- 1Chair of Communication Science, Human-Computer Interaction Center, RWTH Aachen University, Aachen, Germany
- 2Human-Computer Interaction and Usable Safety Engineering, Institute for Multimedia and Interactive Systems, Universität zu Lübeck, Lübeck, Germany
Introduction: The advent of mobile forensics toolkits introduces a technological leverage that allows legal authorities to access information stored on mobile devices, thus shining a new light on law enforcement procedures. These pocket-sized devices, mobile phones, accumulate a plethora of user data, effectively becoming a beacon for individual identification. However, the prospect of exploring this data within a criminal inquiry raises palpable concerns about potential privacy encroachments. Consequently, there exists an urgent need to balance the instrumental value of these technologies with their potential to intrude upon privacy, ensuring a framework that remains legally and ethically sound.
Methods: In our study, we offer a contemplative view on the public reception of such measures, informed by interviews and a conjoint study conducted across two representative cohorts from Germany and Austria (n = 2040).
Results: Our analysis indicates a marked preference for the release of geo-spatial data over more personal content, such as photographs. Additionally, respondents showed a higher acceptance for automated analysis in comparison to human manual evaluation. The divergence between the two countries was negligible.
Discussion: In summary, despite the inherent concerns, the use of these mobile forensics tools demonstrated a high degree of public acceptance. The results highlight the significance of aligning legislation based on data types rather than analysis purposes, which can enhance the general public's comprehension of laws and potentially contribute to societal advancement. Furthermore, the research emphasizes the importance of ethical evaluations and transparent communication in the implementation of automated mobile forensics systems for civil security purposes, effectively addressing concerns regarding privacy infringement and data analysis.
1. Introduction
There are enormous societal benefits associated with the increasing global collection and use of digital cloud data (Bryant et al., 2008; Gudivada et al., 2015). Amidst the demands for an effective and prosperous digital transformation, societies find themselves confronted with a crucial trade-off between comprehensive data collection and utilization for the greater good, on one hand, and the potential risks of data misuse on multiple levels, on the other (Dritsas et al., 2006; Anandaraj and Kemal, 2017; Jindal et al., 2023). Among the benefits, social and societal progress in all areas, e.g., health, commerce, mobility, production, security, and safety, has been reached and will still further develop through the availability of Big Data. However, the use of ubiquitous data comes with serious legal, technical, and social disadvantages, mainly in terms of privacy loss, intrusion into intimacy, and personal rights (Nissenbaum, 2010; Hayes et al., 2020; Huang et al., 2023). Hence, as a side effect of the digital transformation in general, digital information and communication technology devices have become not only carriers of sensitive data but also more and more unique to their user. Based on these characteristics, a smartphone could be used as a personal identifier and/or a carrier of evidence in a law enforcement scenario. Equipped with mobile forensic systems, law authorities can collect, examine, analyze, and report digital evidence without damaging the device (Dogan and Akbal, 2017; Losavio et al., 2018).
While this area of digital mobile forensics is mostly covered by the study of technical perspectives and possibilities, public acceptance and the informed decision for data sharing and its consequences are equally important. Hence, questions of social, legal, and ethical implications have to be discussed to ensure adequate democratic coverage (Gantz and Reinsel, 2012; Dhirani et al., 2023). Digital mobile forensics is an emerging field that specifically addresses processes, methods, and analytics of any material that can be collected in digital devices (Dritsas et al., 2006; Cruz-Cunha and Mateus-Coelho, 2020; Alenezi, 2023). Digital forensics is mostly related to the use of mobile device data in the context of crime prosecution (Carrier, 2003; Du et al., 2020; Saranya and Usha, 2023). Criminal application fields cover breaking the law in the context of data collection and analysis and use and involve legislative and executive entities but also civil cases in the context of privacy protection and personal rights.
The discourse around digital mobile forensics should not be confined to technical possibilities and capacities. The issue of public acceptance plays a substantial role and deserves equal consideration. Thus, it is crucial that we engage in a discourse around the social, legal, and ethical implications to ensure that democratic principles are upheld in the deployment of these technologies. As privacy is a fundamental right (Pfisterer, 2019) and information privacy comprises the control over (the access and use of) personal information (Ermakova et al., 2014), dilemmas may arise between the individual right to privacy and various benefits of mobile forensics for law enforcement and public security, such as improved evidence provision (Al-Dhaqm et al., 2020) or detection of suspicious patterns (Barmpatsalou et al., 2018).
Mobile phones inadvertently collect an extensive array of data without the user's awareness or control over the data collection process, such as dating apps analyzing music preferences (Hayes et al., 2020). Ordinarily, these data are not shared with third parties. However, in law enforcement scenarios, such data may be employed. This distinguishes mobile forensics from other privacy-sensitive domains such as social networks or smart homes, where users intentionally disclose personal information to data providers. Furthermore, there is insufficient research examining the evaluation of these data by indirect users who do not directly interact with the system. It is important to differentiate between direct and indirect users, with direct users engaging in direct interactions with the system while indirect users are affected by its use, even without direct interaction (ISO, 2011). Indirect users, in this context, are the owners of phones that are being analyzed by criminal investigators, as owners of smartphones never interact with the mobile forensic software directly. Another example would be uninvolved peers of suspects—whose mobile phones were confiscated—as data of the peers might be on that phone.
The preservation of privacy is a fundamental right (Pfisterer, 2019). Control over personal information, which forms the cornerstone of information privacy according to the study by Ermakova et al. (2014), presents an intriguing quandary when juxtaposed with the advantages of mobile forensics. This technology equips law enforcement and public security agencies with enhanced capabilities, including effective evidence collection (Al-Dhaqm et al., 2020) and the identification of suspicious patterns (Barmpatsalou et al., 2018). These benefits are made possible by the copious amount of data that mobile phones amass as part of their regular operation, often without the user's knowledge or consent. Consequently, there is an urgent need for improved policymaking (Hayes et al., 2020). It is important to note that, in normal circumstances, this information remains isolated and is not shared with third parties.
However, the narrative changes dramatically in a law enforcement scenario where this data may be actively exploited. This situates mobile forensics distinctly apart from other privacy-sensitive spheres such as social networks or smart homes, where personal data are knowingly and willingly shared with service providers. What remains inadequately explored is the evaluation of these systems, particularly from the perspective of “indirect users,” who may never interact with the system directly, yet find themselves affected by its use (ISO, 2011). This distinction between direct and indirect users unveils an important layer of user interaction and its implications for privacy and security demand further investigation.
2. Questions addressed and empirical procedure
We aim to provide first insights into the public's acceptance of and preferences for mobile forensics. To do so, we use a two-step empirical research approach. In the first exploratory step, we explore the public's opinion on mobile forensics and important influencing factors using guided interviews. In the second step, we apply a choice-based conjoint approach to experimentally assess the importance of relevant influencing factors based on large, census-representative samples from Germany (n = 1,039) and Austria (n = 1,001). The selection of countries refers to a joint project in which both countries were involved.
The joint project SmartIdentifikation aimed to explore the feasibility of utilizing smartphone-based or border-collected data in a socially accepted, ethically sound, and legally secure manner for analyzing refugee flows, detecting smuggling routes, and identifying involved individuals [Federal Ministry of Education and Research, BMBF]. By employing a two-country setting, we were able to shed light on potential international police cooperation, a vital element in investigating crimes such as human trafficking (Marturana et al., 2011). Additionally, this approach allowed us to address the research gap concerning comparative analysis (Kimmelmann et al., 2022) among states already engaged in border and migration control cooperation (Karamanidou et al., 2020; Comte and Lavenex, 2022), thus enhancing our understanding of factors that influence civil society participation in the context of refugee migration and integration (Simsa, 2017).
