- Department of Informatics, Umeå University, Umeå, Sweden
Personal health and wellbeing have grown as an object of study within HCI research and many individuals undergoing weight loss journeys use persuasive systems, such as weight loss apps, to guide them. These systems rely on various principles to persuade users toward their health goal and have in much research been considered having positive effects. There are however also parallel findings indicating unwanted effects. To explore the effects of persuasive system design, we conduct a longitudinal exploratory expert evaluation of 10 free weight loss tracking apps by emulating drastic weight loss for an extended period of time. The objective of the exploration is to unravel potential negative effects of using such systems. Our findings highlight risks of negative effects as some apps take a passive stance toward drastic weight loss, while others actively encourage it through design. We contribute to a growing strand of health related HCI research by mapping out the relation between design and negative consequences of persuasive system use.
1. Introduction
Persuasive systems have grown in popularity and persuasive principles are commonly found on websites, social media, games, and apps. These systems, purposefully designed to motivate a behavior (often referred to as target behavior), have proven successful across a range of studies (Webb et al., 2010; Cugelman et al., 2011; Orji and Moffatt, 2018) in various domains. However, like all technologies, persuasive systems can have positive as well as harmful effects on their users (Berdichevsky and Neuenschwander, 1999; Stibe and Cugelman, 2016; Nyström and Stibe, 2020). Alongside the increased occurrence of persuasive systems, we have seen a growing interest in research regarding ways in which persuasiveness can encourage positive alterations of human behavior. Researchers have targeted a wide range of behaviors such as reduced energy use (Petersen et al., 2007), improved study habits (Filippou et al., 2016) and the health domain has received its fair share of attention. Scholars have, for example, studied how persuasive principles can help individuals overcome addictive behaviors (Canale et al., 2015; Klein et al., 2019), manage diseases (Schnall et al., 2015) and overcome mental health issues (Arshad et al., 2019). A large part of this research focuses on explorations of how to use persuasive systems, principles and technologies to improve personal wellbeing and wellness, covering target behavior such as healthy eating, increased physical activity and weight management (Kaptein et al., 2012; Orji and Moffatt, 2018; Espinoza and Baranauskas, 2020). In parallel, developers of commercial products such as health and fitness apps have turned their attention in the same direction, looking for profit. The last decades the world have seen increasingly noticeable effects of an unhealthy lifestyle such as increased global occurrence of obesity and overweight (World Health Organization, 2021), but also increased pressure on individuals to look or weigh a certain way (Davison and Deane, 2010; McCabe et al., 2015; Eikey, 2021). This development creates a golden opportunity for businesses and according to Grand View Research (2023) the global weight management market size was in 2021 valued to 132.7 billion USD with an expected annual growth rate of 9.7% until 2030.
Weight loss can be both necessary as well as dangerous and in parallel with research targeting the potential of persuasive systems, scholars have highlighted harmful aspects of such systems by raising ethical concerns. Even if a researcher, designer, or developer of persuasive systems has honorable intentions the fact still stands, the aim is to change someone else's behavior (Davis, 2009) and outcomes are not always given. In 2006, Atkinson (Atkinson, 2006) focused on unintended consequences of persuasive systems and considered designers as being responsible for all intended and unintended, as well as positive and negative, outcomes. Others have followed and explored unintended and negative outcomes of behavior interventions (Lupton, 2014; Nyström and Stibe, 2020; Osman et al., 2020). In theory, unintended consequences of persuasive systems use may seem like a marginal error, but in reality, such consequences can cause long-term and harmful effects.
In the context of health and wellbeing, scholars have established connections between persuasive system use, health and fitness apps, and eating disorders (Levinson et al., 2017; Simpson and Mazzeo, 2017; Honary et al., 2019; Eikey, 2021). Levinson et al. (2017) established that out of 105 individuals, all diagnosed with eating disorders, 73% considered a calorie tracking application as contributing to their eating disorder. Honary et al. (2019) focused on individuals aged between 18 to 25 and established that almost every second individual had experienced negative behaviors and experiences as a result of using healthy eating and fitness apps. Eikey (2021) showed how diet and fitness apps caused unintended and negative outcomes for their users by both triggering and exacerbating symptoms related to eating disorders.
This paper builds upon work by researchers focusing on unintended and negative consequences of persuasive systems (Lupton, 2014; Stibe and Cugelman, 2016; Nyström and Stibe, 2020) as well as research focusing on the relation between such systems and eating disorders (Levinson et al., 2017; Simpson and Mazzeo, 2017; Honary et al., 2019; Eikey, 2021). With a specific focus on the persuasive system design of weight loss apps we conduct a longitudinal exploratory expert evaluation of 10 free weight loss tracking apps by emulating drastic weight loss. The underlying motivation is to unravel how these applications respond to drastic weight loss behavior. In the context of this paper, we define drastic weight loss as losing more weight, and with a higher pace, than what is recommended. The research question guiding our work is: How does the persuasive design of weight loss tracking apps respond to a drastic weight loss journey?
The paper is structured as follows: The following section presents research on persuasive systems and negative dimensions of system use, while the third section presents the methodological approach and introduces the 10 selected apps. Section four describes our emulated, and drastic, weight loss journey and how the apps responded to it, while section five is devoted to contextualizing and discussing the results in relation to previous research. Lastly, the paper is concluded and suggestions for further research are provided.
2. Persuasive system research and use
In HCI, the question of how design can be used to influence human behavior has been a focal concern for quite some time. A starting point was Donald Norman's work on how to design products in such a way that they become usable and appreciated by their users (Norman, 1998). Norman proposed a set of simple rules consisting of making things visible, exploiting natural relationships between function and control, and to constrain available options with the ambition to cater for effortless and positive user experiences. While Norman had the ambition to support user decision making in design, others soon began to explore how systems could purposefully be designed to influence human behavior (Fogg, 1998, 1999). These efforts resulted in various guidelines and principles for persuasion (Lockton, 2013) and the emergence of persuasive systems (Cialdini, 2001; Harjumaa and Oinas-Kukkonen, 2007; Filippou et al., 2016). This kind of system was early on defined as” … a computing system, device, or application intentionally designed to change a person's attitude or behavior in a predetermined way” (Fogg, 1999) and even if far from all systems can be considered persuasive, we should expect to encounter persuasion whenever we use our mobile phones and apps, visit websites or play video games. Persuasion can be designed for in many different ways and while Fogg (2003) suggested seven strategies of persuasion, Harjumaa and Oinas-Kukkonen (2007) developed these strategies further into 28 principles divided into four specific types: primary task support, dialogue support, system credibility support and social support. Over time, these principles have been relied upon to promote a wide range of behavior changes in a variety of domains (Petersen et al., 2007; Filippou et al., 2016). Petersen et al. (2007) used persuasive principles (data monitoring, feedback, and incentives) to make dormitory residents reduce their use of energy and water, while Filippou et al. (2016) relied on social support principles and focused on improvement of study habits. A majority of the attempts to apply persuasive strategies and principles are found in the health domain (Canale et al., 2015; Schnall et al., 2015; Arshad et al., 2019; Klein et al., 2019), often with a focus on personal wellbeing and wellness, covering target behaviors such as healthy eating, physical activity and weight management (Kaptein et al., 2012; Orji and Moffatt, 2018; Espinoza and Baranauskas, 2020). Kaptein et al. (2012) for example relied on tailoring as a persuasive principle and showed how short text messages tailored for specific individuals had a better effect on snacking behavior than randomized messages. Espinoza and Baranauskas (2020) developed a socially-aware persuasive system to encourage healthy eating, a system that relied on the persuasive principles of trustworthiness, social facilitation, autonomy, emotional and sensorial motivation. In an attempt to unravel just how effective persuasive systems are, Orji and Moffatt (2018) conducted a review of 85 published studies, where persuasive systems had been used to induce health-related behavior. The result showed that 64 studies, out of 85, reported completely positive outcomes, while 17 studies reported partially positive results. Among these studies, the most commonly deployed persuasive principles were: Tracking and monitoring; Audio, Visual and Textual Feedback; Social support, sharing and comparison; Persuasive messages, reminder, and alert; and Reward, points, credits.
