- 1Department of Informatics, Bioengineering, Robotics and Systems Engineering (DIBRIS), University of Genoa, Genoa, Italy
- 2Advanced Robotics, Istituto Italiano di Tecnologia, Genoa, Italy
- 3Rehab Technologies Lab, Istituto Italiano di Tecnologia, Genoa, Italy
This paper addresses the need for collecting and labeling affect-related data in ecological settings. Collecting the annotations in the wild is a very challenging task, which, however, is crucial for the creation of datasets and emotion recognition models. We propose a novel solution to collect and annotate such data: a questionnaire based on the appraisal theory, that is accessible through an open-source mobile application. Our approach exploits a commercially available wearable physiological sensor connected to a smartphone. The app detects potentially relevant events from the physiological data, and prompts the users to report their emotions using a novel questionnaire based on the Ortony, Clore, and Collins (OCC) Model. The questionnaire is designed to gather information about the appraisal process concerning the significant event. The app guides a user through the reporting process by posing a series of questions related to the event. As a result, the annotated data can be used, e.g., to develop emotion recognition models. In the paper, we analyze users' reports. To validate the questionnaire, we asked 22 individuals to use the app and the sensor for a week. The analysis of the collected annotations shed new light on self-assessment in terms of appraisals. We compared a proposed method with two commonly used methods for reporting affect-related events: (1) a two-dimensional model of valence and arousal, and (2) a forced-choice list of 22 labels. According to the results, appraisal-based reports largely corresponded to the self-reported values of arousal and valence, but they differed substantially from the labels provided with a forced-choice list. In the latter case, when using the forced-choice list, individuals primarily selected labels of basic emotions such as anger or joy. However, they reported a greater variety of emotional states when using appraisal theory for self-assessment of the same events. Thus, proposed approach aids participants to focus on potential causes of their states, facilitating more precise reporting. We also found that regardless of the reporting mode (mandatory vs. voluntary reporting), the ratio between positive and negative reports remained stable. The paper concludes with a list of guidelines to consider in future data collections using self-assessment.
1 Introduction
Several techniques for emotion recognition from facial expression (Fasel and Luettin, 2003), speech (El Ayadi et al., 2011), full-body motion (Kleinsmith and Bianchi-Berthouze, 2013), tactile gestures (Niewiadomski et al., 2022), physiological signals (Jerritta et al., 2011) and other data sources have been studied intensively for at least two decades. Independently of the chosen method, all of them require creation of appropriate datasets. Therefore, several approaches exist for affect-related data collection and annotation (for recent surveys see Larradet et al. (2020) and Section 2).
Building datasets to train such models is often performed in laboratory setting by purposely inducing emotions to subjects at specific time intervals. This allows experimenters to control the stimuli and reduce the number of contextual factors that may influence the subjects' reactions. Different types of stimuli are used such as sounds, images and videos (Miranda-Correa et al., 2021) but also more complex techniques including virtual reality experience (Chirico et al., 2018; Marín-Morales et al., 2020; Dozio et al., 2022), playing various types of games (Niewiadomski et al., 2013; Bassano et al., 2019), and interaction with social robots (Redondo et al., 2023). To this date, more rare are studies that have attempted to build real-life (not induced) emotions datasets, i.e., collections of affect-related data, outside of the lab, in reaction to every-day events. In the literature, the terms “in the wild” (Dhall et al., 2013), “in the fray” (Healey et al., 2010), and “in real-life” (Devillers et al., 2005) are used to describe approaches where experimenters do not have direct control over the emotion elicitation process. Subjects are typically monitored during their everyday activities over extended periods of time to gather their most natural reactions.
Difficulties in building the affect-related datasets in ecological settings, e.g., establishing the ground truth, are well documented in the literature. Proper data segmentation and labeling is one of the main challenges. Despite all the challenges around their creation, in the wild datasets should be preferred in the light of results showing that humans' expressive behaviors and physiological reactions may differ between naturalistic settings and laboratory environments (Wilhelm and Grossman, 2010; Xu et al., 2017).
This paper proposes a novel method for collecting and labeling affect-related data in ecological settings. Our system is based on a commercially available sensor and a purposefully developed mobile application (app) that is used to collect physiological data and corresponding self-assessments. The app can work in two modalities: in the first modality (called mandatory) the user is prompted to report on potentially affect-related events detected by the app based on the analysis of the physiological signals acquired by the wearable sensor. In the second modality (called voluntary) the user can report affect-related events whenever they find it suitable. To collect the self-reports on affect-related events we designed the questionnaire based on the appraisal theory. We call this method appraisal-based self-assessment questionnaire (ABSAQ) in the remainder of the paper. The users report their subjective evaluations of the significant events, for example, they may state whether the event had positive or negative consequences, or if it was confirmed or not. We believe that using this method may have several advantages over the traditional methods such as self-reports based on (1) dimensional models, and (2) forced-choice lists of labels. The commonly used approach of self-assessment on emotional states is based on dimensional models [e.g., Russell's two-dimensional model of arousal and valence (Russell, 1980)]. Unfortunately, dimensional models do not provide exhaustive information about affective states. For instance, it is often stated that some very different (in terms of elicitation causes) emotional states such as anger and fear are placed very close to each other in a dimensional model (e.g., Russell's model). We expect that when comparing to reports based on dimensional models, our method (ABSAQ) should provide more detailed information about the emotional state of the user and its causes. Thus, in this paper, we investigate (research question 1) whether the reports obtained with the proposed method are consistent with reports based on dimensional models, while also providing more detailed information.
We also compare our method with a traditional approach based on a forced-choice list. By utilizing our method, self-reporting of affective events can be made easier and more efficient, particularly when compared to a method that relies on the forced-choice list with a lengthy list of labels (i.e., more than 6 Ekmanian emotions). This holds especially true when self-reports are collected in the wild. One appraisal theory (e.g., Ortony et al., 1990) can explain more than 20 different emotions. Thus, in the paper, we compare the self-reports obtained with ABSAQ (our proposed method) and a similarly extensive forced-choice list. In particular, in real-life situations, the number and diversity of emotional reactions are undoubtedly broader compared to data collected in-the-lab conditions through the controlled procedure, which often focus on a limited number of specific emotional experiences and utilize a small set of stimuli (such as videos, images, or sounds). Thus, in the wild, it is particularly important that the self-assessment procedure covers a possibly wide range of emotional experiences, which, in the case of a forced-choice list, means creating one long list of emotional labels. Consequently, we expect (research question 2) to observe substantial differences between ABSAQ reports and the self-assessments based on a forced-choice list. Additionally, in-the-lab data collections usually assume that specific stimulus (e.g., an image of a spider) will elicit a specific emotional reaction (e.g., fear). Using ABSAQ, the participants report their subjective evaluations, and thus the same event (i.e., stimulus) may correspond to different emotions. This method can be more suitable to report real-life experiences.
In the paper, we also investigate (research question 3) whether the way of self-reporting (mandatory, voluntary) may influence the quantity of positive and negative emotions reports. The role of positive emotions was widely studied (Fredrickson, 2001). Several intervention programs encourage or facilitate positive emotions awareness and experience (Moskowitz et al., 2021). We expect that participants are more willing to report positive emotions through voluntary reports and “feel obliged” to report negative ones through mandatory reports. In other words, we check whether the reporting method may influence the quantity of reported positive/negative events. Addressing this question is crucial for the development of innovative tools and methods for collecting affect-related data.
Finally, it is important to stress that the focus of this work is on methodological issues related to the (physiological) data collection and self-reporting. We do not provide any new model for emotion classification, nor do we claim that all emotional states considered in this paper can be differentiated in terms of physiological reactions.
The rest of the paper is organized as follows: after presenting the related works in Section 2, we present our mobile app in Section 3, and the dataset in Section 4. The analysis of the self-reports is presented in Section 5 which is followed by the general discussion in Section 6. We describe briefly the physiological signals dataset collected within this study in Section 7 and conclude the paper in Section 8.
2 Related works
2.1 Applications of appraisal theories
Appraisal theories have been largely used in affect-related studies. For instance, Conati and Zhou (2002) implemented a probabilistic model, using Dynamic Decision Networks, to recognize student's emotional states in an educational game context. For this, they followed the OCC appraisal theory (Ortony et al., 1990) and considered the students' goals and personality. In the video game context, Johnstone (1996) analyzed the relation between acoustic features of the player's vocal responses and the manipulations of some appraisals following Scherer's Component Process Model (Scherer, 2009). The same appraisal manipulations are addressed by van Reekum et al. (2004) in the context of a simple video game to study physiological reactions. In Bassano et al. (2019) a Virtual Reality (VR) game and a software platform collecting the player's multimodal data, synchronized with the VR content, are used to build a dataset. The game used was designed according to the emotion elicitation process described by Roseman's appraisal theory. In Meuleman and Rudrauf (2021) the authors used VR consumer games to elicit emotions in participants in-the-lab conditions. They asked participants to self-report appraisal components, physiological reactions, feelings, regulation, action tendencies, as well as emotion labels and dimensions. Using multivariate analyses, they discovered the relation between reported labels and affect components.