Our study primarily focused on exploring the public perception and acceptance of mobile forensics. We aimed to identify the key factors driving public acceptance of such technologies and determine which types of data, levels of automation, and analysis purposes were most widely accepted.
We thereby contribute to an understanding of the public's acceptance of mobile forensics as well as privacy preferences of people, whose data may be used but who are not in control over its usage, e.g., contact persons of crime suspects. The results contribute to a better understanding of public privacy perception and provide implications for developers and investigators.
3. Materials and methods
In the following, we describe our two-step consecutive empirical research approach: an exploratory qualitative interview study and a choice-based conjoint (CBC) online survey.
3.1. Interview study
To gain a first understanding of the acceptance of mobile forensics use cases and identify factors to be included in the CBC study, interviews with German citizens of different age groups, gender, and (professional) background were conducted. The interview study was guided by the following research question:
• RQ1: What are the relevant factors driving the public acceptance of mobile forensics?
To answer this research question, we conducted semi-structured guideline-based interviews with a professional moderator. The average interview length was approximately 20 min and participants were allowed to abort the interview at any time. The topic of mobile forensics was introduced by a narrative scenario of two investigators talking about mobile forensics. Participants were asked about their previous knowledge and feelings about this technology, their conditionals and concerns for an accepted usage, and, in particular, about a sub-scenario where not only the smartphone of the subject is used for investigation but also the device of a close relative. A final question was asked to the interview participants about their general attitude and feelings again.
All interviews were anonymized and converted to text according to GAT2 basic transcription convention (Selting and Auer, 2011). Every transcript was chosen as a sampling unit and fully considered as a recording unit; short stand-alone responses as the content unit and detailed answers as the context unit (Krippendorff, 2018). Qualitative analysis was conducted as thematic qualitative text analysis by Kuckartz (2014). After the first step of initial text work, four deductive main categories were identified: General attitude, Type of data, Conditionals, and Role of other people's data.
Participants were recruited to cover different age groups, genders, and education levels. We aimed for a random sample of the general population in order to capture typical average behaviors of mobile phone users. Overall, n = 9 interviews were conducted with 4 female and 5 male participants of varying professions. Age ranged from min = 24 years to max = 72 years with an average of M = 44.66(SD = 16.40), while 3 participants were of higher, 4 of intermediate, and 2 of lower scholarly education levels. All participants were frequent smartphone users with no prior knowledge of mobile forensics. Participation was not gratified, and all participants volunteered to take part in the interview study.
3.2. Choice-based conjoint (CBC) study
Building on the findings from the interview study, a CBC questionnaire was designed. The leading research questions for the conjoint study were as follows:
• RQ2: How important are the three factors such as type of data, automation level, and analysis purpose for public acceptance?
• RQ3: Which types of data, automation levels, and analysis purposes are most accepted?
• RQ4: Are there differences in these preferences between the German and Austrian public?
In CBC, complex decision processes can be mimicked as several factors are evaluated in conjoint and need to be weighed against each other, which provides a more realistic evaluation situation than typical rating scales (Sawtooth Software, Inc.). CBC is a decompositional procedure, meaning that participants choose their favorite from several presented options. From this, the relevance of individual attributes is derived based on the assumption that the preference for the overall product/option is a function of a set of explanatory variables, named attributes (Baier et al., 2009). In CBC, these attributes are represented by their associated levels which are experimentally varied across the presented options. For example, the attribute “color” would be represented by varying levels such as “green,” “red,” and “blue”.
3.2.1. Selection of attributes
The selection of attributes and their associated levels, i.e., the operationalization of the variables, is critical as the results are relative to one another and are valid only for the used combination of attributes and levels. Therefore, the most relevant attributes of a decision-making process must be covered (Johnson and Orme, 2003). Because of the exploratory nature of our study, the attributes are selected based on the focus groups' findings, and the corresponding levels are developed to reflect mobile forensics realistically and be comprehensive for the participants. Table 1 displays the attributes and their associated levels.
As one essential factor for the acceptance of mobile forensics, the type of data was identified in the focus groups. The type of data—and its individually perceived sensitivity—is one of the main influences on the acceptance of data collection and analysis, which is also confirmed by empirical research in other contexts, in which the data provider intentionally discloses the data (Li, 2011; Mothersbaugh et al., 2012; Schomakers et al., 2020). As the perceived sensitivity of data increases, individuals tend to have higher privacy concerns regarding its usage, which consequently leads to a lower willingness to disclose that data (Bansal et al., 2010; Mothersbaugh et al., 2012). Previous research has indicated that the perception of data sensitivity is influenced by various factors. For instance, the sensitivity of data can be influenced by whether it contains personally identifying information (Malheiros et al., 2013), is associated with physical, monetary, social, or psychological risks (Milne et al., 2017), and originates from a particular source (Rohm and Milne, 2004). Additionally, the perception of information sensitivity is highly individual and dependent on culture (Markos et al., 2017; Schomakers et al., 2019). Location data have been highlighted as very sensitive data (besides medical and financial data which are not included here) (Staiano et al., 2014; Milne et al., 2017; Schomakers et al., 2019, 2020). As privacy and privacy-related preferences are also strongly dependent on the context (Nissenbaum, 2010; Acquisti et al., 2015; Schomakers et al., 2021b), an assessment of the acceptance of data types for mobile forensics is still needed.
Based on previous research, we hypothesize that:
• H1: The analysis of location data is less accepted than the analysis of the other types of data. The hypothesis is based on previous findings from the literature which suggest that location data are perceived as highly sensible and intrusive from the users' point of view (Staiano et al., 2014; Milne et al., 2017; Schomakers and Ziefle, 2019; Schomakers et al., 2020).
Furthermore, the interview results shed light on the privacy invasion by the person who accesses and investigates the data. However, mobile forensics does not necessarily require a human investigator manually looking at the data; also automated data analysis using artificial intelligence is possible (Sikos, 2021). Therefore, it is highly interesting to gain insights into the public's preferences for either manual or automated data analysis. As the interview findings suggest that one of the key privacy concerns for mobile forensics is the human investigator looking at personal data, automated data analysis may reduce the perceived privacy intrusion. Hypothesis 2 is, therefore, related to the qualitative study which has been carried out prior to the Choice-Based Conjoint study. Hypothesis H2 reads:
• H2: Automated data analysis is preferred to manual data analysis. This hypothesis is based on our qualitative findings (see Section 4.1).
We identified the increased civil security as well as the severity of the crime to be additional conditionals for the acceptance of mobile forensics. These factors describe the purposes of the analysis and refer, on the one hand, to the societal benefit of the data analysis, and, on the other hand, to the legitimacy of the data analysis. The importance of the gained (individual and/or societal) benefit for privacy decisions has been emphasized in various studies (Dinev et al., 2006; Calero Valdez and Ziefle, 2019; Schomakers et al., 2021b), and the purpose of data collection and analysis is of high relevance for privacy rights (Voigt and Von dem Bussche, 2017; Jasserand, 2018).
A relevant research duty for democratic coverage is to gain insights into the public's acceptance of mobile forensics for different analysis purposes. Particularly, the differentiation of crime prevention vs. crime prosecution is highly relevant as it is an important differentiation for law enforcement bodies and their legal rights. However, empirical data about the public's preferences regarding the use of mobile forensics in these cases are lacking.