Despite the broad application of persuasive systems in a wide range of domains and their perceived value, parallel strands of research points toward unintended and unwanted effects of behavior interventions and persuasive systems.
A range of scholars have argued that persuasive strategies, grounded in insights regarding human behavior, can be used to influence individuals in ways that are not in their best interest (Stibe and Cugelman, 2016; Gray et al., 2018; Maier and Harr, 2020). Some researchers, such as Berdichevsky and Neuenschwander (1999) and Fogg (2003), have focused on ethical dimensions of persuasive strategies and the consequences these strategies might have for those persuaded. Even if a researcher, or designer, has the best of intentions, persuasive systems are as fraught with impending ethical faults as other technologies and as the purpose of these technologies is to change someone's behavior, things become even more complicated (Davis, 2009). Persuasive systems should, according to Borgefalk and de Leon (2019), assume responsibility for all realistically anticipated outcomes and Berdichevsky and Neuenschwander (1999) present eight principles for ethical design of persuasive technologies. One of these principles is the golden rule of persuasion; “the creators of persuasive technology should never seek to persuade a person or persons of something they themselves would not consent to be persuaded to do” (Berdichevsky and Neuenschwander, 1999, p. 52). Atkinson (2006) promoted the notion of designer responsibility for all consequences of use, including unpredicted, unintended and unforeseen. Recently, we have seen a growing interest in explorations of effects, including unintended and negative effects, of behavior interventions, encompassing persuasion that might, or might not, rely on technology (Fishbach and Choi, 2012; Lupton, 2014; Stibe and Cugelman, 2016; Nyström and Stibe, 2020). Stibe and Cugelman (2016) focus on unintended outcomes of such interventions and presented the intention-outcome matrix (see Figure 1) to describe various kinds of outcomes, positive as well as negative.
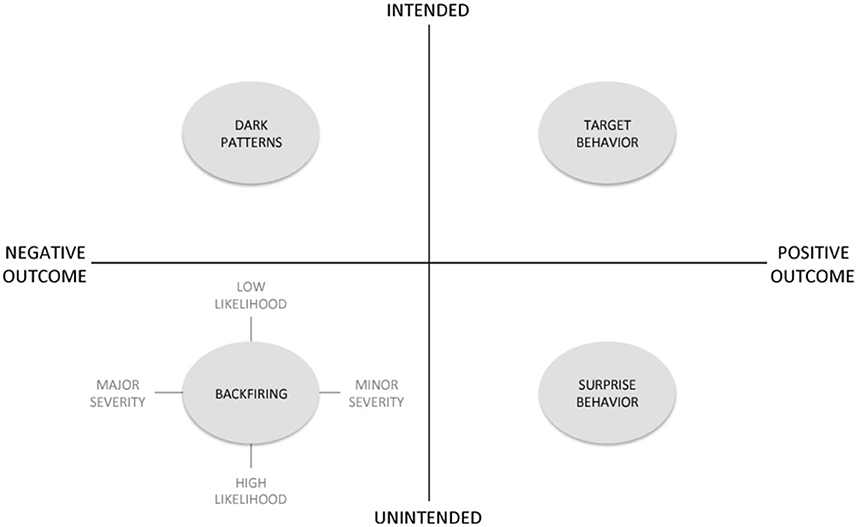
Figure 1. The Intention-Outcome matrix (Stibe and Cugelman, 2016) describes outcomes of behavior interventions as either intended or unintended and as causing either negative or positive outcomes.
According to Stibe and Cugelman (2016) most behavior interventions aim at a certain positive, target behavior, but can also cause additional and unexpected, however still positive, surprise behaviors. An intervention done to promote the target behavior of quitting to smoke can for example have the surprise behavior of less money being spent on cigarettes. Dark patterns is when an intervention has an intended and negative outcome for users but a positive outcome for stakeholders (Brignull, 2013). Scholars have for example reported instances of dark patterns in game design causing negative experiences for players without their consent (Zagal et al., 2013; Linehan et al., 2015). An intervention can also have backfiring effects (Stibe and Cugelman, 2016; Nyström and Stibe, 2020) that occurs when the target audience adopts the opposite target behavior. This makes an intervention, at least partially, responsible for undesirable, sometimes harmful, behavior changes and could consist of an intervention that instead of promoting healthier drinking habits results in increased drinking. Or in the context of weight loss interventions, instead of weight loss, could lead to weight gain. Stibe and Cugelman's (2016) model is incomplete in the way that it fails to recognize negative surprise behaviors that are not oppositional to the intended target behavior. One can easily imagine that an intervention done to promote the target behavior of quitting to smoke can lead to an unwanted increased consumption of sweets and candy. Alternatively, in the context of health, that an intervention for weight loss could lead to development of eating disorders.
In the health domain, persuasive interventions often consist of technological interventions, promoting healthier eating habits or increased physical activity. Such technological interventions have become a substantial component in the growing market of global weight management, a market with an expected annual growth rate close to 10% (Grand View Research, 2023). As indicated by Orji and Moffatt (2018) such interventions can have both positive and negative effects and Levinson et al. (2017) suggested a connection between usage of persuasive systems and eating disorders. Out of 105 individuals, all diagnosed with eating disorders, almost three out of four considered a calorie tracking application as contributing to their condition. In a review of healthy eating and fitness apps Honary et al. (2019) established that two-thirds of the apps allowed reporting 50% less than the recommended daily calorie intake and that almost a fifth of the apps allowed underweight goal setting. This should be seen as hazardous in a world where at least 9% of the global population are affected by eating disorders, one of the deadliest mental illnesses worldwide (Arcelus et al., 2011), causing one death every 52 min (Deloitte Access Economics, 2020). Eikey (2021) focused specifically on the effect of diet and fitness apps on eating disorder behaviors by conducting a three-step study with 24 participants, all having suffered from eating disorders. The study, based on surveys, a think-aloud exercise and semi-structured interviews, showed how apps developed to support health and wellbeing, caused unintended and negative outcomes for users. The apps were considered to both trigger and exacerbating symptoms related to eating disorders by focusing heavily on quantification (also shown in Honary et al., 2019), providing certain types of feedback and promoting overuse. Among other things, it was concluded that app feedback and visualization, such as choice of colors and messages, contributed to these negative effects. Many participants described feelings of being rewarded when being shown green progress bars and felt guilt, embarrassment and shame, when being shown red visualizations, indicating that the calorie budget had been exceeded.
With a foundation in previous research on persuasive systems and their potential unintended and negative effects, but also in research focusing on the relation between such systems and eating disorders, we set out to explore how persuasive system design respond to emulated drastic weight loss. More specifically, we conduct a longitudinal exploratory expert evaluation of 10 free weight loss tracking apps. This in order to unravel how these apps respond to drastic weight loss and how they potentially pave the way for unintended and negative consequences.
3. Method
Within the health domain it has become increasingly common to survey and evaluate persuasive mobile systems to study aspects such as engagement, functionality and perceived quality, or to identify risks related to usage (Schoeppe et al., 2017; Honary et al., 2019). Within the field of HCI we are no strangers for similar practices and have for example developed practices for evaluating systems by using them ourselves (Neustaedter and Sengers, 2012) or by involving experts in the review (Rieman et al., 1995; Tondello et al., 2016). In this paper we have used our own skills as knowledgeable in the field of persuasive design. As we also wanted to focus on how the design of the selected apps responded to an emulated and drastic weight loss journey, in terms of overall weight loss and speed, we found it necessary to deploy a longitudinal approach where the process could be traced over time. Consequently, we decided to conduct a longitudinal exploratory expert evaluation with the focus on exploring how persuasive systems i.e., weight loss tracking apps, respond to drastic weight loss behavior (in terms of overall weight loss and weight loss speed). This type of longitudinal expert evaluation is not an uncommon methodological approach in HCI and have for example, been used by Galbraith et al. (2006), as well as Daudén Roquet and Sas (2018).