2.2 Methods for emotional self-reporting in the wild
According to Scherer (2005), existing techniques for emotional state self-reporting can be divided into two groups: free response and fixed-response labeling. While the first group allows for a higher precision of labeling [custom labels (Isomursu et al., 2007), verbal reports (Muaremi et al., 2013)], it makes it difficult to develop machine learning recognition models due to a potentially wide range of emotion labels selected by users. Constrained solutions include the usage of a finite list of labels (e.g., Nasoz et al., 2004) or dimensional models such as valence-arousal (e.g., Healey et al., 2010) or pleasure-arousal-dominance (e.g., Kocielnik et al., 2013). More user-friendly techniques may be used for reporting such as emoticons (Meschtscherjakov et al., 2009). Affect dimensions are often reported through the Self-Assessment Manikin (SAM) method (Isomursu et al., 2007) or through 2D point maps (Carroll et al., 2013).
In Schmidt et al. (2018), guidelines are provided for emotional labeling in the wild by comparing the results of different methods. A combination of manual reports and automatically triggered prompts is advised, as well as providing the means to the user to manually correct the timespan of an emotional event. Unlike Schmidt et al. (2018), which used time-based trigger, in this study prompting based on physiological cues (Myrtek and Brügner, 1996) was used and an experimenter-free data gathering protocol was implemented.
2.3 Methods for emotional physiological data collection
Emotion recognition from physiological data collected in-the-lab has been studied by different research groups (Shu et al., 2018). Most of the studies use measurements of Heart Rate (HR), Skin Conductance (SC), ElectroDermal Activity (EDA), Galvanic Skin Response (GSR), Skin Temperature (ST), and Respiration. The combinations of several signals, e.g., HR, EDA, and ST, have also been studied (e.g., Nasoz et al., 2004). Studies using data collected in ecological settings are rare, and most of them focus primarily on stress detection (Plarre et al., 2011; Hovsepian et al., 2015; Gjoreski et al., 2017) and moods (Zenonos et al., 2016). In real-life settings, the physiological data labeling and segmentation (i.e., defining the start and end of an emotion) are the main challenge (Healey et al., 2010). A few studies used mobile apps to collect both physiological data and affect-related states. Healey et al. (2010) conducted a real-life experiment using a mobile phone app to study different labeling methodologies for physiological data collection. They collected data and self-reports in the form of discrete labels and dimensional models (valence and arousal) and drew attention to some difficulties linked to self-reporting.
A large number of studies on automatic emotion recognition from physiological signals obtained good recognition rates (Jerritta et al., 2011) but very few of the proposed methods were then tested on data collected in the wild. Wilhelm and Grossman (2010) presented the risks of that approach by comparing physiological signals of in-the-lab induced stress and the ones occurring in ecological settings (e.g., watching a soccer game). They found the heart rate during the latter greatly superior to the former. Similarly, Xu et al. (2017) considered the validity of using in-the-lab collected data for ambulatory emotion detection. Their findings suggested that EDA, ECG, and EMG greatly differ between real-life and laboratory settings and that using such methodology results in low recognition rates (17%–45%). Thus, these results show that it is important to develop methods to build the datasets in the wild.
3 Appraisal theory-based app for data collection and labeling in the wild
We created a new system for physiological data collection and self-reports to satisfy the following requirements:
1. Can be used to capture the data of spontaneous emotions during daily activities;
2. Is minimally intrusive;
3. Guides the user through a process of reporting relevant events, by acquiring the necessary information to infer the related affective states, and without asking the user to pick any emotional label;
4. Guides the user to provide self-assessments by differentiating emotions from moods;
5. Detects the relevant events from the physiological data and prompts the user about it;
6. Provides a limited set of classes or categories of affective experiences that can be used to develop classification models.
The proposed solution consists of a self-assessment questionnaire based on appraisal theory, a commercially available wearable physiological sensor, and, a state-of-the-art event detection algorithm. A mobile application (app) developed ad-hoc allows the user to voluntary report affect-related events as well as report events detected through the physiological data analysis.
3.1 Self-reporting about relevant events
To address the requirements (3), (4), and (6) an questionnaire based on appraisal theory was designed. It serves to acquire the data about the whole appraisal process around the event (see Section 3.3.1 for details on the questionnaire). The questionnaire can be presented in a form of decision tree such that consecutive steps correspond to single appraisals. In this way, instead of a scoring potentially long list of emotion labels (in our experiment we use 22 different states), the participants answer to a set of questions that in most cases are binary (i.e., with answers “yes” or “no”). By collecting information based on such appraisal process, we expected to gather more consistent annotation of corresponding physiological signals.
To address the requirement (5), the app may work in two modalities. In the first modality, by utilizing the existing algorithm proposed by Myrtek and Brügner (1996), the app detects changes (such as additional heart rate) in physiological signals sent in real-time by the sensor. These changes may be related to certain emotional states. When these changes are detected, the app prompts users to provide an evaluation of the event, guiding them through the reporting process.
In the second modality, the user marks significant moments over the day (by pressing the button available on the bracelet of the wearable sensor) and later uses the same questionnaire to annotate the event. Both modalities are available all the time. When the person wears the sensor, the connection is maintained between the app and the sensor.
The reports result in a discrete number of classes (that can be represented by some emotional labels) corresponding to a combination of appraisals. They can, therefore, be used to build emotion classifiers using machine learning techniques. Additionally, the reports provide more information about the event (i.e., details on what led to the emotion). So, they can be potentially used not only for emotion classification but also to train the models that detect single appraisals from physiological data. Such models have rarely been investigated so far (Smith, 1989).
3.2 Sensors
The Empatica E4 bracelet1 allowed us to fulfill requirements 1 and 2. This medical device was chosen for its sensors relevant to emotion detection: BVP, EDA, and ST as well as kinematic data through a 3D accelerometer. Its small size allows for long data collection without being bothersome. The device comes with an API for mobile applications and an already processed BVP to Inter Beat Interval (IBI). The sensor has also been used in the past for research purposes (Gjoreski et al., 2017).
The iPhone-based (iOS) mobile app uses a Bluetooth connection to collect physiological data from the E4 bracelet.
3.3 The application modules
The mobile app (see Figure 2) is composed of three modules.
3.3.1 The self-assessment module
This module is designed to collect information about relevant emotional events. Using this module, the users first provide the duration of a relevant event. The maximum duration was set to 5 min, because emotions are usually short experiences (compared to moods that can also be reported with the same app, see below for details). The user can manually reduce the event duration time (see Figure 2B). Next, they answer a series of questions (see Figures 2C, D) according to the ABSAQ questionnaire. In the last step, they evaluate the emotion intensity.
To create a questionnaire, the Ortony, Clore, and Collins (OCC) model (Ortony et al., 1990) was chosen as it was successfully used in affective computing applications in the past (Bartneck, 2002; Conati, 2002). Additionally, a set of appraisals in the OCC model, and the representation (i.e., decision tree) match our objectives and are easily understandable even by non-experts. The OCC can explain the elicitation of 22 different emotional states that can be triggered by some events, objects, or agents. It is important to notice that in the OCC model, the authors use the concept of emotion groups, which usually contain more than one label.2 For example, the Resentment group (i.e., being displeased about an event presumed to be desirable to someone else) contains labels such as envy, jealousy, and resentment, while the Reproach group (i.e., disapproving someone else's blameworthy actions) contains labels such as appalled, contempt, despise, disdain, indignation, and reproach. In Figure 1 we provide one label for each group.
