- 1Institute of Transportation Systems, German Aerospace Center (DLR), Berlin, Germany
- 2Cognitive and Engineering Psychology, Department of Psychology, Chemnitz University of Technology, Chemnitz, Germany
- 3Institute of Transportation Systems, German Aerospace Center (DLR), Braunschweig, Germany
The successful integration of highly automated vehicles (HAV) in future mixed traffic environments will depend, among other things, on their seamless, safe, and accepted interaction with other road users. Therefore, appropriate combination of light signals, as external human-machine interface (eHMI), and driving behavior, as dynamic human-machine interface (dHMI), is required consistently in order to develop trust of following manual drivers in HAVs. Especially, in borderline traffic scenarios where HAVs are confronted with challenges, such as loss of connectivity, so-called minimal risk maneuvers (MRMs) are performed abruptly. Here, understanding communication via eHMI and dHMI is crucial for road safety, as drivers need to prepare for maneuvers themselves. Therefore, two consecutive, explorative online video studies were conducted. Firstly, the appropriate braking dynamics for an MRM were evaluated. Secondly, insights into the eHMI communication strategy of an HAV during an MRM were gained. The overall aim of this work is to present strategies for implicit and explicit communication channels of an HAV in order to promote learned trust during MRMs from the perspective of drivers who follow them. The results show that adding novel eHMI designs (e.g., warning sign, 360° LED light-band) to conventional light signals positively affects the user experience in a first contact interaction. The findings could have a positive impact on the development of trust in HAVs. In conclusion, specific eHMI communication strategies can be highly supportive for following manual drivers in MRM scenarios, which may lead to legislative considerations in the future.
1. Introduction
Ever-advancing technological trends in automated driving fuel the introduction of highly automated vehicles (HAV; SAE Level 4) into urban transportation (SAE., 2021b). In mixed traffic environments, successful deployment of HAVs will also depend on efficient and seamless interaction with other road users, e.g., cyclists, pedestrians, and drivers of conventional vehicles (Brown and Laurier, 2017; Josten et al., 2019; Schieben et al., 2019). So far, other road users only have little or no experience with HAVs, which leads to the challenge of how to develop trust in HAVs. Moreover, high usability and high value of information content are necessary to ensure safe interaction and well-functioning deployment in traffic to promote learned trust. Therefore, adequate communication between HAVs and road users is required. Here, two distinctive communication channels are used to enable well-working interaction with surrounding road users (Bengler et al., 2020). On the one hand, external human-machine interfaces (eHMIs) could transmit explicit communication signals in terms of conventional light signals (e.g., turn indicators) and novel concepts (e.g., 360° LED light-bands). On the other hand, the vehicle's motion transmits implicit communication signals to its surrounding environment and is often referred to as dynamic HMI (dHMI). Research manifested benefits of harmonizing both communication channels (Lau et al., 2021b). This seems to be important to foster safety, understanding, acceptance, and trust in HAVs. Comprehension and trust are of particular importance in traffic situations in which the HAV reaches its limits of operation, either due to technical issues, unforeseen traffic and weather circumstance or when leaving its operational design domain (ODD). In such situations, the automation acts responsibly and puts the vehicle in a so-called minimal risk condition (MRC) for safety. However, the HAV most often will not stop suddenly but rather perform a safe and deliberate stopping maneuver, which is called minimal risk maneuver (MRM). Here, the explicit and implicit communication channels of the HAV are critical to inform the interacting road users. Particularly, following manual drivers need to understand the situation and intention of the HAV. They must react appropriately to the MRM ahead of them and prepare suitably for a safe maneuver themselves. It is important that the explicit and implicit communication of the HAV is compliant and comprehensible for the following drivers.
Thus, this paper focuses on the holistic communication strategy of dynamic and external HMI of an HAV during a minimal risk maneuver. Hence, the following research question was addressed: To build trust and ensure road safety, how should HAVs communicate during an MRM to the following driver? Therefore, this paper follows a two-folded research approach by conducting two consecutive user studies. First, appropriate vehicle braking dynamics (dHMI) in terms of longitudinal deceleration during MRMs were evaluated in a first study. Second, insights into the explicit communication (eHMI) strategies of HAVs during MRMs were gained in a second user study. The second user study builds on the results of the first study. The overall goal of this work is to present explicit and implicit communication strategies so that MRMs are perceived as understandable, rather uncritical, and of an acceptable level of user experience in order to promote trust in HAVs from a following driver's perspective.
2. Theoretical background
The following sections describe the theoretical background of this work, namely, external and dynamic HMIs of HAVs, minimal risk maneuvers, and trust in HAVs. Subsequently, the research question of this paper is presented in detail.
2.1. Visual external communication of HAVs with other traffic participants
Effective communication between HAVs and other road users is highly relevant for the well-working deployment of HAVs and an overall safe interaction in the near future (Merat et al., 2018; Schieben et al., 2019). Therefore, external communication strategies of HAVs are used to communicate deliberately with the surrounding traffic to ensure safety and trust (Schieben et al., 2019; Bengler et al., 2020). In general, external communication can take place implicitly, e.g., via vehicle dynamics, or explicitly, e.g., via light signals (Sucha et al., 2017; Bengler et al., 2020).
Vehicles transmit information implicitly to other road users via driving dynamics, often referred to as dynamic human-machine interface (dHMI), which can help in anticipating the vehicle's intention (Risto et al., 2017; Bengler et al., 2020). For example, the vehicle's braking behavior at a crossing can inform pedestrians implicitly about an intended yielding (Dietrich et al., 2020). On a straight road, the temporal changes in the longitudinal position characterize the braking behavior of the vehicle (Dietrich et al., 2020). Accordingly, for the design of dHMIs during stopping maneuvers, the longitudinal component of the vehicle's braking behavior is highly relevant and will be focused on in the following. Moreover, for the design of appropriate dHMIs, a holistic perspective needs to be considered in which, on the one hand, the perspective of the passengers inside the vehicle, and on the other hand, the perspective of the surrounding road users is addressed (Bengler et al., 2020; Hesse et al., 2021). Regarding the passenger's perspective, continuous braking of a partially automated vehicle with a deceleration rate of −1.35 m/s2 is perceived as pleasant and well-timed (Scherer et al., 2016). The consideration of defensive braking behavior from the passenger's point of view also has support from other studies (Lange et al., 2014; Festner et al., 2017). Focusing on the perspective of the surrounding road users, pedestrians at crosswalks prefer a gentle, automated or manual deceleration of about −1.0 m/s2 to a late, more severe deceleration of −2.0 m/s2 (Bazilinskyy et al., 2021). Furthermore, strong deceleration followed by coasting was perceived as cooperative behavior by the surrounding road users (Dietrich et al., 2020). However, passengers may perceive this dHMI as uncomfortable. This demonstrates the importance of a holistic perspective when designing dHMIs. As limitation, current research on dHMIs often lacks the perspective of the following road users and, therefore, so far, no clear statements can be made about the appropriate braking behavior during stopping maneuvers of HAVs in traffic.
Additionally, vehicles can transmit information explicitly to surrounding road users via conventional light signals (e.g., turn indicators) and novel concepts (e.g., light emitting concepts like a 360° LED light-band), which is often referred to as external human-machine interface (eHMI) (Bazilinskyy et al., 2019; Rouchitsas and Alm, 2019; Schieben et al., 2019, 2020; Dey et al., 2020; Lau et al., 2021b; Wilbrink et al., 2021). In today's traffic, at least in Germany drivers can indicate their initiated stopping maneuver by actuating their turn indicator (Färber, 2016). Stimuli that can activate relevant schemata or mental models are easy to identify (Endsley, 1995). Hence, practitioners should implement these saliently in eHMIs, especially when facing potentially critical events like MRMs. In that sense, the adoption of symbols (like conventional traffic signs), explicit messages, or even anthropomorphic visuals via displays on HAVs has been subject to research (Dey et al., 2020; Rouchitsas and Alm, 2022, 2023). The question arises how HAVs will communicate intention, perception or otherwise in the near future with their surrounding environment, e.g., by conventional already existing eHMIs, e.g. indicators, novel eHMIs or a combination of both. One possible solution would be the design of 360° LED light-band eHMIs that enable high visibility for all road users around the vehicle (Weber et al., 2019; Dey et al., 2020; Wilbrink et al., 2021). Regarding the effects of light-band eHMIs, research showed that pedestrians trust an HAV equipped with eHMI more compared to no eHMI (Kaleefathullah et al., 2022). They also felt safer when the HAV was equipped with an eHMI indicating the vehicle's yielding intent compared to no eHMI or an eHMI only displaying the vehicle's automation status (Lau et al., 2022). Mirnig et al. (2021) investigated the use of an intention-based light-band eHMI for a highly automated shuttle and showed that the eHMI supported a smooth interaction between the shuttle and the surrounding traffic. The authors stated that the eHMI helped other road users to better understand the vehicle's intention and, accordingly, to adapt their behavior to the shuttle's behavior. This is supported by other studies showing that pedestrians felt better informed when the HAV is equipped with a LED light-band eHMI (Lau et al., 2021a). In general, it is recommended that these eHMIs should be aligned with the dHMI to prevent misunderstandings (Bengler et al., 2020; Schieben et al., 2020; Hesse et al., 2021; Wilbrink et al., 2021). In this context, cooperative stopping and yielding maneuvers could be performed with a 360° LED light-band eHMI in combination with an appropriate dHMI, i.e., strong deceleration in the beginning that decreased linearly (Avsar et al., 2021). Results showed that the eHMI was perceived as supportive and was well understood by the interacting manual drivers after only a few trials and a coherent combination of eHMI and dHMI was rated with a high usability.
However, so far, only little research has focused on the design of eHMIs for the interaction of an HAV with its following road users (Powelleit et al., 2018; Schindler et al., 2020a). Overall, the well-coordinated interplay of both external communication signals seems important to enhance the interaction in mixed traffic environments and to foster safety, understanding, acceptance, and trust in HAVs (Bengler et al., 2020; Kaleefathullah et al., 2022; Lau et al., 2022). However, it is not yet defined how exactly this interplay should take place, in particular, during a stopping maneuver, i.e., an MRM. Therefore, this study addresses the question of how both external communication channels should be utilized to build trust in HAVs and increase traffic safety.