From a benefit perspective, an even higher benefit may arise from the prevention of planned crimes in contrast to the prosecution of past crimes. However, the legitimacy of investigating the data of persons who may plan to commit a crime may be controversial—as elegantly discussed in the Hollywood movie Minority Report. Therefore, we study the public preferences regarding the analysis purpose, distinguishing crime prosecution and crime prevention. Considering our interview results, the barriers of crime prevention are hypothetically higher because of negative narratives (e.g., “surveillance state”).
• H3: Mobile forensics for crime prosecution is more accepted than crime prevention. This hypothesis is based on our qualitative findings (see Section 4.1).
3.2.2. The study design
The choice tasks were embedded into a questionnaire, which introduced the topic of mobile forensics and assessed sociodemographic characteristics as well as key attitudes of the respondents. The questionnaire started with questioning age, gender, education, and profession. In the second part, technology readiness (Neyer et al., 2012) and disposition to value privacy (Xu et al., 2008) were addressed using six-point Likert scale. The final question of the survey was a single net promoter score item (1–10) whether participants felt positive or negative about the use of mobile forensics.
For the CBC part, the participants were asked to put themselves in the following scenario: Imagine that a person suspected of being connected to a crime has been picked up and is carrying a smartphone. This smartphone has been confiscated as evidence and is now to be evaluated to preserve evidence. Then, 10 choice tasks were carried out. In each choice task, the participants were presented with two options comprised of the three attributes, such as type of data (what), analysis automation level (how), and analysis purpose (why) with varying levels (cf. Figure 1). The task was to choose the most acceptable option.
3.2.3. Recruiting and data analysis
The survey data were gathered from panel members of an independent research company. Quotas were set to acquire a census-representative sample regarding age, gender, federal state, and education level of adults from Austria and Germany. The quality of the data was ensured using several attention questions and excluding speeders as well as incomplete data sets.
The conjoint data were analyzed using Hierarchical Bayes (HB) estimation to assess individual-level values for the relative importance of the attributes and the part-worth utilities of the levels. The relative importance shows how important each attribute is for the acceptance of mobile forensics. The part-worth utilities describe the preferences for the levels. These are reported as zero-centered differences to allow comparisons between the levels of the different attributes. The mean values for the German and Austrian subsamples are calculated and reported separately. The mean root likelihood of the model is 0.65. As two concepts are shown per choice task, the worst would be 0.5 so that the estimates fit the model moderately.
To test differences between the Austrian and German samples, t-tests were used in the sample description. For the conjoint data, Bayesian t-tests were used on the individual zero-centered utility scores and the individual relative importance. We report the t-test results alongside the Bayes factor as these are more commonly known, but we interpret mainly the Bayes factor. The level of significance was set at 5%. To avoid alpha error inflation, Bonferroni–Holm correction is used on the significance values when several tests were calculated.
3.2.4. Sample description
The sample comprises 1,001 Austrian and 1,038 German participants. The demographic characteristics of the sample are displayed in Table 2 for the overall sample and the national subsamples.
The technology readiness of the sample is rather high (M = 4.40, SD = 0.87) and shows no significant differences between the German and Austrian samples [t(2,037) = 1.30, p = 0.193]. The privacy disposition is rather high as well with M = 4.01 (SD = 1.11) and shows no significant differences between the nations [t(2,037) = 1.91, p = 0.056].
4. Results
4.1. Qualitative interview results
Figure 2 shows the final result of the thematic content analysis, with Challenges being an inductive main category. Overall, we could identify 156 content units and 16 inductive sub-categories.
While asking participants about their general attitude toward the provided mobile forensics scenario, most participants expressed a positive or even enthusiastic position, as far as some conditions were met. Only a few participants were rather skeptical, and none was fundamentally against mobile forensics.
As already mentioned, participants were not explicitly asked about the types of data that could be used for investigation. They mentioned local data, such as GPS or mobile network, text message data, that are stored directly on the device (SMS / MMS), and messenger data, that are stored in apps or servers, device data such as contacts or phone identifiers, as well as user account data for social networks or online forums. All of these types of data were mentioned without a specific connotation in terms of privacy but more under the perspective of data variety. Image data, such as pictures or camera images, were described as quite privacy intrusive or even intimate.
When talking about conditionals for mobile forensic systems, participants stated that the system should lead to increased civil security by providing clear evidence and faster investigation. In addition, mobile forensics might also provide a feeling of security through crime prevention, either by bringing criminals to justice and therefore preventing further crimes, but also by preventing terrorist attacks.
While discussing the privacy invasion of the system, participants implicitly tied the invasiveness to human primary users. They argued that they might feel uncomfortable thinking about other people investigating their smartphones and noticed that policemen (not police as an institution) could see everything they want. One participant even argued that policemen should put under surveillance while using the system to prevent privacy intrusion.
Data security was found to be a major conditional in a way that only the executing police should be able to use the data for an explicit investigation. Finally, subjects disagreed on whether the system should be used for particularly serious crimes, such as murder, or also for lighter ones, such as vandalism.
When being asked about the role of other people's data, some participants noticed during the interview that most types of data carry information about non-suspected individuals, i.e., message data always contains information about at least one other individual. In addition to that, participants mostly argued that the analysis of suspects' smartphones and data—with the already mentioned characteristics—should be the standard case of mobile forensics. On the other hand, the analysis of the data of suspects' contact persons was considered potentially useful or even generally accepted. One participant raised the condition that the smartphone of contact persons should be provided voluntarily, and another participant mentioned that close relatives of subjects might have the right to refuse to testify, which should be adapted to this digital context. Interestingly, when discussing the data of completely uninvolved people, some participants switched their perspectives and ensured that they would provide their own smartphone data to help the police. This was explained by the fact that this does not cause any harm to the participant and could furthermore contribute to the fight against crime.
The last main category contains upcoming challenges. Two participants mentioned that the benefit of investigation might arise only from the aggregation and correlation of data, such as people being in the same place on a regular basis. Furthermore, a mobile forensic system might even increase the effort for investigators by providing a huge amount of data or organizational effort, and finally, it was mentioned that data manipulation might provide an alibi to a criminal.
4.2. Conjoint study results
Table 3 provides descriptive statistics for attribute importance scores for Germany, Austria, and both countries combined. Overall, the type of data was of the highest relative importance (M = 48.54, SD = 17.86), followed by the type of analysis (M = 31.48, SD = 18.86) and analysis purpose (M = 19.97, SD = 15.34).
The following bar chart (Figure 3) shows that the aforementioned order of average stays the same for German and Austrian subsamples. The inferential comparison yields a significant difference in the importance of the analysis purpose [], with analysis purpose being more important in Austria (M = 20.96, SD = 16.03) than in Germany (M = 19.03, SD = 14.67). However, this difference has a small effect size (d = 0.13).