3.1. Sampling—Choosing apps
According to Buildfire.com, 98% of global app revenue comes from apps available for free, which indicates that only a small proportion of the population are willing to pay for downloads. This, in combination with the fact that eating disorders are more common in younger age groups (Eikey, 2021), with limited financial means, made us focus our sampling on apps free for download. We are fully aware that such a focus might introduce a bias in our sampling procedure, if we assume that purchased apps would perform better, but given the reasons mentioned above we decided to focus on apps available for free. Both authors were in possession of android smartphones (Samsung Galaxy 9 and Huaweii P30), from that a decision was made to select apps from Google Play. On October 1st 2021, at a Swedish university, we searched the google play store for “free weight loss tracker” and sorted the results based on app popularity. While all weight loss apps support users in losing weight, the ways in which it is done differs. A weight loss tracker is an app that primarily focuses on helping the user to keep track of their weight loss through reoccurring logging of data. The kind of data that is commonly logged/tracked throughout a weight loss journey is typically a user's weight and other kinds of measurements. Due to algorithmic curation and personalization we relied on the search result from one of the authors and further refined the selection of apps by applying a set of selection criteria. These criteria, besides that apps should be free for download, were as follows:
- A selected app should not require logging of meals and exercise (often required in many weight loss apps) as this would complicate the study quite radically by requiring identical, and constant, entries in all apps.
- An app should be possible to use given our research design (e.g., apps with free trial periods or that did not allow us to define a weight goal were excluded).
- We also wanted to avoid including several apps from the same provider as these are often very similar in terms of functions and style.
We decided on the 10 most popular apps, matching our selection criteria, that all had rankings between 1.0 and 4.8, had at least been downloaded 100 000 times and had on average been downloaded 2,510,000 times. In the Table 1, we present the sampled apps.
3.2. Procedure
We initiated our study on October 1st, 2021, by installing all apps on our android smartphones. We created users, one man and one woman, both given the age of 35 years and with a weight that, given a length of 187 cm for the man and 176 cm for the woman, would generate a BMI over 30 (105 kg for the man and 95 kg for the woman).
According to the American center of disease control (CDC, 2021) a person with a BMI over 25 is considered overweight, while a person with a BMI over 30 is considered obese. BMI is a well-established way to classify people based on their weight and we defined weight goals that would bring us below a BMI of 18 and consequently place us in the underweight range (CDC, 2021). Using BMI as classification tool for measuring peoples' health is far from unproblematic (Humphreys, 2010; Tomiyama et al., 2016). Humphreys (2010) argue for the dangers associated with using a tool that fails to consider individual, age related and racial differences as a proxy for health, while Tomiyama et al. (2016) showed how BMI, in comparison to cardiometabolic measurements, can lead to misclassifications of peoples' health status. Besides spending resources on treatments for people who don't need them, and failing to spend them on those who do, a result of using BMI as a proxy for health can in many cases be exacerbated suffering and stigmatization. In the context of this paper we were however forced to use BMI as it is the measurement used in the selected apps.
Given that both users should start with a BMI over 30, and aim for a target BMI of 18, we ended up with the weight goals of 60 kg for the man and 55 kg for the woman. We set out to have an initial weight loss pace of 2 kg per week and thereby exceeding a safe weekly weight loss of 0.5–1 kg as recommended by Centers for Disease Control and Prevention (CDC) (CDC, 2022). CDC further clarifies that a faster weight loss might cause health problems such as gallstones, malnutrition, and make an individual feel unwell and tired. Relying on these recommendations from CDC for deciding what constitutes a drastic weight loss journey could be questioned, especially if we add a more holistic perspective on health. One can imagine a situation where an individual can benefit from a drastic weight loss, for a limited period of time, in order to reach positive long-term effects, such as being allowed to undergo gastric bypass surgery. Still, in order to explore our research question we found it necessary to rely on these recommendations.
During the first week we set up our profiles and explored the apps without logging any weight loss. This in order to try them out and ensure they matched our selection criteria. We further explored the information provided in the apps by going through sections such as Terms of condition, FAQs, Info, Purpose of the app etc., to see if any weight loss recommendations, in terms of how fast and how much weight it is healthy to loose, were provided. The first weight loss was registered the 8th of October and every Friday until the end of the study (24th and 31st of December as an additional week was required for the male user). We further decided to allow notifications from the apps every Monday, Wednesday, and Friday at noon. Data was gathered, throughout the process, through a structured weekly procedure consisting of taking screenshots of every instance of use with all the apps such as when users were created, when notifications were received, when new weight were added and when the apps reacted to these changes in any way.
On a few occasions we provoked the apps to study their reactions. These provocations consisted of once gaining weight (1 kg), once not reporting weight and to increase our weight loss speed to first 4 kg/week and later to 8 kg/week.
3.3. Data analysis
Our data was analyzed through an iterative and structured process where we continuously, every Friday, analyzed reactions from the apps and as a consequence developed extensive knowledge of their response to our emulated weight loss behavior. We applied a structured procedure for the data gathering and, among other things, focused on the following questions:
• How, and to which extent, do the apps provide feedback on a drastic weight loss in terms of overall weight loss and weight loss speed?
• If, and in that case how, do the apps encourage our emulated behavior?
• If, and in that case how, do the apps dissuade our emulated behavior?
• What persuasive principles are applied in the design and how?
In the end of the study, we ended up with 596 screenshots from the apps. We created a Miro-board for the analysis, transferred and structured all screenshots for a deeper analysis. A sample of the Miro-board is provided in Figure 2.
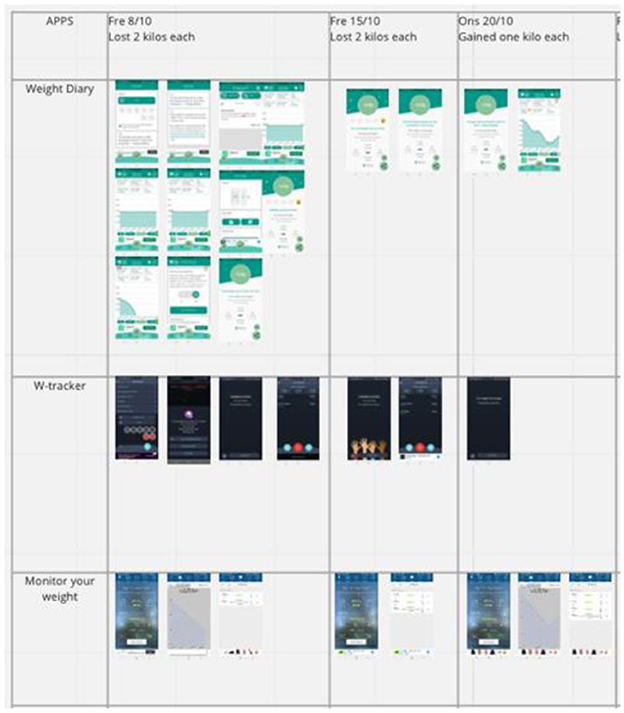
Figure 2. A sample view of the Miro-board, used in our analysis, showing the different apps in the left column, dates for data gathering sessions in the top row, and screenshots from each app in the center.
