- Cognitive Science, University of California, San Diego, La Jolla, CA, United States
In this article, I consider how scientific theories may explain architectural atmosphere. Architects use atmosphere to refer to a holistic, emergent property of a space that partly determines the mood of inhabitants. It is said to be a “subtle, intangible, ambient quality of a place” that also significantly shapes the way we interact with a space. It is caused by the way light, texture, materials, layout, geometry, acoustics, smell, and other perceptual properties influence affect. But it goes beyond these individually because of non-linear interactions between them. In sections one and two, I explain what an externalist account of the atmosphere would look like. This is an interpretation that objectifies the atmosphere, treating it as a complex causal property of buildings and spaces, accessible to scientific study through ethnographic research, through quantifying and minutely observing and recording humans and the buildings they are in, and then using machine learning and statistical analyses to identify correlations. The goal is to push the identification of the underlying external attributes as far as possible, ultimately to where a machine might enter a room, move around, and then label its atmosphere. In section three, I explore an internalist or subjectivist account of the atmosphere. This is the position that pushes back on machine identification of atmospheres. A subjectivist interpretation is harder to study scientifically because it involves knowing so much about the inner state and the history of a person. Culture, incoming mood, prior experience and associations, interests, tasks, social interaction, and more may all affect mood. Section four explores the frequently underestimated role—on emotion and space comprehension—played by the tasks that occupants perform while in a space, and the way their surrounding social and technological context intrudes on their encounter. I introduce and defend the view that tasks, social context, and nearby technology situate a person in a different environment than when they are inactive. This complicates the search for atmosphere. Nonetheless, I end on an optimistic note that there may yet be a place for atmosphere in the neuroscience of architecture, but it will be much different than our current thinking.
I believe that the inner quality of a place has a physical presence, and that this presence can be experienced and recognized.
Peter Zumthor
Atmospheres are totalities: atmospheres imbue everything, they tinge the whole of the world or a view, they bathe everything in a certain light, unify a diversity of impressions in a single emotive state.
Gernot Böhme(Böhme, 2013).
In this article, I consider how scientific theories may explain one of the more intractable concepts in architecture: atmosphere.
Backstory. Before launching this study, I interviewed over a dozen architects to understand their views on architectural atmosphere. Their interpretations were broad and vague leaving me skeptical about finding a place for atmosphere in a sober science of architecture. It is not that scientists doubt that architecture influences mood. The real question is: after accounting for the way light, texture, materials, layout, geometry, acoustics, smell, and other perceptual properties each influence affect, what is left to explain? The atmosphere seems to be an extra ill-defined property of space, and an overly subjective one, at that.
The literature on the impact of sensory and geometric properties on mood—the initial focus of a scientific theory of atmosphere—is disappointing. It is both coarse and substantially reiterates conventional architectural wisdom. Typical studies provide gross statistical measures that show, for example, that natural light increases positive emotions, reduces stress and depression, and improves overall wellbeing, whereas artificial light is more associated with negative emotions, stress, depression, and poor sleep quality (Boubekri et al., 2014). These are good results to establish empirically; but they are hardly unexpected, and more importantly, they are far removed from the concerns of lighting designers who work with light three-dimensionally, looking for strategic placements to shape the atmosphere. The same applies to sleep research. To learn which wavelengths facilitate sleep and which inhibit sleep is genuinely important (Lunn et al., 2017); but it is not nuanced enough for lighting design that is complex and many dimensional. Because good lighting design is a major component in creating atmosphere, we need to push this sort of research much farther if we are to explain mood.
Moreover, how do the many dimensions of light, sound, smell, texture, geometry, etc., interact? If there is one reason to think architectural atmosphere is a separate, holistic, or emergent property of the various sensory properties of a space, it is that all these factors interact and collectively impact an occupant's feelings in a non-linear manner. A change in smell can immediately change an atmosphere. Think of how the inviting aroma of baking bread can change your experience of a greasy diner. A change in sound may reverse that change—such as hearing people begging for bread. Even more striking, by altering acoustics—and not just changing the sound of social activity—a dull space may be transformed, becoming magical, potentially inducing a feeling of awe (Valenzuela et al., 2020). Clearly, we need a more holistic classification of the effect of environments on occupants. The notions of holism and emergence are a good reason to keep an open mind to the reality of a high-level property such as atmosphere.
Balancing what architects think and what science can explore is my goal. Here is an example of the challenge.
In his books “Thinking Architecture” (Zumthor, 2010) and “Atmospheres: Architectural Environments, Surrounding Objects” (Zumthor, 2006), Peter Zumthor argues that atmosphere is an essential element of architecture with the power to deeply affect the way people feel and experience a building. He believes that a building's atmosphere is created by the interplay among its materials, forms, and spaces, as well as the light, sound, temperature, humidity, and other sensory elements present. It has a complex multi-sensory basis, but it goes beyond perception, he suggests. It is registered or sensed, but not literally perceived. An atmosphere, he states, is a “subtle, intangible, ambient quality of a place” that significantly shapes the way we interact with a space. It typically gives rise to a sense of comfort, tension, or other emotional feelings; it can influence the way people socially interact; and it explains why some spaces have meaning or purpose for some people, as is often the case with meditation halls, churches, or grade schools. This is a tall order for a single construct.
Furthermore, an atmosphere can be effective whether we are aware of it or not, or whether we are aware that our mood is being altered. And we can be affected in idiosyncratic ways. The exact psychological effects an atmosphere has on a person is sensitive to their culture, training, personal associations, memories, interests, and the specific context in which a building is being used, such as for a ceremony or boring meeting. Even the other people present in a space can significantly influence the emotions and response of an individual, whether we are with a loved one, part of a rowdy crowd, or too close to someone we detest. What our goals, activities, and tasks are, also significantly influence our reception of the atmosphere; consider how different the same space will feel when on a romantic date vs. playing a wild game of hide and seek. How are we to analyze these contributing or confounding factors?
And then there are the words that architects use to describe an atmosphere: warm, intimate, dynamic, energetic, creepy, gloomy, organic, relentless, and festive. The list is much larger. Norberg-Schulz (1984) in describing the Chartres Cathedral, uses “light,” “transcendent,” and “sacred”. He refers to the space as being “luminous” and the play of light as creating a sense of “mystery” and “grandeur”. To describe the Parthenon, he uses words such as “majestic,” “grand,” “imposing,” and “serene”. Johanni Pallasmaa frequently uses words such as “sensual,” “tactile,” and “haptic” to describe the atmospheres created by the buildings of Finnish architect Alvar Aalto, which he argues are designed to engage the senses in a holistic way (Böhme et al., 2014). Zumthor speaks of buildings feeling “intimate,” “meditative,” “monumental,” or “mysterious.” Are these not personal judgments of the space—descriptive terms rather than terms that convey an unambiguous feeling or affect value? What is a feeling? What is an atmosphere?
As cognitive and neuroscience advance, our goal is that concepts like atmosphere will be given a scientific interpretation or else be shown to be imprecise but nonetheless pointing at definable properties whose emotional effect on people can be explained. Perhaps we will need to define micro-atmospheres or transient atmospheres to recognize the sensitivity of a structure's atmosphere to where a person is in that space, or the time of day or the activities happening simultaneously.
The method and approaches I discuss reflect my preconceptions of what a cognitive and neuroscience of architecture may look like; one that emphasizes that people experience buildings and atmospheres enactively through their interaction and biased interests.
For this study, I assume that:
• An architectural atmosphere is a general mood induced by a space (Zumthor, 2006; Arbib et al., 2023).1, 2 It is an important aspect of design, and it plays an important role in creating the overall experience for visitors and occupants when in a space.
• Perceiving subjects are never stationary; atmospheres are probed, felt, and experienced, not just through eye movements, and strategies of listening and smelling, but through bodily involvement with the 3D space and surfaces nearby. We encounter an atmosphere through trajectories of perceptual, motoric, and cognitive-affective engagements; all our senses are involved including sensory contact that we are unaware of.
• Each perceiving subject has its own history, inner biases, and cognitive-affective apparatus. Two people never encounter an environment in exactly the same way. We enactively engage a space in our individual ways.
• We cannot pretend that people have no tasks or interests when in a space. These concerns change the very environment they are in. The atmosphere may change depending on what a person is doing, where they are in a space, and their trajectory getting there.
Given these assumptions, it is tempting to say that an atmosphere is co-constituted: it neither resides completely in the external environment—the externalist view—nor in the internals of the agent—the internalist view. It emerges through encounters and interaction. I accept this. It is probably the dominant view among careful proponents (Böhme, 2005). Importantly, though, this view also emphasizes that sensing an atmosphere is significantly different than perceiving color or perceiving that a person is in pain; both are complex judgments that seem to have subjective and objective components and so might be proposed as analogies.
When a person judges that a surface is red, the best theory explains this by considering more things than the wavelength of light emanating from the surface. The color of nearby surfaces, the brightness of the surrounding light, and even the behavioral context can all affect color perception. On some theories, language and culture matter too (Gilbert et al., 2006).3 So, it looks like color perception relies on external and internal attributes. The same applies to the ability to decide if someone is in pain. We all have a good idea of the external processes or events that can cause pain, but culture, gender, age, and social context also matter when judging how much pain someone is in. Despite these complexities, it is plausible to suggest that the perception of another's pain and the theory that explains color vision can be explained in a reductionist manner without heavily relying on knowing much about the unique traits of individual people. With care, we can identify the complex relations between external attributes—light and light context for color, behavior, and cultural context for pain—that will allow normal observing subjects to judge color and to decide whether others are in pain, in normal circumstances. And we can define normality. For greater precision, we can even define what is normal for people in different cultures.
The result: We believe we can train a machine to recognize color and attribute pain observationally.
By contrast, sensing an atmosphere, even an atmosphere we define as an external attribute of the world, will be much more complex and ultimately need to refer to and explain the inner processes of the sensing subject. Machines will have to know much about the subjects in the room—including their interests, tasks, and social context—before they can reliably infer the atmosphere they experience.4 Defining who is normal and what normal circumstances are is far more complex for atmosphere than pain.
I explore the topic of atmosphere in six sections.
In section one, I explain what I mean by an externalist account of the atmosphere. This is an interpretation that objectifies the atmosphere, treating it as a complex causal property of buildings and spaces, accessible to scientific study through ethnographic research, through quantifying and minutely observing and recording humans and the buildings they are in, and then using machine learning and statistical analyses to identify correlations.
The resultant theory would associate atmospheric judgments and human psychological and physiological reactions with the architectural elements that “induce” them. Given that the notion of atmosphere is linked to the moods and emotions it provokes, an externalist theory must also incorporate a method to gauge people's emotional state as they enter and engage with a space. The goal is to push the identification of the underlying casually relevant external features as far as possible, to the point where a machine might enter a room, move around, and then label the atmosphere a given person would feel.
In section two, I give a list of current methods that could be used to identify feature clouds, methods to label them, and methods to assign them an affect value. Feature clouds are one way of defining the objective basis of an atmosphere; affect maps are one way of explaining their emotional impact.
In section three, I explore an internalist or subjectivist account of the atmosphere. This is the position that pushes back on machine identification of atmospheres. A subjectivist interpretation is harder to study scientifically because it involves knowing so much about the inner state and the history of a person. It also requires a worked-out theory of how neuro-cognitive and neuro-affective systems figure in atmosphere processing. It is why the field of human building experience is in its infancy. It nicely shows, however, one sort of theory that a mature neuroscience for architecture should aim for—a theory of human cognition of atmospheres, how buildings engender affective responses in humans (and perhaps animals) as a function of internal state and external features.
In section four, I explore the frequently underestimated role—both on emotion and on space comprehension—played by the tasks that occupants perform while in a space, and the way their surrounding social and technological context intrudes on their encounter. Most of our life is mediated by our tasks, our social relations, and our technology. They strongly determine what we attend to, what we interact with, and what we recall. We cannot have a theory of atmosphere that ignores how influential the role of tasks, activities, and social interaction is in atmosphere encounter. This inevitably expands the theory to include a discussion of task cognition and social interaction, and their effect on emotion and perception. Obviously, what a person is doing at the time of measurement is one of the biggest determinants of their affective state. Consider how the emotions raised by a heated argument swamp the meditative states “supposed” to be induced by a Zen teahouse. Or how the mental act of concentrating on a crossword puzzle may be the dominant factor in one's momentary feelings despite sitting in a noisy cafe. It matters what we do in a space. Since mood is more like a moving average of emotional states, the more a person is engaged in a fluctuating activity, the more their mood is dependent on what they spend most of their time doing.
One provocative line of thought is that tasks, social context, and nearby technology may actually situate a person in a different environment or a psychologically different space than when they are inactive. When they are inactive, their brain's default mode network is dominant (Raichle, 2015)5, the opposite is true when they are focused on an outside-world task. Too often people assume that an atmosphere is connected to the feeling one has when entering a space rather than inhabiting it. This bizarre elevation of first impressions over dwelling and inhabiting often distorts thinking about atmosphere.
In the fifth section, I present several analogies to illustrate how we might conceptualize atmosphere, given the numerous factors and confounding variables that contribute to its complexity.
In section six, I delve into what this means for designers. Can we reasonably expect the emergence of an atmosphere plugin anytime soon?
1. Externalist accounts of atmosphere
To get at the scientifically accessible core of atmosphere, I begin with an externalist's assumption that an atmosphere is an objective something in the world, and it can be designed. If such a design is properly implemented, then a “normal” or “ideal” human agent will feel the intended atmosphere. I say “feel” because atmospheres, we are told, are not perceived. They are something that human occupants can pick up, attune to (Pérez-Gómez, 2016), register (McCormack, 2008)6, or “sense” (Pallasmaa, 2011)7, and they consist of a complex of discernable properties that causes people to feel specific emotions, or to color their mood in a definite manner.
Few champions of atmosphere advocate for a uniquely externalist view. Virtually everyone agrees that people often differ in their reaction to environmental ambience and that what one person calls serene, another might call boring or uninteresting, or a third might be swamped by personal associations and have a completely different cognitive-affective response. Furthermore, there is no proper scientific notion of a “normal” or “ideal” agent, as I initially suggested, because normal agents have personal life histories that differentiate them. A visitor to a monastery will surely experience that space differently than a resident monk. Both are normal.
Yet, something must be generalizable. There must be some link between external features and emotion or mood state, else why would architects be so confident that the atmospheres they say they are creating are what occupants actually register? Unless they are just wrong.