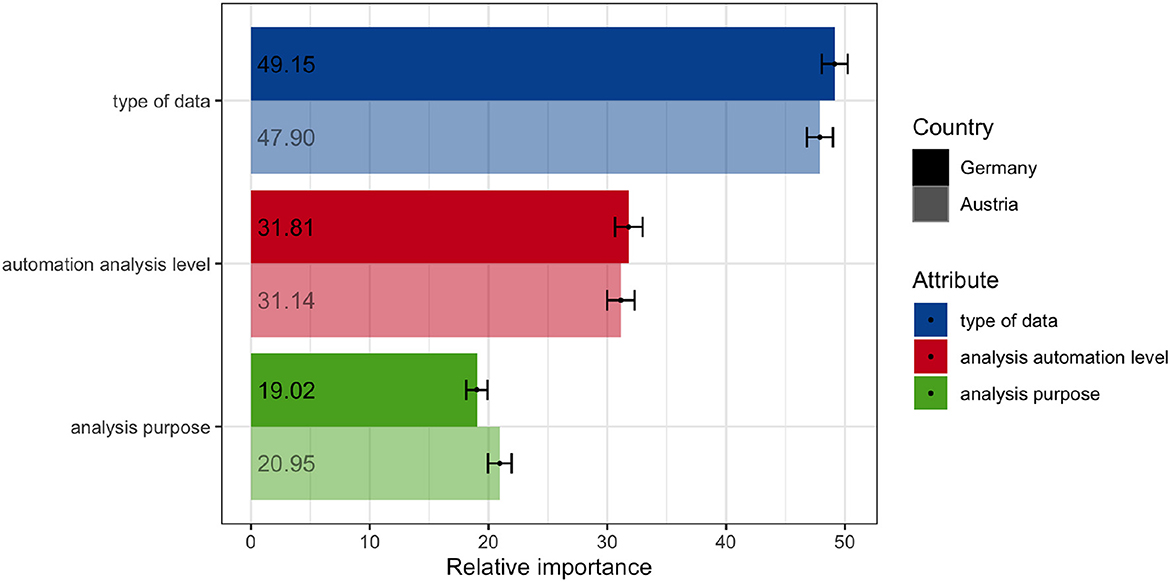
Figure 3. Average relative importance scores in bilateral comparison between Germany (n = 1,039) and Austria (n = 1,001). Error bars denote 95% CI, and labels denote the average score.
Accordingly, Table 4 shows descriptive statistics and comparison for part-worth utilities. The most accepted type of data to be analyzed is location data (M = 62.16, SD = 43.31), followed by sms/mms data (M = 4.03, SD = 38.00). Data analysis, messenger data (M = −3.06, SD = 38.72), device data (M = −6.88, SD = 56.90), user account data (M = −23.67, SD = 40.30), and image data (M = −32.59, SD = 43.07) are less preferred. Regarding the other two attributes, automated analysis is favored over manually (M = 18.04, SD = 52.03, resp., M = −18.04, SD = 52.03), and analysis for prosecution is favored over prevention (M = 15.85, SD = 34.33, resp., M = −15.85, SD = 34.33).
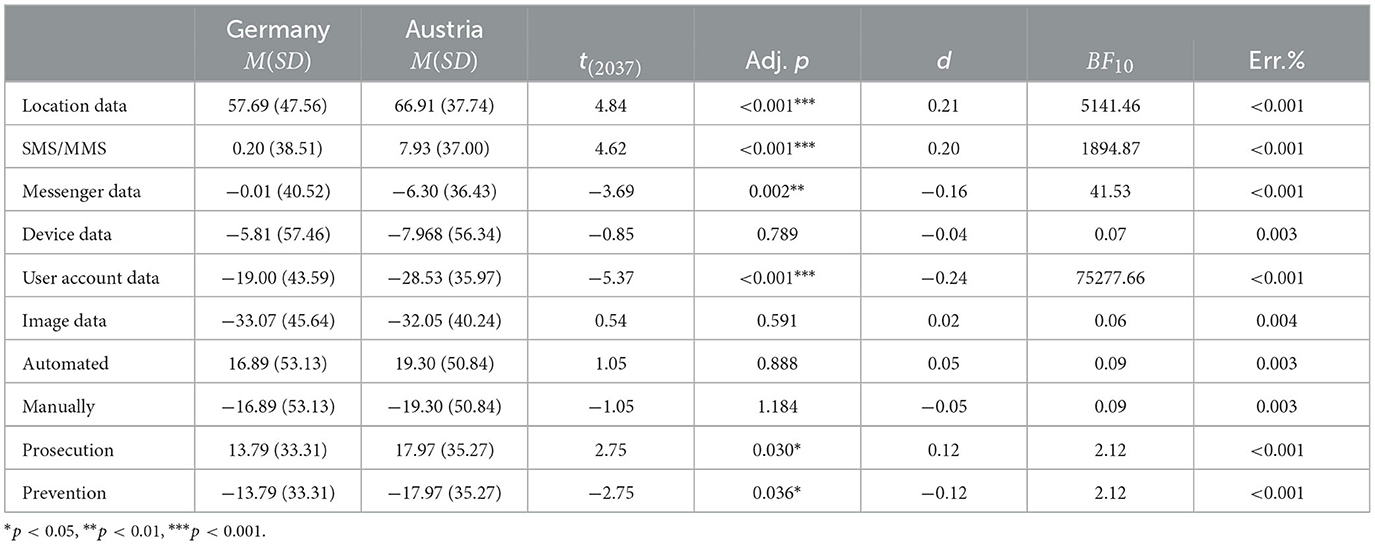
Table 4. Comparative descriptive statistics and Bayesian country comparison for part worth utility levels.
Comparing Germany and Austria, there are four significant differences in the part-worth utilities of different data types. Location data [] are slightly (d = 0.21) more favored in Austria (M = 66.91, SD = 37.74) than in Germany (M = 57.69, SD = 47.56). The significant difference regarding sms/mms [] is of similar effect size (d = 0.20) and in the same direction: the analysis of sms/mms is slightly more accepted in Austria (M = 7.93, SD = 37.00) compared with Germany (M = 0.20, SD = 38.51). On the other hand, messenger data [t(2,037)=-] and user account data [t(2,037)=-] are slightly more accepted in Germany (messenger data M = −0.01, SD = 40.52; user account data M = −19.00, SD = 43.59) than in Austria (messenger data M = −6.30, SD = 36.43; user account data M = −28.53, SD = 35.97). As with the previous ones, those effects are weak (messenger data d = −0, 16, user account data d = −0.20).
Finally, regarding the analysis purpose, there is another weak (d = 0.12 resp. d = −0.12) yet significant effect [, resp. ]. Data analysis with the aim of prosecution is slightly more accepted in Austria (M = 17.97, SD = 35.27) than in Germany (M = 13.79, SD = 33.31), respective prevention of crime is slightly more accepted in Germany (M = −13.79, SD = 33.31) than in Austria (M = −17.97, SD = 35.27).
In summary, the type of data is more important than the automation analysis level and the analysis purpose, and the most accepted mobile forensic system uses location data and operates automated for the purpose of crime prosecution. Overall, participants were positive about the use of mobile forensics as described in the questionnaire and as stated in the net promoter score item (M = 7.25, SD = 2.45, Md = 8.00).
5. Discussion
In our study, we employed a mixed-method approach, combining qualitative interviews and a conjoint study, to compare the importance of various attributes in the context of mobile forensics. Our primary research goals were two-fold: First, to identify the relevant factors influencing public acceptance of mobile forensics; and second, to gain a deeper understanding of the disparities in mobile forensic system characteristics and the divergent perceptions between individuals in Germany and Austria.
5.1. Key findings
Our findings revealed substantial disparities in the average importance assigned to different attributes, namely, data particles, type of analysis, and analysis purpose. Notably, we unearthed a significant discrepancy that highlights the type of data as the most influential factor in determining the acceptance of mobile forensic approaches, followed by the type of analysis, be it automated or manual, which carries approximately half the weight in decision-making processes. Conversely, the purpose of analysis emerged as the least influential factor.
Regarding the average importances, we observed only marginal distinctions between Austria and Germany, with minimal variations detected across a few levels.