Based upon a chronological structure of each app, shown in the figure above, we collaboratively analyzed how the apps responded to our weight loss journey, including how they used persuasive principles to encourage and/or dissuade our emulated weight loss process. In detail, we started out by familiarizing ourselves with the data through reviewing all screenshots together, from all apps and all dates of entry. We then carefully and systematically studied all screenshots, from all apps, on each specific date of entry. For this process we made use of a printed Miro-board, mark-up pens and took notes on app reactions, identified instances of persuasive principles used, highlighted patterns and differences. We focused primarily on ways through which apps persuaded, often through persuasive principles, or dissuaded the emulated behavior throughout the entire process.
4. Findings—A weight loss journey
We outline the findings from our longitudinal study based upon a chronological structure and by providing descriptions and screenshots illustrating the design of the selected weight loss tracking apps. We use images from both our female and male profile but have blurred certain elements in the screenshots for integrity reasons. We further present figures to support the reader's understanding and not always in a completely authentic chronological order.
4.1. Setting up and starting the weight loss journey
After downloading the apps, we explored them to see if, and in that case how, they provided any information regarding what is and what is not a recommended weight loss. We searched through sections such as Terms of condition, FAQs, Info, Purpose of the app etc. and found that two out of 10 apps (No. 3 and 9) presents disclaimers and advise users to consult their doctors before starting to use the apps. Two out of 10 apps (No. 6 and 7) provide explanations about what BMI is, how it is calculated, and what a certain value means. The next step consisted of us creating user profiles including choosing gender (male and female), setting our age (choosing 35 y/o for both profiles), defining our real height (187 cm and 175 cm), our decided start weights (105 kg and 95 kg) and target weight (60 kg and 55 kg). Two out of 10 apps offered us to calculate an ideal weight goal (Figure 3, first image) but did not object when we answered no and instead accepted our much lower weight goal. We further added a target date for our weight loss journey, a date matching a weight loss speed of 2 kg per week. However, two out of 10 apps (No. 1 and 2) did not support such entries, even if all supported entries of target weight. App No. 5 automatically calculated a target date that was far later than what our planned weight loss speed implicated (Figure 3, middle image). When changing date to a much earlier one the app did however not object. App No. 3 (Figure 4, right image) informed us that experts advise against losing more than 1 kg per week, but after a click on the continue button a much earlier target date was accepted without further comments made.
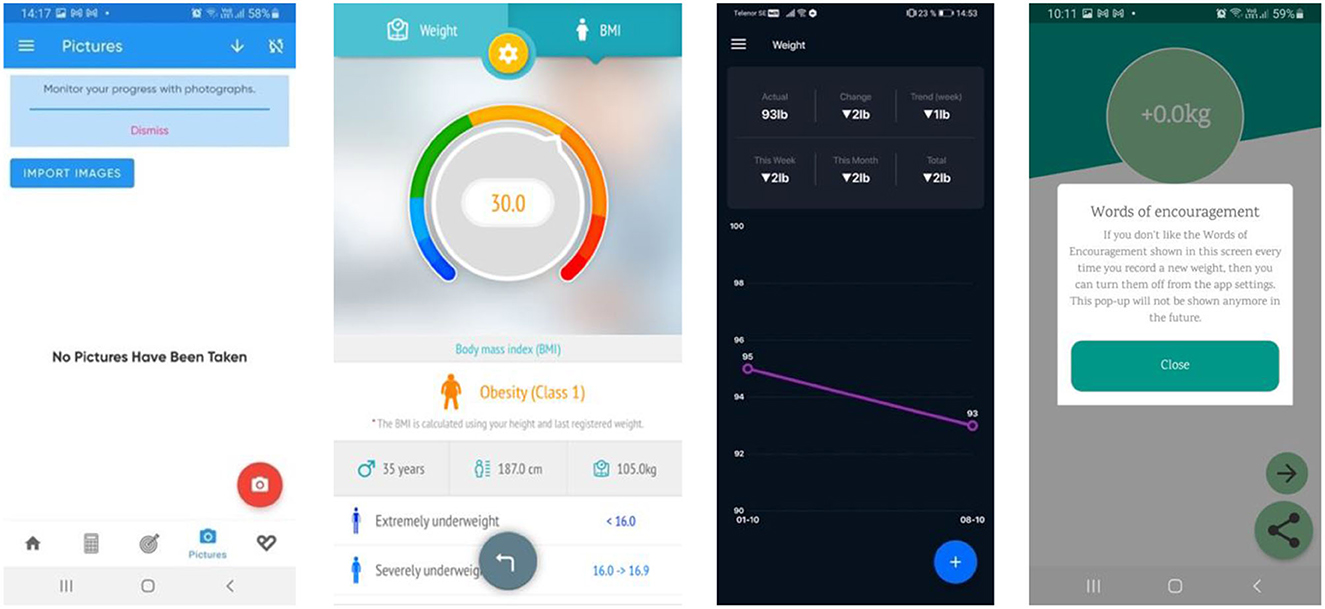
Figure 4. Screenshots of app reactions when starting weight was registered. From the left, app No. 1 offers upload of images to monitor progress, app No. 10 classify the weight entry as obese on a color scale, app No. 2 plots the weight in a graph, and app No. 3 offers words of encouragement.
In some cases we were asked to register our current activity level or physique, when this happened we chose medium or the average option. Two out of 10 apps (No. 3 and 8) offered us to document our weight loss journey with pictures or to create before and after pictures (Figure 4, left image), while two apps (No. 8 and 9) offered us to measure our bodies in different ways (e.g., waist, neck and shoulders). We chose not to.
Upon registering our weights most of the apps displayed our current weight status as overweight. Four out of 10 apps (No.1, 3, 5, and 10), labeled us as belonging to the category “Obese, Class 1” and three apps (No. 1, 7, and 9) indicated our starting weight and BMI using a color scale, similar to a speedometer (Figure 4, middle-left image). The scale ranged from a red zone (overweight) to a blue zone (underweight). One app (No. 10) also showed our weight status placed in a scale of different weight classes ranging from “Morbid Obesity” to “Extremely Underweight” and an associated human figure mirroring the weight class in color and form. For overweight the figure is broad and red, for extremely underweight the figure is thin and blue, and for normal weight its green and has its arm raised above the head. The other apps just noted our weight without indicating what weight category we belonged to. All apps plotted our weight in a graph with weight on the y-axis and time on the x-axis, without referring to any weight class (Figure 4, middle-right image). In the graphs, six out of 10 apps (No. 4, 5, 6, 7, 9, 10), also marked our target weight goal, while app No. 5 in addition displayed our determined weight loss progress in a graph alongside our weight loss trend.
When creating accounts, we accepted an offer of receiving words of encouragements from the only app (No. 3) that offered us such an opportunity (Figure 4, right image) and further decided to receive reminders every Monday/Wednesday/Friday (at noon) from the nine apps that offered such an opportunity (No. 5 did not).
4.2. Notifications, app reactions and provocations
The following week we received our first notifications from the apps. Most notifications were rather passive and just reminded us to enter our weight by saying something like “It is time for weighing” (No.4), “Weight reminder. Tap to enter” (No. 2), or “Did you weigh yourself today?” (Figure 5, left image). App No. 3 provided messages of a more persuasive kind saying: “How can we help you? Will it be easy? Nope. Will it be worth it? Absolutely. You downloaded the app for a reason. Record your weight and check the new progress.”