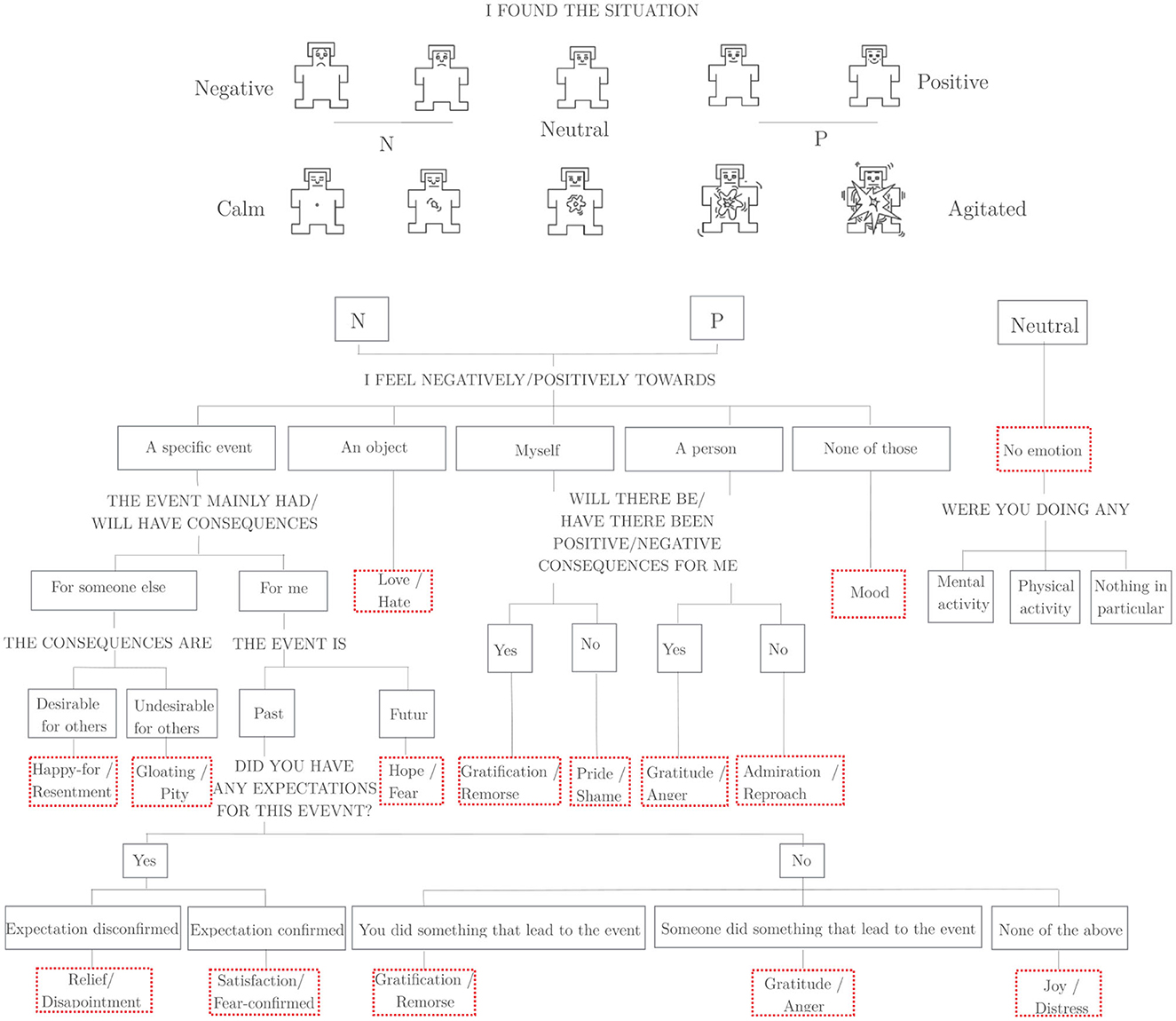
Figure 1. ABSAQ questionnaire. The final labels (emotions) are not displayed to the subjects. Red boxes contain two labels: one positive and one negative (the final label is selected according to the valence value reported at the beginning). The upper part of the Figure is based on Bradley and Lang (1994).
To collect the information about the relevant events, the participants report valence and arousal using five point scale based on SAM Mannekin questionnaire (Bradley and Lang, 1994). Next, the sequence of questions is displayed to the participant, following the structure presented in Figure 1. When designing the questionnaire small changes were introduced in relation to the original model. The main reason was to differentiate mood from emotions. Indeed, according to Clore and Ortony (2013), moods are unconstrained in meaning, while emotions are directed at specific objects, events or people. Therefore, a branch was added to report such “unconstrained in meaning” experiences (see Mood branch in Figure 1).
For instance, the person that is expecting a meeting with their boss, might choose the following sequence. First of all, they might consider their emotional state to be negative (valence) and of high arousal. Their emotional state would be caused by a specific event (here a meeting), which mainly had (or will have) consequences for themself. The event will take place in future. The corresponding emotion group in OCC model is called Fear emotions (and fear is an example of emotion belonging to this group). Thus, physiological signals gathered by the app at that moment would be labeled with the above sequence of appraisals which corresponds to Fear emotion group. Obviously, using our method enables individuals to report their personal evaluations, i.e., the same event (here an incoming meeting) could evoke hope in another individual.
The Valence rating was additionally used to identify “no emotion” reports. If the participant picks Valence = 3 (neutral), they are forwarded to “no emotion” part. This is consistent with OCC theory (Ortony et al., 1990), according to which, emotions are valenced experiences.
3.3.2 The event detection module
This module is used to detect relevant events from the data in real-time. The additional heart rate method (Myrtek and Brügner, 1996) was used to detect relevant events and prompt the user to report their emotion at this time. It consists in detecting heart rate increases that are unrelated to activity (estimated using the accelerometer). Detected events create a mandatory events list, which is always accessible to the user on a separate tab of the app. By implementing this algorithm requirement 5 was fulfilled. The minimum time interval between two mandatory events is fixed at 1 h to avoid the generation of too many related prompts. If two or more events are detected within an hour, only the first event is added to the mandatory list, and the remaining ones are ignored.
3.3.3 The notification module
It reminds the user to wear the device and to report the events from the mandatory event list if any unreported events are left on the list. Reminders are generated every 15 min. after the event. When the connection with the wristband is lost, notifications are generated by phone every 15 s until reconnection.
3.4 The application functionalities
The five tabs are available to the user. Two of them are used to report the events. The first tab called “mandatory” contains a list of automatically detected events for which the reports are not provided yet. When such an event is detected by the event detection module (see Section 3.3.2), a new entry is added to this list (see Figure 2A). Such events are processed according to the procedure described in Section 3.3.1. If there are events on the list, the user receives notification messages (see Figure 2F).
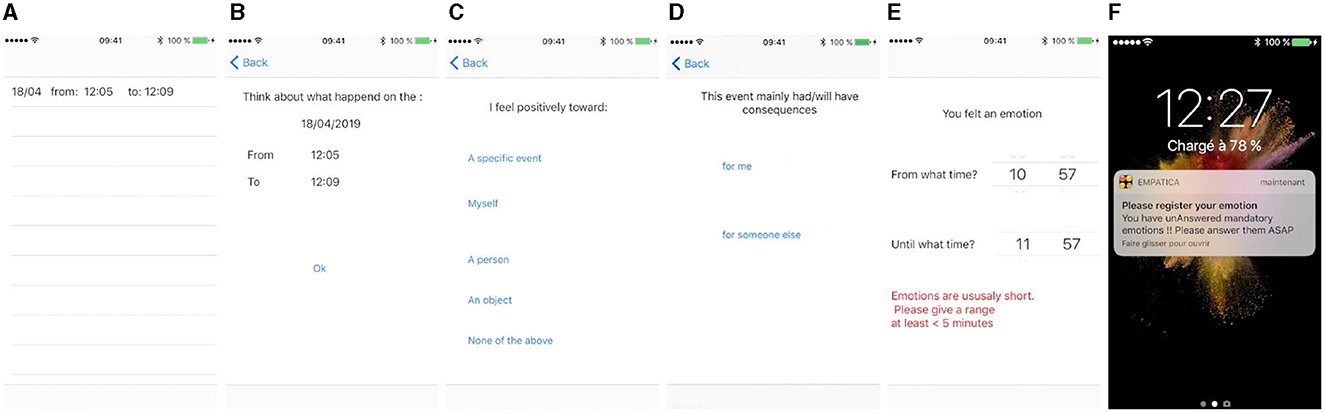
Figure 2. Some screenshots of the app: (A) mandatory tab with an unreported event on the list, (B) first screen of the self-assessment procedure used for mandatory reports, (C, D) reporting questions of ABSAQ report, (E) providing the voluntary report with a warning about the event duration, (F) prompt sent to the user about unreported events.
The users can also voluntarily report an undetected event in two different ways. First, they can use the second tab called “voluntary,” and select manually the start and end time (see Figure 2E) of an event then continue reporting using the emotion definition module). Secondly, the user can also add an event to the list by pressing the bracelet's button. Reporting about the events immediately (i.e., during the emotional experience) might be difficult, especially when it is related to a strong emotional state. By pressing the button, the users manually add a new entry to the list of events. Such an event will have a precise timestamp corresponding to the moment of pressing the button. The participants can report about the event later (as they do for events detected automatically by the app).
The remaining three tabs of the app allow for a better experience with the app. They are used to temporarily stop the notifications, check the battery level and visualize the reports using graphics.
4 Data collection
The main aim of this study is to validate the new self-reporting procedure in the wild. To assess the utility of our ABSAQ questionnaire, we asked people to use our app for a period of 7 days. The participants were said that they would participate in the physiological data collection in the wild, and thus, they should provide self-reports for all the events detected by the app during this period, but also their additional voluntary reports about other events when they find it appropriate. Importantly, participants were unaware of the real aim of this study (i.e., evaluation of the reporting mode). Instead, they were said that their data would be used to develop novel machine learning models for emotion detection and to improve existing algorithms of the app.
The data collection was performed in two stages. In the first stage, participants used the app described in Section 3. In the second stage, another group of participants used a slightly modified version of the app. An additional question was added at the end of reporting procedure to collect also labels (see research question 2). In more detail, after reporting about the event with ABSAQ, the participants were asked to choose one label from the list containing all the labels that are present in the OCC model (22 labels). This was done to check the differences between the two ways of self-reporting.