The purest form of externalism, for argument's sake, is that there is a physical quality—albeit immensely complex—shared by every instance of a given type of atmosphere. There are external factors that make an atmosphere warm or intimidating, calming or energizing, cozy, inspiring, majestic, or serene. Perhaps for a given type of atmosphere, each instance shares a family resemblance (as all games do) with the others, or perhaps they share an invariant but hard-to-specify relational property (as all human faces do). Regardless of the exact nature of the connection, for an externalist, some well-defined similarity function, based on environmental features, must exist.
Take serene as an example: a theory of serene atmospheres will explain why the material cause of the feeling or mood of serenity as felt in one room is relevantly similar to the material cause of all other examples of serene atmospheres, no matter how different superficially those other rooms are. Consider a serene teahouse and a serene bedroom. Not everyone must feel serene when in a room that some people call serene. But many will. Our theory must allow different people to feel different things because a given physical space may map into many moods—perhaps the way an ambiguous figure maps into multiple interpretations. Rooms may be atmospherically ambiguous; they are registered differently by some people, or even by the same person at different times (see Figures 1A, B).
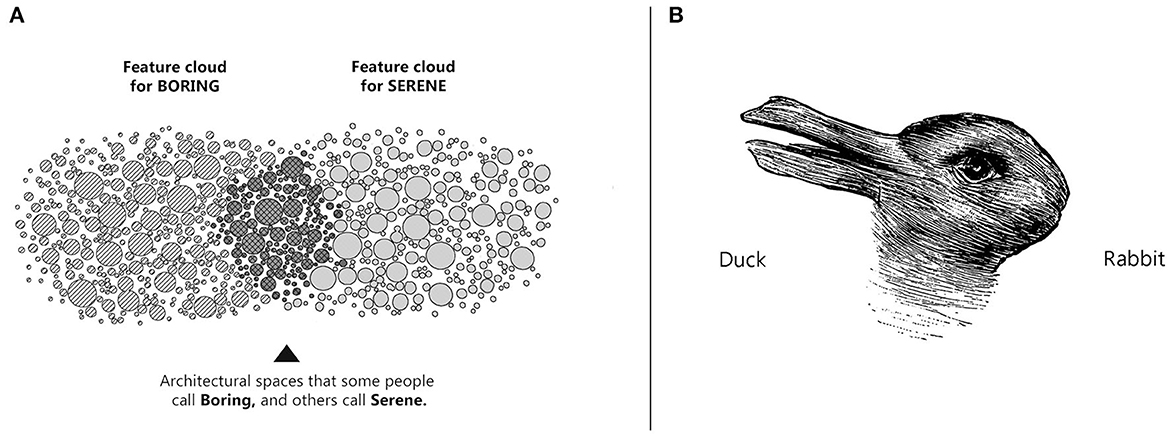
Figure 1. In (A), the left cloud represents the feature space that corresponds to the many rooms, spaces, and buildings that some people call boring. The right cloud represents the feature space of the spaces some people call serene. Some of the same spaces are called boring by some and serene by others. These can be treated as ambiguous, or caused by individual differences, or by context, in much the way the drawing in (B) can be seen as a duck by some and a rabbit by others, or a duck at some moments and a rabbit at others.
Mathematically, this means that the relation between mood and physical space is a many-many relation. Many different rooms may have the same atmosphere; and the same room may present itself as many different atmospheres. Still, we assume one fundamental constraint. Two distinct atmospheres cannot evoke identical affective states in the same person because there is a semantic connection between atmosphere and mood. If a person feels serene in a room at time t1, then although she can feel anxious in that same room at another time t2, it will be because the atmosphere that she registers at t2 is different. If her feeling changes, then the atmosphere she registers must change. The same room can cause more than one atmosphere, but not simultaneously to the same person.
What follows now is a description, at a high level, of how to define and identify atmospheres using a mix of quantitative and qualitative methods.
1.1. Feature space
An atmosphere, understood from a big data approach, can be visualized as a cluster of points in a high-dimensional feature space (see Figure 2). Each point in the space represents the features of a particular room within an architectural typology plotted as values on feature dimensions such as light, sound, scent, materials, air quality, and so on. There will be dozens of such feature dimensions, probably hundreds or thousands. Each room or space might change over the course of a day, and if so, there will be multiple points for that room.
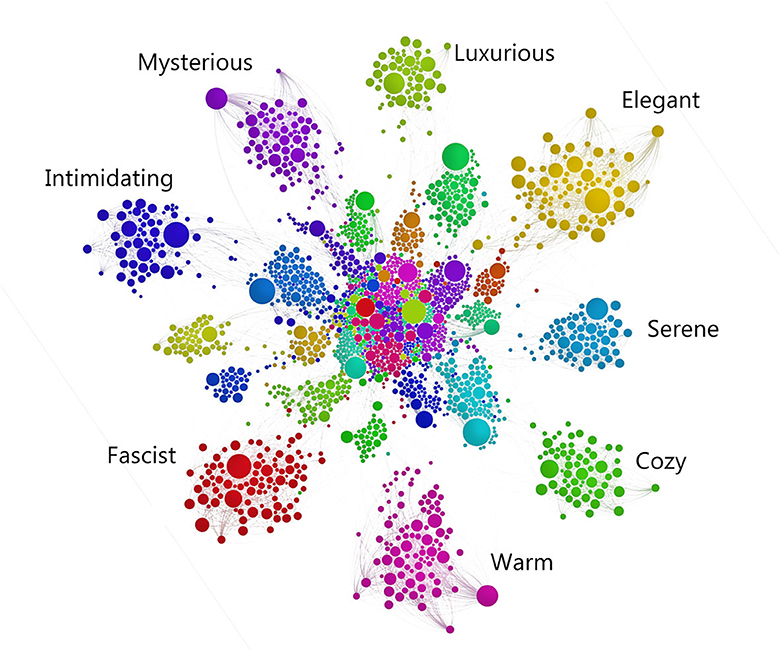
Figure 2. Clouds of points in feature space are labeled by the atmosphere they create. Each point represents a particular room or space. Large circles represent many rooms that are very similar, or perhaps the same room at different times. The clouds may overlap, but in a high-dimensional space, it is likely that there is substantial differentiation on some dimensions, so rooms that have much in common may also engender different moods because of other attributes, such as light, scent, materials, noise, and acoustics. Image credit: adapted from https://www.kaggle.com/code/minc33/visualizing-high-dimensional-clusters/notebook#Introduction.
If externalism is right about the physical basis of judgments of atmosphere, all the buildings or spaces we call cozy, regardless of typology, can be represented as points (vectors of feature values) forming a distinct cloud of relatively close neighbors in this space. It is likely that there are several such clouds because rooms, such as a den, may be cozy for different reasons than intimate rooms for prayer or for dining. It is also possible that rooms that are superficially quite different might be classified as cozy too. If that is so, we expect there to be a relational property they share at a deeper level—i.e., it is possible for a machine to recognize what cloud, what atmosphere, a given room or space belongs to, regardless of the type of room it is. If the feature space is large enough, each type of atmosphere should produce distinct point clouds (or small disjunctions of distinct point clouds). Buildings and rooms that people are inclined to call monumental will cluster in one cloud, buildings and rooms they call mysterious, institutional, intimidating, and so on will cluster in different clouds. What makes them all instances of one or another atmosphere can be expected to be some highly complex relational property of the features in each cluster.
An analogy can be found in face recognition. What makes the features of a face a human face is some complex spatial relation among noses, eyes, and mouths of the right sort. Modern face recognition systems dive into finer distinctions than the features we use in common sense. However, to a first approximation, we can think of a human face as a relational structure tying geometric and color features into a coherent whole—a human face. Monkeys, dogs, and other face types—including Neanderthals—will all be typed differently. Humans from different races and age groups will also be classifiable as sub-types of human faces. Similarly, cozy rooms, despite detailed differences noticeable in breakfast nooks, TV rooms, and bedrooms should still share a higher-order similarity sufficient to invoke a feeling of coziness regardless of room.
1.2. The problem of specifying dimensions
The deepest problem with a feature space view—aside from the huge amount of data we will need to populate it—is that there is no easily defended procedure for identifying the dimensions to use to compare rooms or spaces. What constitutes an architecturally significant feature—i.e., a feature that might make a difference to atmosphere registration? And what is the relevant granularity to specify those features? Should we describe the windows in a room as windows with size, shape, and wall location, the doorways as jams with mullions of a certain thickness? This is reminiscent of the original problem of specifying the relevant features of a face for facial recognition. We might start with common sense features. But we can be confident that they will be replaced by working directly with pixel intensities, or in the case of rooms, with feature intensities in voxels. A more likely approach for atmosphere recognition, then, is to go straight to a technical quantitative account, as was done with faces. In architecture, this means using fundamental dimensions about light, odor, haptics, and acoustics, all based on the best scientific theory of these and tied to voxels.
If a scientific account is pursued, the n-dimensional space must be expressive enough to include measures of all the properties that can affect people's sensory discriminations. Acousticians will be needed to gather in situ measures of acoustics. Psychophysicists will be required to measure haptic properties, scent, and more. The data will soon become immensely complex. For instance, on some accounts, people can discriminate a trillion scents (Bushdid et al., 2014; Richard and Jason, 2015)8 Most of this capacity can apparently be explained by using a 10-dimensional representation of odor perceptual space (Castro et al., 2013). To use these 10 dimensions, as with all other feature measures, the geometry of the physical architectural space will have to be represented. The simplest method would be to import a 3D model of the physical space. See Figure 3 for a space with complex geometry. Using a model for this space, we can show the gradients of smell at each point.
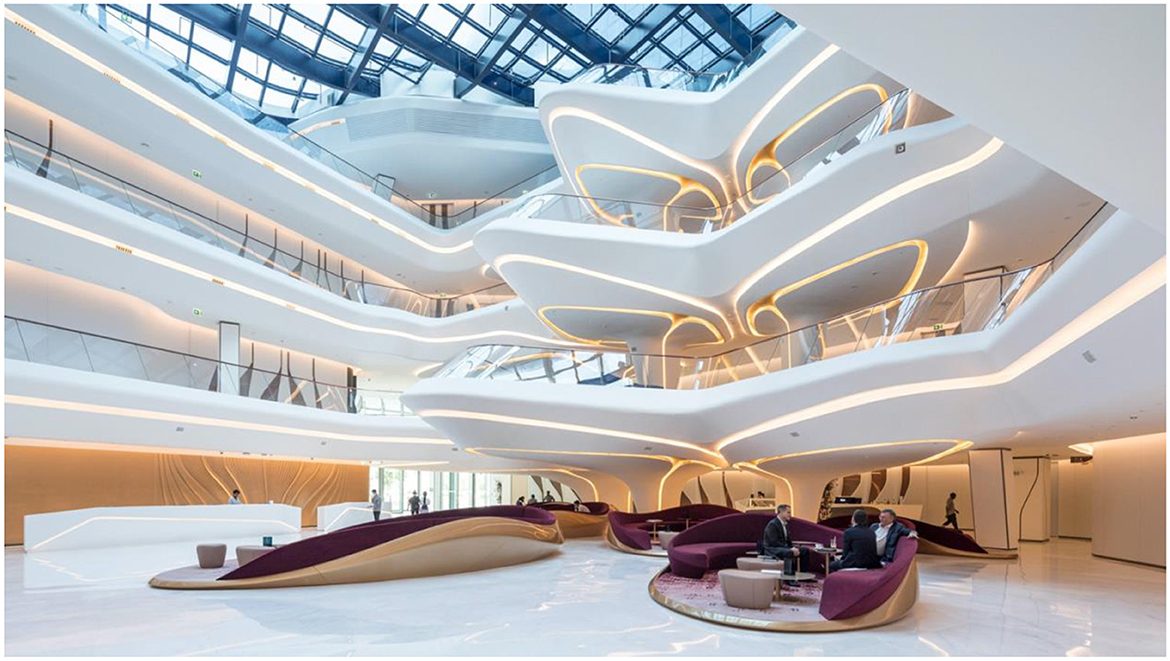
Figure 3. The interior of the ME Dubai hotel at the Opus by Zaha Hadid Architects contains parametric furniture and curving sculptural balconies. How is such an interior to be adequately characterized without reference to the parametric specification of surfaces? Our geometric model will have to be characterized mathematically. Image credit: https://www.zaha-hadid.com/architecture/opus/ by permission of ZHA.
Given such a 3D model, the next step is to choose the granularity at which to voxelize the model. For each voxel, the physical and sensory values would be entered as a vector. Most of these values may be inferred by using VR or metaverse-like assumptions that fill in the light, sound, and touch parameters in each voxel. See Figure 4 for an illustration of a voxelized shape. Wall voxels will have predictable haptic, light, and reflectance values, as well as solidity, but probably not taste or smell values. Space voxels will be treated as filled with air, odor gradients and wind. Common sense features such as doors, windows, and floor tiles will not be primitive features in this space. But they can be discovered through deep learning. They will be reducible to be complicated equivalence classes of light, texture, and geometry.
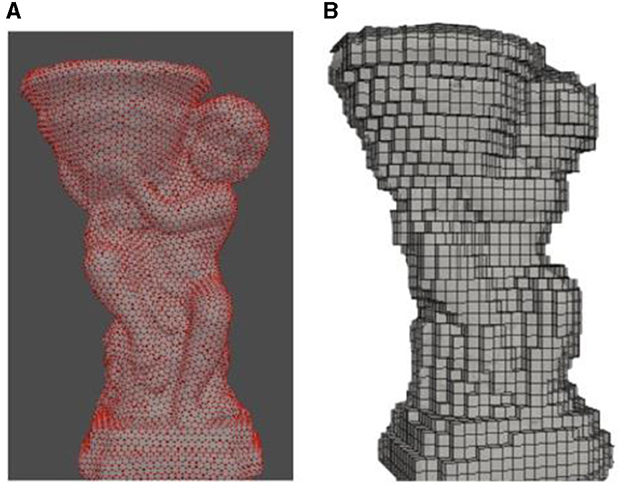
Figure 4. Two representations of shape are shown here. In (A), a mesh representation of an angel statue is mapped into a voxel representation, as shown in (B). To study the atmosphere in a room, we represent the surfaces and interior volume as a system of voxels. Each voxel contains a vector of values for each feature we have defined. Because humans move through space and their trajectory affects what they see, feel, and smell, a more complete model would also contain values for the path by which a voxel was entered or encountered adding further complexity to the model.
A partial list of the sort of physical dimensions that might be used initially to identify an atmosphere is itemized in Table 1.9 The list is a non-technical collection of physical dimensions and is woefully incomplete. Column one names the top-level physical dimensions—light, acoustics, scent, materials, etc.; column two shows their sub-dimensions: for light, these include the three properties of color (hue, value, and saturation), plus several others, such as natural vs. artificial;10 column three specifies the data type, numerical or qualitative—for acoustics, these are numbers for loudness, reverberation, and resonance. Column four indicates whether the dimension is discrete or continuous. This sort of approach is halfway between a commonsense feature approach akin to noses and eyebrows for faces and the full n-dimensional account based on voxels.