The survey yielded a key finding that underscores the varying degrees of sensitivity associated with different types of data. Specifically, when it comes to geospatial data, which can potentially lead to the easy and unique identification of users, the acceptance of its use was found to be the highest. On the other hand, image data exhibited the lowest acceptance of use. An intriguing observation emerged when considering the preferred mode of analysis: Participants surprisingly favored analysis conducted by automated systems over analysis performed by manually investigating officers. Evidently, the act of having our data viewed by a human was perceived as a more invasive breach of privacy compared with examination by an automated system, despite the latter's ability to scrutinize our data in a more comprehensive manner. Additionally, the analysis of qualitative data shed light on the overall positive perception of mobile forensic systems. This positive perception, however, hinges on the condition that the system design adequately addresses both primary and indirect users' requirements.
Contrary to our initial hypothesis (H1), the acceptance of location data was unexpectedly high among the subjects. It appears that participants may not be fully aware of the potential invasiveness associated with location data. Alternatively, it is plausible that location data are perceived as particularly useful or beneficial in some way, leading to its greater acceptance. Conversely, the analysis of image data was perceived as invasive and intimate. This suggests that participants recognize the personal and sensitive nature of image data, which could explain the lower acceptance of its use compared with other types of data.
Hypothesis H2 has been confirmed by the findings, as subjects exhibited a preference for automated analysis over manual analysis. This preference suggests that participants are more inclined toward the utilization of automated systems for evaluating data. Moreover, the perception that automated evaluation is less intrusive could be attributed to the absence of human involvement in directly inspecting, for example, images. Instead, the use of algorithms in the automated analysis might be perceived as less invasive. This observation supports the notion that users consider the level of effort exerted by investigators when evaluating the acceptability of data analysis methods. H3 could also be confirmed, as prosecution is more accepted than crime prevention. This might be due to dystopian connotation but also because of further and more in-depth conditionals.
Overall, we found only minor differences between Germany and Austria, in terms of privacy perceptions (Figure 4). The disparities in the sensitivity perception of different types of data between the two cultures also appear to be influenced by distinct privacy laws and practices, as highlighted by previous studies (Schomakers et al., 2019, 2021a). However, based on our acceptance study, there is little evidence to suggest that international cooperation on a bilateral level would be problematic, as the results demonstrate a high degree of similarity between the two countries. Comparatively, Germans tend to exhibit higher privacy concerns compared with many other countries, such as Turkey. Nevertheless, when comparing Germany to Austria, the differences in privacy concerns are relatively small (Krasnova and Veltri, 2010; Wilkowska et al., 2021). This similarity can potentially be attributed to cultural similarities, particularly the in-depth historical restrospection and reflection of World War II and subsequent political shifts. Furthermore, both Austria and Germany share a common ground in technology skepticism and media role (Metag and Marcinkowski, 2014).
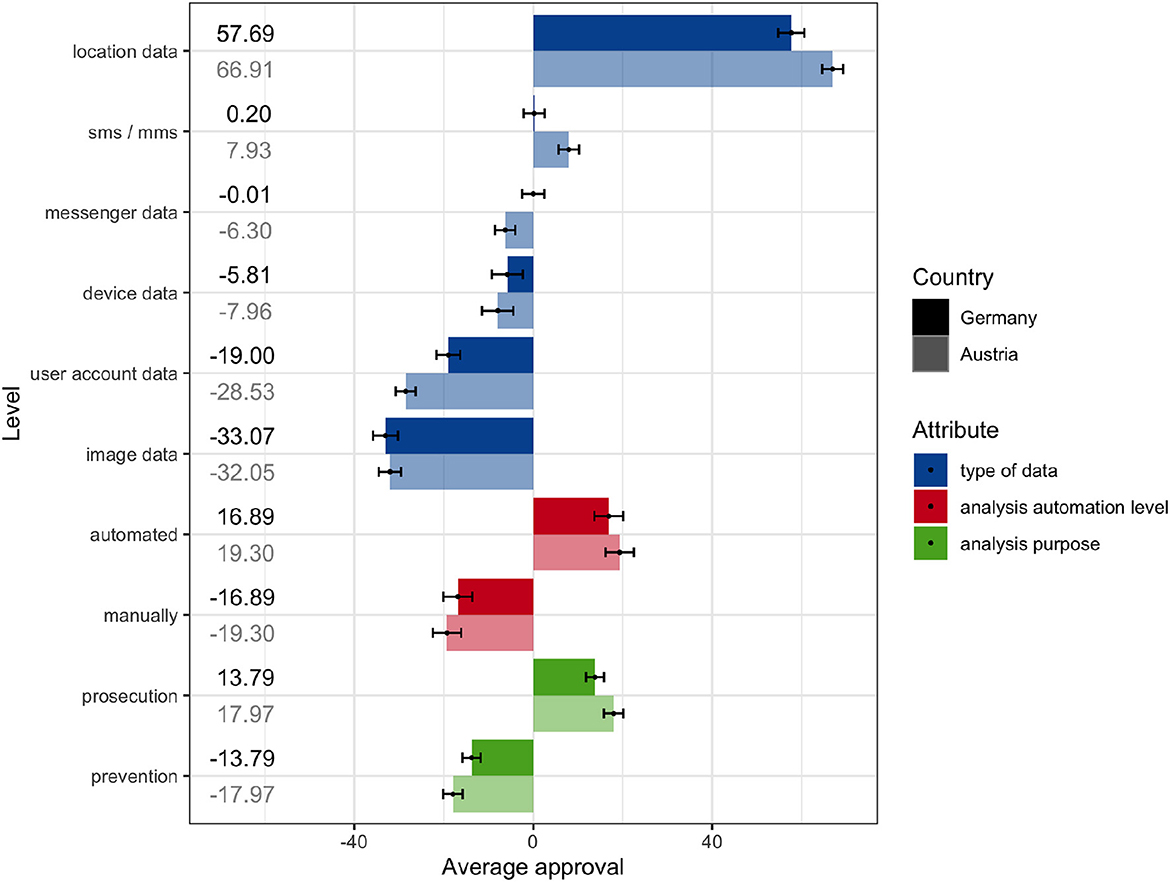
Figure 4. Average part-worth utilities in bilateral comparison between Germany (n = 1,039) and Austria (n = 1,001). Error bars denote 95% CI, and labels denote the average score.
5.2. Managerial recommendations and application
Several significant points emerge from the research that can provide valuable insights for various stakeholders and address societal issues.
5.2.1. Digital literacy and media education
First, there is a crucial need for enhanced digital literacy and media education. To make well-informed decisions concerning digital transformation, it is essential for both media and academic institutions to place greater emphasis on fostering digital literacy. A recent survey conducted in Europe (Eurostat, 2022) indicates varying levels of digital literacy across countries, highlighting the urgent necessity to educate citizens in all aspects of digital and mobile media usage (Ribble et al., 2004). Ribble et al. argue that digital education plays a pivotal role in promoting public awareness regarding the appropriate and intentional use of digital media, as well as a deep understanding of the consequences associated with digital behaviors, encompassing what is often referred to as Digital Citizenship.
“Digital citizenship can be defined as the norms of behavior with regard to technology use. As a way of understanding the complexity of digital citizenship and the issues of technology use, abuse, and misuse, we have identified nine general areas of behavior that make up digital citizenship” (Ribble et al., 2004).
This claim includes a global call to action in media education and data literacy that includes knowledge about the potential dangers and benefits when using digital media (Rouvroy and Poullet, 2009; Ziefle et al., 2016). This claim is directed to public education and policymakers but is increasingly shifted to an individual responsibility of mobile device users (Rouvroy and Poullet, 2009; Tene and Polonetsky, 2012).
By doing so, the public can be better equipped to engage in discussions about digital transformation and make informed decisions based on a comprehensive understanding of the subject matter.