After 1 week we reported a weight loss of 2 kg upon which the apps reacted in different ways. Most apps only reacted by showing the weight loss and the current weight without much feedback. Three out of 10 apps (No. 1, 6, 7) showed the weight loss in green text in a table of measured weights (Figure 5, middle-left image), while three apps (No. 4, 5, 9) had the same list but without colors. However, app No. 5 also showed a status bar with five filled fields for the female user, but only four filled fields for the male, likely due to calculations of weight loss in comparison to start weight. Eight out of 10 apps also displayed the weight loss trend in a graph (No. 1, 2, 3, 4, 6, 7, 8, 9) and in app No. 6 this was shown alongside the table of measured weights. Most of these apps just plotted the new weight to create a weight loss curve (in combination with the target weight), but app No. 5 showed three different trends in the graph (Figure 5, middle-right image). One with the chosen weight loss pace and estimated target date (in red), another with our measured weights (in blue), and yet another in green. Without being sure we believe this green curve to represent a suggested weight loss journey as the target date is placed later in time than what we chose from the start. Three out of 10 apps provided positive feedback saying “That's it! You have lost weight” (No. 3), “CONGRATULATIONS! You lost 2.0 kg. 38.0 kg left to your goal” (No. 4), and “-2 kg! Congratulations. You were able to reduce your weight with 2 kg. Now only 38 kg is left to reach your target weight!” (No. 2). Two of these apps provided animations such as raised hands (No. 4) or fireworks (No. 9) with sound of cheering (Figure 5, right image) and one of them showed a happy smiley (No. 3). The reactions were similar the following weeks once weight loss was registered. Once registering our weight loss, two out of 10 apps (No. 3 and 9) offered the opportunity to share the results. This resulted in sharing the congratulatory view as an image through the phone's available options for sharing material, such as when sharing any picture or content (e.g., Messenger, Facebook, SMS).
In a later step we decided to report a weight increase of 1 kg to provoke the apps, but little happened. App No. 3 provided the following feedback “It always seem impossible until it is done—Nelson Mandela. You have gained weight” and displayed our new weight. This app, that showed a happy smiley after previous weight losses, now showed no smiley at all. Three out of 10 apps (No. 1, 9 and 10) displayed our weight gain in red text and app No. 7 used yellow text in comparison to green text that was used when weight was lost. App No. 5 showed a progress bar with five fields where two were filled with orange color (instead of green for the previous weeks with weight loss). Another app (No. 3) stated that we had been able to increase our weight with 1 kg and that only 34 kg (for the male) and 31 kg (for the female) remained until meeting our weight goal. The other apps just noted our weight and changed graphs accordingly.
After a couple of weeks, we decided to increase our weight loss to 4 kg per week, four times more than the recommended weight loss pace, to see if such a weight loss speed provoked reactions from the apps. None of the apps reacted in ways significantly different from when 2 kg were lost per week. App No. 5 now provided slightly more positive feedback to the male user by showing five filled green fields, in comparison to four shown with a lower weight loss speed. The two apps that provided more concrete positive feedback (No. 4 and 9), with animations and sound, reacted in the same way as before, even if the weight loss speed had doubled. Another week we provoked the apps by not registering any weight. This caused no reactions from the studied apps, and we received the same notifications as we did every other week. Another provocation was to increase our weight loss even further, to 8 kg per week. This caused no additional reactions at all from the apps and was displayed in the same ways as when 2 and 4 kg were lost. This weight loss speed increase swiftly brought us to the closure phase of our study.
4.3. The end of the weight loss journey
In the 12th week the female user reached her weight goal, and the male user went from normal weight to underweight. The male user reached his weight goal week 13. Almost none of the apps acted differently when we reached the underweight category, in comparison to how they had reacted before. App No. 3 showed a happy, but slightly less happy, smiley than the week before (without explaining why), but on the other hand also provided the feedback “Marvelous. You have lost weight.” App No. 5 still showed us the completely green status bar and five out of 10 apps (No. 3, 5, 7, 9, and 10) informed us about being underweight (Figure 6, left image) or belonging to the category Mild thinness (App No. 3).
Once reaching our target weight, two out of 10 apps (No. 5 and 10) informed the male he now was severely underweight or, as presented by App No. 7, now belonging to the group “Undernutrition and anorexia” (Figure 6, middle-left image). Five out of 10 apps (No. 3, 4, 5, 6, 9) provided positive feedback for reaching the target weight by stating “- 8 kg. Congratulations. You were able to reduce your weight ... You have reached your target weight of 60 kg!” (No. 9), “You reached your target weight, which you set yourself at the weight of 105.0 kg. Keep it up )” (No. 6), “CONGRATULATIONS! Goal achieved!” (No. 4), and “Congratulations! You have reached your weight goal” (No. 3). App No 5, simply said “Congratulations!” and the name of the profile while also stating that the profile was severely underweight (Figure 6, middle-right image). Two apps alongside the written feedback displayed images of a medal (Figure 6, right image) and a winner's cup (No. 4). App No. 3 presented a surprising view showing the BMI of the male user and offered to change the height, without a clear explanation for why this change was suggested. The other apps reacted the same way as they always did when a new weight was entered with updated weights and graphs.
5. Discussion
The paper builds upon previous work focusing on unintended and negative consequences of persuasive systems (Lupton, 2014; Stibe and Cugelman, 2016; Nyström and Stibe, 2020) and research focusing on the relation between such systems and eating disorders (Levinson et al., 2017; Simpson and Mazzeo, 2017; Honary et al., 2019; Eikey, 2021). With a focus on the design of persuasive weight loss apps we conducted a longitudinal expert evaluation of 10 weight loss tracking apps, through an emulated drastic weight loss journey, to explore the following research question: How does the persuasive design of weight loss tracking apps respond to a drastic weight loss journey? The study is motivated by the increased use of persuasion in system design, the notion of unintended consequences of using such systems and the prevalence of eating disorders (Arcelus et al., 2011). The results clearly indicate how the providers of the studied apps, used by more than 24 million users in total, take little responsibility in relation to the users of their persuasive systems and their health. This lack of responsibility is something that could lead to negative outcomes (Osman et al., 2020) where apps, instead of promoting reduced weight, could promote health threatening conditions such as eating disorders.
The apps included in the study are all persuasive in nature and have all been “… intentionally designed to change a person's attitude or behavior in a predetermined way” (Fogg, 1999). The degree to which they are persuasive however differs. Some of the apps show a somewhat passive approach to our weight loss journey where designers make use of a rather limited set of persuasive principles and apply them in rather cautious ways. These apps, like many apps in the health domain (Orji and Moffatt, 2018) primarily employ the principles of Tracking and monitoring and Persuasive messages, while other apps rely on a larger repertoire of principles. The apps applying a more active approach rely on additional principles such as Audio, Visual and Textual Feedback, Social support (to some extent), sharing and Comparison, and Reward, points, credits.
Stibe and Cugelman (2016) makes a distinction between intended and unintended outcomes of behavior interventions in their Intention-Outcome matrix. Given the methodological approach of this study it is hard to know for sure what design decisions that belong to one of these categories, or the other. However, to actively encourage, or passively accept, a weight loss journey where too much weight is lost and too fast, fits poorly with ethical principles for design and development of persuasive systems (Berdichevsky and Neuenschwander, 1999; Borgefalk and de Leon, 2019) and can in the end lead to negative consequences for their users (Stibe and Cugelman, 2016; Nyström and Stibe, 2020). One such consequence could be that an individual, instead of losing weight in a healthy manner, runs the risk of ending up as underweight and suffering from several health-related conditions. In the context of the Intention-Outcome matrix (Stibe and Cugelman, 2016), that would constitute a surprise behavior, a negative one.
In the beginning of our study, we lost 2 kg per week, twice as much as recommended (CDC, 2022). We then doubled that loss to 4 kg per week and then doubled it again to 8 kg per week. Losing as much weight as 8 kg per week is perhaps not realistic, but our intention with this radical increase of weight loss was to see if there were any kind of threshold in the apps for how much weight loss they were willing to accept. It turned out that only one of the apps informed us that we were planning to lose too much weight per week. Another app suggested a more distant target date, which in practice would have reduced our weight loss speed per week. However, none of these apps stopped us when overlooking these suggestions and entering our weight loss. A similar omission was noted when entering our target weights, below the recommended BMI (CDC, 2021) where only two of the apps offered us to calculate our ideal weight. The fact that eight apps allowed underweight weight goals is a percentage much higher than what was found in Honary et al. (2019) where 21% of their reviewed healthy eating and fitness apps allowed such goals. No objections were made from the two apps when we turned the offers down.