One important factor still needs to be added. Atmospheres are encountered over time, not all at once. This means that the trajectory of the encounter makes a difference. Action and enaction count. A person who moves through a space without attending to the “right” attributes, or in the right manner, may never perceive the features needed to trigger atmosphere identification. A space walked through from left to right may feel completely different than one walked through from right to left or walked through from right to left but backwards, as if in rewind mode. This might be addressed by defining trajectories or “feature streams” in voxel space. This will add dimensions such as time, position, gaze, and of course spatial position that can be simulated and collected. Each new dimension is a step toward greater verisimilitude of a subject's experience of the space. However, it greatly increases the dimensionality and intricacy of the model.
Because of this complexity, the idea of describing all the atmosphere-relevant features of a building as a vector in a comprehensible n-dimensional feature space is a gross idealization. The important characteristics that distinguish one atmosphere from another will have to be discovered by machine classification and the result is likely to be unintuitive, similar to how the mathematical equations that identify the holistic features of a human face, such as the angular distance between hundreds of pixel-sized shading intensities on nose, eyes, and mouth, end up being incomprehensible as an intuitive feature.
Hope remains though. The state of the art in facial recognition, as in other big data analyses based on machine learning, is to analyze the latent space of a learning network to isolate a collection of higher-level features that are more comprehensible to humans, such as hairline, hair style, and race (Voynov and Babenko, 2020; Kammoun et al., 2022). Success has been mixed. But the field is new, and expectations are running high. Accordingly, with a large enough dataset of rooms and atmospheres—and much of this can be generated through measurements first in a real space and then simulated in a digital twin—there is hope that: (i) the key determinants of the atmosphere may be found and (ii) prototypical atmospheres can be generated. For instance, cozy atmospheres, serene, majestic, etc.
1.3. Fundamental assumption
This optimistic prediction has a chance of being true only if human judgments of the atmosphere are consistent enough to provide a clear signal for each atmosphere. If there is too much variance in atmosphere judgment among people, then an externalist approach crumbles. It is to that we now turn.
2. Methods for specifying an atmosphere
In this section, I describe how to find a name for an atmosphere, how to identify its feature cloud, then how to specialize a named feature cloud according to the emotion sub-clouds generated. Measuring the affect—i.e., emotion—of people when in a space plays a central role in identifying feature clouds for atmospheres.
A scientific method for studying the atmosphere must cover three sub-methods:
• Explain how to determine an intuitive name for an atmosphere—one that has affective implications. Identify a word for the primary feeling of a space. Typically, such a name will incorporate an implicit appraisal of the space (cheerful, mysterious, intimidating, etc.), thereby going beyond the familiar words used to name emotions (angry, sad, exuberant, etc.).
• Identify the relevant dimensions of affect and explain how these can be reliably measured.
• When a person is in a distinct architectural atmosphere (of the sort we have given an intuitive name to) it is necessary to specify the physical features of that atmosphere and correlate it with the measured affective states.
2.1. Naming atmospheres: using word clouds
Words serve two functions in our analysis of the atmosphere.
• They provide labels for rooms, atmospheres, and feature clouds.
• They constitute prima facie evidence for the affective state subjects are in when they are in a room because words and phrases often encode an implicit judgment of affect.
Affect—whether called mood or emotion—is at the heart of the idea of atmosphere. But so are words for both the feeling and the sense of an atmosphere. To understand the atmosphere of a given situation, we first examine the words and replies people provide in response to questions. Field work is needed to collect these (Berthoz and Hill, 2005; Mauss and Robinson, 2009).11 Without appealing to the words that subjects use to tell us their impressions, we have no way of knowing what atmosphere they think they register.
Here is a method to identify words that can be used to label point clouds in feature space. Collect responses to questions and probes. Plot each expression in semantic space (Landauer and Dumais, 1997; Lowe, 2001). The space must be continuous enough to have a metric that permits clustering words into similarity groups—word clouds. What some people call cozy, others may call sentimental, maudlin, cluttered, lower-class, cloying, and oppressive. We can use closeness in semantic space to machine cluster all these different words into one or more word clouds. Once we have these semantic clusters, we need to name them in an intuitive manner. By computing the semantic center—the centroid—of each word cloud (cluster), we can look for the closest word in our language to that centroid and use that as the label for the cloud. We can also think of this semantic center as the prototypical value of a specific atmosphere.
This cloud of words, classified by machine learning as constituting a “natural” group, has a centroid close to the semantic value for “cozy.” Even though the location of that centroid is unlikely to be identical in meaning space to the location of the English word “cozy”, the word we will use to refer to that group will be “cozy”. Going forward, we use this word as a label for the atmosphere we asked people to give us names for. “Cozy” might be a good word to name an atmosphere that is warm, intimate, comfortable, safe, snug, and sheltered. Even if no one actually used “cozy” to describe any of the architectural spaces we showed them, the word cozy may still be the best term to use to name all the spaces where people say “I feel safe here,” “This is a warm space,” and “It's snug and intimate.”
Figure 5 displays a word cloud of two atmospheres, cozy and intimidating, each derived from a cluster of semantically related terms. The cloud is a useful visualization of how to describe an atmosphere, but it tells us nothing about the actual features of the rooms people were in when they used “cozy” or its cousins.
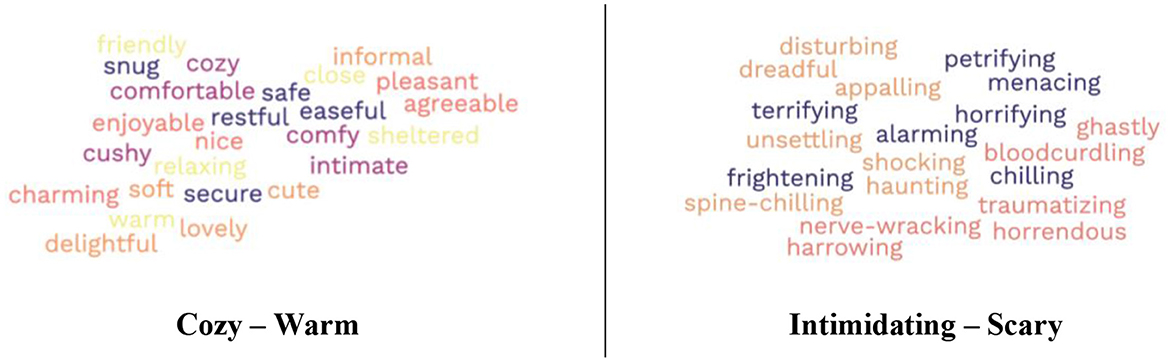
Figure 5. The terms used to describe cozy atmospheres and intimidating atmospheres are shown here as word clouds. With a little imagination, they can be understood as labeled points in a many-dimensional semantic space.
To identify the features of cozy spaces in an n-dimensional feature space, we now cluster the set of feature vectors whenever someone used a word or phrase in the cozy word cloud. This is the method used to label the feature space clouds in Figure 2. It is explicitly shown in Figure 6.
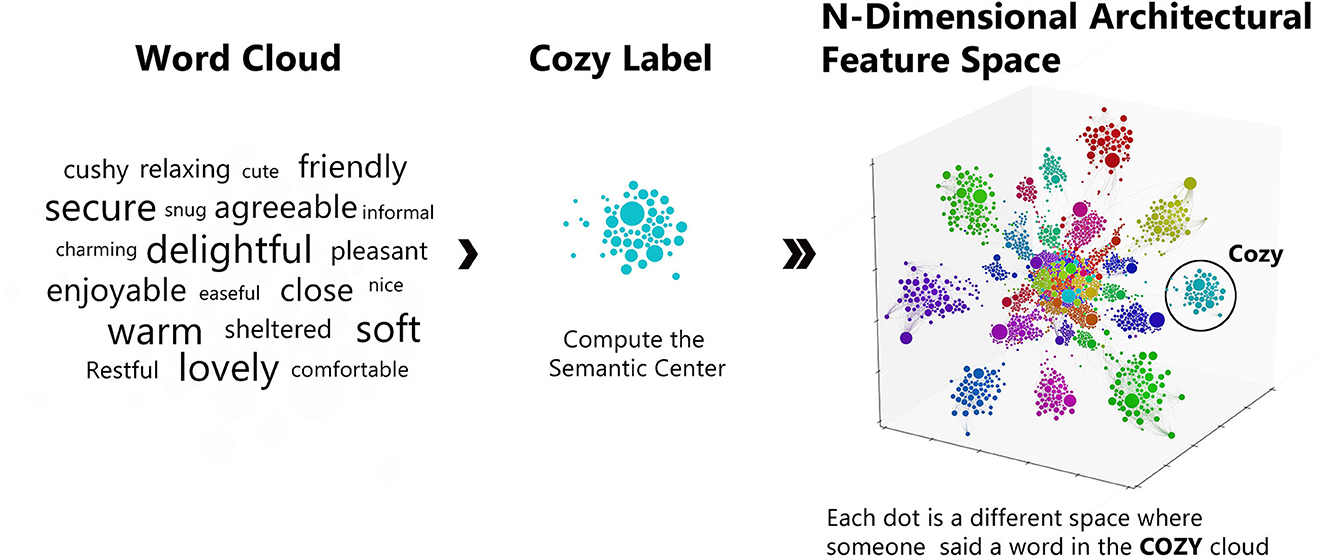
Figure 6. For any specific space or room that elicits from a subject, a word in the cozy word cloud will be plotted in the n-dimensional feature space. We assume that all these labeled points will make a cloud that a machine could eventually learn to classify. The cozy cloud defines a learnable set. Jointly, the set of cozy cognates demarcates the boundaries of cozy atmospheres.
One benefit of this approach is that it allows us to interpolate to other possible rooms (feature vectors) that are cozy even though no one has ever seen them or judged their atmosphere. If a person were to enter one of those rooms, we would predict that they would use the word “cozy” or one of its semantic cousins to name the atmosphere they feel.
2.2. Measuring affect
The next step is to narrow the feature clouds in Figure 6 by using a more precise measure of affect than those available in ordinary English words or phrases and to assign this more theoretical measure of emotion to each atmosphere's name. Thus we move from a commonsense classification based on words and simple phrases to a more technical classification based on more precise measures of affect. The strategy is to tighten the specification of what falls into an atmosphere feature cloud by using a scientific measure of affect collected from people when they are in “cozy” spaces.
For instance, according to our semantic cloud, rooms that are called “cute” belong in the cozy feature cloud. However, perhaps cute rooms reliably elicit a measured affect that is on average different than those called “cozy.” In that case, cute rooms should be separately labeled within the cozy feature cloud. “Cozy” may remain a coarse descriptor of a type of atmosphere, but as we gather more quantitative measures of affect, we gradually distinguish sub-clouds within it such as “cute,” “friendly,” and so on.
Currently, there are five main quantitative approaches to identifying a person's emotional or mood state (see Figure 7).
• Self-report and answers to questions,
• Speech modulation (intonation, volume, and prosody) when in a space,
• Facial expression,
• Body analysis (posture, gesture, and full body movement),
• Physiological measures such as skin temperature, electrodermal activity, HR and HRV, EEG, etc.
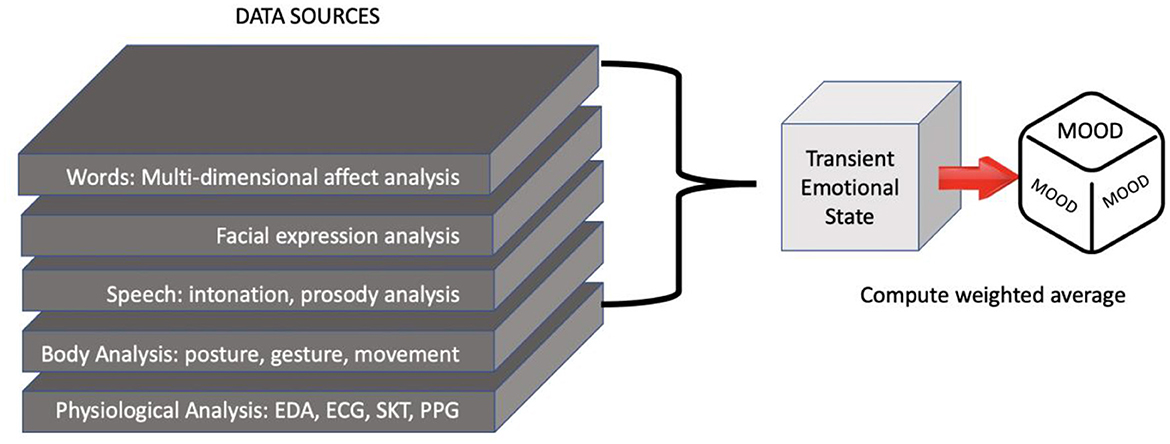
Figure 7. Five sources of data on emotion can be collected and used jointly to infer emotional state.
These five sources are collectively used to infer the momentary emotional state of a person. When collected over time, they can be used to compute a weighted average that some believe is what is meant by “mood.”
One open question, not addressed here, is how to differentially weigh the five sources. In part, this is a question about how to evaluate the implications of explicit vs. implicit sources of information about emotion. Words, for instance, are an explicit response to questions about the nature of the space. “Which of these two spaces is cozier, and why? Describe how they make you feel?” The remaining four measures, however, are all implicit measures where subjects are unaware of what their physiology and behavior reveal to experimenters about their emotional state. Mood is computationally inferred from a trajectory of emotional states.
What follows now is a brief discussion of each of these methods.
2.2.1. Affect derived from words
Multi-dimensional sentiment analysis is a technique that uses deep language processing to determine the overall sentiment of a piece of text as positive, negative, or neutral (Maas et al., 2011; Wang et al., 2016). This is done by identifying words, phrases, and sentence structures associated with specific emotions, using both lexicon-based approaches (which utilize pre-existing lists of words associated with different emotions) and machine-learned models trained on labeled text data (Boyd et al., 2022). It does not use the centroid of our word collection. It works with a database of associations between words/phrases and feelings. Sentiment analysis can be used to infer emotions such as happiness, sadness, anger, fear, surprise, and disgust and combinations of these, ultimately plotting an emotion almost anywhere on a two- or three-dimensional affect space.