2.2. Minimal risk maneuver
Safe HAV operation requires proper maneuvers and seamless road user interaction in all traffic scenarios. For HAVs (SAE level 4), the automated driving system (ADS) takes full control over the vehicle and no driver backup is necessary. In level 4 automation, HAVs will operate in geographically and functionally defined environments. The ADS is particularly designed and well trained for these operational design domains (ODD). Although the ADS should be capable of handling all driving scenarios by itself, system failures or sensor errors may still occur. For example, if an HAV finds itself in circumstances of false detection, misperception (i.e., in highly complex traffic scenarios which are not solvable), vehicle malfunction, or leaving its ODD the vehicle needs to stop and put itself into a so-called minimal risk condition (MRC) (Gertz et al., 2021; SAE., 2021a). A MRC is defined as “stable, stopped condition to which a user or an ADS may bring a vehicle after performing the DDT [dynamic driving task] fallback in order to reduce the risk of a crash when a given trip cannot or should not be continued” (SAE., 2021a). Hence, this means that all AVs of higher automation levels (level 3 and higher) need to be able of performing a specific driving maneuver to get into a MRC at any times. This driving maneuver is called minimal risk maneuver (MRM) and often preferably aims to put the vehicle safely in an MRC on the road's hard shoulder (Karakaya et al., 2020).
Nowadays, it is unclear how exactly an MRM is to be performed by the ADS. In the future, this could depend on factors such as vehicle specification (type, size, etc.) and vehicle registration (Reschka, 2016). Additionally, also failure type and magnitude for the respective traffic scenario could play a role whether to conduct an MRM (SAE., 2021a). MRMs as a method to ensure traffic safety are of high importance and magnitude in future HAV design and deployment (Gertz et al., 2021). In situations where MRMs are performed, each HAV needs to communicate its intentions with passengers and surrounding road users comprehensively (Karakaya et al., 2020; Nyberg et al., 2021). Other connected AVs could receive such information via V2V communication protocols (Schindler et al., 2020b). However, non-connected road users and especially following manual drivers have to be informed, too. We assume that the following the drivers need to comprehend the behavior of the HAV because it is highly important to react safely and prepare for an appropriate maneuver themselves. Especially in such borderline traffic situations, effective and unambiguous external communication between HAVs and following drivers is the key to ensuring road safety and for fostering trust in HAVs. It is important that drivers are capable of recognizing the MRM as such. This means that they should be able to tell the difference between a planned and unplanned HAV stop, because appropriate reactive maneuvers and consequences of wrong behavior differ (e.g., a planned curbside stop could imply that people may cross the street soon). Utilizing synchronized eHMI and dHMI communication channels and strategies present a plausible way to communicate HAV intention and behavior to following drivers (Schindler et al., 2020a). However, this aspect has not been sufficiently studied yet. Although HAVs are required by German law to utilize hazard warning lights to communicate MRC, it remains unclear how the MRM should be communicated visually (BfJ, 2003, 2013a,b). Assuming that in the eyes of following drivers, MRMs are conducted spontaneously and unforeseen, matching explicit and implicit communication of HAVs can be seen as essential in effectively preventing critical situations and collisions. By exceeding legislative requirements and utilizing HMI strategies when performing the MRM, gained insights for emergency stimuli of drivers could potentially promote safety and trust (Gasser et al., 2012).
2.3. Trust
To describe trust in general, this paper uses the definition from Lee and See (2004) who define trust as “the attitude that an agent will help achieve an individual's goals in a situation characterized by uncertainty and vulnerability”. Hence, uncertainty plays an important role to trust and is defined as a lack of information about an agent or environment, which causes an inability to predict another agent's behavior (Baxter and Montgomery, 1996; Kramer, 1999). Uncertainty increases humans' engagement with systems or agents in the search for information. For example, when individuals meet, they interact communicatively in order to exchange information with regard to each other's intentions. In doing so, both agents interact to reduce uncertainty. Following this logic, trust and uncertainty are inversely related (Lewis and Weigert, 1985; Colquitt et al., 2012).
Trust in automated systems can be classified into three layers (Marsh and Dibben, 2003; Hoff and Bashir, 2015). Firstly, dispositional trust is independent of context or agents/systems, but rather presents long-term characteristics like age, culture, gender, or personality traits. Secondly, situational trust is dependent on the context of interaction and can be changed, e.g., by varying a task, circumstances, or agent to interact with. Thirdly, learned trust is based on personal experiences with a system or agent. Learned trust is a dynamic process and can be influenced by experiences as well as current interactions, in which reliability plays an important role (Lee and See, 2004). Raats et al. (2019) summarize the three layers of trust in automated vehicles and state that trust is felt by users and that it is influenced by personal traits, cultural context, and evolves over time through experiences. Trust between humans and machines is characterized by the formation of trust over time through experiences (Muir, 1987). Accordingly, design and development indirectly influence the learned trust of HAVs. Performance (e.g., effort to engage with a system, wellbeing during use, reliance, or situational awareness) of novel systems and traditional user experience (UX) metrics play a highly important role when forming trust with the system (Raats et al., 2019). Hence, the design of explicit and implicit communication channels of HAVs in the form of eHMI and dHMI to following manual drivers needs to be of high informational content and highly comprehensible. Available information, which is easy to understand, supports the driver's prediction of the actions of an HAV during an MRM and assists him or her to react safely. Hence, cautious HMI design for HAV MRMs reduces uncertainty and learned trust in the HAV can be developed consequently. Especially, first interactions with HAV MRMs are of interest for this work to lay out foundations for trust-building factors.
2.4. Research questions
Although HAVs are required to utilize hazard warning lights as a form of conventional eHMI to communicate MRC, currently it remains unclear how the MRM should be signaled toward surrounding road users and especially to following manual drivers. Based on the theoretical background presented above, this work answers the following research question: How should HAVs communicate an MRM implicitly via dHMI (first study) and explicitly via eHMI (second study) to following drivers so that trust and traffic safety is fostered?
Assuming that MRMs are conducted rather spontaneously and unforeseen from the perspective of following manual drivers, explicit and implicit communication of HAVs should be clear and easy to comprehend only when other road users understand and interpreted the behavior and signals of HAVs correctly, critical situations or even collisions are prevented, and traffic safety is improved (Färber, 2016). With comprehensive and unambiguous communication between road users, a common situation awareness and considerate traffic behavior can be achieved. It has been pointed out that HAV behavior is especially difficult to interpret by other road users (Brown and Laurier, 2017; Boersma et al., 2018; Schieben et al., 2019). Especially, for the novel use cases of MRMs, this could prove to be a risk factor for the interaction with road users, respectively. Accordingly, clear and efficient explicit and implicit HAV communication to following drivers needs to be assured. Besides safety, HAV communication toward following manual drivers should provide a sufficient informative value to decrease perceived criticality of MRMs and to foster overall trust in automation, which is seen as a major factor to influence the designated market success of HAVs (Körber, 2019; Morgan et al., 2019; Paddeu et al., 2020). To promote acceptance of HAVs as omnipresent road users, trust must be developed through interactions that are characterized by high pragmatic quality (as a dimension of user experience). Adequate HMI design makes these interactions during HAV maneuvers more comprehensible and affects the development of trust positively (Hoff and Bashir, 2015). Explicit communication strategies via eHMI should guide following drivers toward appropriate reactive driving behavior.
By following an explorative research approach, the goal of this work is to present explicit and implicit communication strategies that are perceived as uncritical, are of high pragmatic quality in terms of user experience, and foster understanding and predictability of HAV behavior during MRM from a following driver's perspective. Furthermore, this work investigates which eHMI information is sufficient from a following driver's perspective to fully understand the intention of the HAV and create situation awareness. Therefore, two consecutive explorative online video studies were conducted. Firstly, appropriate vehicle dynamics (dHMI) during an MRM were evaluated. Secondly, insights into the eHMI communication strategy of an HAV during an MRM were gained.
3. First study: identifying appropriate braking dynamics for MRMs
The next section focuses on the first of the two user studies. The objective of this exploratory study was to find patterns for vehicle dynamics (dHMI), so that following drivers associate the HAV maneuver as rather unplanned.
3.1. Method
The first study was conducted as an initial experimental online study based on rendered videos. MRM performing HAVs were investigated from the perspective of manual drivers following an automated shuttle. The HAVs implicit communication was evaluated regarding the quality of information, trust in automation, criticality, and traffic safety.
3.1.1. Sample
Participants were recruited via personal contacts, via the e-mail distribution list for study participations of Chemnitz University of Technology, Germany, and via the subject database plus LinkedIn website of the Institute of Transportation Systems of the German Aerospace Center. A total of 29 participants were excluded because either most of them, according to data quality checks, did not confidently perceive differences in the HAV driving behavior between conditions or, they completed the questionnaires disproportionately quick (Leiner, 2019). Hence, research data from N = 102 participants (female: 44) aged 19 to 82 years (M = 37.34, SD = 15.17) was included in this first study. Requirements for participation were a minimum age of 18 years and possession of a driver's license for passenger cars, which participants had held for M = 18.79 years (SD = 14.33 years). Annual car mileage of the participants was M = 9,990 km (SD = 8,802 km). The median frequency of car use was reported as “several times per week”, based on a rating scale (“daily”, “several times per week”, “about once per week”, “about once per month”, “less frequently than once per month”, and “never used”). Participants' personal driving styles averaged at M = 3.09 (SD = 1.05), based on a 5-point Likert scale (from 1 = “comfortably” to 5 = “dynamically”). Only six participants (approximately 6%) were not familiar with the topic of automated vehicles. General interest in automated vehicles was M = 3.69 (SD = 1.13), based on a 5-point Likert scale ranging from 1 = “not at all” to 5 = “very strongly”. According to the 6-point Likert scale from 1 = “completely disagree” to 6 = “completely agree”, the Affinity for Technology Interaction questionnaire (ATI, Franke et al., 2019) resulted in a higher score of M = 4.15 (SD = 1.05, α = 0.93) than found for the quota sample reported by Franke et al. (2019). The study was designed and conducted in accordance with the Declaration of Helsinki. Informed consent was obtained from participants before the online experiment. All participants volunteered and had the chance to win one of four universal vouchers worth €25 in a prize draw.
