Is Toxic! First Insights from a Systematic Literature Review on Intervention Systems for Toxic Behaviors in Online Video Games,” in Companion Proceedings of the Annual Symposium on Computer-Human Interaction in Play 3–9.
Zakrzewski, C. (2022). New California Law Likely to Set Off Fight Over Social Media Moderation. The Washington Post.
Zhao, J., Zhang, Y., Chen, B., Schäfer, F., and Anandkumar, A. (2023). Inrank: incremental low-rank learning. arXiv preprint arXiv:2306.11250.
Zhao, Y., and Liu, Q. (2023). Causal ml: python package for causal inference machine learning. SoftwareX 21:101294. doi: 10.1016/j.softx.2022.101294
Zhou, C., and Paffenroth, R. C. (2017). “Anomaly detection with robust deep autoencoders,” in Proceedings of the 23rd ACM SIGKDD International Conference on Knowledge Discovery and Data Mining 665–674. doi: 10.1145/3097983.3098052
Keywords: online games, toxicity, player behavior, content moderation, competitive action games, first-person action games, disruptive behavior moderation
Citation: Kocielnik R, Li Z, Kann C, Sambrano D, Morrier J, Linegar M, Taylor C, Kim M, Naqvie N, Soltani F, Dehpanah A, Cahill G, Anandkumar A and Alvarez RM (2024) Challenges in moderating disruptive player behavior in online competitive action games. Front. Comput. Sci. 6:1283735. doi: 10.3389/fcomp.2024.1283735
Received: 27 August 2023; Accepted: 24 January 2024;
Published: 23 February 2024.
Edited by:
Deller James Ferreira, Universidade Federal de Goiás, BrazilReviewed by:
Luciana Berretta, Universidade Federal de Goiás, BrazilSergio Carvalho, Universidade Federal de Goiás, Brazil
Rachel Kowert, Take This, United States
Copyright © 2024 Kocielnik, Li, Kann, Sambrano, Morrier, Linegar, Taylor, Kim, Naqvie, Soltani, Dehpanah, Cahill, Anandkumar and Alvarez. This is an open-access article distributed under the terms of the Creative Commons Attribution License (CC BY). The use, distribution or reproduction in other forums is permitted, provided the original author(s) and the copyright owner(s) are credited and that the original publication in this journal is cited, in accordance with accepted academic practice. No use, distribution or reproduction is permitted which does not comply with these terms.
*Correspondence: Rafal Kocielnik, cmFmYWxrb0BjYWx0ZWNoLmVkdQ==
†These authors have contributed equally to this work