The words for sentiment analysis come from directly asking someone in a room to describe the atmosphere they sense—i.e., what and how they feel about that room. The more words the better. Subjects are also asked about their current emotional state by using questionnaires, interviews, and rating scales. In a rating scale approach, participants indicate their level of agreement or disagreement with single prompt words or with phases. Another technique focuses more on ambiguity: subjects choose as many atmosphere or emotion words as they currently experience from a list.12 Finally, through open-ended questions, whole sentences can be collected and used to infer the word set best characterizing their sense of room atmosphere and their emotional state.
The value of word approaches, both for coarsely naming feature clouds with a single word and using words to identify associated emotions, is that we can get terms we understand. If we did not have words to name atmospheres, what would we think the atmosphere is? Even if we collected affect measures and mapped them into a two- or three-dimensional space, we still would be missing the idea of the atmosphere. A set of measures that indicated an angry emotion does not really tell us much about an atmosphere. Alternatively, if we collected pictures that people associate with the atmosphere of a room or space, we still need to specify what the set has in common. Without words, how are we to compare the pictures? Or in the case of smell, where the atmosphere is driven by the feeling one gets from the smell of home cooking, or of freshly cut flowers, how are we to understand it? Is it coziness or social warmth?
Words are undoubtably important for thinking about atmospheres. But there is a catch. The inference to emotion or mood, if based on how a subject consciously describes their feelings or attitudes, ties the identity of their emotional state to explicit cognition. When I ask you to describe the feeling of a space, I am asking you to spend a moment and think about the space. Yet, it is known that explicit responses are often misleading and that human experience, especially emotion, is substantially affected by implicit states and processes not accessible to linguistic introspection. Words are a good start in approaching the phenomena of atmosphere, but they hardly provide a foundation for scientific study. We need non-linguistic measures.
2.2.2. Non-linguistic measures for affect
There are four implicit measures based on observation and monitoring of the body and behavior that provide evidence for mood and emotion. Here, they are in brief.
2.2.2.1. Affect from facial expression
Facial expression analysis uses machine learning algorithms to analyze the movements and shapes of a person's face to infer emotional state (Li and Deng, 2020).
In a feature-based approach, specific facial landmarks, such as the position of the eyes, mouth, and eyebrows, and the distance and angle between them determine a probability distribution of different emotional states (Wang et al., 2006).
In a holistic approach, features of the entire face, rather than individual facial landmarks, are identified by using convolutional neural networks (CNNs) to extract whole face features and those are used to classify faces into different emotional states (Lopes et al., 2017).
In action unit recognition, different facial muscle movements are associated with different emotions by deep learning models trained on analyses of the specific action units activated when a certain emotion is perceived. (Tarnowski et al., 2017; Ko, 2018). The pioneer of this approach is Paul Ekman who developed the Facial Action Coding System (FACS) (Ekman and Rosenberg, 1999) based on his theory of six basic patterns of expression (happiness, anger, disgust, fear, sadness, and surprise) (Ekman et al., 1982).
2.2.2.2. Affect from speech prosody
Vocal emotion recognition works by analyzing a person's pitch, speaking rate, volume, and prosody (the rhythm, stress structure, and intonation of speech) to compute a measure of emotional state. For instance, spectral analysis can analyze the frequency and amplitude of words spontaneously spoken in a space to identify emotions such as happiness, sadness, anger, and fear (Prasomphan, 2015). Prosodic analysis focuses on rhythm and intonation to identify emotions such as excitement, surprise, or sarcasm. No one is asked to answer questions. But if they were asked, they would have no idea that the data being studied is not the words they use but rather the way they say them.
2.2.2.3. Affect from body and movement
Body language analysis is based on the idea that certain body movements and positions, especially in hands, arms, and head are associated with specific emotions. Again, there is no emphasis on the words people say as much as any movements they make saying them. Equally, linguistic behavior may be completely absent and how people move in a space is the variable studied. Three forms of movement analysis are:
• Gesture analysis focuses on a person's hand and arm movements to infer their emotional state. Empirically, certain hand and arm movements are associated with specific emotions. A person who is gesturing wildly with their arms may be perceived as excited or angry, while a person who is crossing their arms may be perceived as defensive or closed off.
• Posture analysis infers emotional state from a person's overall body position and alignment, as well as the position of specific body parts such as the head, shoulders, and feet. Standing tall with shoulders back weighs an inference of confidence while slouching weighs an inference of feeling defeated or sad.
• Movement analysis involves observing a person's overall movement patterns, such as how they walk, move, or shift their weight, to infer their emotional state. For example, a person who is moving quickly and energetically may be perceived as excited, while a person who is moving slowly and hesitantly may be perceived as depressed.
2.2.2.4. Affect from physiological measures
This is the neuroscientist's favorite. Since William James' initial work on emotion, affect has always been assumed to have an important physiological component. In James' theory, when humans enter a physiological state related to attraction or aversion, they are en route to an emotion, but their physiological state must be interpreted if they are to experience an actual emotion. Emotion, therefore, has both a physiological and cognitive component; emotion is a state of a cognitive-affective system rather than a state of a physiological affective system simpliciter.
Common physiological measures of emotional state available through inexpensive sensors (Mauss and Robinson, 2009) include heart rate variability (HRV) (Kim et al., 2018), galvanic skin response (GSR) or electrodermal activity (EDA) (Zangróniz et al., 2017), facial electromyography (EMG) (Van Boxtel, 2010) to study facial expressions, skin temperature (Merla and Romani, 2007), and blood pressure (Dzedzickis et al., 2020). EEG is a far more complex measure of emotional state based on neural activity.
It is beyond our scope to discuss the reliability and precision of inferences based on each of these different measures. Virtually, all studies involve plotting affect on a map with at least two dimensions: valence (like-dislike or pleasant-unpleasant) and intensity (high-low or active-subdued). More sophisticated analyses of emotion add more dimensions. In Figure 8A, the affect associated with cozy environments is plotted on a 2D scale. In Figure 8B, a plot of 183 subjects' emotional reaction to the Grand Canyon is shown. Note the wide diversity of emotional reactions in both dimensions: excitedness and pleasantness. As more subjects are tested, a natural grouping into three or four general reactions might emerge.
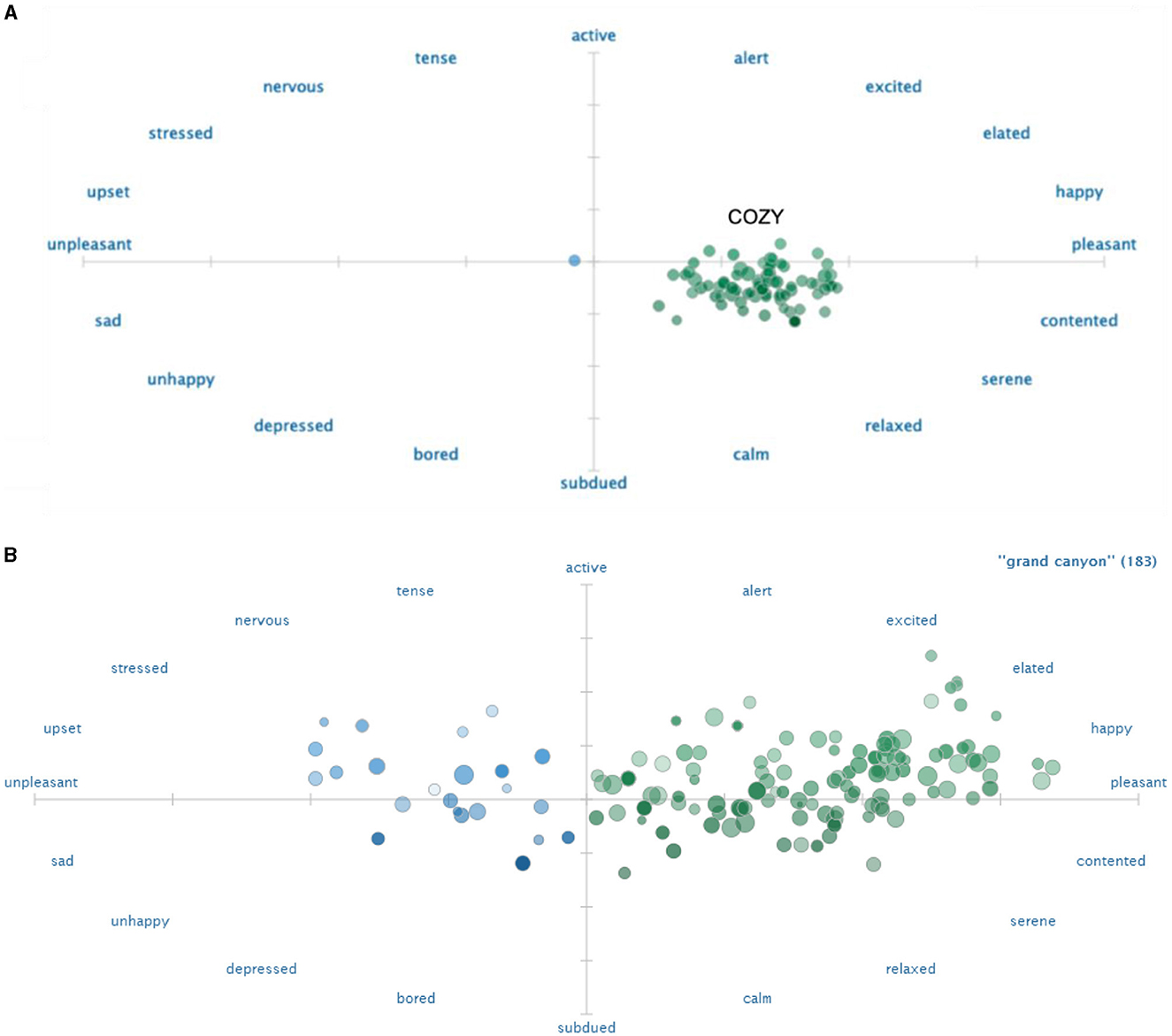
Figure 8. In (A) the graph illustrates emotions that might be identified in individuals when they are tested in environments semantically associated with a “cozy” atmosphere. In (B), an actual plot of emotional values is given for subjects' reactions to the grand canyon.
2.3. Correlating affect state with feature clouds of atmospheres
The final step in an externalist approach is to connect the affect cloud that subjects feel when in a space with the feature cloud for those same spaces. The atmosphere is usually connected more to mood than transitory emotions. Assuming multiple measures of affect are taken, an affect cloud over time provides a measure of emotional range. This can potentially constrain or expand the feature space of a labeled atmosphere. The idea is to move beyond single-word descriptions of feature clouds and regroup the clouds more precisely by using measures of affect, and ideally mood as the mean affect over time. The two—word label and mean affect—are not the same.
For instance, a space labeled calm by our semantic analysis might be measured as eliciting a feeling of being calm, relaxed, or serene. These last are the emotions or affective states. They map quite well to labels, but others, such as anger or surprise, work less well as labels for an atmosphere. What is a surprising or angry atmosphere?
Correlating mean affect with feature clouds is obviously an area requiring major research. We can expect to learn much by trying to understand specific cases. For example, it is possible, and even quite likely, that outliers in a feature space specification of an atmosphere, such as cozy, might be central cases of cozy in affect space. Although a space might appear quite different than paradigmatic cozy spaces, there is something about that space that causes inhabitants to feel it is cozy. The reverse is also possible. A central case of a cozy environment according to its feature space classification might be an outlier in cozy affect space. This might happen when two environments are close in appearance but differ in one or two respects that make all the difference to how people feel; such as having a foul odor or smelling like baked bread (see Figure 9).
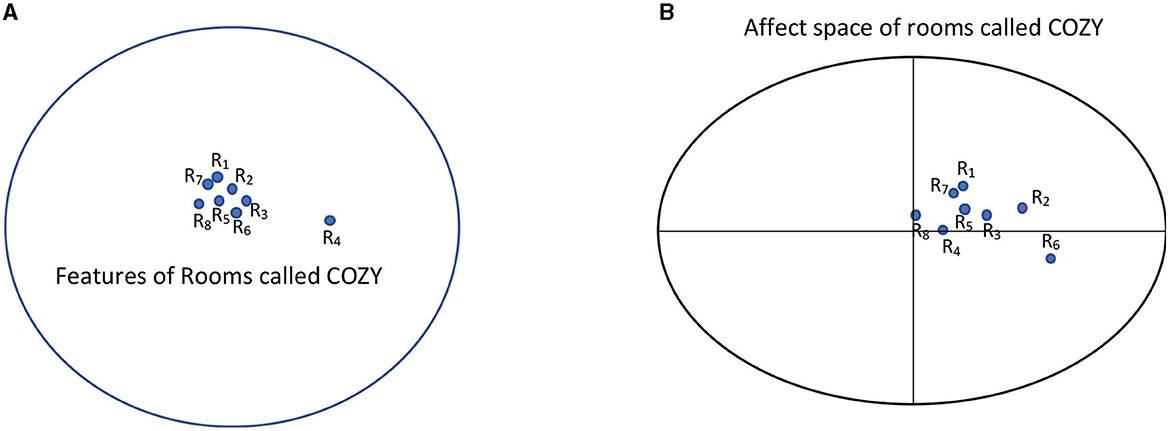
Figure 9. In (A) the features of rooms R1 to R8 are shown plotted in feature space. In (B) their affect value as determined from all affect measures is shown. R4 is an outlier in feature space but central in the affect space of cozy. Because R4 is called cozy and has an affect value near the heart of cozy spaces, we should treat the outlier as part of the feature space of cozy. R6, by contrast, is an outlier in affect space. Should we reject R6 as a member of the cozy feature set? The actual process of balancing affect similarity and feature similarity is bound to be dynamic and involve tradeoffs not shown here.
This ends our account of an externalist theory of atmosphere. There is just one point to reiterate. This procedure will deliver feature clouds for atmospheres that we intuitively understand only if there is broad consistency in the words subjects use to describe the atmospheres of rooms. If people differ significantly, and we have no explanation for why they do, such as that they entered angry, they are busy talking with a friend, etc., the concept of the atmosphere will be too noisy for correlational approaches to be useful. It will cast substantial doubt on the viability of an externalist account.
We now turn to a more subjectivist or internalist approach that challenges the externalist view on first principles.
3. A subjective or internalist account
The opposite of an externalist view of atmosphere is to identify atmosphere with a subject's state of mind and give up the goal of ever finding external features that might reliably serve as the cause of someone judging a space to have this or that atmosphere.
An analogy: beauty is entirely in the mind of the beholder. On this subjectivist view, there is no objective fact about whether something is beautiful because people vary so much in what they judge as beautiful or ugly that the real causes—the things that such judgments correlate with—are far more about their culture, the context of viewing, their age and upbringing, past associations, what they are doing at the time of judgment, or certain internal states, such as dopamine surges, that can be triggered in almost any situation, including ones where the subject is not attending to anything external. Beauty is not definable as a state of the world because it is not reliably intersubjective.