3.1.2. Independent variables
The study was implemented using a 3 × 3 mixed design. The first independent variable was the within-subjects factor braking deceleration as implicit communication (dHMI), for which the longitudinal driving behavior was varied. Braking values selected seemed conceivable from the holistic perspective as well as for MRMs (cf. [2.1]). Thus, “defensive braking” (−1.0 m/s2), “moderate braking” (−2.0 m/s2), and “hard braking” (−2.5 m/s2) were chosen as factor levels. Since in Germany motorized road users are obliged to use light signals to indicate stopping maneuvers, it seemed reasonable to consider relevant conventional light signals as well. Therefore, the between-subjects factor light signal with the levels “no light signal”, “turn signal”, and “hazard warning lights” were used as the second independent variable. Mandatory brake lights were visible during braking in all conditions. The respective light signal became present or not (in the case of “no light signal”) from the point of braking initialization and remained active for the complete braking maneuver. Consequently, each participant saw three different videos including all levels of braking deceleration as within-subjects factor (“defensive braking”, “moderate braking”, and ”hard braking”) combined with one light signal condition as between-subjects factor (“no light signal”, “turn signal”, or “hazard warning lights”).
3.1.3. Dependent variables
The independent variables braking deceleration and light signal were examined using a set of dependent variables, which, as stated in the previous chapters, corresponded to criteria presumed as highly relevant to a following driver's trust building and feeling of safety. Accordingly, a modified version of the understanding/predictability subscale of the standardized Trust in Automation questionnaire (TiA, Körber, 2019) was used to assess the behavior of the HAV during the execution of the stopping maneuver. The four items were adapted (“The behavior of the shuttle was always clear to me”, “I was able to understand why things happened” alternating by the inverse items “The shuttle reacted unpredictably” and “It's difficult to identify what the shuttle will do next”) and applied with a 6-point Likert scale (from 1 = “completely disagree” to 6 = “completely agree”). The mean value across all four items served as understanding/predictability score.
A 6-point Likert scale (from 1 = “completely disagree” to 6 = “completely agree”) was also used for one additional item (“It was clear to me how to adjust my own driving behavior in this situation.”). In this way, the extent to which the behavior of the HAV was helpful for following drivers in preparing for action was examined, which depicts a follow-up step regarding understanding the traffic situation.
Perceived quality of information, as a crucial component to building trust, was assessed by the item “How well did you feel informed about the shuttle's behavior in this situation?”, based on a 7-point Likert scale (from 1 = “very bad” to 7 = “very good”). Quality of information was further subdivided into information content of braking behavior (“The braking movement of the shuttle helped me to assess its behavior.”) and information content of light signals (“The light signals of the shuttle helped me to assess its behavior.”), which both were measured using a 6-point Likert scale (from 1 = “completely disagree” to 6 = “completely agree”).
Criticality of the traffic situation, which is also important for building trust in automated systems, was assessed by the item “How critical was the shuttle's stopping maneuver to you personally?” using a 7-point Likert scale ranging from 1 = “not critical at all” to 7 = “very critical”.
In order to draw conclusions about the extent to which the stopping maneuver of the HAV was identified as a scheduled stop or an emergency stop (e.g., an MRM) participants completed the two items (“This situation is a scheduled stop of the shuttle, I consider...” and “This situation is an emergency stop of the shuttle, I consider...”) by using a 7-point Likert scale ranging from 1 = “not likely at all” to 7 = “absolutely likely”.
3.1.4. Materials
The videos used in this experimental online study were designed with the 3D game engine Unreal Engine (version 4.26.2). Each video clip had a high resolution (1,920 × 1,080 pixels) and lasted approximately 15 s. All videos showed the same two-lane straight road in an urban environment. The perspective of the videos corresponded to the field of view of a person at the steering wheel of a passenger car driving behind an HAV. The 3D model of an EasyMile EZ10 shuttle with customized exterior (no company labels, no additional paintings) was chosen as HAV.
In all videos, initial speeds of HAV and passenger car experienced for the participants as manual drivers were 30 km/h. Time headway of the HAV with respect to the passenger car (ego vehicle) was 2 s (Figure 1 top left) as time headways of < 2 s are perceived as unpleasant by following drivers (Siebert et al., 2014). After the HAV initialized braking, the ego vehicle replicated the braking behavior of the HAV with a delay of 1 s, simulating the human reaction time to known signals such as the brake lights of a lead vehicle (Green, 2000). In addition, the braking behavior of the vehicle in front can have a significant effect on the braking behavior of the vehicle behind. By synchronizing the driving dynamics of the ego vehicle with the dHMI of the HAV, this has been taken into account.
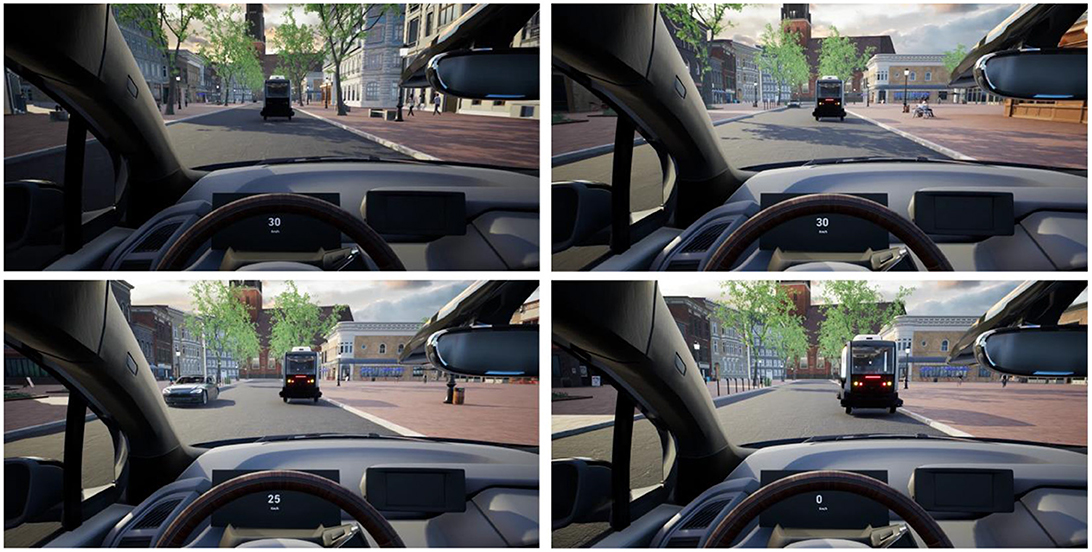
Figure 1. Demonstration of the stopping maneuver of an HAV exemplarily for the test condition “moderate braking, hazard warning lights” in four stages. Top left: Start of driving (t = 0 s). Top right: Begin of braking process (t = 8 s). Bottom left: During the braking process (t = 10 s). Bottom right: Final position of the HAV curbside (t = 14 s).
In each condition, the HAV traveled a total distance of approximately 80 m, stopping at the same curbside position, regardless of the respective braking behavior (see Figure 1 bottom right). The braking deceleration was applied uniformly, so the stopping distance varied accordingly from 34.72 m (“defensive braking”) to 17.36 m (“moderate braking”) to 13.89 m (“hard braking”). To keep video durations constant, the time span the HAV was visible at the final stopping position, i.e., in MRC, varied slightly. In all videos, the brake lights of the HAV were correctly indicated during braking maneuvers by three brake lights, including a high-mounted brake light. The kinematic cue of the lateral driving dynamics of the HAV was kept to a minimum as approaching curbside was carried out linearly throughout the entire braking distance. For the ego vehicle, no lateral dynamics were implemented. In none of the videos was any indication of a stopping situation visible, such as waiting passengers or corresponding signage. There was the same oncoming traffic (one vehicle; see Figure 1 bottom left) in all videos. All signal lights, including brake lights, were designed to be visible exactly at the time of the braking initiation (see Figure 1 top right).
3.1.5. Procedure
The online study was set up using SoSci Survey (https://www.soscisurvey.de). Data collection took place from February to March 2022 and was conducted in German. Participants completed the survey using their own computer, tablet, or smartphone. A welcome page provided information about the study's research topic. Subsequently, participants gave their consent to data processing (in compliance with the German general data protection regulation [DSGVO]) and assured participation requirements. A video streaming and connectivity test was conducted. Thereafter, the traffic situation was illustrated. Participants were instructed that they would be driving a passenger car as ego vehicle themselves in an urban area and following a fully automated shuttle while driving. For clarification, an image of the automated shuttle before the initialization of the braking maneuver was shown (see Figure 1 top left). Subsequently, participants watched the video clips in balanced order according to the 3 × 3 mixed design. Then they filled in the questionnaires, which always included the same items (of all dependent variables). At the end, demographic data was collected and participants were offered to participate in the raffle of the universal vouchers.
3.2. Results
3.2.1. Data preparation and analysis
Statistical analyses were performed using IBM SPSS Statistics, version 26. To gain exploratory insights, univariate, repeated measures ANOVAs were conducted according to the 3 × 3 mixed design. By means of Kolmogorov-Smirnov tests, it was determined that data was not normally distributed across experimental conditions in most cases (approximately 83%). The Levene tests showed that no variance homogeneity was given in some conditions of the factor light signal (approximately 17%). Violations of normal distribution and of variance homogeneity can be neglected for ANOVAs, especially when the group sizes of the between-subjects factor are equal (Pagano, 2013). This applied for light signal conditions at 34 participants each. Mauchly tests revealed that sphericity was present for all dependent variables. Effect sizes for partial η2 were classified according to Cohen's conventions (Cohen, 1988) as small (0.01), medium (0.06), or large (0.14). Pairwise comparisons were made via post hoc tests always using the Bonferroni correction. For all inferential statistical analyses level of significance (α) was set to 0.05 by default.
3.2.2. Results regarding factor “braking deceleration”
Overall, all dependent variables were rated relatively high by the participants, which indicates that the presented stopping maneuvers of the HAV were perceived as comprehensible, informative, and uncritical. However, a significant main effect was found for braking deceleration with large effect sizes for understanding/predictability, perceived quality of information, information content of braking behavior, information content of light signals, and criticality (see Table 1). For preparing for action, a medium effect could be shown. Additionally, braking deceleration had a significant effect on being considered as a scheduled stop or emergency stop, showing large effect sizes.