Based on the findings, we can offer the following recommendations to developers of mobile forensic systems: A system that gains acceptance from the general population should primarily operate in an automated manner, prioritizing the support of the primary user in their mission to contribute to civil security. Location data, while potentially beneficial in combating crime, present the least problematic aspect in this regard. There may even be potential for voluntary provision of such data, providing that robust measures are in place to mitigate any risks of data misuse.
In future, it will be crucial to determine the extent to which the analysis of image data continues to be regarded as intrusive, even in scenarios where human users do not have direct access to th image material.
5.2.2. Implications for legal authorities and policymakers
In light of the findings, it is advisable to recommend that legislative authorities align law formulations based on the types of data rather than the purpose of analysis. This approach would enhance the understandability and comprehensibility of laws for the general public. Such a shift could potentially mitigate the escalating tensions in the migration debate, which is often influenced by perceptions of the legality and enforcement of applicable laws. Moreover, this could indirectly contribute to the overall improvement of society by fostering civic engagement through voluntary initiatives and honorary roles.
Furthermore, the results have implications for police investigations, as they reveal that privacy infringements resulting from data evaluations are determined by the type of data rather than the objective of the evaluation. Notably, as the level of system automation increases, the intensity of privacy invasion decreases. Consequently, it is crucial to ensure a high degree of acceptance of the automation component in systems designed to support criminal investigations. This becomes especially pertinent when incorporating AI assistance, as users' mental models significantly influence their perceptions. Additionally, the findings emphasize the necessity of conducting ethical, legal, and social evaluations of automated mobile forensics in comparison to existing alternatives. While an AI-based approach may present certain challenges in absolute terms, it may be comparatively less problematic than the alternatives currently available. Similarly, when discussing the gradient of automation, debates often focus on complete AI replacement of tasks and jobs, while the optimal solution may involve autonomous user support (Ausat et al., 2023).
In their public communications, authorities employing mobile forensics should emphasize the advantages for civil security while transparently disclosing the initial suspicion and technology-specific benefits. The critical question remains: Whose data are being analyzed and for what purpose? Notably, the data of suspects are considered less sensitive than that of their immediate contacts. Although the latter group may be connected to the crime and their data could assist in its resolution, the qualitative analysis revealed a greater challenge in gaining acceptance for this scenario compared with the analysis of data from entirely unrelated individuals. Interestingly, even those uninvolved persons may still feel they have nothing to hide (Cho et al., 2010).
Overall, a considerable segment of the population in Germany and Austria maintains a favorable perception of mobile forensics. However, this positive view is contingent upon the implementation of effective safeguards against data misuse and the establishment of robust measures to ensure the integrity and resistance to forgery of digital evidence.
5.3. Future research
Further research is imperative to investigate potential variations among indirect users, which may give rise to non-critical combinations, especially concerning automated evaluation and the assessment of image data. Moreover, as we progress, the importance of privacy-compliant AI training will continue to escalate, potentially fostering a novel research domain centered on ethical and environmentally friendly AI training methodologies (Verdecchia et al., 2023). Furthermore, the level of concern pertaining to the aggregation of diverse datasets remains a subject for future investigation.
Author's note
Prior to starting the procedure, the participants were informed that it is of high importance to understand free opinions and attitudes on mobile forensics from the citizens' perspective and that we were very happy if they would share their opinions with us. Still, however we stressed that they are free in taking part or not. Participation in the interview study was completely voluntary, participation in the survey study was reimbursed. Further, we ensured a high standard privacy protection in both studies and let the participants know that none of their answers can be referred to them as persons. Demographic data were also submitted voluntarily and all participants were informed that on request their personal data would be deleted from our encrypted hard drives. After these careful explanations participants reported to feel well informed about the purpose and the aim of the study and their freedom to quit participation at any time. Regarding the privacy policy explanations, the participants reported to understand that high standards were applied and deliberately accepted participation. Participant privacy is a key value that our university has committed itself to uphold. From the comments in the open question fields at the end of the survey, we learnt that those participants were interested in the topic and were keen to look at the results, which we assured them to receive.
Data availability statement
The datasets presented in this study can be found in online repositories. The names of the repository/repositories and accession number(s) can be found below: https://osf.io/ybq9u/?view_only=ecea622e09e343d0baca083823d764bf.
Ethics statement
Ethical review and approval was not required for the study on human participants in accordance with the local legislation and institutional requirements. Written informed consent from the participants was not required to participate in this study in accordance with the national legislation and the institutional requirements.
Author contributions
JRH was responsible for designing both empirical studies, conducting both studies, analyzing the data, and drafting the manuscript. E-MS was responsible for the literature review, data analysis of the quantitative study, and drafting of the manuscript. MZ was responsible for general advisory and editing the manuscript. ACV was responsible for designing both empirical studies, drafting the manuscript, and general advisory. All authors contributed to the article and approved the submitted version.
Funding
This study was funded by the German Federal Ministry of Research and Education as a part of the SmartIdentifikation research project (Grant Number 13N14764).
Acknowledgments
We would like to express our gratitude to Linda Juskowiak and Maxime Metzler for their assistance and support in conducting this research. In the course of refining this manuscript, the authors used ChatGPT 4, a language model developed by OpenAI, to enhance the readability of individual paragraphs. The following prompt was provided: You are a copy-editor for an academic journal and provide improved versions of paragraphs in a document. The preferred style is a classic style. In the process, care was taken to ensure that only non-sensitive data were shared with the service. Furthermore, the authors ensured that the revisions made by ChatGPT 4 did not introduce new concepts or any form of novel intellectual property. The authors conscientiously reviewed and edited the output as necessary, and therefore, accept full responsibility for the final content presented in this publication.
Conflict of interest
The authors declare that the research was conducted in the absence of any commercial or financial relationships that could be construed as a potential conflict of interest.
Publisher's note
All claims expressed in this article are solely those of the authors and do not necessarily represent those of their affiliated organizations, or those of the publisher, the editors and the reviewers. Any product that may be evaluated in this article, or claim that may be made by its manufacturer, is not guaranteed or endorsed by the publisher.
References
Acquisti, A., Brandimarte, L., and Loewenstein, G. (2015). Privacy and human behaviour in the age of information. Science 347, 509–514. doi: 10.1126/science.aaa1465
Al-Dhaqm, A., Abd Razak, S., Ikuesan, R. A., Kebande, V. R., and Siddique, K. (2020). A review of mobile forensic investigation process models. IEEE Access 8, 173359–173375. doi: 10.1109/ACCESS.2020.3014615
Alenezi, A. M. (2023). Digital forensics in the age of smart environments: a survey of recent advancements and challenges. arXiv preprint arXiv:2305.09682. doi: 10.48550/arXiv.2305.09682
Anandaraj, S., and Kemal, M. (2017). “Research opportunities and challenges of security concerns associated with big data in cloud computing,” in 2017 International Conference on I-SMAC (IoT in Social, Mobile, Analytics and Cloud)(I-SMAC) (IEEE), 746–751.