Returning to architecture: let us define an atmosphere as an internal, subjective experience if it is more tied to the unique characteristics of the person experiencing it than to any specific architectural attributes. An atmosphere, on this account, is more of an individualistic or subjective phenomenon requiring an idiographic or idiothetic perspective that focuses on the specifics of individuals and their unique traits and history (Cone, 1986; Millon and Roger, 1995). What any individual experiences is acutely sensitive to their past associations, individual preferences, personal semiotics, professional training, cultural background, current mental state, biological factors like body size, visual acuity, psychological processes such as priming and habituation, and physiological effects such as sensory adaptation that depend on where they have recently been. On a subjectivist account, these, and other contextual factors, such as tasks and interests, social interaction, and incoming mood are causally more influential in how a person registers an atmosphere than external features. If subjectivists are right, a general theory based on external features is impossible because too often it will be swamped by these individualistic factors.
To be more systematic, the deepest obstacles to identifying the atmosphere with features of a space are related to the factors influencing how a person understands and reacts to a situation. These include their:
• Incoming mood
• Cultural predispositions
• Personal semiotics, prior associations, and personality
• Social context and social interaction
• Tasks, activities, and interests
Let us briefly consider each (see Figure 10).
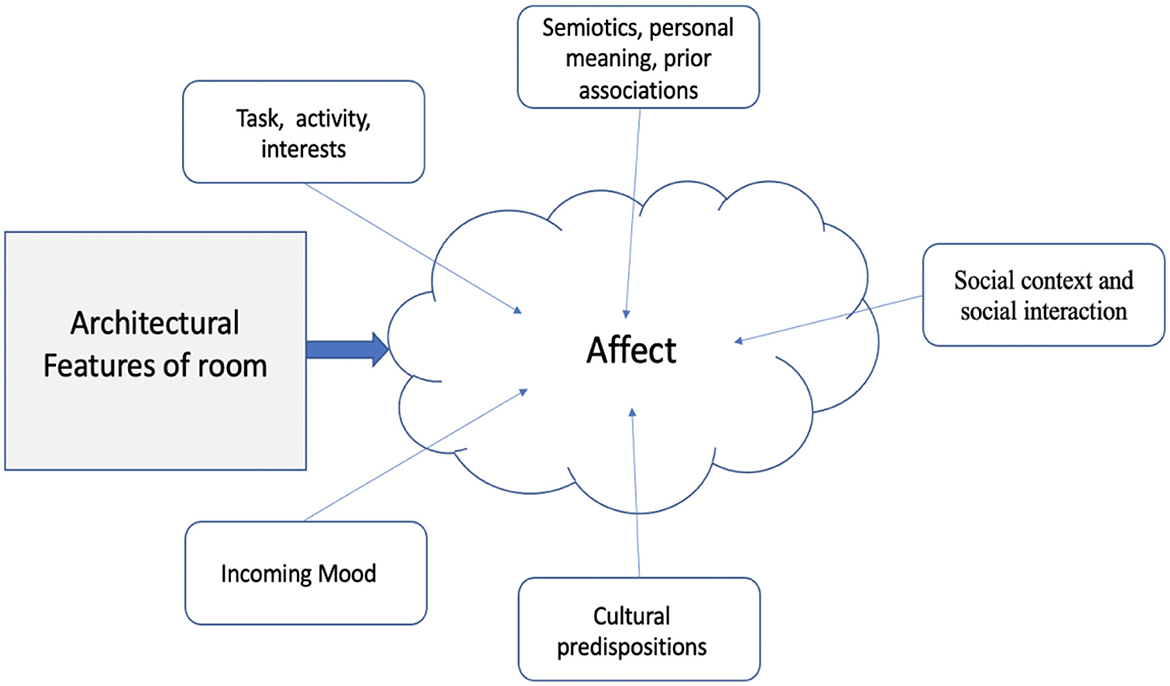
Figure 10. Factors related to the history, predispositions, and culture of a subject strongly affect how she responds to the features of a space, so do contextual conditions such as social context, task and activities, and incoming mood.
3.1. Incoming mood
What are you feeling or thinking when you enter a space? Emotion and mood color the way people experience the present, remember the past, and forecast the future (Forgas and Eich, 2013; Lee and Kim, 2015). People who arrive at a room in a happy state are more likely to feel relaxed, welcomed, and comfortable there than those who arrive in a negative mood (Niedenthal and Setterlund, 1994).
The term “emotional congruence” refers to situations where a person's mood matches the purported atmosphere of their environment. Zadra and Clore (2011) found that stimulus events in the visual field that match an individual's emotional state are perceived more efficiently than other stimuli. This implies that the incoming state affects what a person will look at, how they look at it, and how they then change their mood in response to that biased input. As Simon long ago pointed out (Simon, 1967)13, mood also affects their action and processing priorities. Imagine an architectural critic entering a space after just describing what she likes about the architect, or entering after a disturbing conversation. Incoming mood, of course, is not a dimension of feature space. It is an internal state that colors one's subsequent perceptions, one's subsequent feelings, and even performance.
3.2. Cultural predisposition and personal semiotics
The meaning a person assigns to an architectural space regularly varies with culture and lifelong associations. These are some of the many cognitive accompaniments that figure in shaping affect (Ulrich, 1983). Many emotions are colored by such appraisals (Ulrich, 1983; Keltner and Haidt, 2003).
See Figures 11A, B for two architectural spaces where pride, patriotism, or a sense of belonging to a group are likely to influence how occupants perceive the space. Some people identify with it; perhaps attributing to themselves things they judge the space as embodying or representing, such as beauty, power, and devotion. Each space has a personal meaning to those who work or are likely to visit, and this meaning encourages them to see symbols and assign a semiotic value to what they perceive. Their reaction is quite different than those who recognize none of the semiotics or who feel unconnected.
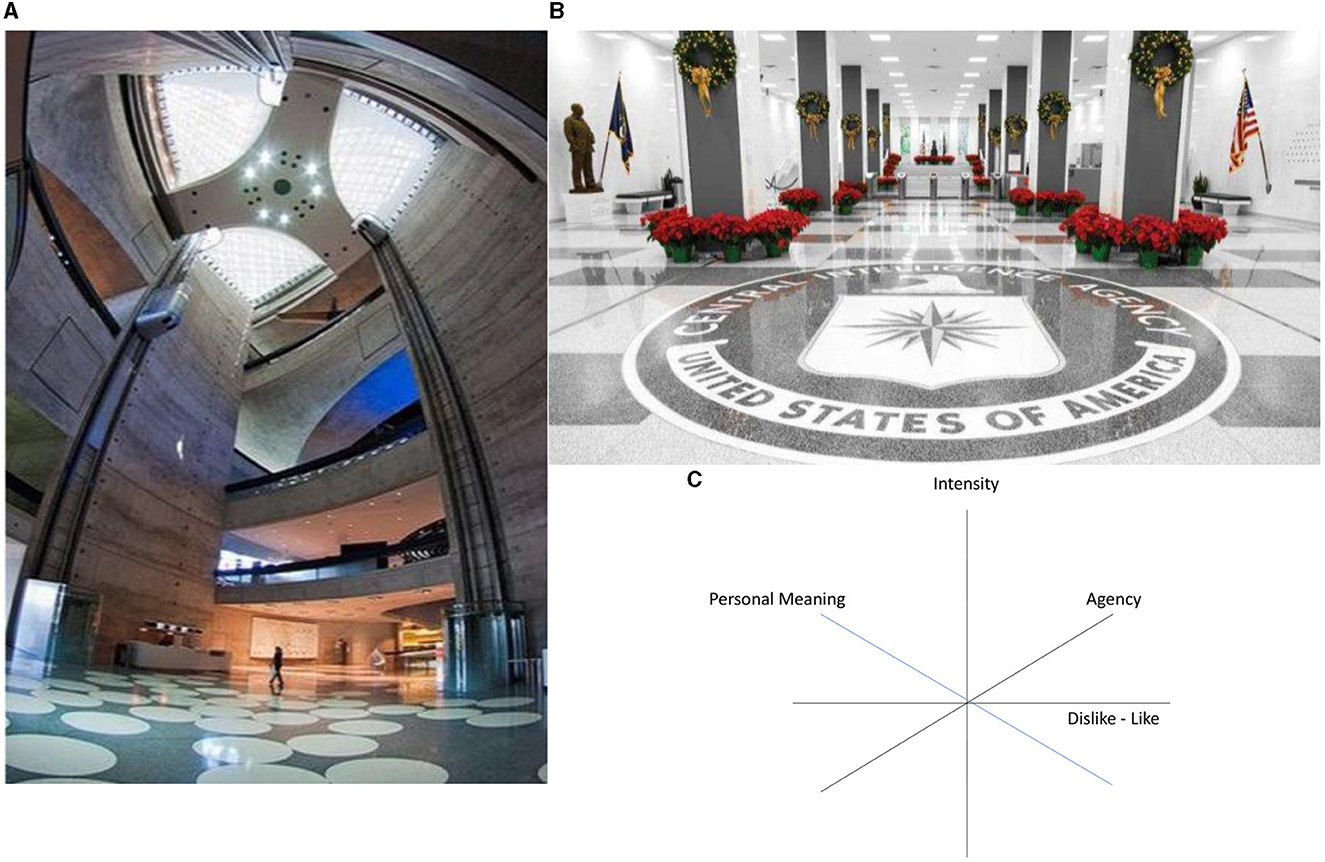
Figure 11. (A) an employee of Mercedes Benz, or even a Mercedes Benz car owner, may be expected to feel this space has more atmosphere or to feel it to be more special because of the semiotic elements of the Mercedes Benz brand that are visible in the space. (B) an employee of the CIA may feel a sense of pride or patriotic pleasure each time s/he enters this otherwise unattractive atrium owing to the personal meaning it has for them. (C) To accommodate the importance of semiotics and personal agency or dominance in a theory of emotions, it is helpful to treat emotion as a cognitive-affective system that has four dimensions: intensity and like/dislike, one's feeling of agency or control over outcomes, and the personal meaning one attributes to an event, process, or structure. Adding a semiotic dimension to affect increases the complexity of the construct.
Factors such as age, experience, and culture, all figure in the quality and complexity of conscious experience of emotion, and they change throughout an individual's life as affective-cognitive structures are formed (Izard and Buechler, 1980). A natural scene that elicits pleasantness in two observers, one an adult and the other a child, may nonetheless produce different emotions despite both adult and child encoding the scene with the same valence and intensity because adults may (i) have antecedent appraisals (Smith and Ellsworth, 1985)—i.e., prior experience with the scene in a manner that carries personal meaning, (ii) the adult may process the scene more deeply, seeing and understanding the scene in ways that are quite remote from how things look superficially (Bodenhausen et al., 1994), and (iii) the effect a scene has on activation in different brain hemispheres changes with neurodevelopment. Hence, child and adult can be expected to respond differently to similar stimuli (Harmon-Jones and Sigelman, 2001).
Feelings driven by “place” is a further example of how culture and semiotics affect emotion and mood. Without addressing what is meant by place in architecture, it is worth emphasizing that people can develop an attachment to places and that being from or growing up in a certain place can figure in their idea of who they are—their identity. The implication is that, at times, a person's historical or culturally engendered feelings about a place can be the dominant cause of emotions or mood, completely swamping the effect of local attributes. Consider the Vatican to a Catholic, or parts of Mecca to a Muslim.
One consequence of emphasizing the importance of culture and semiotics to the interpretation of feeling is that a theory based on arousal and valence looks increasingly inadequate. Other dimensions frequently discussed include a sense of agency or dominance—the ability to change or act in the situation, which clearly may be different for children and adults. This is a move in the right direction. However, it leaves unaddressed how deeply our sense of meaning in a place affects our feelings—a dimension, so far, that resists easy placement on a scale (see Figure 11).
3.3. Associations, personality, and other priors
Beyond semiotics, personality and longer-term personal predispositions regularly bias how a room affects one. A person with a negative or pessimistic view of the world, for instance, is more likely to interpret events in a negative light, leading to an experience of negative emotions such as sadness, anger, or fear, all of which contribute to a non-positive mood. A person with a positive or optimistic view of the world may be more likely to interpret events in a positive light and to experience positive emotions such as happiness, excitement, or contentment. Predispositions matter.
Retrospective views also affect evaluation. “Moments rich with self-relevant information will dominate people's global evaluations of past affective episodes” (Barbara, 2000). Someone who later learned things of real value from an experience may reflectively reevaluate what that episode means. One's first impression of a classroom (or teacher) before any actual teaching has begun may be transformed significantly by what one learned there. The emotions felt when returning to that classroom next year would be colored by one's association: enhanced in value if the associations were good, diminished in value if bad.
3.4. Social context and social interaction
From common sense alone, we know that how people walk through a space, what they do in a space, and how they evaluate a space, whether positively or negatively, depends in part on what they are doing, who they are doing it with, and whether they like or dislike the people they are with. The neural state of a person entering a space differs depending on whether they enter alone or with another. Just the presence of another conspecific affects the brain state (Gallese and Goldman, 1998).
Social context also affects evaluation when two people talk about a space. They may collectively decide what they think and feel, or consider the reaction of a student who is being told by their tutor how to look at the space. Their own interest-driven encounter is biased by the other.
It is uncontentious that the state of a person just before, during, and immediately after being in an architectural space has a significant impact on the affect which that space engenders. The question to ask is: how important are personal state factors relative to external features in determining emotional response? If internal factors end up being more predictive of affect than architectural features, it will be hard to justify the choice of design features as the immediate driver of emotional response.
I now consider the importance of tasks, activities, and interests in shaping one's encounter and experience of architectural spaces.
4. The impact of task, activity, and interests on the environment
Both externalist and internalist accounts uncritically assume that if two people are exposed to the same feature space—the same room objectively construed—then they are in the same environment regardless of what they are doing. This assumption can be challenged. Do shop owners and customers really act in the same environment? Several studies found that shop owners and shoppers differ in how they engage the same retail space, owing to different goals, tasks, and behaviors that shape their perceptions and interactions in the setting (Bäckström and Johansson, 2006). Tasks and objectives frame what is important and attended to. Customers, it was found, focus more on making systematic evaluations of products for purchase while salespeople focus more on the customer and persuading them (Belk, 1988). Their focus of attention, the objects, and the nearby properties they are concerned with are quite different. Tasks, interests, and activities shape our enactive encounters with our environments.