Pairwise comparisons showed that “defensive braking” was rated higher than “moderate braking” and “hard braking” for the criteria understanding/predictability (M = 4.68, SD = 1.00 vs. M = 3.84, SD = 1.21 and M = 3.71, SD = 1.22), preparing for action (M = 5.29, SD = 0.96 vs. M = 4.88, SD = 1.07 and M = 4.86, SD = 1.19), perceived quality of information (M = 5.64, SD = 1.47 vs. M = 4.44, SD = 1.74 and M = 4.28, SD = 1.70), information content of braking behavior (M = 4.75, SD = 1.10 vs. M = 4.18, SD = 1.30 and M = 4.04, SD = 1.26), information content of light signals (M = 4.96, SD = 1.25 vs. M = 4.25, SD = 1.54 and M = 4.14, SD = 1.48), and less critical (M = 2.06, SD = 1.27 vs. M = 3.15, SD = 1.63 and M = 3.47, SD = 1.72) (all p < 0.001). Furthermore, the behavior was significantly more likely to be judged as a scheduled stop when the HAV conducted “defensive braking” (M = 5.18, SD = 1.77) than “moderate braking” (M = 3.99, SD = 1.70) or “hard braking” (M = 3.86, SD = 1.75), all p < 0.001. Conversely, the behavior of the HAV was significantly more likely considered as an emergency stop when “hard braking” (M = 3.72, SD = 1.69) or “moderate braking” (M = 3.66, SD = 1.62) applied than when “defensive braking” (M = 2.48, SD = 1.53) (all p < 0.001). No significant differences were found between the factor levels “moderate braking” and “hard braking” with respect to any of the investigated criteria. Achieved statistical power was consistently high regarding the within-subjects factor braking deceleration (all >0.99).
3.2.3. Results regarding factor “light signal”
For the between-subjects factor light signal, a significant main effect with medium effect sizes was found regarding understanding/predictability, perceived quality of information, information content of light signals, and criticality (see Table 2). No significant differences could be shown for remaining investigated criteria. Pairwise comparisons showed that the braking behavior of the HAV was significantly more understandable or predictable with “turn signal” (M = 4.59, SD = 0.99) than with ”hazard warning lights” (M = 3.92, SD = 0.97; p = 0.012) or “no light signal” (M = 3.72, SD = 0.87; p = 0.001), respectively (see Figure 2). “Turn signal” (M = 5.42, SD = 1.22) was also rated significantly higher than “no light signal” (M = 4.24, SD = 1.30) in terms of perceived quality of information (p = 0.001), which was explicitly confirmed for information content of light signals, where “turn signal” (M = 4.93, SD = 0.91) was also rated significantly higher than “no light signal” (M = 3.96, SD = 1.41), p = 0.003. In addition, “turn signal” (M = 2.47, SD = 1.27) was rated significantly less critical than “no light signal” (M = 3.25, SD = 1.08) (p = 0.042). For the criteria preparing for action, information content of braking behavior and scheduled or emergency stop, no significant main effect of light signal was revealed. For none of the criteria a significant interaction between the factors braking deceleration and light signal was found.
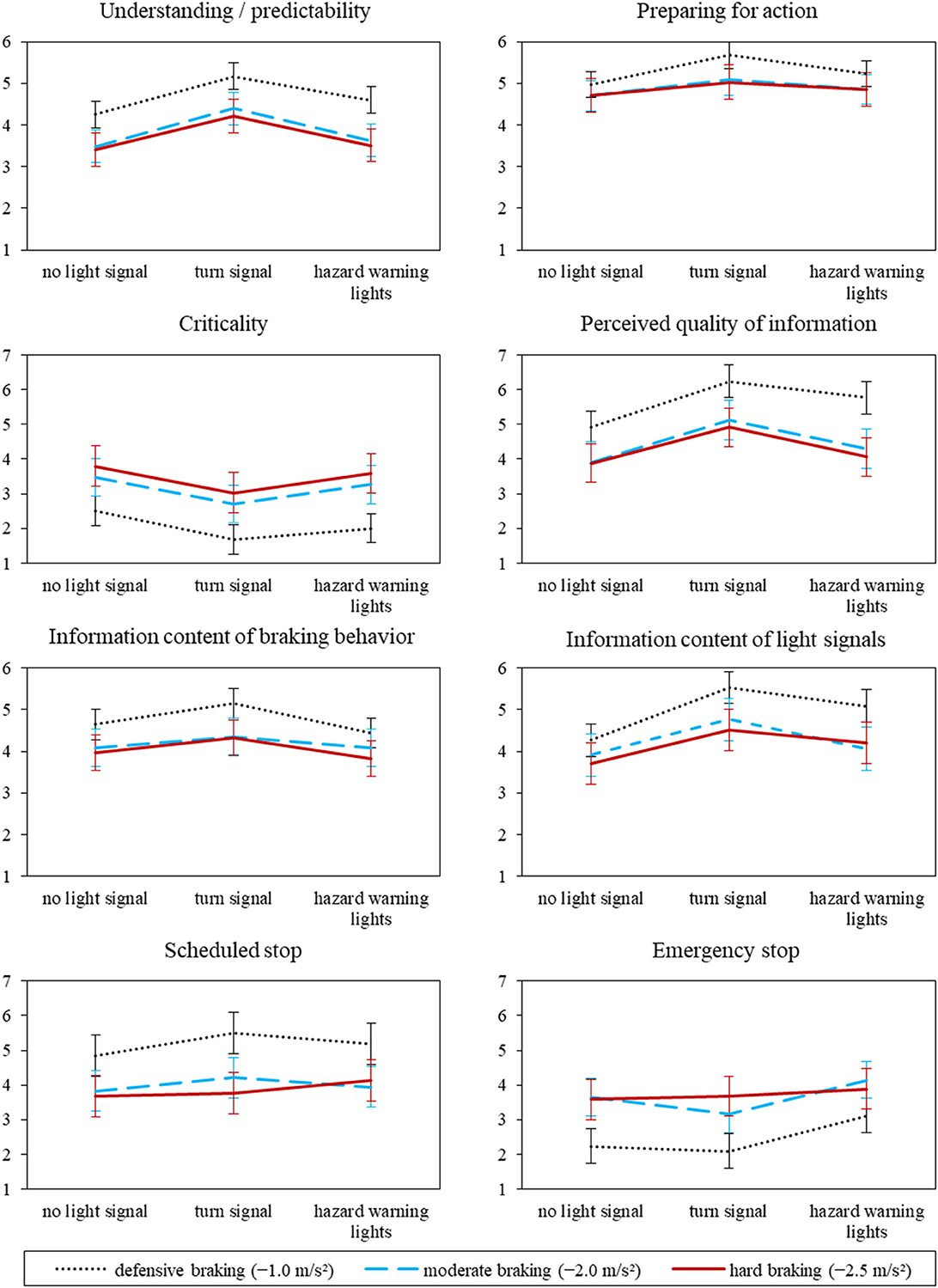
Figure 2. For each criterion, mean ratings of braking deceleration per light signal are displayed. Vertical axes show ratings per criterion. Error bars indicate 95% confidence intervals.
3.3. Implications for study 2
In respect to following drivers' need for information and trust in automation, the study results reveal that a defensive braking pattern (−1.0 m/s2) should be used whenever possible. Although harder braking will reach MRC more quickly, recent research reports hard braking (e.g., Gertz et al., 2021) to be rather unacceptable for MRMs. Time is likely to be a factor when considering MRMs. However, different braking decelerations are conceivable or even unavoidable depending on the specific scenario. Nevertheless, in this present use case of conducting an MRM it may be more appropriate to suggest “moderate braking” or “hard braking” for HAVs because the dHMI clearly signals an emergency stop. Also, when considering dHMIs it is reasonable to view the holistic perspective as any surrounding road users and passengers can be affected by the vehicle dynamics of the HAV (Bengler et al., 2020). Specifically, previous findings show that rather low braking dynamics are preferred (Lange et al., 2014; Festner et al., 2017; Bazilinskyy et al., 2021). No significant differences were found for any of the criteria. Hence, “moderate braking” is to be preferred over “hard braking” to account for this holistic perspective. Therefore, “moderate braking” at −2.0 m/s2 seems to be an applicable longitudinal braking deceleration to inform following drivers properly about an upcoming MRM in order to promote their trust in automation. In terms of conventional light signals, higher ratings for stopping maneuvers in which “turn signal” was used compared to “hazard warning lights” or “no light signal” suggest that it might be reasonable for HAVs to use the turn signal when stopping curbside.
4. Second study: identifying trust building eHMI designs for MRMs
In the following the second study is described in detail. Objective of this second user study was to investigate specific eHMI strategies for an MRM performing HAV to foster following manual drivers' trust in automation. Explicit communication strategies consisting of novel eHMI designs and conventional light signals were evaluated.
4.1. Method
MRMs of HAVs can lead to critical traffic situations where, consequently, it is crucial that following manual drivers understand the driving behavior of the HAVs. In this context, eHMIs have the potential to support following drivers, especially as MRMs are a novel phenomenon in traffic. Thereby, eHMIs need to maintain predictability of the driving behavior of the HAV, prevent situations of uncertainty, and ensure traffic safety. All these criteria affect learned trust in HAVs as well as a positive user experience of the eHMI designs as explicit communication. Similar to the first exploratory study, this second user study was conducted as an experimental online study using rendered videos. The same viewing angle (a manual driver's seat of a vehicle following an HAV) was chosen and the automotive shuttle model (Easymile EZ10) was used to simulate MRMs. The first study showed that “moderate braking” may be best practice for MRMs from a holistic perspective. Therefore, “moderate braking” with −2.0 m/s2 longitudinal deceleration was used, as a matching combination of HAVs dHMI and eHMI should be aspired at all times.