As mentioned above, we do not claim that it is possible to recognize all 22 emotions from physiological signals (and in particular from the data collected in this study). Given that our participants were unaware of the challenges associated with emotion recognition from physiological signals, it is reasonable to assume that they believed their data would be utilized to develop emotion recognition models capable of detecting a wide range of emotional labels, potentially up to 22 labels.
4.1 Data collection protocol and annotation format
Twenty-two subjects (seven females) participated in this study: 14 in the stage 1, and 8 in the stage 2. Most of the participants were students or researchers in the early stages of their research careers (avg. age 31) of various nationalities, living and working in the same city (in Italy). The data were collected during a week of their ordinary work/internship/study activities. The experimental procedures follow the IIT ADVR TEEP02 protocol, approved by the Ethical Committee of Liguria Region on September 19, 2017. After signing the informed consent, the subjects wore the Empatica E4 wristband for 7 days. During this time they were asked to report their emotions using the mobile application previously described in Section 3.
The collected reports are of three types: Mood, Emotion, or No emotion. All reports contained a start time, an end time, an optional comment, and a sequence of answers in ABSAQ (Figure 1). The Arousal and Valence are integers between 1 and 5.
In the remainder of the paper, the term “inferred labels” will refer to the labels inferred from the path according to ABSAQ, and “reported labels” will refer to the labels directly provided by the participants (in stage 2). Similarly, “inferred arousal” correspond to the arousal inferred from the answers in ABSAQ, and “reported arousal” is the value explicitly reported by the participant (at the beginning of the questionnaire).
4.2 Data confidentiality
During the data collection, physiological data and self-assessments are gathered and stored on the smartphone without being transmitted to any cloud or similar online service. The smartphone is secured with a system password to prevent unauthorized access by other individuals (e.g., in case the participant loses the device).
Upon completion of data collection, only researchers involved in the study can access the stored data on the smartphone by connecting it physically to a computer and utilizing appropriate software. They download the data to an offline storage device and follow the best practices to maintain data pseudo-anonymity.
5 The analysis of reports
5.1 General results
Twenty-two participants used the app and sensor and reported their emotions. Some participants used the app for less than the suggested seven days. We include their data in the following analyses. Overall, the reports were collected over 133 days. In total, 709 reports were collected. This corresponds to 32.2 reports on average per person. The highest number of events reported by a single participant was 66, and the lowest was 12 (standard deviation is 13.87). Four hundred two reports described emotional states, 51 moods, 65 mental activities, 32 sport activities, and the remaining 159 did not correspond to any of the above considered categories. Most of the reports (415) were mandatory (i.e., responses to the events detected by the app). One hundred fifty reports were generated after using the button, and the remaining were reported manually in the app. The total number of reports (including emotions and moods) with a positive valence is 290, while the total number of reports with a negative valence is 163.
Table 1 provides detailed information about the labels that are inferred from the questionnaire. We provide the emotion group as well as, one example of a label from each group. In the remaining of the paper, we refer to the whole group by using this label.
Strong differences can be observed in the frequency of label occurrences: gratification group was the most often chosen group (59 times). The group of negative emotions most frequently chosen was Reproach (44 times). The other two frequent groups were: Gratitude picked 45 times and Hope picked 37 times. On the opposite side, the groups such as Gloating, Joy, and Resentment were chosen very rarely, and Relief was never picked.
There is also a relatively high number of reported events (307 in total) that are not related to emotions. These occurrences may be a result of the introduction of mandatory reports (see Section 5.4 for more details).
After observing strong differences in the choice of emotional categories, we check whether some appraisals are more frequent than others. Therefore, in Table 2, we present the frequency of selecting each appraisal path, without considering the valence of the emotional state associated with the reported event. It can be seen that participants often reported emotions toward other persons. The most frequently chosen path in the questionnaire indicates emotions toward other persons that would not bring (or had brought) any consequences to the reporting person (such as admiration or reproach), while emotions toward others that had or would have some consequences for the reporting person (such as gratitude or anger) were at the fourth place. The second most often chosen path corresponds to the emotions toward self that would bring (or had brought) some consequences to the reporting person (such as gratification or remorse). Next, emotions related to some future events that may not be confirmed (such as hope or fear) were picked 56 times. The other frequent path corresponds to moods (i.e., states not related to any specific person, event not object).
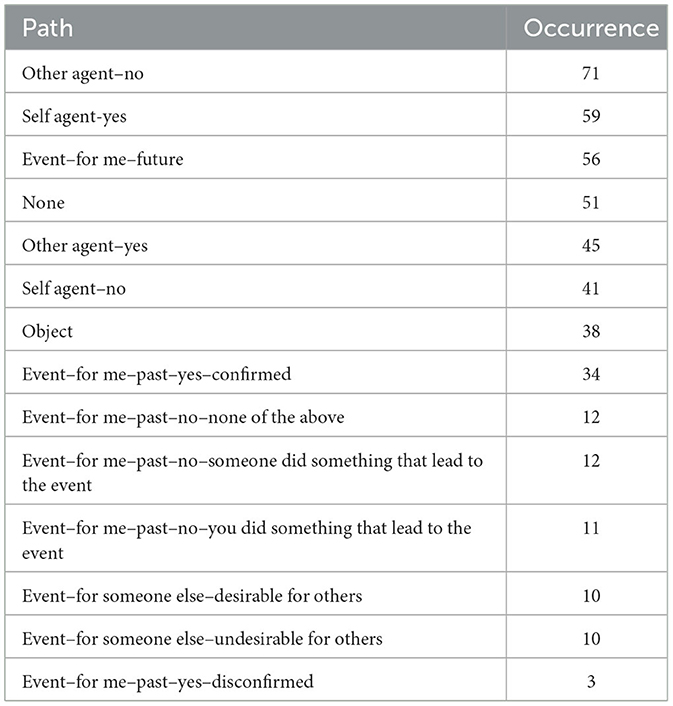
Table 2. The appearance of specific appraisal combinations when merging positive and negative reports (see also Figure 1).
5.2 Dimensional vs. ABSAQ reports
To determine whether the reported arousal and valence align with the answers given in the questionnaire, we calculate the average reported values of arousal and valence for each category. We then compare these values with the arousal and valence values for these labels reported in the literature. For this purpose, we used two works: Whissell (1989) and Hepach et al. (2011) as they consider a huge number of emotional states. In Whissell (1989), the arousal and valence for 107 labels are reported using 7 point scales (from 1 to 7). In Hepach et al. (2011), the arousal and valence for 62 labels are reported using nine point scales.3 Unfortunately, these two publications do not cover all the emotion categories present in the OCC model. The Fear-confirmed, Gloating, Gratification, and Admiration groups are neither present in Whissell (1989) nor in Hepach et al. (2011).
To compare the values, for each emotional category we check all matching labels from an OCC emotion group in Whissell (1989) and Hepach et al. (2011). If more than one label is present then we compute the average value of arousal and valence by taking into consideration all matching labels. At the same time, we also rescale the resulting average values from Whissell (1989) and Hepach et al. (2011) to the range of values used in our experiment (i.e., 1–5). For example, the Disappointment group in OCC model (i.e., being displeased about the disconfirmation of the prospect of a desirable event) contains five labels: dashed-hopes, despair, disappointment, frustration and heartbroken. Two of these labels are present in Whissell (1989): despairing (arousal 4.1, valence 2.0) and disappointed (arousal 5.2, valence 2.4). First, we compute the average values (here: 4.65 and 2.2), and, next, we scale them to the interval [1, 5], obtaining 3.43 for arousal and 1.8 for valence.
In the last step, we compute the 2D distance between the reported average values of arousal and valence, and the average rescaled values of arousal and valence according to Whissell (1989) and Hepach et al. (2011). All the results are in Table 3.