As mentioned above, some of the apps were perceived as passive, while some of the apps relied on a more active approach to encourage our unhealthy weight loss journey. Encouragement was conveyed from the apps in different ways of which several have previously been identified in research (e.g., Eikey, 2021). Most apps included in our study focused heavily on quantification, for example through the use of graphs and constant comparisons between our current weight and target weight. This is an approach that have been pointed out as problematic in previous research (Honary et al., 2019) as it might create fixations on numbers and induce negative emotions. Another example of encouragement was the way in which positive feedback was provided (cheers, congratulations or symbols of medals, fireworks and trophies). Relying on positive feedback is a common approach to encourage use of persuasive systems [72% of the apps included in Honary et al.'s (2019) review made use of positive feedback], while providing negative feedback is a less common approach. Encouragement was also provided in the way colors were used to reinforce wanted, or to prevent unwanted, behavior or status. In several cases weight loss was presented in green, while weight gain was presented in red and when classifying our weights on scales overweight was marked with red and underweight with the more neutral color blue. Eikey (2021) highlight the role of color in feedback and expressed a need for more nuanced visualizations to avoid negative effects. This is in line with our results where the color red was used to show overweight warnings, while blue was used for indicating underweight. In many cases, the use of blue is straightforward and neutral but given our specific weight loss journey it could be deemed as problematic.
Upon reaching our underweight target the neglect of potential negative consequences became apparent as several apps congratulated us on our unhealthy weight loss, while at the same time informing us, we were now undernourished, anorectic or severally underweight. One app also surprised us by offering us to change our height as soon as we had reached the target weight. We cannot say for sure why this message was shown, as a user's actual height hardly changes during a weight loss journey. One possible explanation could be what Stibe and Cugelman (2016), in their Intention-Outcome matrix, label as a dark pattern. These patterns encourage behavior that has negative outcomes for users but a positive outcome for the stakeholders (in the context of this study a stakeholder would be an app provider). Without knowing the intention of the provider we cannot say for sure, it could also be a design flaw.
As previously mentioned, some of the apps took a more passive approach to our unhealthy weight loss journey, while others were more active, providing encouragement. One could see passivity as a way to move the responsibility to the user and several of the apps also placed the user in control of the persuasiveness of the system. This was for example done by allowing users to choose whether they want to get reminders or not, choose to receive words of encouragement or not, or giving them a choice to add before and after pictures (or various body measures). This could be seen as a way to move control from the company, or the designer of an app, to the user. This approach is problematic for two reasons. First, it goes against the claim by Borgefalk and de Leon (2019) that persuasive systems should assume responsibility for all realistically anticipated outcome of their use, and Atkinson's (2006) designer centric perspective where designers of persuasive systems are responsible for the consequences of their use, including unforeseen and unintended usage. But likely also against Berdichevsky and Neuenschwander's (1999) golden rule of persuasion. Secondly, pushing decisions of persuasion toward a user that might develop health threatening conditions (such as eating disorders) is perceived as a hazardous and irresponsible decision.
The insights generated from our study clearly indicate a need for ethics in the design of persuasive weight loss tracking systems in order to avoid negative consequences for their users. Here a tradeoff emerges for the providers of these systems. Should they provide the kind of apps that users seem to demand, financially rewarding but ethically questionable and potentially dangerous for users, or should they provide ethical systems that restrict the autonomy of the user and run the risk of a decreasing customer demand and profit? Based upon the insights provided by this study we argue for restrictions, through design, when it comes to drastic weight loss speed and overall weight loss. When it comes to the application of persuasive principles in weight loss tracking apps, we suggest a two-step process. We suggest that these principles are in a first step applied with the ambition to help users reduce their weight, with a recommended pace, until they have reached a recommended target weight (preferably not based upon BMI). In a second step, the same principles are applied to maintain that healthy stage, which would also combat the risk of weight cycling. This two-step approach would mean that principles, e.g., Audio, Visual and Textual Feedback, Social support, sharing and Comparison, and Reward, points, credits, are the same during the two steps, but the behavior they are designed to induce changes. This would likely decrease the risk of negative consequences and might also increase the longevity of these persuasive systems. The responsibility for making this change lies not only on designers, but also on developers and decision-makers at companies that provide these systems. We further consider organizations that provide access to the systems, in our case Google, as having their share of responsibility and that legislation might be necessary.
6. Conclusions
This paper builds on research focusing on unintended and negative consequences of behavior interventions by focusing on the health domain, specifically the design of persuasive weight loss apps. The research question guiding our work was: How does the persuasive design of weight loss tracking apps respond to a drastic weight loss journey?
To address this research question, we conducted a longitudinal exploratory evaluative study of 10 free weight loss tracking apps, using two different profiles starting as overweight, losing weight too fast, and ending up as underweight. Data was gathered through a structured process where weekly weight entries were made and the responses from the persuasive systems were carefully documented and analyzed through a structured process.
The results show how apps, through the way they are designed and respond to weight loss, might cause negative consequences for their users. The apps included in our study responded to our process in two different ways, where some of the apps remained passive, with a low level of persuasiveness, while other apps took a more active stance and continuously encouraged the drastic weight loss journey. This encouragement was primarily manifested in the designs through reliance on display of quantitative data, textual or symbolic feedback and the use of color and symbols. No matter if an app was considered as passive or active, their designs did little to prevent unintended and negative consequences to occur. In fact, several of the apps did the opposite. This shows how the people behind the studied persuasive systems took little responsibility to prevent negative consequences of system use.
The findings contribute to current research by providing empirical insights on persuasive system use and the relation between design of these systems and potentially negative consequences for users. The insights further contribute to our understanding of how specific design decisions might increase the likelihood of negative consequences to occur and how persuasive systems should be designed to avoid it.
There are several limitations connected to the chosen methodological approach that deserves to be mentioned. The sample size was limited, even if it included the most popular freely available weight loss tracking apps, and a larger sample would have enabled us to shed even more light on the studied phenomenon. We further decided to focus on apps that were freely available for download due to revenue statistics, indicating that few are willing to pay for downloads, and the fact that eating disorders are more common in younger age groups with limited financial means. We are fully aware that more costly alternatives, or apps that also required logging of meals and exercise, could potentially mitigate negative consequences in a better way. In addition, even if the study was longitudinal and generated a rich set of data, none of the researchers suffered from weight problems and the weight loss journey was entirely artificial and predetermined. This brings that the insights provided are not those of actual users, nor of people going through an actual weight loss journey or suffering from eating disorders. On the other hand, this study relied upon firsthand experiences of the design of weight loss tracking apps, from the perspective of researchers, and how these relate to emulated and drastic weight loss journeys. This makes this study different from previous studies undertaken by researchers from other disciplines, to a large extent reliant on secondary data.
To shed additional light on the phenomenon of negative consequences of persuasive system use, and the relation between system design and such consequences, more empirical research is needed. Studies are needed to establish not only what persuasive strategies that are most commonly used in occurrences of negative consequences, but also how the implementation and design of such strategies increases or decreases their potential effect. We also welcome studies focusing on how purchased, and more developed, apps respond to weight loss journeys such as ours.
Data availability statement
The raw data supporting the conclusions of this article will be made available by the authors, without undue reservation.
Author contributions
RH and LÖ contributed to conception and design of the study. RH established the research foundation of the paper, while both took active part in the data gathering and data analysis process. RH was responsible for writing different versions of the paper, while LÖ provided feedback and suggested revisions throughout the process. All authors approved the submitted version.
Funding
The research presented in this paper was funded by the Department of informatics, Umeå University, Sweden.
Acknowledgments
We would like to thank the researchers connected to the research environment Design Informatics for providing feedback on an early draft of this paper.