4.1.1. Sample
For this second study, participation requirements and recruitment were the same as in the first study. Furthermore, as additional recruitment channels, the bulletin boards of the Institute of Psychology at Martin Luther University Halle-Wittenberg, and the Faculty of Mechanical Engineering at Leibniz University Hannover were used. Only complete data sets were considered for data analysis. Furthermore, according to data quality checks, data sets of six subjects were excluded. In total, research data of N = 97 participants (38 female, 58 male, 1 other) aged between 18 and 77 years (M = 38.39, SD = 16.44) were included for further analysis. Participants held a driver's license for M = 20.28 years (SD = 15.99 years). As in the first study, median frequency of passenger car use was reported as “several times per week” and participants' average annual car mileage was M = 9.570 km (SD = 8.888 km), comparably. The same was true for participants' personal driving styles, which was in the medium range with M = 2.98 (SD = 1.04), again, based on a 5-point Likert scale from 1 = “comfortably” to 5 = “dynamically”. Only one participant was unfamiliar with the topic of automated vehicles. Interest in automated vehicles was reported slightly higher at M = 4.01 (SD = 1.07) compared to the first study, based on the same 5-point Likert scale ranging from 1 = “not at all” to 5 = “very strongly”. Attitudes toward automated vehicles tended to be positive on a 5-point Likert scale (from 1 = “negative” to 5 = “positive”) with M = 3.95 (SD = 1.08). As in the first study, the scores of the ATI questionnaire (Franke et al., 2019) showed an above-average mean of 4.36 (SD = 1.00, Chronbach's α = 0.93), again, based on the 6-point Likert scale ranging from 1 = “completely disagree” to 6 = “completely agree”. The study was conducted in accordance with the Declaration of Helsinki. Like the first study, all participants volunteered, gave their informed consent before the online experiment had begun, and at the end of the study, they had the chance to win one of four universal vouchers worth €25 each in a raffle.
4.1.2. Independent variables and HMI design
The different eHMI strategies were investigated using a single-factor design, containing the within-subject factor eHMI design, which had six factor levels.
All factor levels of eHMI design included hazard warning lights. These conventional, learned signals are presumably associated with emergencies in Germany because “hazard warning lights may only be switched on by those who endanger others through their vehicle or who wish to warn others of danger” (BfJ, 2013b). In fact, considering Germany, a HAV would have to switch on the hazard warning lights after reaching MRC (BfJ, 2003). This was realized for the basic test conditions “NW” and “TW” (see Table 3), which consisted only of conventional eHMI components. For the remaining conditions, hazard warning lights were presented continuously, during brake application and at the final stop. Gasser et al. (2012) also suggested switching on hazard warning lights already during MRMs for several HAV driving scenarios. Consequently, as a third purely conventional eHMI component test condition, “W”, a condition already used in the first study (“moderate braking”/“hazard warning lights”) was reinstalled (see Table 3).
As a novel component of eHMI design, the concept of a 360° LED light-band was applied to the HAV (see Table 3). During the uniform motion of the HAV, the 360° LED light-band lit continuously to indicate automated operation. Deceleration of the HAV was signaled by a slow pulsation of the light-band (0.5 Hz) that persisted during stop maneuvers until the HAV came to a complete stop. Cyan was used as a luminous color. In addition, traffic sign 101 (BfJ, 2013c), which indicates caution and a potential danger zone in German road traffic, was adapted as an eHMI component. The symbol was presented via a simulated LED display on the rear window of the HAV (see Table 3). This well-known and common symbol could activate existing schemata or mental models of surrounding road users so that the MRM scenario could be understood without much effort.
Accordingly, the test condition “W” was extended by the 360° LED light-band (“WL”), the warning symbol presented via display (“WS”), and their combination (“WLS”) (see Table 3). Brake light was visible during brake application in all conditions.
4.1.3. Dependent variables
The within-subject factor eHMI design was investigated by means of a set of dependent variables to draw conclusions about its potential to foster following drivers' trust and feelings of safety during HAVs' MRMs. Hence, eHMI design was measured with the same criteria: understanding/predictability, preparing for action, perceived quality of information, and criticality using the identical Likert scales as in the first study (cf. [3.1.3]). For perceived quality of information, however, item formulation was adjusted (“How well did you feel informed about the stopping maneuver by the shuttle's light signals?”). As stated before (cf. [2.3]), these criteria affect learned trust and are the basis to build trust. In addition, the items of the standardized short version of the User Experience Questionnaire (Schrepp et al., 2017) were applied, using semantic differentials ranging from −3 (“negative”) to +3 (“positive”). The mean of the first four items (“obstructive” vs. “supportive”, “complicated” vs. “easy”, “inefficient” vs. “efficient”, and “confusing” vs. “clear”) was used to measure pragmatic quality. The mean across the remaining four items (“boring” vs. “exciting”, “not interesting” vs. “interesting”, “conventional” vs. “inventive”, and “usual” vs. “leading edge”) measured hedonic quality.
Furthermore, the attempt was made to identify the eHMI design that is as simple as possible while still providing sufficient information during MRMs. Hence, from all levels of eHMI design, the one with the highest information sufficiency based on the item “What combination of light signals do you think is sufficient to assess the traffic situation clearly?”, i.e., to understand the shuttle's maneuver, was inquired. In addition, based on the six levels of eHMI design, the ranking by overall preference (“Which combination of light signals would you personally prefer?”) was collected from best (“I like the most”) to last (“I like the least”) to gain an understanding of overall user preferences for eHMI designs during MRMs.
4.1.4. Materials
To investigate trust fostering potentials of eHMIs during heading MRMs of HAVs from the following drivers' perspective this second experimental online study was conducted, which was again set up using rendered videos. Video material was created using Unreal Engine (version 4.26.2). Videos had a duration of approximately 15 s and showed the HAV from the perspective of a following driver's seat. The urban setting was identical to the first study (see Figure 3). Throughout, a moderate braking with −2.0 m/s2 was implemented as the constant dHMI. The various eHMI designs were added accordingly in each video. Additionally, driving parameters were controlled again. The vehicles' initial speeds were 30 km/h and the driving distance was about 80 m. Time headway between HAV and ego vehicle was 2 s from the start. The following driver's simulated reaction time was 1 s to the braking of the HAV. The ego vehicle also decelerated moderately with −2.0 m/s2. During the entire MRM, the HAV moved sideways toward the curb. The eHMI signals were turned on at the same time as the brake lights, which illuminated in the same way and point of time in all test conditions.
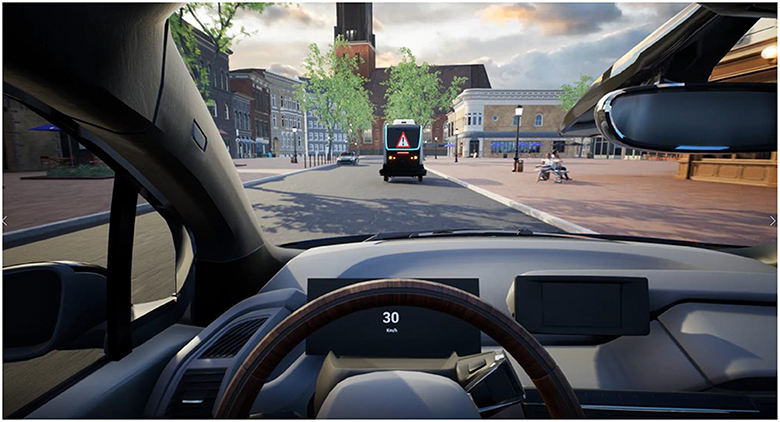
Figure 3. Excerpt from a video presented to the participants in this study showing the HAV conducting an MRM and communicating via eHMI strategy consisting of hazard warning lights, 360° LED light-band, plus warning symbol (“WLS”).
4.1.5. Procedure
This second study was set up again using SoSci Survey. Data collection was carried out from April to June 2022 and was conducted in German. Participants took part in the online survey independently. Devices allowed were PC, tablet, or smartphone. Up to the point of instructing the participants, the online questionnaire procedure was identical to the first study. Only the welcome page was changed due to the new research question. The requirements for automated vehicles to reach MRC with an MRM were explained in detail to the participants. However, for better understanding, an MRM was referred to as a safety stop, but still giving a full definition. Subsequently, the novel eHMI design concepts 360° LED light-band and warning symbol via display were presented and explained. The traffic situation was instructed identically as in the first study by showing the perspective of a driver following a fully automated shuttle to the participants (cf. [3.1.5]). However, in this study, participants were informed about an imminent stopping maneuver of the HAV in the form of a safety stop, which they were asked to take into consideration when evaluating the eHMI. Then, participants watched the video clips in randomized order and filled in the questionnaires each time. Afterwards, an overview of the six different eHMI designs was presented to the participants. They then had to select the eHMI design they thought would be sufficient for assessing the shown traffic situation. After that, each participant ranked the eHMI designs according to overall preference in a sorting task. At the end, demographic data was collected and participants were offered entry into a raffle.
4.2. Results
4.2.1. Data preparation and analysis
IBM SPSS Statistics was used for statistical analysis. The effect of the within-subjects factor eHMI design on understanding/predictability, preparing for action, perceived quality of information, criticality, pragmatic quality, and hedonic quality was examined using single factor, univariate, repeated-measures ANOVAs. Kolmogorov-Smirnov tests showed that the assumption of normal distribution could not be followed (about 89 %). Since the ANOVA is considered as a robust test procedure in this regard, the missing normal distribution could be neglected (Pagano, 2013). Mauchly tests revealed that sphericity was not met for any of the criteria. Therefore, depending on the value of epsilon, the Huynh-Feldt (ε > 0.75) or Greenhouse-Geisser method (ε < 0.75) was used to adjust degrees of freedom (Girden, 1992). Effect sizes for partial η2 were classified as small (0.01), medium (0.06), or large (0.14) according to Cohen (Cohen, 1988). In addition, the overall preference of eHMI design for MRM was investigated using Friedman's analysis of variance for ranks. For the rank distribution, Kendall's W was also determined and interpreted as weak (0.10), moderate (0.30), or strong (0.50) agreement of participants' rank assignments (Kraska-Miller, 2013). For the pairwise comparisons of each eHMI design condition, post-hoc tests were performed. For both repeated-measures ANOVAs and Friedman tests, Bonferroni correction was always applied to the standard 0.05 significance level.
4.2.2. Results regarding factor “eHMI design”
When the HAV displayed the examined eHMI designs for the MRM, the HAV's behavior tended to be evaluated rather positively. Repeated-measures ANOVAs still revealed that eHMI design each had a large effect on understanding/predictability, preparing for action, perceived quality of information, criticality, pragmatic quality, and hedonic quality (see Table 4).
The HAV's behavior was judged to be most understandable or predictable, respectively, for the eHMI designs “WLS” (M = 4.76, SD = 1.04) and “WS” (M = 4.63, SD = 1.10), both of which were rated significantly higher than all other designs (from p = 0.014 to p < 0.001). Furthermore, “TW” (M = 4.14, SD = 1.19), “WL” (M = 4.05, SD = 1.13), and “W” (M = 3.78, SD = 1.24) were rated significantly higher (all p < 0.001) than “NW” (M = 2.83, SD = 1.21). In addition, “TW” was assessed significantly higher than “W” (p = 0.028) (see Figure 4).