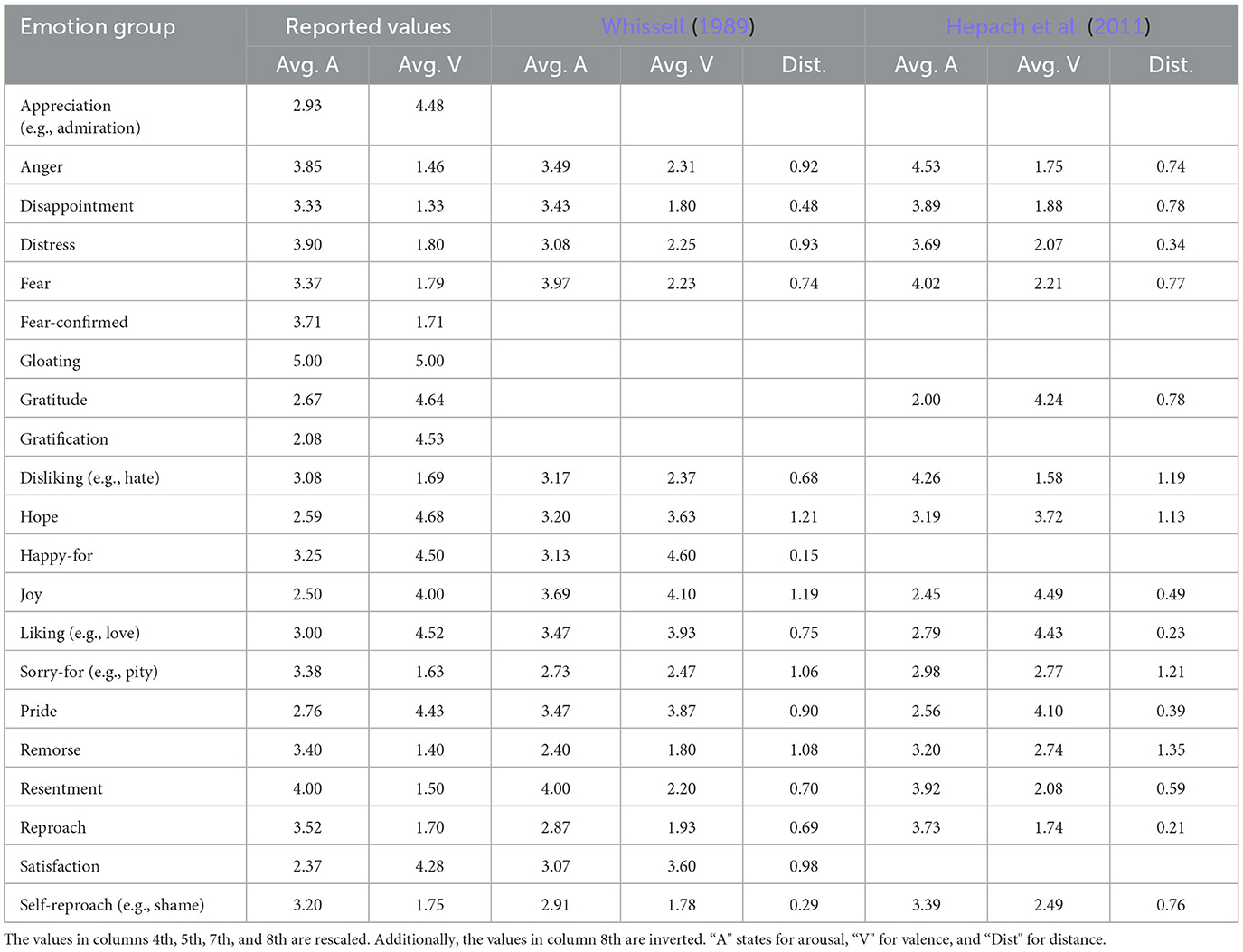
Table 3. The reported average arousal and valence, and comparison to the average values in the literature.
It is important to recall that when the participants choose the valence value (first step of the questionnaire), their choice determines the remaining path in the report. It is not permitted to report the emotional state with valence equal to 3 (i.e., neutral), as such a state is not considered an emotion in the OCC model. In our questionnaire, a valence value of 3 corresponds to physical or mental activity, rather than an emotion.
As it can be seen in Table 3, distances between the average reported arousal and valence, and the values computed from the inferred labels by using values provided by Whissell (1989) and Hepach et al. (2011) are small for most of emotion groups. The average distance is 0.8 when using (Whissell, 1989) and 0.73 when using Hepach et al. (2011). These two values are similar to the average distance between the values reported by Whissell (1989) and Hepach et al. (2011) for the same set of labels, which is 0.74. It let us believe that the reported arousal and valence are in general consistent with the answers given to the second part of the questionnaire (i.e., appraisal-based part). Consequently, below we discuss only a small number of cases for which these results vary the most.
The strongest differences were observed for: (i) the Hope group, for which reports indicate much higher valence but lower arousal than it is reported in the literature, (ii) the Sorry-for Group (e.g., pity), for which reports show higher arousal but lower valence; and (iii) the Remorse Group. At the same time, we also notice differences between the two sources, namely (Whissell, 1989) and Hepach et al. (2011). This is evident in the case of the Joy and Hate groups, for which the reported values in our study are similar to only one of the two sources.
We also look at the difference between arousal reported by our participants and the one that can be inferred from the labels using Whissell (1989). Here relatively strong differences were observed again for Joy and Remorse groups. When comparing the values of reported arousal with Hepach et al. (2011), noticeable differences occur only for the Disliking group (e.g., hate).
It is important to notice that some of these groups have very few instances (e.g., the Joy group, see Table 1) which may explain not optimal results for these groups.
5.3 Forced-choice vs. ABSAQ reports
The Table 4 provides detailed information about the labels explicitly reported by participants and those that are inferred from the ABSAQ.
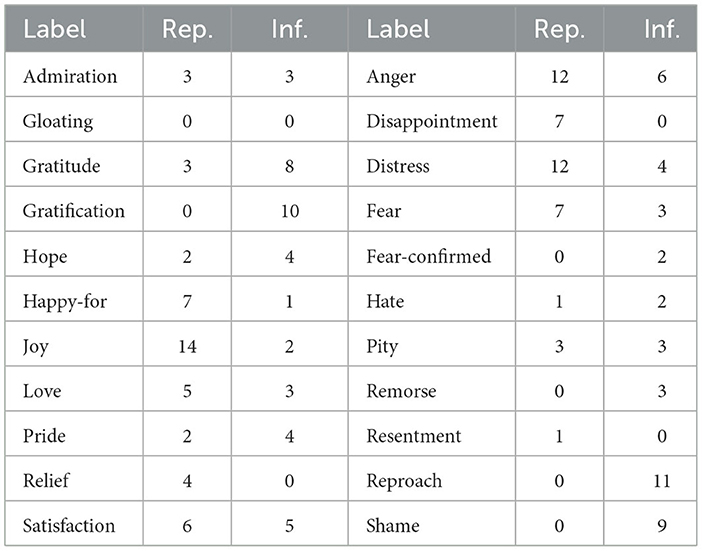
Table 4. Number of reported (rep.) labels directly selected by participants and inferred (inf.) from the questionnaire in stage 2.
Out of the 89 reports considered in stage 2, there were only six instances where the explicitly given label and the label inferred from ABSAQ precisely corresponded. These instances occurred twice for distress and gratitude, and once for joy and satisfaction. Occasionally (three cases) the reports do not match even in terms of valence (i.e., the individuals may have selected a negative emotion while the ABSAQ indicated a positive one, or vice versa).
A detailed analysis of Table 4 shows that participants tended to pick basic or “Ekmanian” labels when providing the reports. Joy was the most often chosen label (14 times). Anger and distress (which can be considered related to sadness) were at the second place (12 times each), and fear was at third place (ex aequo, with happy-for label). However, when considering the ABSAQ, the most frequently chosen appraisal sequences corresponded to negative emotions toward other individuals (e.g., reproach label) and toward oneself (e.g., shame label), as well as positive emotions toward oneself with positive consequences (gratification). It is interesting to see that the labels: reproach, shame, and gratification were not picked even once from the forced-choice list. In general, the labels inferred from ABSAQ are better distributed (standard deviation 3.12) compared to forced-choice list reports (standard deviation 4.18).
When analyzing disagreements between reported emotions and inferred labels, certain patterns were observed to be more frequent than others. For positive emotions, the reported label of happy-for often coincided with the Gratitude group, while the reported joy aligned with the Pride and Gratification groups. For negative emotions, the reported label of anger frequently coincided with the Reproach group.
5.4 Mandatory vs. voluntary reports
Additionally, the relation between valence and the mandatory or voluntary character of the report was calculated. Table 5 presents the number of positive, negative, mandatory and voluntary reports.
Contrary to our expectations, the participants reported negative emotions with equal frequency in both reporting modalities: when prompted to do so and when reporting voluntarily. The percentage of mandatory positive reports is 63.2% of all mandatory reports, while the percentage of voluntary positive reports is 62.5% of all voluntary reports.
The relatively high contribution of the voluntary reports was observed for the emotion groups, for which, at least according to the current state-of-the-art, automatic detection from the physiological data is particularly challenging or even impossible: such as Gratitude, Gratification, Reproach, and Admiration. Probably the simple algorithm used to detect significant events (see Section 3.3.2) is not suitable, and, consequently, these emotions were relatively more often reported voluntarily.
5.5 Auxiliary analyses
The mobile application was programmed in such a way that it was possible to identify when subjects changed their mind when reporting their emotional state. For instance, one may select “A specific event,” then, once the next question is displayed, go back and “A person” instead. We notice that 32 times participants changed their opinion when reporting.