Ausat, A. M. A., Massang, B., Efendi, M., Nofirman, N., and Riady, Y. (2023). Can chat GPT replace the role of the teacher in the classroom: a fundamental analysis. J. Educ. 5, 16100–16106. doi: 10.31004/joe.v5i4.2745
Baier, D. B., Baier, D., and Brusch, M. (2009). Conjointanalyse. Heidelberg: Springer. doi: 10.1007/978-3-662-63364-9
Bansal, G., Zahedi, F. M., and Gefen, D. (2010). The impact of personal dispositions on information sensitivity, privacy concern and trust in disclosing health information online. Decis. Support Syst. 49, 138–150. doi: 10.1016/j.dss.2010.01.010
Barmpatsalou, K., Cruz, T., Monteiro, E., and Simoes, P. (2018). Mobile forensic data analysis: suspicious pattern detection in mobile evidence. IEEE Access 6, 59705–59727. doi: 10.1109/ACCESS.2018.2875068
Bryant, R., Katz, R. H., and Lazowska, E. D. (2008). Big-data computing: creating revolutionary breakthroughs in commerce, science and society. Available online at: https://www.immagic.com/eLibrary/ARCHIVES/GENERAL/CRA_US/C081222B.pdf
Calero Valdez, A., and Ziefle, M. (2019). The users' perspective on the privacy-utility trade-offs in health recommender systems. Int. J. Hum. Comput. Stud. 121, 108–121. doi: 10.1016/j.ijhcs.2018.04.003
Carrier, B. (2003). Defining digital forensic examination and analysis tools using abstraction layers. Int. J. Digit. Evid. 1, 1–12.
Cho, H., Lee, J.-S. S., and Chung, S. (2010). Optimistic bias about online privacy risks: testing the moderating effects of perceived controllability and prior experience. Comput. Hum. Behav. 26, 987–995. doi: 10.1016/j.chb.2010.02.012
Comte, E., and Lavenex, S. (2022). Differentiation and de-differentiation in eu border controls, asylum and police cooperation. Int. Spectator 57, 124–141. doi: 10.1080/03932729.2022.2021011
Cruz-Cunha, M. M., and Mateus-Coelho, N. R. (2020). Handbook of Research on Cyber Crime and Information Privacy. Pennsylvania:IGI Global. doi: 10.4018/978-1-7998-5728-0
Dhirani, L. L., Mukhtiar, N., Chowdhry, B. S., and Newe, T. (2023). Ethical dilemmas and privacy issues in emerging technologies: a review. Sensors 23, 1151. doi: 10.3390/s23031151
Dinev, T., Bellotto, M., Hart, P., Russo, V., Serra, I., and Colautti, C. (2006). Privacy calculus model in e-commerce - A study of Italy and the United States. Eur. J. Inform. Syst. 15, 389–402. doi: 10.1057/palgrave.ejis.3000590
Dogan, S., and Akbal, E. (2017). “Analysis of mobile phones in digital forensics,” in 2017 40th International Convention on Information and Communication Technology, Electronics and Microelectronics (MIPRO) (IEEE), 1241–1244. doi: 10.23919/MIPRO.2017.7973613
Dritsas, S., Gritzalis, D., and Lambrinoudakis, C. (2006). Protecting privacy and anonymity in pervasive computing: trends and perspectives. Telemat. Inform. 23, 196–210. doi: 10.1016/j.tele.2005.07.005
Du, X., Hargreaves, C., Sheppard, J., Anda, F., Sayakkara, A., Le-Khac, N.-A., et al. (2020). “SOK: exploring the state of the art and the future potential of artificial intelligence in digital forensic investigation,” in Proceedings of the 15th International Conference on Availability, Reliability and Security (New York, NY: Association for Computing Machinery), 1–10. doi: 10.1145/3407023.3407068
Ermakova, T., Baumann, A., Fabian, B., and Krasnova, H. (2014). “Privacy policies and users' trust: does readability matter?” in AMCIS. Available online at: https://boris.unibe.ch/68895/
Eurostat. (2022). How many citizens had basic digital skills in 2021? — ec.europa.eu. Available online at: https://ec.europa.eu/eurostat/web/products-eurostat-news/-/ddn-20220330-1 (accessed June 30, 2023).
Federal Ministry of Education Research (BMBF) (2018). Available online at: https://www.sifo.de/sifo/en/research-projects/society/migration-issues/approved-projects-in-the-field-ivil-security-migration-issues.html (accessed June 30, 2023).
Gantz, J., and Reinsel, D. (2012). The digital universe in 2020: big data, bigger digital shadows, and biggest growth in the far east. IDC iView 2007, 1–16.
Gudivada, V. N., Baeza-Yates, R., and Raghavan, V. V. (2015). Big data: promises and problems. Computer 48, 20–23. doi: 10.1109/MC.2015.62
Hayes, D., Cappa, F., and Le-Khac, N. A. (2020). An effective approach to mobile device management: security and privacy issues associated with mobile applications. Digit. Bus. 1, 100001. doi: 10.1016/j.digbus.2020.100001
Huang, Y., Li, Y. J., and Cai, Z. (2023). Security and privacy in metaverse: a comprehensive survey. Big Data Mining Anal. 6, 234–247. doi: 10.26599/BDMA.2022.9020047
ISO (2011). Systems and Software Engineering – Systems and Software Quality Requirements and Evaluation (SQuaRE) – System and Software Quality Models. Standard, International Organization for Standardization, Geneva.
Jasserand, C. (2018). Subsequent use of GDPR data for a law enforcement purpose: the forgotten principle purpose limitation. Eur. Data Prot. Rev. 4, 152. doi: 10.21552/edpl/2018/2/6
Jindal, D., Kaushik, M., and Bahl, B. (2023). “Emerging trends of privacy and security in cloud computing,” in AIP Conference Proceedings (AIP Publishing). doi: 10.1063/5.0148999
Johnson, R., and Orme, B. (2003). “Getting the most from CBC,” in Sequim: Sawtooth Software Research Paper Series, Sawtooth Software.
Karamanidou, L., Kasparek, B., and Hess, S. (2020). Border management and migration control–comparative report. Zenodo. doi: 10.5281/zenodo.3732864
Kimmelmann, N., Miesera, S., Moser, D., and Pool Maag, S. (2022). “Inclusion for all in vet? a comparative overview of policies and state of research about migration, integration and inclusion in Germany, Austria and Switzerland,” in Migration and Inclusion in Work Life–The Role of VET: Emerging Issues in Research on Vocational Eduction & Training, 117–165.
Krasnova, H., and Veltri, N. F. (2010). “Privacy Calculus on Social Networking Sites: Explorative Evidence from Germany and USA,” in Proceedings of the Annual Hawaii International Conference on System Sciences, 1–10. doi: 10.1109/HICSS.2010.307
Krippendorff, K. (2018). Content Analysis: An Introduction to its Methodology. Sage Publications. doi: 10.4135/9781071878781
Kuckartz, U. (2014). Qualitative Text Analysis: A Guide to Methods, Practice and Using Software. Sage. doi: 10.4135/9781446288719
Li, Y. (2011). Empirical studies on online information privacy concerns: literature review and an integrative framework. Commun. Assoc. Inform. Syst. 28, 453–496. doi: 10.17705/1CAIS.02828
Losavio, M. M., Chow, K., Koltay, A., and James, J. (2018). The internet of things and the smart city: legal challenges with digital forensics, privacy, and security. Sec. Privacy 1, e23. doi: 10.1002/spy2.23
Malheiros, M., Preibusch, S., and Sasse, M. A. (2013). ““Fairly truthful”: the impact of perceived effort, fairness, relevance, and sensitivity on personal data disclosure,” in Trust and Trustworthy Computing: 6th International Conference, TRUST 2013 (Berlin; Heidelberg: Springer Verlag), 250–266.