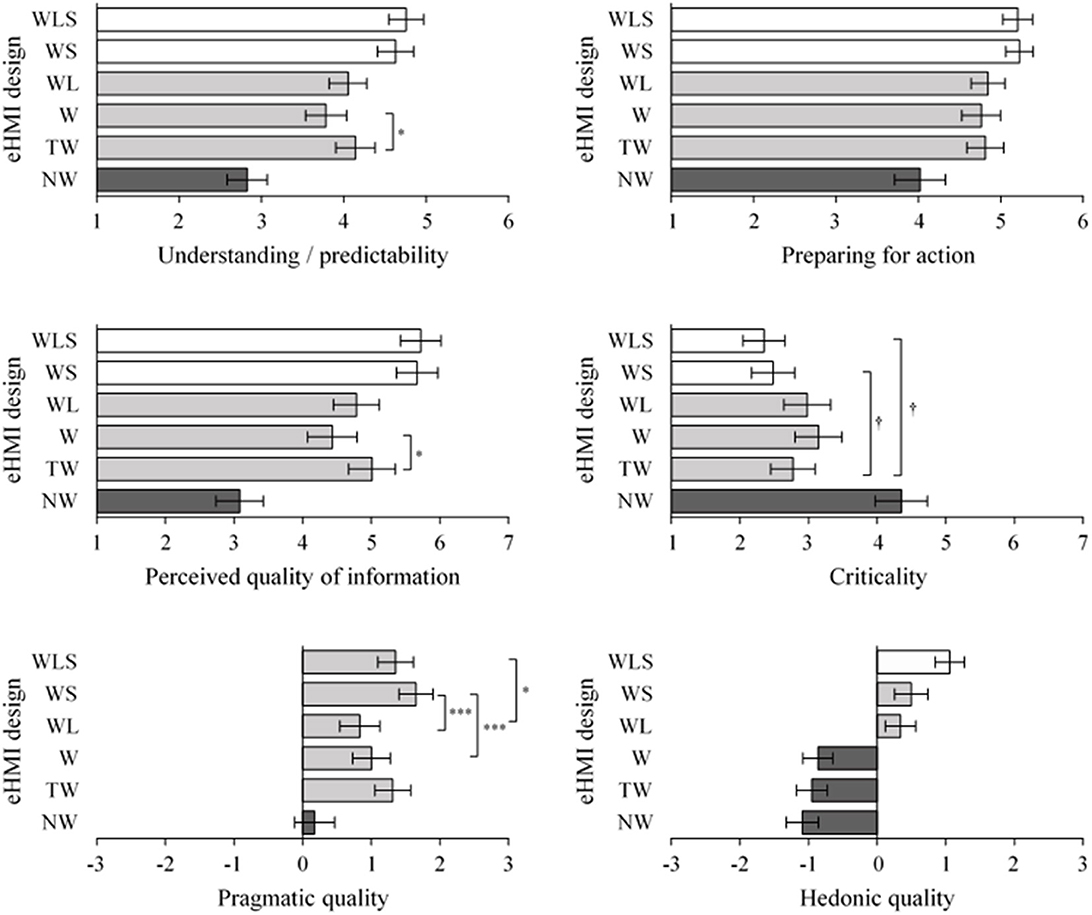
Figure 4. Investigated criteria relevant to building trust in automation of HAVs' following drivers experiencing MRMs in dependence of the factor eHMI design. Factor levels are “NW” (no light signal except brake lights plus hazard warning lights in MRC), “TW” (turn signal plus hazard warning lights in MRC), “W” (hazard warning lights continuously), “WL” (hazard warning lights plus 360° LED light-band), “WS” (hazard warning lights plus warning symbol via display), and “WLS” (hazard warning lights, 360° LED light-band, plus warning symbol via display). Significantly different ratings (α = 0.05) of the respective eHMI designs are represented by different gray scales of the bars per criterion, otherwise noted with brackets. Horizontal axes show available rating units per criterion. Error bars indicate 95% confidence intervals. p = ns. *p < 0.05. ***p < 0.001.
With regard to the criterion preparing for action a similar pattern became evident. “WS” (M = 5.23, SD = 0.82) and “WLS” (M = 5.21, SD = 0.90) were each rated significantly higher than the remaining eHMI designs (from p = 0.009 to p < 0.001). “NW” (M = 4.02, SD = 1.52) was each rated significantly lower (all p < 0.001) than the three eHMI designs “WL” (M = 4.85, SD = 1.02), “TW” (M = 4.81, SD = 1.10), and “W” (M = 4.76, SD = 1.17) (see Figure 4).
Similar gradations were identified for the eHMI designs' perceived quality of information. “WLS” (M = 5.72, SD = 1.47) and “WS” (M = 5.67, SD = 1.49) were evaluated significantly higher than the other four eHMI designs (from p = 0.043 to p < 0.001). “TW” (M = 5.01, SD = 1.69), “WL” (M = 4.78, SD = 1.65), and “W” (M = 4.43, SD = 1.79) were rated significantly more informative than “NW” (M = 3.08, SD = 1.71), all p < 0.001. Additionally, “TW”, which was rated significantly higher than “W” (p = 0.036) (see Figure 4).
For the stopping maneuver of the HAV, “WLS” (M = 2.35, SD = 1.52) and “WS” (M = 2.48, SD = 1.56) were also rated significantly less critical than the three eHMI designs “WL” (M = 2.98, SD = 1.68), “W” (M = 3.14, SD = 1.70), and “NW” (M = 4.35, SD = 1.89) (from p = 0.042 to p < 0.001). “TW” (M = 2.77, SD = 1.60) together with “WL” and “W” were rated as significantly less critical only compared to “NW” (all p < 0.001) (see Figure 4).
Concerning the pragmatic quality of the eHMI designs, only “NW” (M = 0.18, SD = 1.44) stood out, which was rated significantly lower than the other eHMI designs (from p = 0.011 to p < 0.001) (see Figure 4). When considering these five levels of eHMI design, “TW” (M = 1.31, SD = 1.29) did not differ from any of the other designs. “WS” (M = 1.65, SD = 1.22) was rated significantly higher than “W” (M = 1.00, SD = 1.36) and “WL” (M = 0.83, SD = 1.44) (both p < 0.001); “WLS” (M = 1.36, SD = 1.28) was only rated significantly higher than “WL” (p = 0.027).
In terms of hedonic quality, the eHMI designs that included only conventional eHMI components were rated significantly lower than the eHMI designs that had novel eHMI components. Consequently, “NW” (M = −1.09, SD = 1.16), “TW” (M = −0.95, SD = 1.12), and “W” (M = −0.86, SD = 1.09) were each rated significantly lower than the innovative eHMI designs (all p < 0.001), but did not vary among themselves (see Figure 4). “WLS” (M = 1.06, SD = 1.06) was rated significantly higher than “WS” (M = 0.50, SD = 1.19) and “WL” (M = 0.34, SD = 1.09) (both p < 0.001), neither of which differed significantly.
4.2.3. Information sufficiency and individual preferences of the eHMI designs
Regarding sufficiency of the information provided by the eHMI designs, participants (N = 97) were rather indecisive. “TW” (n = 31) was most frequently selected as sufficient in the presented traffic situation, closely followed by “WS” (n = 30), and “WLS” (n = 19). Less mentioned were “WL” (n = 8), “W” (n = 8), and “NW” (n = 1).
Kendall's Coefficient of Concordance, W = 0.177, also revealed only weak agreement among participants' ranking of the eHMI designs according to overall preference. However, for the distribution of ranks in terms of overall preference, the Friedman test revealed significant differences of eHMI design, χ2(5) = 85.64, p < 0.001. “WLS” (Mdn = 1) was the most frequently selected rank 1 (see Table 5), which, except for “WS,” was significantly more preferred than the remaining four eHMI designs (all p < 0.010). The pairwise comparisons further revealed that the distributions of “WS” (Mdn = 3), “WL” (Mdn = 3), and “W” (Mdn = 4) did not differ significantly but were preferred over the two eHMI designs “TW” and “NW” (from p = 0.041 to p < 0.001). “TW” (Mdn = 5) and “NW” (Mdn = 5) did not differ significantly.
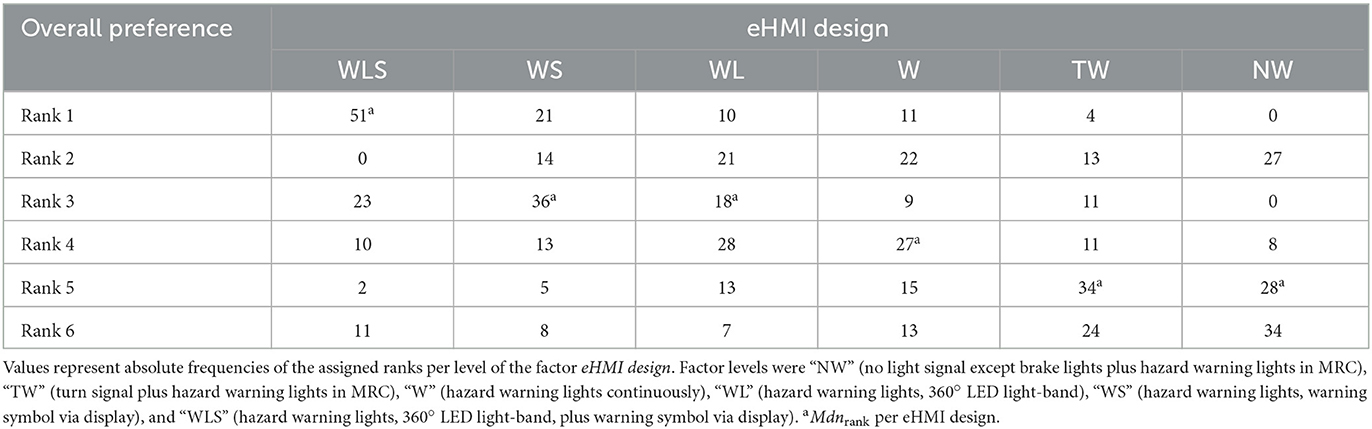
Table 5. Overall preference regarding the investigated eHMI designs for HAVs' MRMs from the perspective of following drivers.