Additionally, participants were able to add comments when they desired. Such disclosure of personal information was made optional in order to respect the subjects' privacy. In total, only 37 such reports were collected. These comments associated with the inferred label give additional information about the reporting process. Most of the optional comments given by the participants seem to fit the self-assignments, a “software crash” (ID 1) is likely to induce “distress,” and “a meeting” to induce fear (ID 3). For instance, the comment “itchy annoying mosquito bite” (ID 4) is interesting. In this case, the participant's emotion appears to be directed toward the reason for the pain, that is the mosquito, as the subject selected the sequence “I feel negatively toward A person,” resulting in the inferred label of anger. This highlights the benefits of utilizing appraisal theory for self-assessment.
6 Discussion
In stages 1 and 2, we observed that the reported arousal and valence values do not differ substantially from the values found in the literature corresponding to inferred labels. However, only a very small number of explicitly provided labels in stage 2 correspond to inferred labels. Thus, both results confirm our expectations (research question 1 and 2). Our structured approach allows the user to describe their emotional states by offering a set of relatively simple questions with a limited number of options. In the stage 2, the most often reported emotion is joy, which is considered a generic description of a positive experience. Our belief is that when seeing a list of 22 labels the participants choose the well-known label without considering the subtle differences between different positive states such as gratitude and gratification. A completely different situation arises when considering the ABSAQ questionnaire and inferred labels. In this case, the two most often reported states are gratitude and gratification. A similar preference toward the popular (i.e., basic, Ekmanian) labels is observed when analyzing the negative emotions. In this case, anger is the most frequently reported label (on a pair with distress). The same tendency is observed in the entire dataset: positive emotions toward self or other people with positive consequences (Gratitude and Gratification) are the most frequently inferred emotion groups, along with negative emotions toward others with no consequences (Reproach group). This result shows that there might be a bias toward selecting more well-known emotional labels (such as basic emotions) when they are explicitly listed. This should be taken into consideration in future studies when using a self-assessment procedure. We believe that our approach helps the participants to focus on the possible causes, and in consequence, to report more precisely about their affective state.
Surprisingly, we discovered that there is no difference in terms of positive and negative reports when comes to the modality of reporting (research question 3). Our expectations were not confirmed as in both modalities (voluntary and mandatory) a similar ratio of negative and positive reports were observed. This contradicts our hypothesis that participants would be more eager to voluntarily report positive emotions than negative ones. We did not observe differences in valence ratio between two reporting modalities, and both of them have some important advantages. The mandatory reports were rated as emotions 56% of the time and 78% of the emotional reports were mandatory. On one hand, it means that nearly one quarter of the emotional events would not be annotated, if the voluntary modality was not available. On the other hand, 88% of the mood labels were picked in mandatory modality, which shows that current approach to detect the emotional states is not optimal. Thus, we recommend the researchers to use both modalities (i.e., mandatory and voluntary) in the future data collection studies.
Our study brings several additional interesting observations. First, it can be noticed that a low number of emotional reports per day was collected (three emotions per day were reported in average, and 5.32 reports per day in total, that is, including other activities). The number of reports per day is clearly lower when compared to previous works, e.g., Trampe et al. (2015). In Trampe et al. (2015), the app prompts randomly the user a fixed number of times per day (the number of daily questionnaire requests is preset). Such an approach can result in situations when the app prompts the user to report something, even if they do not experience anything that would be worth reporting. In Trampe et al. (2015) even 90% of reports indicate an emotional experience, and the authors comment on this result stating that “People's everyday life seems profoundly emotional.” In our approach, several mandatory reports (i.e., prompted by the app) result in non-emotional experience (e.g., mental activity). In total 36% of the reports concern non-emotional experiences. Thus, we recommend that other researchers also consider introducing the possibility for participants to report on activities unrelated to emotions, even in data collections in-the-wild focusing on emotional states.
Second, some emotions groups are chosen more frequently than others (Figure 3) with Gratification counting a total of 59 reports while groups such as Gloating and Resentment counting only two reports. At first sight, it might be surprising that some well-known labels such as joy are rarely present in the dataset. On the other hand, it has to be acknowledged that in this study we distinguished a high number of positive emotions, compared to other studies. In total, 11 different positive emotional states are considered (while in several other studies, all positive states are covered with one generic label of joy). This result confirms the necessity of a more fine-grained analysis of positive emotional states also in future studies. Adding the possibility to report a variety of positive emotions provides interesting information. The most commonly chosen group, i.e., the Gratification group, encompasses positive states that are directed toward oneself and are associated with having or expecting positive consequences for the reporting person. This result could potentially be attributed to the fact that the majority of our participants were students or researchers in the early stages of their research careers. It is possible that individuals belonging to such a group often experience positive emotions related to personal achievements. We also notice differences in the label frequency between our study and (Trampe et al., 2015). In Trampe et al. (2015) the most frequently reported emotions were joy, followed by love, anxiety, and satisfaction.
In general, positive emotions are reported more often than negative ones. At the same time, it should be noticed that the ratio between reported positive and negative emotions in our study is 1.7, which is far from the postulated relation 3:1 (Fredrickson and Losada, 2005), and also lower than the ratio observed in other studies, e.g., 2.59 in Trampe et al. (2015). The disparity can be observed between participants (see Figure 4) with four individuals who reported more negative than positive states, and one participant (ID12) whose nearly 80% of reports was positive. This result, however, may also be influenced by the specific demographics and occupational situation of the participants.
We also observed that some individuals reported a relatively low number of emotions, such as subject 5 who reported only 11 emotional labels over the course of one week, while others reported a higher number, such as subject 7 who reported 48 emotions (see Figure 5). The observed disparity in the reports could be influenced by differences in the number of emotional stimuli encountered by the individuals during their participation in the experiment. It is, however, known that individuals may vary in their level of emotional awareness, with some being more attuned to their emotions than others (Myrtek et al., 2005). Based on these findings, we recommend to vary the duration of data collection time with respect to this factor, and permit some participants to use the app longer than others in future works.
7 Open dataset
The dataset collected during the experiment described in the previous Section is freely available and can be used by researchers to unravel the challenges of emotion detection in the wild. The data annotation consists of both appraisals and corresponding emotional labels. The dataset includes emotional states that are rarely considered in other publicly available physiological datasets. All the data was collected with the E4 wristband.
The physiological signals had the following frame rates: GSR—4 data points per second, BVP—64 data points per second, ST—4 data points per second, ACC—32 data points per second, IBI—Calculated from BVP, one data point for each BVP peak. No signal post-processing or filtering was applied to the data.
Both the app code (iPhone) and the physiological data gathered during the experiment are freely available at https://gitlab.com/flarradet/epsdi.
8 Conclusions and future works
In this paper, a new tool was proposed to collect physiological signals and self-assessments in ecological settings. Our solution inspired by appraisal theories, allows users to self-report the appraisal process around relevant events. The reports can be of two types: voluntary and requested by the app (when a substantial change in the physiological data is detected). We also performed the data collection with 22 participants who used the app for 133 days in total.
The proposed data collection methodology proven to be effective in gathering self-assessment on affect-based events in the wild. Self-assessments obtained through our technique are consistent with the reported valence and arousal, but they differ substantially from self-assessments provided with a forced-choice list. We observed that regardless of the reporting mode (mandatory vs. voluntary reporting), the ratio between positive and negative reports is stable. Last but not least, when using a forced-list choice the participants have a tendency to pick labels of basic emotions (e.g., anger, joy), this is not the case for the ABSAQ questionnaire which additionally provides insights into the causes of emotional states.
To our knowledge, this is the first app for data collection and self-assessment based on appraisal theory. It can be used by other researchers, e.g., to extend the dataset. The dataset might be used in the future, e.g., to train specific classifiers, by choosing the relevant subset of the appraisals and emotional labels.
The additional advantage of using appraisal theory for reporting is that the information about the appraisal process can be used to develop models for single appraisals (and not emotional labels). For instance, one can use physiological data to train detection models, focusing on whether an event is confirmed (or not), or whether it is (un)expected (see Mortillaro et al., 2012). It is in line with Scherer's Component Process Model (Scherer, 2009) according to which the behavioral changes correspond to specific appraisals rather than emotional labels. To sum up, our approach allows the researchers to develop two different types of models (for emotions and appraisals).
Some limitations of this work should be mentioned. First of all, we do not claim that it is possible to detect 22 different emotions by using existing sensors (and physiological signals in general). Building the recognition model is out of the scope of this work. Secondly, taking into account the number of participants, and time of recordings, the number of reported events is surprisingly low. Some technical issues revealed during the data collection (e.g., device disconnection) may hand a limited impact on it. Similarly, the event detection algorithm used by the app is rather simple and the event detection should be improved by training appropriate machine learning models, for instance, on the dataset collected in this experiment. Third, this study uses one appraisal theory only. It is envisaged to explore other theories (e.g., Roseman et al., 1996) in future works.