Compare the experience of movie custodians and moviegoers. Due to their extended tenure at the theater and the requirements of their job, custodians see things moviegoers do not. They see garbage under seats; they see which ceiling lights are unusually dim, or that a small tear in the screen has expanded. Moviegoers, meanwhile, may notice the beauty of the ceiling's molding, or that their seat reclines uncommonly low or smoothly. They may be struck by the lovely hue of the red lights against the carpet. First-time attendees may judge the space to be novel, interesting, and innovative. Not so for veterans.
Enactive cognition offers a model of how to think about the contribution that individuals make to their own experience of an atmosphere. On the enactive view of perception (Paolo and Thompson, 2014), we do not experience the world all at once, as if it is an objective form that stamps itself on us the way light stamps itself on a camera sensor. Perception is active, sometimes interactive. We move our eyes, and we attend to this or that, focusing more narrowly on certain attributes, oblivious to others. We are rarely stationary. We walk, touch, and approach; and these interactions affect the sequence of what we experience and how. Two people rarely encounter a room the same way. Attention, action, and interests matter and differentiate us.
Two technical terms, “task environment” and “activity space” can be invoked to explain why it is not always best to treat the environment a subject is in, to be the geometric or physical space surrounding them. The pivotal notion is mental focus. When someone is solving a problem—say playing chess—what matters is the location of pieces in chess space. Their physical room does not matter, the physical position of their opponent does not matter, and the location of the chess board does not matter. All that matters is their position in the chess space. Were there a physical chess board present, the way they project meaning onto the pieces and squares on the board will lead them to internally represent the board not so much in geometrical space as in an abstract graph structure of states and transitions—nodes and links—that specifies locations in the state space of chess (Gobet et al., 2004). The players could continue their game on computers miles apart, and still recreate their exact locations in chess space. Obviously, chess space and physical space are not the same. The question is: what environment are they operating in? The physical one which they must understand to physically move pieces, or the keyboard monitor environment they use to play online, or is it the chess environment—chess state space—which is identical across different physical implementations?
It may seem that a task environment is a cognitive concept and not a configuration in external space. That is not how Newell and Simon (1972) defined it. For them, the task environment is the external context, while the problem space is the internal representation of that context. Different players may represent the problem space of a task differently, and because of their different representations, the problem may be easier or harder to solve. However, the task environment of the two—the legal states given the constraints and terms of the problem, and the actions that are permitted as moves in the task space—remain the same for both players. So, on this account, the environment that a person is in, during the period when they are actively thinking and solving a problem, has an abstract description that maps onto physical things the way the meaning of words maps onto the type face of text. It means that we can inhabit many environments in the same physical space, each one depending on our task at the time.
I think that everyone knows this already. Squash and racquetball in the UK are played on the same court with slightly different rules. The games are distinct. In a very real sense, the environment faced by squash players is different than that faced by racquetball players despite their material equivalence. Checkers and chess players operate in different environments despite sharing the same black-and-white checkered board. And in the restaurant world, servers and bussers working the same tables operate in different task environments because their roles differ.
The implication for atmosphere cognition is that two people, even those similar in culture, age, and personal history, might evaluate the atmosphere of the same architectural space differently, owing entirely to the different tasks or activities they are engaged in. Whatever feelings a material space might confer on an idle visitor can easily be overridden as soon as that visitor becomes active in a task. What then is the real atmosphere of a space? Tasks often generate their own feelings (e.g., losing or running slow). This question becomes especially problematic if it is accepted that moods are treated as a running average of emotional affect values. We cannot simplistically identify an atmosphere as what a space feels like when we are idle, or when we first enter, given that as soon as we have an activity our feelings change.
This concludes our discussion of whether an atmosphere should be deemed a construct that is ultimately definable over material properties of the environment or whether it is so dependent on momentary details of inhabitants that there is nothing bounded that can be justifiably called the external cause of feelings, emotions, or moods.
5. Emotion as foreground, mood as background
Those of us who find it hard to resist the realist's urge to find something material as the cause of our emotional reactions to architecture may hope that the distinction between an emotion and a mood may give us what we want. Mood and emotion are related but distinct concepts. So far we have been looking at measures of emotional feelings as they arise moment by moment in specific contexts. This is a foreground notion. Maybe the atmosphere is better seen as a subtle backdrop, akin to the bass beat in music or a recurring theme that does not dictate the melody (or our emotions), yet still exerts its influence and shows up in long-term analysis.
In this brief section, I enumerate a few well-known differences between mood and emotion and then consider possible analogies for how an atmosphere, as an external reality, might affect our mood.
5.1. Differences among duration, cause, and intensity
In psychological literature, emotions are short-lived feelings (Ekman, 1992) that are typically triggered by a specific event or situation (Mulligan and Scherer, 2012). They are intense, usually in response to a clear cause that elicits a rapid, automatic, and unconscious activation of specific areas of the brain, such as the amygdala (LeDoux, 2012) and the prefrontal cortex (Etkin et al., 2011; Dixon et al., 2017). They are associated with changes in neurotransmitters and hormones, such as adrenaline and cortisol.
Moods, by contrast, are more general and long-lasting feelings. They are less intense than emotions and typically lack a clear cause-and-effect relationship (Stephan, 2017). They can be positive, such as feeling content or satisfied, or negative, such as feeling down or gloomy. They are often described as a background feeling that is present for a longer period, and they can be influenced by a variety of factors such as life events, physical health, and personality. This is reflected at the neurophysiological level with more gradual and sustained changes in neural activity in the activity of neurotransmitters and hormones, such as serotonin, dopamine, and endorphins, and the activity of brain regions involved in reward and motivation, such as the amygdala and ventral striatum (Helion et al., 2019). It is sometimes said that mood reflects a running average of sequential emotional events together with an underlying internal affective state (Eldar et al., 2016).
Examples of everyday mood states that are not indicative of a mental disorder include happiness, contentment, excitement, calmness, nostalgia, hope, boredom, enthusiasm, satisfaction, and melancholia. But the exact choice of terms and distinctions that theorists use seems highly individual. Moods, like emotions, are often initially measured using self-report measures, such as rating scales or questionnaires that ask people to rate their current mood. However, unlike emotions, questions about mood also ask subjects how they have been feeling over a certain period of time, and about overall feelings, such as happiness, sadness, or anxiety An example of self-report measures of mood is the Profile of Mood States (POMS) (McNair et al., 1992). It assesses six dimensions of mood: tension-anxiety, depression-dejection, anger-hostility, vigor-activity, fatigue-inertia, and confusion-bewilderment.
It is unclear how the mood associated with an atmosphere is to be identified given the large difference in time between emotional response and the gradual formation of a mood. One problem is that architects and commentators do not agree on how fast subjects register an atmosphere. Zumthor says “We perceive atmosphere through our emotional sensibility, a form of perception that works incredibly quickly.” He holds this view despite often speaking of atmosphere as something not directly perceivable, and despite recognizing that we interact with a space, it can take time to pick up the mood of a place. This last point echoes the idea in many theoretical accounts (Eldar et al., 2016) that we integrate over the history of our experiences when choosing how to describe our mood.
Given the complexity of the emotion–mood relationship, there is no way to simply extend our analysis of the emotional state to the mood state. There is a chance that when a subject is alone in a space, the notion of momentum might be made operational. During those moments, when there are no social relations to muddy the attribution of emotion, the key factors that drive experience are the subject's personal biases, their activity, and the environment. The environment is the external factor, their activity is an interactive factor, and their personal biases are subject-dependent, though not necessarily subjective.
Much of this study has been about the challenge these non-external confounds present. Even objective factors such as where a person is in a space, how long they are in it, and what they are doing change the mental and physical state of a person, and that is before we consider the personal meaning a space has for them, their mood immediately before entering a space and the nature of their social relation to others close by. Perhaps it will be possible to reduce some action-related confounds by distinguishing emotions by their intentionality—an attitude to a chess move is not an attitude directed at the physical atmosphere. Or perhaps with careful experimentation, we can probe a person's emotional state as we manipulate factor after factor. We might then be able to develop a theory about the complex interaction of all these factors. It will not be easy.
5.2. A visual analogy of atmosphere as background value
In thinking about analogies, I find myself repeatedly drawn to Fourier's analysis of series. The power of this analysis is that it breaks any digital signal into a sum of simple sine waves with offsets and amplitudes. This would simplify our idea of what an atmosphere is: it would be analogous to a single or small complex of “waves” that operate in the background. Other things, such as social interaction, task activity, incoming mood, and the rest, would be additional factors, again, all analogous to waves, or compound waves that add to or subtract from the emotional impact of the underlying background that is being called the atmosphere. The overall feeling we have in a space would be analogous to a complex sound that is the complete result of wave summation (see Figure 12).
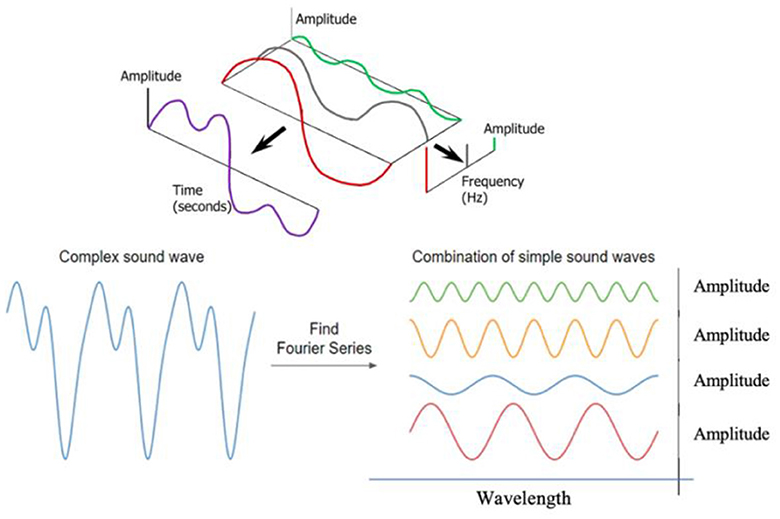
Figure 12. The feeling we experience in a space may be compared to a complex sound wave. Shown here are two different ways of looking at a Fourier analysis of complex sound waves. In the top image, the complex wave is shown on the left as a linear result of combining different wave frequencies each with a different amplitude. None are offset—i.e., out of phase—but the formalism allows for phase modulation. In the lower image, the complex wave is again decomposed into simple waves with varying frequency and amplitude. They are all in phase. For realism, we should assume that each wave that is not an atmosphere can vary in intensity (amplitude) and there can be new waves introduced as things happen, such as starting a new task or meeting a person. The result is a potentially non-linear change in the sequence of compound waves that might reflect the changing emotions a person feels in a space.
Pursuing this idea, to explain why we are not always aware of the atmosphere of a place, or why we fail to register it in an emotional way, we can invoke the process of attention. Attention either amps up or tamps down the “wave” we associate with the background atmosphere. Or it might leave the background wave unaltered but boosts the intensity of other factors. When engaged in a task, for instance, we may barely notice the background atmosphere because we are focusing so strongly on other features of our activity space. Incoming mood or semiotics too can be accommodated by treating them as a variety of waves that can diminish, enhance, or completely alter the emotion that would be attached to the background atmosphere on its own.
The one serious downside of this simple analogy is that all the waves in the Fourier transform are additive. They are linearly superposed. Hence, they can not combine or interact in a non-linear manner, a feature very much typical of our experience of atmospheres.
This need not derail the analogy. Fourier analysis explains the physical compositionality of sound; it does not explain the experiential non-linearity of sound perception. The experience of a chord is quite different than the experience of each note. Consider consonant and dissonant chords. A single note on its own, and not coming after other notes, is neither consonant or dissonant nor harmonious, sad, or happy. Add a second note simultaneously and suddenly our feeling may change. Chords have feelings, single notes do not. All this subjective variation is completely outside the physical theory of harmonic compositionality. Yet, it still tells us something deep about sound. The factors influencing how a person registers an atmosphere are surely as complex as the ways waves interact, and further, as complex as musical feeling.
Here is another similarity. Fourier analysis only explains the simultaneous composition of simple frequencies. Music is about change in sound. Nothing about Fourier analysis constrains the way sound can change. Chord changes can be arbitrarily diverse and non-linear. Accordingly, a major aspect of the non-linearity that people experience in music is caused by changes in input. Similarly, the non-linearity of feelings that people experience in buildings may be caused by changes in any of the factors that influence feeling, including attention. We know that these factors constantly change as people move, encounter others, interact with objects, and engage in tasks.
Thus, even if a background atmosphere remains constant—the way a bass beat might endure across chord changes—the other sounds it combines with, the chords or melodies running over it, all change. Hence, overall experience changes. Arbitrary non-linearity of how we register the compositionality of the parts of an atmosphere and our overall experience of a building can be explained on analogy with changing wave patterns over time.14
6. Design implications
Understanding the connection between a building's atmosphere and the emotional responses of its occupants is a complex task. This might lead some to question how architects might benefit from empirical studies of the atmosphere.
My viewpoint is that atmosphere assessment will evolve into an empirically based tool—a plugin—with customizable settings that architects can use to gauge the emotional resonance of their designs. Given a 3D model of a space, complete with defined lighting, textures, and materials, an atmosphere tool will calculate the light and sound experienced by selected subject types as they move around the space. Having a good classification of subject types will be important. These calculations will then be translated into emotional impact values. Designers will consider these values alongside other factors when deciding between potential design concepts. This same tool could also be integrated into generative design systems, allowing designers to set desired emotional responses and moods, and then assess potential designs accordingly.
There's a catch, though. The predictions about how people feel as they move along various paths will be presented as distributions. People naturally have different emotional responses to a space. It could feel cozy, intimate, joyful, or even stifling, depending on the person, time of day, how they feel, occasion, weather, their age, and how they move. These other factors, treated earlier as confounds, add a layer of complexity that forces our tool to generate a distribution of emotions. Distributions are notoriously hard to draw morals from unless they are collapsed to mean or median values.
Herein lies the challenge. While designers can easily comprehend averages, relying on them can be misleading. Consider this: how would an 'average' person, with 'average' characteristics and companions, feel as they navigate a specific path? Even if multiple average people—'personas'—are used instead of a single prototypical person or user model, this is still problematic.
Research in recommendation systems, such as Spotify, has found that relying on a single average or single median for music recommendations leads to unsatisfactory results (Elliott, 2020). Consider a gym setting. The music tastes among gym-goers are diverse. When the most popular or average music is broadcast, individuals usually choose their own headphones over listening to the venue's choice. This demonstrates a clear preference for personalized experiences. Increasing the number of personas and delivering music based on each individual's closest persona, should yield more favorable ratings. But how many of these personas are needed? Spotify uses thousands (Charlotte, 2021).
Like gym music, architecture broadcasts to everyone. The nature of agent-building interaction changes when occupants wear earphones, and it will change even more when buildings themselves are adaptive. If we can adjust buildings dynamically to cater to identified personas, atmosphere plugins during the design process are likely to become particularly valuable.