Markos, E., Milne, G. R., and Peltier, J. W. (2017). Information sensitivity and willingness to provide continua: a comparative privacy study of the United States and Brazil. J. Public Policy Market. 36, 79–96. doi: 10.1509/jppm.15.159
Marturana, F., Me, G., Berte, R., and Tacconi, S. (2011). “A quantitative approach to triaging in mobile forensics,” in 2011IEEE 10th International Conference on Trust, Security and Privacy in Computing and Communications (IEEE), 582–588. doi: 10.1109/TrustCom.2011.75
Metag, J., and Marcinkowski, F. (2014). Technophobia towards emerging technologies? A comparative analysis of the media coverage of nanotechnology in Austria, Switzerland and Germany. Journalism 15, 463–481. doi: 10.1177/1464884913491045
Milne, G. R., Pettinico, G., Hajjat, F. M., and Markos, E. (2017). Information sensitivity typology: mapping the degree and type of risk consumers perceive in personal data sharing. J. Cons. Aff. 51, 133–161. doi: 10.1111/joca.12111
Mothersbaugh, D. L., Foxx, W. K., Beatty, S. E., and Wang, S. (2012). Disclosure antecedents in an online service context: the role of sensitivity of information. J. Serv. Res. 15, 76–98. doi: 10.1177/1094670511424924
Neyer, F. J., Felber, J., and Gebhardt, C. (2012). Entwicklung und validierung einer kurzskala zur erfassung von technikbereitschaft. Diagnostica 58, 87–99. doi: 10.1026/0012-1924/a000067
Nissenbaum, H. (2010). Privacy in Context: Technology, Policy, and the Integrity of Social Life. Stanford, CA: Stanford University Press.
Pfisterer, V. M. (2019). The right to privacy–a fundamental right in search of its identity: uncovering the Cjeu's flawed concept of the right to privacy. German Law J. 20, 722–733. doi: 10.1017/glj.2019.57
Ribble, M. S., Bailey, G. D., and Ross, T. W. (2004). Digital citizenship: addressing appropriate technology behavior. Learn. Lead. Technol. 32, 6.
Rohm, A. J., and Milne, G. R. (2004). Just what the doctor ordered: the role of information sensitivity and trust in reducing medical information privacy concern. J. Bus. Res. 57, 1000–1011. doi: 10.1016/S0148-2963(02)00345-4
Rouvroy, A., and Poullet, Y. (2009). “The right to informational self-determination and the value of self-development: reassessing the importance of privacy for democracy,” in Reinventing Data Protection? (Springer), 45–76. doi: 10.1007/978-1-4020-9498-9_2
Saranya, S., and Usha, G. (2023). “Forensic analysis of online social network data in crime scene investigation,” in Artificial Intelligence and Blockchain in Digital Forensics (River Publishers), 183–209.
Sawtooth Software, Inc. (2017). “The CBC system for choice-based conjoint analysis,” in Sawtooth Software Technical Paper Series, 98382. Available online at: https://sawtoothsoftware.com/resources/technical-papers/cbc-technical-paper, (accessed June 30, 2023).
Schomakers, E.-M., Lidynia, C., Müllmann, D., Matzutt, R., Wehrle, K., Ziefle, M., et al. (2021a). Insights on data sensitivity from the technical, legal and the users' perspectives-practical suggestions on how to raise more awareness for the assumed exercise of informational self-determination. Comput. Law Rev. Int. 22, 8–15. doi: 10.9785/cri-2021-220103
Schomakers, E.-M., Lidynia, C., Müllmann, D., and Ziefle, M. (2019). Internet users' perceptions of information sensitivity–insights from germany. Int. J. Inform. Manage. 46, 142–150. doi: 10.1016/j.ijinfomgt.2018.11.018
Schomakers, E.-M., Lidynia, C., and Ziefle, M. (2020). All of me? Users' preferences for privacy-preserving data markets and the importance of anonymity. Electron. Mark. 30, 649–665. doi: 10.1007/s12525-020-00404-9
Schomakers, E.-M., Lidynia, C., and Ziefle, M. (2021b). The role of privacy in the acceptance of smart technologies: applying the privacy calculus to technology acceptance. Int. J. Hum. Comput. Interact. 38, 1276–1289. doi: 10.1080/10447318.2021.1994211
Schomakers, E.-M., and Ziefle, M. (2019). “Privacy concerns and the acceptance of technologies for aging in place,” in International Conference on Human-Computer Interaction (Orlando, FL: Springer), 313–331.
Selting, M., and Auer, P. (2011). A system for transcribing talk-in-interaction: Gat 2 translated and adapted for english by elizabeth couper-kuhlen and dagmar barth-weingarten. Gesprächsforschung–Online-Zeitschrift zur verbalen Interaktion 12, 1–51.
Sikos, L. F. (2021). AI in digital forensics: ontology engineering for cybercrime investigations. Wiley Interdiscipl. Rev. Forensic Sci. 3, e1394. doi: 10.1002/wfs2.1394
Simsa, R. (2017). Leaving emergency management in the refugee crisis to civil society? The case of Austria. J. Appl. Sec. Res. 12, 78–95. doi: 10.1080/19361610.2017.1228026
Staiano, J., Oliver, N., Lepri, B., de Oliveira, R., Caraviello, M., and Sebe, N. (2014). “Money walks: a human-centric study on the economics of personal mobile data,” in Proceedings of the 2014 ACM International Joint Conference on Pervasive and Ubiquitous Computing (New York, NY), 583–594.
Tene, O., and Polonetsky, J. (2012). Big data for all: privacy and user control in the age of analytics. Nw. J. Tech. Intell. Prop. 11, 27.
Verdecchia, R., Sallou, J., and Cruz, L. (2023). A systematic review of Green <scp>AI </scp>. WIREs Data Mining and Knowledge Discovery. doi: 10.1002/widm.1507
Voigt, P., and Von dem Bussche, A. (2017). “The EU general data protection regulation (GDPR),” in A Practical Guide, 1st edn. (Cham: Springer International Publishing), 10–5555.
Wilkowska, W., Offermann-van Heek, J., Florez-Revuelta, F., and Ziefle, M. (2021). Video cameras for lifelogging at home: preferred visualization modes, acceptance, and privacy perceptions among German and Turkish participants. Int. J. Hum. Comput. Interact. 37, 1436–1454. doi: 10.1080/10447318.2021.1888487
Xu, H., Dinev, T., Smith, H. J., and Hart, P. (2008). “Examining the formation of individual's privacy concerns: toward an integrative view,” in International Conference on Information Systems (Paris), 6.
Keywords: privacy, mobile forensics, conjoint, mixed method, acceptance
Citation: Hildebrandt JR, Schomakers E-M, Ziefle M and Calero Valdez A (2023) Understanding indirect users' privacy concerns in mobile forensics — A mixed method conjoint approach. Front. Comput. Sci. 5:972186. doi: 10.3389/fcomp.2023.972186
Received: 17 June 2022; Accepted: 22 June 2023;
Published: 13 July 2023.
Edited by:
Kostas Karpouzis, Panteion University, GreeceReviewed by:
Ahmad Rabiah, Technical University of Malaysia Malacca, MalaysiaOlga Angelopoulou, University of Warwick, United Kingdom
Copyright © 2023 Hildebrandt, Schomakers, Ziefle and Calero Valdez. This is an open-access article distributed under the terms of the Creative Commons Attribution License (CC BY). The use, distribution or reproduction in other forums is permitted, provided the original author(s) and the copyright owner(s) are credited and that the original publication in this journal is cited, in accordance with accepted academic practice. No use, distribution or reproduction is permitted which does not comply with these terms.
*Correspondence: Julian Romeo Hildebrandt, aGlsZGVicmFuZHRAY29tbS5yd3RoLWFhY2hlbi5kZQ==