Future work will focus on additional evaluations of the ABSAQ questionnaire. While our main aim is to introduce a new self-assessment method in the wild, we also believe that we need to perform more controlled experiments. The different methods of self-assessment can be compared when events (stimuli) that potentially may elicit emotional states are experimentally controlled (e.g., in the virtual environment, see a preliminary work by Bassano et al., 2019). This would allow us to compare the reports provided by different participants about the specific events. The study presented in the paper was conducted on a homogeneous group of young adults living in Western culture during a week of their ordinary work activity. We recommend replicating the study on different populations. While the main results (i.e., three main research questions) are not likely to be strongly affected by demographic factors, these factors as well as, the main activity or lifestyle might influence some secondary results such as the ratio of reported positive and negative emotions. Some individual's characteristics, such as the ability to correctly interpret one's own emotional states or neurodivergence, might also have a certain impact on results and thus should be studied more thoroughly in the future.
Several applications of this work are envisaged. First of all, the work is part of project TEEP-SLA, which aims at automatically detecting emotions from physiological signals for Amyotrophic Lateral Sclerosis (ALS) patients. The long-term aim is to create a large dataset to be used in emotion recognition from physiological data collected in natural settings. Moreover, people are already willing to report their emotions on mobile apps for the sole purpose of self-monitoring. As wearable sensors become more popular, our approach may enable large data collections, and boost the research on affect recognition. While in this study, the app and ABSAQ are used to collect the physiological data and self-assessments, we believe that the same methodology can be used to collect self-reports for other data sources, e.g., audio data. Consequently, a large number of affective computing applications may benefit from the more accurate and efficient tools for data collection and labeling in the wild.
Data availability statement
The datasets presented in this study can be found in online repositories. The names of the repository/repositories and accession number(s) can be found at: https://gitlab.com/flarradet/epsdi.
Ethics statement
IIT ADVR TEEP02 protocol approved by the Ethical Committee of Liguria Region. The studies were conducted in accordance with the local legislation and institutional requirements. The participants provided their written informed consent to participate in this study.
Author contributions
RN: Conceptualization, Data curation, Formal analysis, Investigation, Methodology, Visualization, Writing—original draft, Writing— review & editing. FL: Data curation, Formal analysis, Investigation, Methodology, Software, Visualization, Writing—original draft. GB: Writing—original draft, Writing—review & editing, Methodology. LM: Resources, Supervision, Validation, Writing—original draft, Writing—review & editing, Funding acquisition, Project administration.
Funding
The author(s) declare financial support was received for the research, authorship, and/or publication of this article. This work was partially supported by Fondazione Roma as part of the project TEEP-SLA.
Conflict of interest
The authors declare that the research was conducted in the absence of any commercial or financial relationships that could be construed as a potential conflict of interest.
The author(s) declared that they were an editorial board member of Frontiers, at the time of submission. This had no impact on the peer review process and the final decision.
Publisher's note
All claims expressed in this article are solely those of the authors and do not necessarily represent those of their affiliated organizations, or those of the publisher, the editors and the reviewers. Any product that may be evaluated in this article, or claim that may be made by its manufacturer, is not guaranteed or endorsed by the publisher.
Footnotes
1. ^https://www.empatica.com/en-eu/research/e4/ (accessed 4th September 2019).
2. ^In the remainder of the paper, we use a capital letter, when we refer to an emotion group, e.g., Reproach group.
3. ^The meaning of the valence scale is reversed in Hepach et al. (2011), i.e., it starts “very positive” and it ends at “very negative.”
References
Bartneck, C. (2002). “Integrating the OCC model of emotions in embodied characters,” in Workshop on Virtual Conversational Characters (Princeton, NJ: Citeseer), 39–48.
Bassano, C., Ballestin, G., Ceccaldi, E., Larradet, F. I., Mancini, M., Volta, E., et al. (2019). “A VR game-based system for multimodal emotion data collection,” in Motion, Interaction and Games (New York, NY: ACM), 38. doi: 10.1145/3359566.3364695
Bradley, M. M., and Lang, P. J. (1994). Measuring emotion: the self-assessment manikin and the semantic differential. J. Behav. Ther. Exp. Psychiatry 25, 49–59. doi: 10.1016/0005-7916(94)90063-9
Carroll, E. A., Czerwinski, M., Roseway, A., Kapoor, A., Johns, P., Rowan, K., et al. (2013). “Food and mood: just-in-time support for emotional eating,” in 2013 Humaine Association Conference on Affective Computing and Intelligent Interaction (IEEE), 252–257. doi: 10.1109/ACII.2013.48
Chirico, A., Ferrise, F., Cordella, L., and Gaggioli, A. (2018). Designing awe in virtual reality: an experimental study. Front. Psychol. 8, 2351. doi: 10.3389/fpsyg.2017.02351
Clore, G. L., and Ortony, A. (2013). Psychological construction in the OCC model of emotion. Emot. Rev. 5, 335–343. doi: 10.1177/1754073913489751
Conati, C. (2002). Probabilistic assessment of user's emotions in educational games. Appl. Artif. Intell. 16, 555–575. doi: 10.1080/08839510290030390
Conati, C., and Zhou, X. (2002). “Modeling students' emotions from cognitive appraisal in educational games,” in Intelligent Tutoring Systems, eds S. A. Cerri, G. Gouardères, and F. Paraguaçu (Berlin: Springer Berlin Heidelberg), 944–954. doi: 10.1007/3-540-47987-2_94
Devillers, L., Vidrascu, L., and Lamel, L. (2005). Challenges in real-life emotion annotation and machine learning based detection. Neural Netw. 18, 407–422. doi: 10.1016/j.neunet.2005.03.007
Dhall, A., Goecke, R., Joshi, J., Wagner, M., and Gedeon, T. (2013). “Emotion recognition in the wild challenge 2013,” in Proceedings of the 15th ACM on International Conference on Multimodal Interaction (New York, NY: ACM), 509–516. doi: 10.1145/2522848.2531739
Dozio, N., Marcolin, F., Scurati, G. W., Ulrich, L., Nonis, F., Vezzetti, E., et al. (2022). A design methodology for affective virtual reality. Int. J. Hum. Comput. Stud. 162, 102791. doi: 10.1016/j.ijhcs.2022.102791
El Ayadi, M., Kamel, M. S., and Karray, F. (2011). Survey on speech emotion recognition: features, classification schemes, and databases. Pattern Recognit. 44, 572–587. doi: 10.1016/j.patcog.2010.09.020
Fasel, B., and Luettin, J. (2003). Automatic facial expression analysis: a survey. Pattern Recognit. 36, 259–275. doi: 10.1016/S0031-3203(02)00052-3
Fredrickson, B. (2001). The role of positive emotions in positive psychology. the broaden-and-build theory of positive emotions. Am. Psychol. 56, 218–226. doi: 10.1037/0003-066X.56.3.218
Fredrickson, B., and Losada, M. (2005). The positive affect and the complex dynamics of human flourishing. Am. Psychol. 60, 678–86. doi: 10.1037/0003-066X.60.7.678
Gjoreski, M., Luštrek, M., Gams, M., and Gjoreski, H. (2017). Monitoring stress with a wrist device using context. J. Biomed. Inform. 73, 159–170. doi: 10.1016/j.jbi.2017.08.006
Healey, J., Nachman, L., Subramanian, S., Shahabdeen, J., and Morris, M. (2010). “Out of the lab and into the fray: towards modeling emotion in everyday life,” in International Conference on Pervasive Computing (Cham: Springer), 156–173. doi: 10.1007/978-3-642-12654-3_10
Hepach, R., Kliemann, D., Gruneisen, S., Heekeren, H., and Dziobek, I. (2011). Conceptualizing emotions along the dimensions of valence, arousal, and communicative frequency – implications for social-cognitive tests and training tools. Front. Psychol. 2, 266. doi: 10.3389/fpsyg.2011.00266
Hovsepian, K., al'Absi, M., Ertin, E., Kamarck, T., Nakajima, M., and Kumar, S. (2015). “cstress: towards a gold standard for continuous stress assessment in the mobile environment,” in Proceedings of the 2015 ACM International Joint Conference on Pervasive and Ubiquitous Computing (New York, NY: ACM), 493–504. doi: 10.1145/2750858.2807526
Isomursu, M., Tähti, M., Väinämö, S., and Kuutti, K. (2007). Experimental evaluation of five methods for collecting emotions in field settings with mobile applications. Int. J. Hum. Comput. Stud. 65, 404–418. doi: 10.1016/j.ijhcs.2006.11.007
Jerritta, S., Murugappan, M., Nagarajan, R., and Wan, K. (2011). “Physiological signals based human emotion recognition: a review,” in 2011 IEEE 7th International Colloquium on Signal Processing and its Applications (Penang: IEEE), 410–415. doi: 10.1109/CSPA.2011.5759912
Johnstone, T. (1996). “Emotional speech elicited using computer games,” in Proceeding of Fourth International Conference on Spoken Language Processing. ICSLP'96, Vol. 3 (IEEE), 1985–1988. doi: 10.1109/ICSLP.1996.608026
Kleinsmith, A., and Bianchi-Berthouze, N. (2013). Affective body expression perception and recognition: a survey. IEEE Trans. Affect. Comput. 4, 15–33. doi: 10.1109/T-AFFC.2012.16
Kocielnik, R., Sidorova, N., Maggi, F. M., Ouwerkerk, M., and Westerink, J. H. (2013). “Smart technologies for long-term stress monitoring at work,” in Proceedings of the 26th IEEE International Symposium on Computer-Based Medical Systems (Porto: IEEE), 53–58. doi: 10.1109/CBMS.2013.6627764
Larradet, F., Niewiadomski, R., Barresi, G., Caldwell, D. G., and Mattos, L. S. (2020). Toward emotion recognition from physiological signals in the wild: approaching the methodological issues in real-life data collection. Front. Psychol. 11, 1111. doi: 10.3389/fpsyg.2020.01111
Marín-Morales, J., Llinares, C., Guixeres, J., and Alcañiz, M. (2020). Emotion recognition in immersive virtual reality: from statistics to affective computing. Sensors 20, 5163. doi: 10.3390/s20185163
Meschtscherjakov, A., Weiss, A., and Scherndl, T. (2009). Utilizing emoticons on mobile devices within esm studies to measure emotions in the field. Proc. MME Conjunct. MobileHCI 9, 3361–3366.