5. Discussion
Identifying explicit and implicit communication strategies used by HAVs to build trust during MRMs was the focus of this research. In this work, very first insights are presented about how communication via dHMI and eHMI could ensure trust of following drivers toward HAVs during an MRM. Therefore, two user studies were conducted consecutively. First, appropriate vehicle dynamics of HAVs during MRMs. Second, suited HAV eHMI communication strategies during MRMs were identified. Both aspects of external communication of HAVs were investigated. The aim was to identify factors that increase the trust of following drivers. By focusing on vehicle dynamics in a first study, the research team was able to assure that eHMI strategies for MRMs investigated in the second user study are in line with a holistic perspective of external communication with HAVs to surrounding road users. Results showed that participants expected different braking deceleration for MRMs than for planned stops. In terms of transparency, this implies that HAVs trajectories should be adapted, depending on incident and cause to foster trust in the automated driving system. Moderate braking behavior seems suitable for MRM, also when taking passengers inside the HAV into account. Combining moderate braking with conventional light signals in the form of turn indicator seem to be an appropriate HAV strategy to announce unplanned curbside stopping. Overall, results for vehicle dynamics (in consideration of conventional light signals) from the first user study seem to be valuable for future MRM research.
In the second study, which builds upon the findings of the first, the main goal was to investigate explicit eHMI communication strategies during MRM conduction. Moreover, based on the first study, moderate braking dynamics were used for implicit communication, i.e., the vehicle's dynamics, during the MRM. Overall, the results of the study showed that adding novel eHMI light signals (i.e., warning symbol, 360° LED light-band) to conventional light signals affected all investigated trust-building metrics significantly in a positive way. In particular, the subjectively perceived quality of information was increased, leading to a greater readiness to take action. Accordingly, self-reported facets of trust in the automation and perceived criticality of the situation were influenced positively by the addition of novel eHMI concepts. Hence, more information in MRMs is beneficial for predicting the behavior of the HAV, which is in line with the literature (Baxter and Montgomery, 1996; Kramer, 1999). But also more sophisticated eHMI strategies could imply a more advanced technological state and better performance, which could be trusted more presumably (Raats et al., 2019). In general, results show a very coherent pattern and emphasize a potentially positive effect of eHMI design on learned trust toward HAVs. Moreover, solely showing the hazard warning lights (only in MRC) was evaluated significantly lowest across almost all variables. Furthermore, it became evident that the dHMI and brake light used in the present study were not sufficient as indications of an impending MRM and additional information were desired. In particular, when considering that trust of drivers is learned over time when following HAVs, this could be a factor for traffic safety, eventually. In this context, not only did the 360° LED light-band and the warning symbol improve pragmatic quality of the HAV during MRM, participants even evaluated the addition of novel eHMI communication with significantly higher hedonic quality. On the one hand, this could be based on the novelty effect, so that new eHMIs seem to be more exciting (Tulving and Kroll, 1995; Koch et al., 2018). Especially in combination with the new HAV technology, this result may be rooted in the expectations of the participants. On the other hand, participants received more explicit information from the HAV in a situation of uncertainty, i.e., in which more information was needed and sought by the participants. This aspect is also reflected in the highest rating for hedonic quality when all possible light signals were presented (i.e., 360° LED light band, warning symbol and hazard lights).
During an MRM, constantly flashing hazard warning lights don't provide additional directional information. This might explain why the presentation of turn indicator with hazard warning lights (only in MRC) was rated significantly higher in terms of understanding/predictability and perceived quality of information compared to hazard warning lights only. Also, in terms of information sufficiency, the combination of turn indicator and hazard warning lights (in MRC) was mentioned most frequently. Nevertheless, participants expressed the general preference for continuous hazard warning lights to a slightly greater extent than the combination of hazard warning lights and turn indicator. These results seem quite interesting. However, from an eHMI design perspective, the clearly defined criteria of understanding/predictability and perceived quality of information in that specific situation seem to be of more relevance for following drivers' UX and development of trust. Consequently, for the design of conventional eHMI design components for MRM, the use of turn indicator and hazard warning lights should be favorable. Presumably, the turn signal should be used during braking (or even earlier) and hazard warning lights should only be used immediately in MRC.
In addition, following drivers' trust in HAVs was improved by using a display showing a familiar “caution” symbol. The used traffic sign for caution was presented via display at the rear of the shuttle and builds on the basic design of the Vienna Convention on Road Signs (UNO, 1968). It is used in a variety of countries and is universally recognized. However, when it comes to differentiating the novel eHMI concepts (360° LED light-band rather subtle, symbol more specific) no clear statement could be made in terms of hedonic quality and user preferences. However, results indicate that the symbol seems beneficial in terms of perceived criticality, preparing for action, information quality, pragmatic quality and comprehensibility/predictability. Hence, using well known symbols in eHMIs should be beneficial to facilitate trust in HAVs. But also new and holistic concepts of explicit communication seem to already foster trust. However, following drivers would not benefit from purely perception-based communication strategies, which have already been investigated using 360° LED light-bands (Schieben et al., 2019). From this point of view, further HAV to road user communication research should focus on the slowly pulsating, intention-based 360° LED light-band. This study showed that the development of trust in HAVs can be affected positively by novel eHMI concepts in a very first contact scenario. It also indicates that specific eHMI communication strategies could be very supportive in developing trust in HAVs of other surrounding road users in different MRM interaction scenarios (Schindler et al., 2020a; Hesse et al., 2021; Lau et al., 2021a; Wilbrink et al., 2021; Kaleefathullah et al., 2022). In a nutshell, HAV communication to following drivers should be tailored for specific scenarios. Aligning implicit and explicit communication, and enhancing conventional light signals with novel eHMI strategies seems promising to foster trust in HAVs. Furthermore, novel eHMIs that evoke existing mental models of users seem beneficial to reduce uncertainty when interacting with HAVs.
5.1. Limitations
Both studies showed trust-building characteristics of HAVs for following drivers during MRMs. However, both studies were conducted as online studies with only short video sequences. Moreover, the traffic situation presented in the short videos was highly controlled, i.e., the stimulus material displayed the driver's perspective. Due to the highly controlled environment, the internal validity of the study could be assured. Nevertheless, the conditions could have decreased the participants' immersion. Thus, the transferability of this study's results regarding real traffic (i.e., external validity) might be limited. The short videos provided only a brief glimpse of a specific traffic situation (with relatively narrow lanes, an oncoming vehicle, a speed limit of 30 km/h, and a fixed distance to the HAV). Furthermore, since the braking behavior of the ego vehicle in the first study was varied according to the dHMI of the HAV, participants may have rated not only the dHMI of the HAV but also the driving dynamics of the ego vehicle differently as a confounding factor. However, a hard braking HAV will cause a following vehicle to brake harder as well. Therefore, it was probably only realistic to adjust the driving dynamics of the ego vehicle. Without this adaptation, either very large distances to the ego vehicle would have to be chosen, or unnatural critical situations would have occurred. In other study formats, e.g., driving simulator studies, this would be obsolete, as participants would drive the ego vehicle themselves. In the present studies, participants had to evaluate the communication strategies of the HAV in a passive role and could not react to the HAV maneuver. In forcing participants into a driving maneuver themselves, the evaluation of the different dHMI and eHMI designs could potentially differ. In the second online study, the participants were primed that an MRM would happen. Thus, it was not an unforeseen scenario. While unlikely, it is also possible that the wording “safety-stop” instead of MRM influenced participants' ratings. Another limitation could be that the eHMI designs were new to the participants (novelty effect). Also, the prototype character and the selection of prototypes have an influence on evaluation. Thus, other eHMI design concepts and other stages of prototype development could lead to different results. However, the results show that the addition of novel eHMI concepts was rated significantly more positively than the conventional light signals alone. Another limitation to the results is that they focus on participant's first contact with eHMI of HAVs (primary encounter), which is a crucial aspect when developing trust.
5.2. Future work
Ensuing from this research, future work should continue to focus on novel forms of eHMI design in various facets to investigate communication strategies with other road users. Therefore, various HAV maneuvers like MRM should be further investigated but also different perspectives of road users should be considered building trust in new automated mobility and to ensure traffic safety. With such an approach, understanding about broadly applicable eHMI design strategies could be gained. In order to validate the present results, a similar study should be conducted, for example, in a driving simulator. Accordingly, the participants should need to react according to the HAV behavior and communication. More complex traffic scenarios could be investigated and the MRM would appear more unforeseen. Potentially safety-critical traffic scenarios could also be presented to help introduce HAVs into future mixed traffic. Research of possible eHMI solutions could be conducted with a mixture of various maneuvers, including MRM. Eventually, more insights about appropriate eHMI communication could be gained for each scenario. Future research should also focus on the timing when eHMI signals are sent toward other road users, as this present study gave light signals simultaneously with a change of dynamic movement. This study focused on first contact interaction with HAVs and thus, future research should investigate how and which eHMI design strategies are favorable in terms of trust after long-term use. Furthermore, research about interaction with HAVs should be conducted in a more complex field study, also with real vehicle interaction, to increase external validity of such studies.
6. Conclusion
This work serves as the starting point for eHMI design for HAVs when conducting MRM. By focusing on the perspective of a following driver, the presented work tries to draw preliminary conclusions on how eHMI design could lead to high trust regarding HAVs and traffic safety in MRMs. The first study showed that moderate braking increases the awareness of following drivers and helps distinguish between planned and unplanned stops. In contrast to defensive braking, participants perceived moderate braking of HAVs as an indicator of MRMs, rather than a planned stop. Results indicate that implicit communication of HAVs should be conducted so that MRMs can be identified by surrounding traffic participants. Additional to the dHMI characteristics for MRM, explicit communication signals to the following drivers were investigated thoroughly in a second user study. Novel eHMI communication strategies (namely 360° LED light-band and virtual symbols) supported the development of trust by improving the information supply, increasing the user experience, and reducing the perceived criticality of the maneuver. Consequently, adding specific eHMI designs to conventional light signals can foster trust in HAVs. This aspect should be taken into consideration by legislative decision makers so that HAVs external communication channels can be exploited in favor of safe interaction with surrounding road users. Future eHMI design needs further research to harmonize explicit communication across other use cases and under consideration of various road users. The results presented contribute to the understanding of trust-building factors of HAVs from the point of view of the following drivers.