Conflict of interest
The authors declare that the research was conducted in the absence of any commercial or financial relationships that could be construed as a potential conflict of interest.
Publisher's note
All claims expressed in this article are solely those of the authors and do not necessarily represent those of their affiliated organizations, or those of the publisher, the editors and the reviewers. Any product that may be evaluated in this article, or claim that may be made by its manufacturer, is not guaranteed or endorsed by the publisher.
References
Arcelus, J., Mitchell, A. J., Wales, J., and Nielsen, S. (2011). Mortality rates in patients with anorexia nervosa and other eating disorders. A meta-analysis of 36 studies. Arch. Gen. Psychiatry 68, 724–31. doi: 10.1001/archgenpsychiatry.2011.74
Arshad, R., Ali Baig, M., Tariq, M., and Shahid, S. (2019). “Acceptability of persuasive prompts to induce behavioral change in people suffering from depression,” in Human-Computer Interaction – INTERACT 2019: 17th IFIP TC 13 International Conference, Paphos, Cyprus, September 2–6, 2019, Proceedings, Part IV (Berlin: Springer), 139. doi: 10.1007/978-3-030-29390-1_7
Atkinson, B. M. C. (2006). “Captology: a critical review,” in Persuasive Technology, eds W. A. IJsselsteijn, Y. A. W. de Kort, C. Midden, B. Eggen, and E. van den Hoven (Berlin, Heidelberg: Springer), 171–182. doi: 10.1007/11755494_25
Berdichevsky, D., and Neuenschwander, E. (1999). Toward an ethics of persuasive technology. Commun. ACM 42, 51–58. doi: 10.1145/301353.301410
Borgefalk, G., and de Leon, N. (2019). “The ethics of persuasive technologies in pervasive industry platforms: the need for a robust management and governance framework,” in Persuasive Technology: Development of Persuasive and Behavior Change Support Systems, eds H. Oinas-Kukkonen, K. T. Win, E. Karapanos, P. Karppinen, and E. Kyza (Cham: Springer), 156–167. doi: 10.1007/978-3-030-17287-9_13
Brignull, H. (2013). Dark patterns: inside the interfaces designed to trick you. The Verge, Aug. 29, 2013. Available online at: https://www.theverge.com/2013/8/29/4640308/dark-patterns-inside-the-interfaces-designed-to-trick-you (accessed January 04, 2022).
Canale, N., Vieno, A., Santinello, M., Chieco, F., and Andriolo, S. (2015). The efficacy of computerized alcohol intervention tailored to drinking motives among college students: a quasi-experimental pilot study. Am. J. Drug Alcohol Abuse 41, 183–187. doi: 10.3109/00952990.2014.991022
CDC (2021). “All About Adult BMI,” Centers for Disease Control and Prevention, August 27, 2021. Available online at: https://www.cdc.gov/healthyweight/assessing/bmi/adult_bmi/index.html (accessed February 11, 2022).
CDC (2022). “Healthy Weight Loss,” Centers for Disease Control and Prevention, January 24, 2022. Available online at: https://www.cdc.gov/healthyweight/losing_weight/index.html (accessed February 11, 2022).
Cialdini, R. (2001). The science of persuasion. Sci. Am. 284, 76–81. doi: 10.1038/scientificamerican0201-76
Cugelman, B., Thelwall, M., and Dawes, P. (2011). Online interventions for social marketing health behavior change campaigns: a meta-analysis of psychological architectures and adherence factors. J. Med. Internet Res. 13, e17. doi: 10.2196/jmir.1367
Daudén Roquet, C., and Sas, C. (2018). “Evaluating mindfulness meditation apps,” in Extended Abstracts of the 2018 CHI Conference on Human Factors in Computing Systems (Montreal QC), 1–6. doi: 10.1145/3170427.3188616
Davis, J. (2009). “Design methods for ethical persuasive computing,” in Proceedings of the 4th International Conference on Persuasive Technology - Persuasive '09 (Claremont, CA), 1. doi: 10.1145/1541948.1541957
Davison, K. K., and Deane, G. D. (2010). The consequence of encouraging girls to be active for weight loss. Soc. Sci. Med. 70, 518–525. doi: 10.1016/j.socscimed.2009.10.061
Deloitte Access Economics (2020). The Social and Economic Cost of Eating Disorders in the United States of America: A Report for the Strategic Training Initiative for the Prevention of Eating Disorders and the Academy for Eating Disorders. Available online at: https://www.hsph.harvard.edu/striped/report-economic-costs-of-eating-disorders/ (accessed September 21, 2023).
Eikey, E. V. (2021). Effects of diet and fitness apps on eating disorder behaviours: qualitative study. BJPsych Open 7, e176. doi: 10.1192/bjo.2021.1011
Espinoza, G. E. T., and Baranauskas, M. C. C. (2020). “Motivation, persuasion and healthy eating: a case study on a socially-aware persuasive system design,” in Proceedings of the 19th Brazilian Symposium on Human Factors in Computing Systems (Diamantina), 1–10. doi: 10.1145/3424953.3426475
Filippou, J., Cheong, C., and Cheong, F. (2016). Combining the fogg behavioural model and hook model to design features in a persuasive app to improve study habits. arXiv. [preprint]. doi: 10.48550/arXiv.1606.03531
Fishbach, A., and Choi, J. (2012). When thinking about goals undermines goal pursuit. Organ. Behav. Hum. Decis. Process. 118, 99–107. doi: 10.1016/j.obhdp.2012.02.003
Fogg, B. J. (1998). “Persuasive computers: perspectives and research directions,” in Proceedings of the SIGCHI Conference on Human Factors in Computing Systems (CHI '98) (New York, NY: ACM Press/Addison-Wesley Publishing Co.), 225–232. doi: 10.1145/274644.274677
Fogg, B. J. (2003). Persuasive Technology: Using Computers to Change What We Think and Do. San Francisco, CA: Morgan Kaufmann Publishers Inc. doi: 10.1145/764008.763957
Galbraith, C. S., Ehrlich, S. B., and DeNoble, A. F. (2006). Predicting technology success: identifying key predictors and assessing expert evaluation for advanced technologies. J. Technol. Transfer 31, 673–684. doi: 10.1007/s10961-006-0022-8
Grand View Research (2023). Weight Management Market Size, Share and Trends Analysis Report by Function (Diet, Fitness Equipment, Surgical Equipment, Services), By Region (APAC, North America) and Segment Forecasts, 2022 – 2030. Available online at: https://www.grandviewresearch.com/industry-analysis/weight-management-market (accessed April 28, 2023).
Gray, C. M., Kou, Y., Battles, B., Hoggatt, J., and Toombs, A. L. (2018). “The dark (patterns) side of UX design,” in Proceedings of the 2018 CHI Conference on Human Factors in Computing Systems (New York, NY), 1–14. doi: 10.1145/3173574.3174108
Harjumaa, M., and Oinas-Kukkonen, H. (2007). “An analysis of the persuasiveness of smoking cessation web sites,” in Proceedings of the Second International Symposium on Medical Information and Communication Technology (Oulu: CWC), 1–14.