Meuleman, B., and Rudrauf, D. (2021). Induction and profiling of strong multi-componential emotions in virtual reality. IEEE Trans. Affect. Comput. 12, 189–202. doi: 10.1109/TAFFC.2018.2864730
Miranda-Correa, J. A., Abadi, M. K., Sebe, N., and Patras, I. (2021). Amigos: a dataset for affect, personality and mood research on individuals and groups. IEEE Trans. Affect. Comput. 12, 479–493. doi: 10.1109/TAFFC.2018.2884461
Mortillaro, M., Meuleman, B., and Scherer, K. R. (2012). Advocating a componential appraisal model to guide emotion recognition. Int. J. Synth. Emot. 3, 18–32. doi: 10.4018/jse.2012010102
Moskowitz, J. T., Cheung, E. O., Freedman, M., Fernando, C., Zhang, M. W., Huffman, J. C., et al. (2021). Measuring positive emotion outcomes in positive psychology interventions: a literature review. Emot. Rev. 13, 60–73. doi: 10.1177/1754073920950811
Muaremi, A., Arnrich, B., and Tröster, G. (2013). Towards measuring stress with smartphones and wearable devices during workday and sleep. Bionanoscience 3, 172–183. doi: 10.1007/s12668-013-0089-2
Myrtek, M., Aschenbrenner, E., and Brügner, G. Psychophysiological Research Group at the University of Freiburg (FRG) (2005). Emotions in everyday life: an ambulatory monitoring study with female students. Biol. Psychol. 68, 237–255. doi: 10.1016/j.biopsycho.2004.06.001
Myrtek, M., and Brügner, G. (1996). Perception of emotions in everyday life: studies with patients and normals. Biol. Psychol. 42, 147–164. doi: 10.1016/0301-0511(95)05152-X
Nasoz, F., Alvarez, K., Lisetti, C. L., and Finkelstein, N. (2004). Emotion recognition from physiological signals using wireless sensors for presence technologies. Cogn. Technol. Work 6, 4–14. doi: 10.1007/s10111-003-0143-x
Niewiadomski, R., Beyan, C., and Sciutti, A. (2022). Affect recognition in hand-object interaction using object-sensed tactile and kinematic data. IEEE Trans. Haptics 16, 112–117. doi: 10.1109/TOH.2022.3230643
Niewiadomski, R., Mancini, M., Baur, T., Varni, G., Griffin, H., Aung, M., et al. (2013). “MMLI: multimodal multiperson corpus of laughter in interaction,” in Human Behavior Understanding, Volume 8212 of Lecture Notes in Computer Science, A. Salah, H. Hung, O. Aran, and H. Gunes(Cham: Springer International Publishing), 184–195. doi: 10.1007/978-3-319-02714-2_16
Ortony, A., Clore, G. L., and Collins, A. (1990). The Cognitive Structure of Emotions. Cambridge: Cambridge University Press.
Plarre, K., Raij, A., Hossain, S. M., Ali, A. A., Nakajima, M., Al'Absi, M., et al. (2011). “Continuous inference of psychological stress from sensory measurements collected in the natural environment,” in Proceedings of the 10th ACM/IEEE International Conference on Information Processing in Sensor Networks (IEEE), 97–108.
Redondo, M. E. L., Niewiadomski, R., Rea, F., Incao, S., Sandini, G., Sciutti, A., et al. (2023). Comfortability analysis under a human–robot interaction perspective. Int. J. Soc. Robot. doi: 10.1007/s12369-023-01026-9
Roseman, I. J., Antoniou, A. A., and Jose, P. E. (1996). Appraisal determinants of emotions: Constructing a more accurate and comprehensive theory. Cogn. Emot. 10, 241–278. doi: 10.1080/026999396380240
Russell, J. A. (1980). A circumplex model of affect. J. Pers. Soc. Psychol. 39, 1161. doi: 10.1037/h0077714
Scherer, K. R. (2005). What are emotions? and how can they be measured? Soc. Sci. Inf. 44, 695–729. doi: 10.1177/0539018405058216
Scherer, K. R. (2009). The dynamic architecture of emotion: evidence for the component process model. Cogn. Emot. 23, 1307–1351. doi: 10.1080/02699930902928969
Schmidt, P., Reiss, A., Dürichen, R., and Van Laerhoven, K. (2018). “Labelling affective states in the wild: Practical guidelines and lessons learned,” in Proceedings of the 2018 ACM International Joint Conference and 2018 International Symposium on Pervasive and Ubiquitous Computing and Wearable Computers (New York, NY: ACM), 654–659. doi: 10.1145/3267305.3267551
Shu, L., Xie, J., Yang, M., Li, Z., Li, Z., Liao, D., et al. (2018). A review of emotion recognition using physiological signals. Sensors 18, 1–41. doi: 10.3390/s18072074
Smith, C. (1989). Dimensions of appraisal and physiological response in emotion. J. Pers. Soc. Psychol. 339–353. doi: 10.1037/0022-3514.56.3.339
Trampe, D., Quoidbach, J., and Taquet, M. (2015). Emotions in everyday life. PLoS ONE 10, 1–15. doi: 10.1371/journal.pone.0145450
van Reekum, C., Johnstone, T., Banse, R., Etter, A., Wehrle, T., Scherer, K., et al. (2004). Psychophysiological responses to appraisal dimensions in a computer game. Cogn. Emot. 18, 663–688. doi: 10.1080/02699930341000167
Whissell, C. M. (1989). “Chapter 5 - The dictionary of affect in language,” in The Measurement of Emotions, eds R. Plutchik, and H. Kellerman (Cambridge:Academic Press), 113–131. doi: 10.1016/B978-0-12-558704-4.50011-6
Wilhelm, F. H., and Grossman, P. (2010). Emotions beyond the laboratory: theoretical fundaments, study design, and analytic strategies for advanced ambulatory assessment. Biol. Psychol. 84, 552–569. doi: 10.1016/j.biopsycho.2010.01.017
Xu, Y., Hübener, I., Seipp, A.-K., Ohly, S., and David, K. (2017). “From the lab to the real-world: an investigation on the influence of human movement on emotion recognition using physiological signals,” in 2017 IEEE International Conference on Pervasive Computing and Communications Workshops (PerCom Workshops) (IEEE), 345–350.
Zenonos, A., Khan, A., Kalogridis, G., Vatsikas, S., Lewis, T., Sooriyabandara, M., et al. (2016). “Healthyoffice: mood recognition at work using smartphones and wearable sensors,” in 2016 IEEE International Conference on Pervasive Computing and Communication Workshops (PerCom Workshops) (Sydney, NSW: IEEE), 1–6. doi: 10.1109/PERCOMW.2016.7457166
Keywords: emotion recognition, appraisal theories, data collection, self-assessment, physiological data, ecological setting, basic emotions
Citation: Niewiadomski R, Larradet F, Barresi G and Mattos LS (2024) Self-assessment of affect-related events for physiological data collection in the wild based on appraisal theories. Front. Comput. Sci. 5:1285690. doi: 10.3389/fcomp.2023.1285690
Received: 30 August 2023; Accepted: 18 December 2023;
Published: 11 January 2024.
Edited by:
Alessia Celeghin, University of Turin, ItalyReviewed by:
Marco Viola, University Institute of Higher Studies in Pavia, ItalyJoan Cruz, Escuela Colombiana de Ingenieria Julio Garavito, Colombia
Copyright © 2024 Niewiadomski, Larradet, Barresi and Mattos. This is an open-access article distributed under the terms of the Creative Commons Attribution License (CC BY). The use, distribution or reproduction in other forums is permitted, provided the original author(s) and the copyright owner(s) are credited and that the original publication in this journal is cited, in accordance with accepted academic practice. No use, distribution or reproduction is permitted which does not comply with these terms.
*Correspondence: Radoslaw Niewiadomski, cmFkb3NsYXcubmlld2lhZG9tc2tpQHVuaWdlLml0