Even before the era of adaptive buildings, however, an atmosphere plugin could prove useful if we moderate our goals and concentrate on individual aspects—such as light, acoustics, noise levels, and textures—or selected feature clusters. Following this line of reasoning, the significance of a tool becomes more evident. Designers are typically required to provide reasons for their decisions. When choosing a 3D lighting setup, they are usually trying to solve specific problems, such as creating a serene or peaceful atmosphere. Some choices are better than others. How much can we trust a designer's personal convictions about the emotional effects of various lighting types? Would not data-driven recommendations be beneficial? What about the impact on conversations involving two, three, or more people? An algorithm that classifies conversation length, liveliness, and other factors in relation to lighting could provide insightful information.
Imagine a different scenario where the lighting in question is designed to suit specific tasks. A well-designed plugin should include both task and persona as parameters. No one expects this plugin anytime soon because there are endless such queries. How will people respond to specific types of reflective noise, acoustics, or rooms with features such as teak walls, carpeted floors, or large spaces while responding to emails or solving an Excel problem? This is why I believe our best chance for modality analysis, and eventually atmosphere analysis, lies in quantifying buildings—equipping them with hundreds or thousands of sensors and monitoring people's movements within these spaces. We need to leverage the potential of big data to tackle these multi-dimensional challenges before we can design suitable experiments to uncover the fundamental principles that dictate the atmospheric rhythm of a space.
And yet even a big-data approach is problematic. How do we know we are capturing the right data—the right features, user attributes, and behavior?
Picture yourself entering an art gallery bustling with hundreds of visitors. Do you sense the atmosphere of the building, or do you sense the collective impact of the people and the structure? The crowd exudes emotions such as excitement, reverence, wonder, and joy. Is not that a part of the atmosphere?15 Now, consider stepping into a museum—a vast warehouse—where you are greeted by a gigantic blue whale hanging from the ceiling. Its grandeur and awe-inspiring presence are overwhelming. Does not the whale, with its commanding presence, define and shape the true atmosphere of this space?
Visualize a Zen Garden: tranquil, silent, and filled with the scent of pristine nature. Now picture it teeming with dozens of visitors snapping photos and conversing. The atmosphere changes completely. Should we not consider these situational elements when discussing atmosphere? People's feelings or perceptions of their surroundings depend on more than just the fundamental architecture.
Large cathedrals are another example: is their atmosphere defined by the solitary monk at Compline, or is it more communal, influenced by the collective experience of hundreds of parishioners during a Sunday mass? And what about football stadiums? Should we examine them when they are empty or when they are in use?
This challenge highlights what many believe16 is overlooked in architectural atmosphere discussions: How the design of a building shapes social behavior. Just as Le Corbusier wrote “A house is a machine for living in” (Corbusier, 1986), we might consider that the atmosphere of buildings should be evaluated when they are occupied and in use. This does not derail a big data approach to identifying atmosphere, as data can be used to infer group emotion. However, it complicates the task of identifying the architectural features that elicit those emotions. The system can no longer draw simple correlations between a building's features and the emotions of its occupants because a significant source of emotion may be what the other people are doing. The atmosphere cannot be tightly linked to basic architectural features, as the social activity of people is equally, if not more, important. Which architectural features stimulate such activity?
The same principle applies to furniture, installations, and music as much as to what the people in a space are doing. In discussing atmosphere, I have followed the common practice of considering the causal elements to be features of the core architecture, excluding furniture, plants, other people in the room, or the known function of the room. Yet, we all understand that people's reactions to a space are influenced by all these factors.
Patrik Schumacher has presented a related point (Schumacher, 2016). He argues that one of the primary objectives of architecture is to enable socially coordinated behavior. In his view, atmosphere is not so much about the emotions people experience, but rather the cognitive state that a building prompts. When a building is legible, individuals understand the formality of the space, where they should get together and how, the suitable social distance to maintain, the appropriateness of side conversations, and the level of focus the space demands, much like a library where quiet work is the norm. Schumacher's viewpoint underscores the importance of 'reading the room' with ease, not based on observing the behavior of others—which would equate to reading people—but on the structural and social affordances, as well as the spectrum of constraints and opportunities of the form and surfaces of the space.
The perspectives of Penn and Schumacher make construction of a plugin more difficult because they change the definition of the external environment. Schumacher expands the set of externalist features to include functional and semiotic properties of the building; Penn expands the environment to include the reaction of other people in the space.
7. Conclusion
I have been exploring the methods and challenges involved in adopting a scientific approach to the ill-defined concept of architectural atmosphere. The eventual role of atmosphere in the emerging field of cognitive and neuro science for architecture, in my view, remains uncertain. Even casual polling of subjects in buildings reveals significant variations in linguistic assessments of atmosphere. Some of this variation can be explained and accommodated. Some cannot.
For example, some differences among subjects are due to differences in cultural background, personality, age, and experience. This may be resolved by grouping subjects into types—many subject types! Additional variance in atmospheric pick-up may be reduced by theorizing how tasks and activities influence mood, leading to further qualifications if we understand how tasks affect mood. Another qualification is necessary if we acknowledge—as we must—that how a person moves through a space affects their emotions. To accommodate this factor, we might introduce the concept of sub-spaces within a larger space. This might help because the structure and appearance of space depends on where we are and how we are moving. There are further factors that alter judgment of atmosphere—whether implicit or explicit—such as personal associations or meanings that an individual attaches to a specific location or space. Possibly these can be predicted if we have greater knowledge of subjects.
But some differences in how people register atmosphere may be due to factors we have not considered. For instance, in our main analysis, we took the primary causal elements of a space to be features of its core architecture, excluding furniture, plants, other people in the room, or the known function of the room. This is common practice. Yet, we all know that people's responses to a space depend on all these factors. And Penn and others have emphasized this. Beyond social elements, activities as simple as sitting on furniture may have a major influence on how people register atmosphere. Individuals register space one way when they are sitting in a comfy sofa and feeling tranquil, when standing or moving, they register it quite differently. Posture and energy expenditure matter. Physiological state change often impacts emotional feeling. Furniture leads to physiological change.
Functional features of architecture, such as those that convey social norms or that bias conduct, are another problem. They might eventually be inferred from correlations between features and behavior, but these correlations would be lagged because the effect of a building may stretch out over time. Schumacher's complaint remains a challenge.
Yet, despite the dizzying number of causal parameters that need be considered, I remain optimistic about the potential for a scientific approach to atmosphere. It may not encompass the comprehensive, holistic concept that simultaneously embraces all modalities any time soon. In my opinion, we should concentrate on specific design elements such as lighting design, acoustics, color, and material—all aspects that architects and designers believe have psychological impact. But if data analytics is beginning to measure the effects of music on mood, wellbeing, and health (Matsuo et al., 2019; Dingle et al., 2021); why suppose we can not apply data analytics to specific sensory elements of buildings?
Data availability statement
The original contributions presented in the study are included in the article/supplementary material, further inquiries can be directed to the corresponding author.
Author contributions
The author confirms being the sole contributor of this work and has approved it for publication.
Acknowledgments
I have many people and events to thank. For my interest in architectural atmospheres I thank Moving Boundaries, a science meets architecture two week long workshop where I heard passionate arguments for the importance of atmospheric mood. For critical discussions I thank Alan Penn for his surprising suggestion that emergent social activity of groups is as much the determinant of atmosphere as architectural features of the space; to Patrik Schumacher for his skepticism about the importance of emotion in atmosphere and for sharing his completely different view that atmosphere is really social semiotics; to an anonymous reviewer for insightful comments on the first version of the paper; to Sergei Gepshtein for many long hours of conversation and specifically for his elaboration on the state of the art in facial recognition. I also thank Yota Kyriakopoulou for her help with several figures. Finally, I thank the Academy of Neuroscience for Architecture for their intellectual promotion of these inquiries.
Conflict of interest
The author declares that the research was conducted in the absence of any commercial or financial relationships that could be construed as a potential conflict of interest.
Publisher's note
All claims expressed in this article are solely those of the authors and do not necessarily represent those of their affiliated organizations, or those of the publisher, the editors and the reviewers. Any product that may be evaluated in this article, or claim that may be made by its manufacturer, is not guaranteed or endorsed by the publisher.
Footnotes
1. ^This is the introductory definition offered in Arbib et al. (2023). Systems of Systems: Architectural Atmosphere, Neuromorphic Architecture, and the Well-Being of Humans and Ecospheres. In The Routledge Companion to Ecological Design Thinking (pp. 64-74). Routledge.
2. ^Zumpthor among others does not restrict our emotive-cognitive engagement of a building to its interiors. He also regard how a building relates to its environment, or how “it becomes part of its surroundings” to also constitute an atmosphere we can have a bodily engagement with (Zumthor, 2006).
3. ^The theory that color perception can be influenced by a perceiver's language and culture is often associated with the Sapir–Whorf Hypothesis. This theory suggests that the structure of a language can affect its speakers' cognition or world view, including their perception of color. In “Whorf hypothesis is supported in the right visual field but not the left” by A. L. Gilbert et al. (2006), the authors found that language influences color discrimination strongly in the right visual field but not in the left visual field, suggesting that people view the right half of their visual world through the lens of their native language.
4. ^The atmosphere registered by parents of a bride is another example of subject and activity dependence. For parents who are overjoyed at their daughter's marriage, the hall or ceremony room may fill them with a sense of joyousness. To a parent distraught from concern with upcoming inadequate guest arrangements, the hall may feel oppressive or insufficient. What is a normal subject?
5. ^The default network mode of the brain is a set of brain regions that are active when the brain is at rest and not focused on any particular task. It is associated with daydreaming, self-reflection, and spontaneous thoughts. Characteristics of the default network mode of the brain include increased activity in the medial prefrontal cortex and posterior cingulate cortex; decreased activity in the dorsolateral prefrontal cortex; enhanced connectivity between the default-mode network and other brain regions; increased activity in the hippocampus, associated with memory formation and recollection; increased activation of the amygdala, associated with emotional processing; increased activity in the insula, associated with awareness of the body and its environment; and enhanced activation of the ventromedial prefrontal cortex, associated with decision-making but also with self-reflection. Raichle (2015). The brain's default mode network. Annual review of neuroscience, 38, 433-447.
6. ^“Distributed yet palpable, a quality of environmental immersion that registers in and through sensing bodies whilst also remaining diffuse, in the air, ethereal” (McCormack, 2008: 413).
7. ^Pallasmaa calls this our ‘existential sense'. He uses this term to refer to a more embodied sense of being and acting in a space or surrounds rather than just using all one's senses, as important as that is. See Pallasmaa, Juhani. “Architecture and the Existential Sense.” In Art and the Senses, edited by Francesca Bacci & David Melcher. New York: Oxford University Press, 2011.
8. ^The methodology used to estimate this number has been challenged and largely debunked with new estimates that might return human olfactory discrimation to 5,000 odors as it was assumed before 2014. Richard C Gerkin, Jason B Castro. The number of olfactory stimuli that humans can discriminate is still unknown. eLife, 2015; 4 doi: 10.7554/eLife.08127
9. ^To be fully accurate, it will be necessary to describe environmental properties in terms of views, since a person does not have 360-degree vision, and different things are discernable depending on each subject's trajectory. This drastically increases the dimensionality of the space. Rather than points, a room might better be represented as a set of trajectories through it. In principle, voxel formalism can accommodate this in a straightforward manner, though clustering will be more difficult. It is to be assumed that simplifications and abstractions will have to be introduced.
10. ^The distinction between natural and artificial light might prove to be unnecessary in more technical models since it is likely to be definable in terms of fundamental light dimensions.
11. ^In Mauss and Robinson (2009). Measures of emotion: A review. Cognition and emotion, 23(2), 209-237. The authors discuss the validity and reliability of using verbal response to emotion-evocative stimuli as a measure to assess affective feeling. They found that measures are not reliably pointillistic but rather useful for identifying the dimensions of a feeling. Berthoz and Hill (2005). The validity of using self-reports to assess emotion regulation abilities in adults with autism spectrum disorder. They found that self-reports are generally self-consistent across occasions even among those on the autistic spectrum. At a more operational level for architecture, business consultants use surveys to measure the attitude and behavioral responses of shoppers to different architectural styles. They ask participants to rate different architectural styles on a series of affective and behavioral measures such as willingness to stay, willingness to spend money, interest in learning more about the place, and desire to return.
12. ^More sophisticated methods such as conjoint analysis can be used to have subjects make binary comparisons between different rooms to judge which is more ‘serene', etc. This is a powerful method for assigning a specific value to each feature.
13. ^For instance, a chess player deeply engaged emotionally in the outcome of a game will stop her game if she notices her house is on fire. If you shift your mood, you are likely to shift information acquisition strategies, shift evaluation of utility of environmental states, and accordingly, shift what you do.
14. ^A second way non-linearity can be explained by varying the weighting of emotion over time. When mapping emotion to mood, we accepted that mood might be a simple trend or temporal average of emotion. But there is no reason why this is optimal. If I become extremely angry for a few moments, the impact of that emotion on my mood might extend far longer than a simple moving average would predict. In a non-linear model, we might treat high intensity emotions as far more influential on mood than weak emotions.
15. ^This is a point emphasized by Alan Penn in conversation.
16. ^Alan Penn in conversation first proposed this criticism. Ava Fatah Gen. Schieck independently raised this question.
References
Arbib, M., Banasiak, M., and Villegas-Solís, L. O. (2023). Systems of Systems: Architectural Atmosphere, Neuromorphic Architecture, and the WellBeing of Humans and Ecospheres. In The Routledge Companion to Ecological Design Thinking. (Routledge). (pp. 64-74) doi: 10.4324./9781003183181-7
Bäckström, K., and Johansson, U. (2006). Creating and consuming experiences in retail store environments: comparing retailer and consumer perspectives. J. Retail. Consum. Serv. 13, 417–430. doi: 10.1016/j.jretconser.02005
Barbara, L. F. (2000). Extracting meaning from past affective experiences: the importance of peaks, ends, and specific emotions. Cogn. Emot. 14, 577–606. doi: 10.1080/026999300402808
Belk, R. W. (1988). Possessions and the extended self. J. Consu. Res. 15, 139–168. doi: 10.1086/209154
Berthoz, S., and Hill, E. L. (2005). The validity of using self-reports to assess emotion regulation abilities in adults with autism spectrum disorder. Eur. Psychiatry 20, 291–298. doi: 10.1016/j.eurpsy.06013
Bodenhausen, G. V., Kramer, G. P., and Süsser, K. (1994). Happiness and stereotypic thinking in social judgment. J. Person. Soc. Psychol. 66, 621. doi: 10.1037/0022-3514.66.4.621
Böhme, G. (2005). “Atmosphere as the subject matter of architecture” in Natural Histories. Herzog and de Meuron, Switzerland.