Honary, M., Bell, B. T., Clinch, S., Wild, S. E., and McNaney, R. (2019). Understanding the role of healthy eating and fitness mobile apps in the formation of maladaptive eating and exercise behaviors in young people. JMIR Mhealth Uhealth 7, e14239. doi: 10.2196/14239
Humphreys, S. (2010). S. The unethical use of BMI in contemporary general practice. Br. J. Gen. Pract. 60, 696–697. doi: 10.3399/bjgp10X515548
Kaptein, M., De Ruyter, B., Markopoulos, P., and Aarts, E. (2012). Adaptive persuasive systems: a study of tailored persuasive text messages to reduce snacking. ACM Trans. Interact. Intell. Syst. 2, 1–25. doi: 10.1145/2209310.2209313
Klein, P., Lawn, S., Tsourtos, G., and van Agteren, J. (2019). Tailoring of a smartphone smoking cessation app (Kick.it) for serious mental illness populations: qualitative study. JMIR Hum. Factors 6, e14023. doi: 10.2196/14023
Levinson, C. A., Fewell, L., and Brosof, L. C. (2017). My fitness pal calorie tracker usage in the eating disorders. Eat. Behav. 27, 14–16. doi: 10.1016/j.eatbeh.2017.08.003
Linehan, C., Harrer, S., Kirman, B., Lawson, S., and Carter, M. (2015). “Games against health: a player-centered design philosophy,” in Proceedings of the 33rd Annual ACM Conference Extended Abstracts on Human Factors in Computing Systems, Seoul Republic of Korea (New York, NY: ACM), 589–600. doi: 10.1145/2702613.2732514
Lockton, D. (2013). Design with Intent: A Design Pattern Toolkit for Environmental and Social Behaviour Change [PhD Thesis] Brunel University School of Engineering and Design. Available online at: http://bura.brunel.ac.uk/handle/2438/7546 (accessed February 07, 2022).
Lupton, D. (2014). Self-Tracking Modes: Reflexive Self-Monitoring and Data Practices. Rochester, NY: Social Science Research Network, SSRN Scholarly Paper ID 2483549. doi: 10.2139/ssrn.2483549
Maier, M., and Harr, R. (2020). Dark design patterns : an end-user perspective. Hum. Technol. 16, 170–199. doi: 10.17011/ht/urn.202008245641
McCabe, M. P., Busija, L., Fuller-Tyszkiewicz, M., Ricciardelli, L., Mellor, D., Mussap, A., et al. (2015). Sociocultural influences on strategies to lose weight, gain weight, increase muscles among ten cultural groups. Body Image 12, 108–114. doi: 10.1016/j.bodyim.2014.10.008
Neustaedter, C., and Sengers, P. (2012). “Autobiographical design in HCI research: designing and learning through use-it-yourself,” in DIS '12: Proceedings of the Designing Interactive Systems Conference (New York, NY: ACM), 10. doi: 10.1145/2317956.2318034
Norman, D. (1998). The Psychology Of Everyday Things. Available online at: https://www.bookdepository.com/The-Psychology-Of-Everyday-Things-Don-Norman/9780465067091?redirected=trueandutm_medium=Googleandutm_campaign=Base4andutm_source=PTandutm_content=The-Psychology-Of-Everyday-ThingsandselectCurrency=EURandw=AF7DAU9S572HDNA8V3T0andgclid=Cj0KCQiAr5iQBhCsARIsAPcwROM64vfRV5D7aGMtqytT0Mb4W38gb_Tqhd9OnkKrNWp5VfuC8onDzTwaAndvEALw_wcB (accessed February 11, 2022).
Nyström, T., and Stibe, A. (2020). “When persuasive technology gets dark?” in Lecture Notes in Computer Science, eds M. Themistocleous, M. Papadaki, and M. M. Kamal (Cham: Springer), 331–345. doi: 10.1007/978-3-030-63396-7_22
Orji, R., and Moffatt, K. (2018). Persuasive technology for health and wellness: state-of-the-art and emerging trends. Health Inform. J. 24, 66–91. doi: 10.1177/1460458216650979
Osman, M., McLachlan, S., Fenton, N., Neil, M., Löfstedt, R., Meder, B., et al. (2020). Learning from behavioural changes that fail. Trends Cogn. Sci. 24, 969–980. doi: 10.1016/j.tics.2020.09.009
Petersen, J. E., Shunturov, V., Janda, K., Platt, G., and Weinberger, K. (2007). Dormitory residents reduce electricity consumption when exposed to real-time visual feedback and incentives. Int. J. Sustain. High. Educ. 8, 16–33. doi: 10.1108/14676370710717562
Rieman, J., Franzke, M., and Redmiles, D. (1995). “Usability evaluation with the cognitive walkthrough,” in Conference companion on Human factors in computing systems - CHI '95 (Denver, CO: ACM), 387–388. doi: 10.1145/223355.223735
Schnall, R., Bakken, S., Rojas, M., Travers, J., and Carballo-Dieguez, A. (2015). mHealth technology as a persuasive tool for treatment, care and management of persons living with HIV. AIDS Behav. 19 (Suppl 2), 81–89. doi: 10.1007/s10461-014-0984-8
Schoeppe, S., Alley, S., Rebar, A. L., Hayman, M., Bray, N. A., Van Lippevelde, W., et al. (2017). Apps to improve diet, physical activity and sedentary behaviour in children and adolescents: a review of quality, features and behaviour change techniques. Int. J. Behav. Nutr. Phys. Act. 14, 83. doi: 10.1186/s12966-017-0538-3
Simpson, C. C., and Mazzeo, S. E. (2017). Calorie counting and fitness tracking technology: associations with eating disorder symptomatology. Eat. Behav. 26, 89–92. doi: 10.1016/j.eatbeh.2017.02.002
Stibe, A., and Cugelman, B. (2016). “Persuasive backfiring: when behavior change interventions trigger unintended negative outcomes,” in Lecture Notes in Computer Science, eds A. Meschtscherjakov, B. De Ruyter, V. Fuchsberger, M. Murer, and M. Tscheligi, Vol. 9638 (Cham: Springer). doi: 10.1007/978-3-319-31510-2_6
Tomiyama, A., Hunger, J., Nguyen-Cuu, J., and Wells, C. (2016). Misclassification of cardiometabolic health when using body mass index categories in NHANES 2005–2012. Int. J. Obes. 40, 883–886. doi: 10.1038/ijo.2016.17
Tondello, G. F., Kappen, D. L., Mekler, E. D., Ganaba, M., and Nacke, L. E. (2016). “Heuristic evaluation for gameful design,” in Proceedings of the 2016 Annual Symposium on Computer-Human Interaction in Play Companion Extended Abstracts (Austin, TX: ACM), 315–323. doi: 10.1145/2968120.2987729
Webb, T. L., Joseph, J., Yardley, L., and Michie, S. (2010). Using the internet to promote health behavior change: a systematic review and meta-analysis of the impact of theoretical basis, use of behavior change techniques, and mode of delivery on efficacy. J. Med. Internet Res. 12, e4. doi: 10.2196/jmir.1376
World Health Organization (2021). Obesity and Overweight. Available online at: https://www.who.int/news-room/fact-sheets/detail/obesity-and-overweight (accessed February 10, 2022).
Zagal, J., Björk, S., and Lewis, C. (2013). Dark patterns in the design of games. Foundations of Digital Games Conference, FDG 2013, May 14–17, Chania, Greece. Available online at: http://www.fdg2013.org/program/papers/paper06_zagal_etal.pdf (accessed September 21, 2023).
Keywords: design, persuasive systems, weight loss apps, weight loss tracking apps, unintended consequences
Citation: Harr R and Öhlund L (2023) Congratulations! You are severely underweight—Exploring negative consequences of persuasive system design. Front. Comput. Sci. 5:1215588. doi: 10.3389/fcomp.2023.1215588
Received: 02 May 2023; Accepted: 15 September 2023;
Published: 29 September 2023.
Edited by:
Gerrit C. Van Der Veer, University of Twente, NetherlandsReviewed by:
Elizabeth A. Boyle, University of the West of Scotland, United KingdomIvan Miguel Pires, Universidade da Beira Interior, Portugal
Copyright © 2023 Harr and Öhlund. This is an open-access article distributed under the terms of the Creative Commons Attribution License (CC BY). The use, distribution or reproduction in other forums is permitted, provided the original author(s) and the copyright owner(s) are credited and that the original publication in this journal is cited, in accordance with accepted academic practice. No use, distribution or reproduction is permitted which does not comply with these terms.
*Correspondence: Rikard Harr, rikard.harr@umu.se