Böhme, G. (2013). The art of the stage set as a paradigm for an aesthetics of atmospheres. Ambian. Environ. Sens. Architect. et espace urbain. 4, 315. doi: 10.4000./ambiances.315
Böhme, G., Elíasson, Ó., Pallasmaa, J., and Borch, C. (2014). Architectural Atmospheres: On the Experience and Politics of Architecture. Basel: Birkhäuser.
Boubekri, M., Cheung, I. N., Reid, K. J., Wang, C. H., and Zee, P. C. (2014). Impact of windows and daylight exposure on overall health and sleep quality of office workers: a case-control pilot study. J. Clin. Sleep Med. 10, 603–611. doi: 10.5664/jcsm.3780
Boyd, R. L., Ashokkumar, A., Seraj, S., and Pennebaker, J. W. (2022). The Development and Psychometric Properties of LIWC-22. Austin, TX: University of Texas at Austin, p. 1-47.
Bushdid, C., Magnasco, M. O., Vosshall, L. B., and Keller, A. (2014). Humans can discriminate more than 1 trillion olfactory stimuli. Science 343, 1370–1372. doi: 10.1126/science.1249168
Castro, J. B., Ramanathan, A., and Chennubhotla, C. S. (2013). Categorical dimensions of human odor descriptor space revealed by non-negative matrix factorization. PLoS ONE 8, e73289. doi: 10.1371/journal.pone.0073289
Charlotte, H. (2021). Why Spotify's music recommendations always seem so spot on. Popular Science. Published. PM EST. Available online at: https://www.popsci.com/technology/spotify-audio-recommendation-research/ (accessed December 2, 2008).
Cone, J. D. (1986). Idiographic, nomothetic, and related perspectives in behavioral assessment. in Conceptual Foundations of Behavioral Assessment eds R. O. Nelson and S. C. Hayes (New York: Guilford), (pp. 111–128).
Dingle, G. A., Sharman, L. S., Bauer, Z., Beckman, E., Broughton, M., Bunzli, E., et al. (2021). How do music activities affect health and wellbeing? A scoping review of studies examining psychosocial mechanisms. Front. Psychol. 12, 713818. doi: 10.3389/fpsyg.2021.713818
Dixon, M. L., Thiruchselvam, R., Todd, R., and Christoff, K. (2017). Emotion and the prefrontal cortex: an integrative review. Psychol. Bullet. 143, 1033. doi: 10.1016/j.tics.11004
Dzedzickis, A., Kaklauskas, A., and Bucinskas, V. (2020). Human emotion recognition: review of sensors and methods. Sensors 20, 592. doi: 10.3390/s20030592
Ekman, P. (1992). An argument for basic emotions. Cogn. Emot. 6, 169–200. doi: 10.1080/02699939208411068
Ekman, P., Friesen, W. V., and Ellsworth, P. (1982). What emotion categories or dimensions can observers judge from facial behavior? in Emotion in the Human Face. 2nd ed, ed Ekman P (Cambridge, UK: Cambridge University Press), p. 1982, 39–55.
Ekman, P., and Rosenberg, E. L. eds. (1999). What the Face Reveals: Basic and Applied Studies of Spontaneous Expression Using the Facial Action Coding System (FACS). New York, NY: Oxford University Press.
Eldar, E., Rutledge, R. B., Dolan, R. J., and Niv, Y. (2016). Mood as representation of momentum. Trends Cogn. Sci. 20, 15–24. doi: 10.1016/j.tics.07010
Elliott, D. (2020). Selecting music for exercise: the music preferences of UK exercisers. Cogent Psychol. 7, 1802928. doi: 10.1080/23320201802928
Etkin, A., Egner, T., and Kalisch, R. (2011). Emotional processing in anterior cingulate and medial prefrontal cortex. Trends Cogn. Sci. 15, 85–93. doi: 10.1016/j.tics.2010.11.004
Forgas, J. P., and Eich, E. (2013). “Affective influences on cognition: Mood congruence, mood dependence, and mood effects on processing strategies”, in Handbook of psychology: Experimental psychology eds A. F. Healy, R. W. Proctor, I. B. Weiner (John Wiley & Sons, Inc) 61–82.
Gallese, V., and Goldman, A. (1998). Mirror neurons and the simulation theory of mind-reading. Trends Cogn. Sci. 2, 493–501. doi: 10.1016/S1364-6613(98)01262-5
Gilbert, A. L., Regier, T., Kay, P., and Ivry, R. (2006). Whorf hypothesis is supported in the right visual field but not the left. Proceed. Nat. Acad. Sci. 103, 489–494. doi: 10.1073/pnas.0509868103
Gobet, F., Retschitzki, J., and Voogt, d. e. A. (2004). Moves in Mind: The Psychology of Board Games. New York, NY: Psychology Press.
Harmon-Jones, E., and Sigelman, J. (2001). State anger and prefrontal brain activity: evidence that insult-related relative left-prefrontal activation is associated with experienced anger and aggression. J. Personal. Soc. Psychol. 80, 797. doi: 10.1037/0022-3514.80.5.797
Helion, C., Krueger, S. M., and Ochsner, K. N. (2019). Emotion regulation across the life span. Handbook Clin. Neurol. 163, 257–280. doi: 10.1016/B978-0-12-804281-6.00014-8
Izard, C. E., and Buechler, S. (1980). Aspects of consciousness and personality in terms of differential emotions theory. In Theories of emotion (pp. 165-187). Academic Press. doi: 10.1016./B978-0-12-558701-3.50013-2
Kammoun, A., Slama, R., Tabia, H., Ouni, T., and Abid, M. (2022). Generative adversarial networks for face generation: a survey. ACM Comp. Surv. 55, 1–37. doi: 10.1145/3527850
Keltner D. and Haidt, J.. (2003). Approaching awe, a moral, spiritual, and aesthetic emotion. Cogn. Emot. 17, 297–314. doi: 10.1080/02699930302297
Kim, H. G., Cheon, E. J., Bai, D. S., Lee, Y. H., and Koo, B. H. (2018). Stress and heart rate variability: a meta-analysis and review of the literature. Psychiatry Invest. 15, 235. doi: 10.30773/pi.0817
Ko, B. C. (2018). A brief review of facial emotion recognition based on visual information. Sensors 18, 401. doi: 10.3390/s18020401
Landauer, T. K., and Dumais, S. T. (1997). A solution to Plato's problem: the latent semantic analysis theory of acquisition, induction, and representation of knowledge. Psychol. Rev. 104, 211. doi: 10.1037/0033-295X.104.2.211
Lee, D. J., and Kim, Y. H. (2015). The effect of mood on the perception of room atmosphere. J. Environ. Psychol. 43, 19–26. doi: 10.1016/j.jenvp.2015.03.001
Li, S., and Deng, W. (2020). Deep facial expression recognition: a survey. IEEE Transact. Affect. Comp. 13, 1195–1215. doi: 10.1109/TAFFC.2020.2981446
Lopes, A. T., De Aguiar De Souza, E. A. F., and Oliveira-Santos, T. (2017). Facial expression recognition with convolutional neural networks: coping with few data and the training sample order. Patt. Recog. 61, 610–628. doi: 10.1016/j.patcog.07026
Lowe, W. (2001). Towards a theory of semantic space. In Proceedings of the Annual Meeting of the Cognitive Science Society. Vol. 23.
Lunn, R. M., Blask, D. E., Coogan, A. N., Figueiro, M. G., Gorman, M. R., Hall, J. E., et al. (2017). Health consequences of electric lighting practices in the modern world: a report on the national toxicology program's workshop on shift work at night, artificial light at night, and circadian disruption. Sci. Total Environ. 607, 1073-1084. doi: 10.1016/j.scitotenv.07056
Maas, A., Daly, R. E., Pham, P. T., Huang, D., Ng, A. Y., Potts, C., et al. (2011). Learning word vectors for sentiment analysis. In Proceedings of the 49th Annual Meeting of the Association for Computational Linguistics: Human Language Technologies (pp. 142-150).
Matsuo, M., Masuda, F., Sumi, Y., Takahashi, M., Yoshimura, A., Yamada, N., et al. (2019). Background music dependent reduction of aversive perception and its relation to P3 amplitude reduction and increased heart rate. Front. Human Neurosci. 13, 184. doi: 10.3389/fnhum.2019.00184
Mauss, I. B., and Robinson, M. D. (2009). Measures of emotion: a review. Cogn. Emot. 23, 209–237. doi: 10.1080/02699930802204677
McCormack, D. P. (2008). Engineering affective atmospheres on the moving geographies of the 1897 Andrée expedition. Cult. Geograph. 15, 413–430. doi: 10.1177/1474474008094314
McNair, D. M, Lorr, M, and Droppleman, L. F. (1992). Profile of Mood States. San Diego, CA: Educational and Industrial Testing Service.
Merla, A., and Romani, G. L. (2007). Thermal signatures of emotional arousal: a functional infrared imaging study. In 2007 29th Annual International Conference of the IEEE Engineering in Medicine and Biology Society (pp. 247-249). IEEE.
Millon, T., and Roger, D. (1995). Disorders of Personality: DSM-IV and Beyond, 2nd ed. New York: John Wiley and Sons.
Mulligan, K., and Scherer, K. R. (2012). Toward a working definition of emotion. Emot. Rev. 4, 345–357. doi: 10.1177/1754073912445818
Newell, A., and Simon, H. A. (1972). Human Problem Solving. Englewood Cliffs, NJ: Prentice-Hall (Vol. 104, No. 9).
Niedenthal, P. M., and Setterlund, M. B. (1994). Emotion congruence in perception. Personal. Soc. Psychol. Bullet. 20, 401–411. doi: 10.1177/0146167294204007
Pallasmaa, J. (2011). “Architecture and the Existential Sense.” In Art and the Senses, edited by Francesca Bacci and David Melcher. New York: Oxford University Press.
Paolo, D. i., and Thompson, E. (2014). “The enactive approach,” in The Routledge Handbook of Embodied Cognition (Routledge), (pp. 68-78)..
Pérez-Gómez, A. (2016). Attunement: architectural meaning after the crisis of modern science. MIT Press 3, 1. doi: 10.7551/mitpress/0010001
Prasomphan, S. (2015). “Improvement of speech emotion recognition with neural network classifier by using speech spectrogram,” In 2015 International Conference on Systems, Signals and Image Processing (IWSSIP) (pp. 73-76). IEEE. doi: 10.1109./IWSSIP.2015.7314180
Raichle, M. E. (2015). The brain's default mode network. Ann. Rev. Neurosci. 38, 433–447. doi: 10.1146/annurev-neuro-071013-014030
Richard, C. G., and Jason, B. C. (2015). The number of olfactory stimuli that humans can discriminate is still unknown. eLife. 4, e8127. doi: 10.7554/eLife.08127
Schumacher, P. (2016). Advancing social functionality via agent-based parametric semiology. Architect. Des. 86, 108–113. doi: 10.1002/ad.2031
Simon, H. (1967). Motivational and emotional controls of cognition. Psychol. Rev. 74, 29–39. doi: 10.1037/h0024127
Smith, C. A., and Ellsworth, P. C. (1985). Patterns of cognitive appraisal in emotion. J. Personal. Soc. Psychol. 48, 813. doi: 10.1037/0022-3514.48.4.813
Tarnowski, P., Kołodziej, M., Majkowski, A., and Rak, R. J. (2017). Emotion recognition using facial expressions. Proc. Comp. Sci. 108, 1175-1184. doi: 10.1016/j.procs.05025
Ulrich, R. S. (1983). “Aesthetic and affective response to natural environment,” in Behavior and the Natural Environment. Boston, MA: Springer, (pp. 85-125).
Valenzuela, J., Díaz-Andreu, M., and Escera, C. (2020). Psychology meets archaeology: psychoarchaeoacoustics for understanding ancient minds and their relationship to the sacred. Front. Psychol. 11, 550794. doi: 10.3389/fpsyg.2020.550794
Van Boxtel, A. (2010). “Facial EMG as a tool for inferring affective states,” in Proceedings of Measuring Behavior (Vol. 2).
Voynov, A., and Babenko, A. (2020). “Unsupervised discovery of interpretable directions in the GAN latent space,” in International Conference on Machine Learning (pp. 9786-9796). PMLR.
Wang, J., Yin, L., Wei, X., and Sun, Y. (2006). “3D facial expression recognition based on primitive surface feature distribution,” in 2006 IEEE Computer Society Conference on Computer Vision and Pattern Recognition (CVPR'06) (IEEE) (Vol. 2). doi: 10.1109/CVPR.2006.14
Wang, J., Yu, L. C., Lai, K. R., and Zhang, X. (2016). “Dimensional sentiment analysis using a regional CNN-LSTM model,” in Proceedings of the 54th Annual Meeting of the Association For Computational Linguistics (pp. 225-230).
Zadra, J. R., and Clore, G. L. (2011). Emotion and perception: the role of affective information. Wiley Interdiscip. Rev. Cogn. Sci. 2, 676–685. doi: 10.1002/wcs.147
Zangróniz, R., Martínez-Rodrigo, A., Pastor, J. M., López, M. T., and Fernández-Caballero, A. (2017). Electrodermal activity sensor for classification of calm/distress condition. Sensors 17, 2324. doi: 10.3390/s17102324
Zumthor, P. (2006). Atmospheres: Architectural Environments, Surrounding Objects. Basel, Boston: Birkhäuser.
Keywords: atmosphere, architecture, neuroscience for architecture, big data, moods, emotion recognition, architectural experience
Citation: Kirsh D (2023) Atmosphere, mood, and scientific explanation. Front. Comput. Sci. 5:1154737. doi: 10.3389/fcomp.2023.1154737
Received: 31 January 2023; Accepted: 26 July 2023;
Published: 16 October 2023.
Edited by:
Ava Fatah Gen Schieck, University College London, United KingdomReviewed by:
Michal Gath, University College London, United KingdomMaria Lorena Lehman, Independent Researcher, Coral Gables, FL, United States
Copyright © 2023 Kirsh. This is an open-access article distributed under the terms of the Creative Commons Attribution License (CC BY). The use, distribution or reproduction in other forums is permitted, provided the original author(s) and the copyright owner(s) are credited and that the original publication in this journal is cited, in accordance with accepted academic practice. No use, distribution or reproduction is permitted which does not comply with these terms.
*Correspondence: David Kirsh, ZGtpcnNoQGdtYWlsLmNvbQ==