Data availability statement
The original contributions presented in the study are included in the article/supplementary material, further inquiries can be directed to the corresponding author.
Ethics statement
Ethical review and approval was not required for the study on human participants in accordance with the local legislation and institutional requirements. The patients/participants provided their written informed consent to participate in this study.
Author contributions
FH, SH, MW, and MO: conceptualization and methodology. SH and MW: data curation and visualization. SH: formal analysis and investigation. FH, SH, and ML: writing—original draft. FH, SH, ML, MW, and MO: writing—review and editing. MO: project administration, funding acquisition, and supervision. All authors contributed to the article and approved the submitted version.
Funding
This research was funded by the German Federal Ministry for Digital and Transport within the research project ViVre (Grant No.: 01MM19014A).
Conflict of interest
The authors declare that the research was conducted in the absence of any commercial or financial relationships that could be construed as a potential conflict of interest.
Publisher's note
All claims expressed in this article are solely those of the authors and do not necessarily represent those of their affiliated organizations, or those of the publisher, the editors and the reviewers. Any product that may be evaluated in this article, or claim that may be made by its manufacturer, is not guaranteed or endorsed by the publisher.
References
Avsar, H., Utesch, F., Wilbrink, M., Oehl, M., and Schießl, C. (2021). “Efficient communication of automated vehicles and manually driven vehicles through an external human-machine interface (eHMI): evaluation at T-junctions,” in Communications in Computer and Information Science. HCI International 2021 – Posters, eds. C. Stephanidis, M. Antona, and S. Ntoa (Cham: Springer International Publishing) 224–232. doi: 10.1007/978-3-030-78645-8_28
Baxter, L. A., and Montgomery, B. M. (1996). Relating: Dialogues and Dialectics. New York: Guilford Press.
Bazilinskyy, P., Dodou, D., and de Winter, J. (2019). Survey on eHMI concepts: The effect of text, color, and perspective. Transpor. Res. F. Traffic Psychol. Behav. 67, 175–194. doi: 10.1016/j.trf.2019.10.013
Bazilinskyy, P., Sakuma, T., and de Winter, J. (2021). What driving style makes pedestrians think a passing vehicle is driving automatically? Appl. Ergon. 95, 103428. doi: 10.1016/j.apergo.2021.103428
Bengler, K., Rettenmaier, M., Fritz, N., and Feierle, A. (2020). From HMI to HMIs: towards an HMI framework for automated driving. Information, 11, 61. doi: 10.3390/info11020061
BfJ (2003). Straßenverkehrsgesetz (StVG) § 1d Kraftfahrzeuge mit autonomer Fahrfunktion in festgelegten Betriebsbereichen. Available online at: https://www.gesetze-im-internet.de/stvg/__1d.html (accessed July 24, 2023).
BfJ (2013a). Straßenverkehrs-Ordnung (StVO) § 15 Liegenbleiben von Fahrzeugen. Available online at: https://www.gesetze-im-internet.de/stvo_2013/__15.html (accessed July 24, 2023).
BfJ (2013b). Straßenverkehrs-Ordnung (StVO) § 16 Warnzeichen. Available online at: https://www.gesetze-im-internet.de/stvo_2013/__16.html (accessed July 24, 2023).
BfJ (2013c). Straßenverkehrs-Ordnung (StVO) Anlage 1 (zu § 40 Absatz 6 und 7) Allgemeine und Besondere Gefahrzeichen. Available online at: https://www.gesetze-im-internet.de/stvo_2013/anlage_1.html (accessed July 24, 2023).
Boersma, R., van Arem, B., and Rieck, F. (2018). Application of driverless electric automated shuttles for public transport in villages: the case of appelscha. World Electr. Vehicle J. 9, 15. doi: 10.3390/wevj9010015
Brown, B., and Laurier, E. (2017). “The trouble with autopilots,” in Proceedings of the 2017 CHI Conference on Human Factors in Computing Systems eds. G. Mark, S. Fussell, C. Lampe, M. Schraefel, J. P. Hourcade, C. Appert, et al. (New York, NY, USA: ACM) 416–429. doi: 10.1145/3025453.3025462
Colquitt, J. A., Lepine, J. A., Piccolo, R. F., Zapata, C. P., and Rich, B. L. (2012). Explaining the justice-performance relationship: trust as exchange deepener or trust as uncertainty reducer? J. Appl. Psychol. 97, 1–15. doi: 10.1037/a0025208
Dey, D., Habibovic, A., Löcken, A., Wintersberger, P., Pfleging, B., Riener, A., et al. (2020). Taming the eHMI jungle: A classification taxonomy to guide, compare, and assess the design principles of automated vehicles' external human-machine interfaces. Transp. Res. Interdisc. Perspect. 7, 100174. doi: 10.1016/j.trip.2020.100174
Dietrich, A., Maruhn, P., Schwarze, L., and Bengler, K. (2020). “Implicit communication of automated vehicles in urban scenarios: effects of pitch and deceleration on pedestrian crossing behavior,” in Advances in Intelligent Systems and Computing. Human Systems Engineering and Design II eds. T. Ahram, W. Karwowski, S. Pickl, and R. Taiar (Cham: Springer International Publishing) 176–181. doi: 10.1007/978-3-030-27928-8_27
Endsley, M. R. (1995). Toward a theory of situation awareness in dynamic systems. Hum. Fact. 37, 32–64. doi: 10.1518/001872095779049543
Färber, B. (2016). “Communication and communication problems between autonomous vehicles and human drivers,” in Autonomous Driving eds. M. Maurer, J. C. Gerdes, B. Lenz, and H. Winner (Berlin, Heidelberg: Springer Berlin Heidelberg) 125–144. doi: 10.1007/978-3-662-48847-8_7
Festner, M., Eicher, A., and Schramm, D. (2017). “Beeinflussung der Komfort-und Sicherheitswahrnehmung beim hochautomatisierten Fahren durch fahrfremde Tätigkeiten und Spurwechseldynamik [Influence of non-driving tasks and lane change dynamics on feelings of comfort and safety in highly automated driving],” in 11. Workshop Fahrerassistenzsysteme und automatisertes Fahren 63–73. Available online at: https://www.uni-das.de/images/pdf/veroeffentlichungen/2017/07.pdf (accessed July 24, 2023).
Franke, T., Attig, C., and Wessel, D. (2019). A personal resource for technology interaction: development and validation of the affinity for technology interaction (ATI) scale. Int. J. Hum. Comput. Inter. 35, 456–467. doi: 10.1080/10447318.2018.1456150
Gasser, T. M., Arzt, C., Ayoubi, M., Bartels, A., Bürkle, L., Eier, J., et al. (2012). Rechtsfolgen zunehmender Fahrzeugautomatisierung [Legal consequences of increasing vehicle automation]. Berichte der Bundesanstalt für Straßenwesen. Unterreihe Fahrzeugtechnik, 83, 125. Available online at: https://bast.opus.hbz-nrw.de/opus45-bast/frontdoor/deliver/index/docId/541/file/F83.pdf (accessed July 24, 2023).
Gertz, C., Maaß, J. B., Grote, M., Diebold, T., Mantel, R., Röntgen, O., et al. (2021). Endbericht des Projektes TaBuLa. Final report of the TaBuLa project.
Girden, E. R. (1992). ANOVA: Repeated measures (1. print). Sage university papers: Vol. 84. Newbury Park, Calif.: Sage Publ. doi: 10.4135/9781412983419
Green, M. (2000). “How Long Does It Take to Stop?” Methodological analysis of driver perception-brake times. Transport. Hum. Fact. 2, 195–216. doi: 10.1207/STHF0203_1
Hesse, T., Oehl, M., Drewitz, U., and Jipp, M. (2021). Holistic, context-sensitive human-machine interaction for automated vehicles. ATZ worldwide, 123, 46–49. doi: 10.1007/s38311-020-0603-4
Hoff, K. A., and Bashir, M. (2015). Trust in automation: integrating empirical evidence on factors that influence trust. Hum. Fact. 57, 407–434. doi: 10.1177/0018720814547570
Josten, J., Kotte, J., and Eckstein, L. (2019). “Expectations of Non-automated Road Users for Interactions in Mixed Traffic,” in Advances in Intelligent Systems and Computing. Advances in Human Aspects of Transportation eds. N. Stanton (Cham: Springer International Publishing) 469–480. doi: 10.1007/978-3-319-93885-1_42
Kaleefathullah, A. A., Merat, N., Lee, Y. M., Eisma, Y. B., Madigan, R., Garcia, J., et al. (2022). External human-machine interfaces can be misleading: an examination of trust development and misuse in a CAVE-based pedestrian simulation environment. Hum. Fact. 64, 1070–1085. doi: 10.1177/0018720820970751
Karakaya, B., Kalb, L., and Bengler, K. (2020). A video survey on minimal risk maneuvers and conditions. Proc. Hum. Fact. Ergon. Soc. Ann. Meet. 64, 1708–1712. doi: 10.1177/1071181320641415
Koch, M., Luck, K., von Schwarzer, J., and Draheim, S. (2018). “The novelty effect in large display deployments-Experiences and lessons-learned for evaluating prototypes,” in Proceedings of 16th European conference on computer-supported cooperative work-exploratory papers.
Körber, M. (2019). “Theoretical Considerations and Development of a Questionnaire to Measure Trust in Automation,” in Advances in Intelligent Systems and Computing. Proceedings of the 20th Congress of the International Ergonomics Association (IEA 2018) eds. S. Bagnara, R. Tartaglia, S. Albolino, T. Alexander, and Y. Fujita (Cham: Springer International Publishing) 13–30.
Kramer, R. M. (1999). Trust and distrust in organizations: emerging perspectives, enduring questions. Ann. Rev. Psychol. 50, 569–598. doi: 10.1146/annurev.psych.50.1.569
Kraska-Miller, M. (2013). Nonparametric Statistics for Social and Behavioral Sciences. London: Chapman and Hall/CRC. doi: 10.1201/b16188
Lange, A., Maas, M., Albert, M., Siedersberger, K.-H., and Bengler, K. (2014). “Automatisiertes Fahren - So komfortabel wie möglich, so dynamisch wie nötig [Automated driving - As comfortable as possible, as dynamic as necessary],” in VDI-Berichte, 30. VDI-VW-Gemeinschaftstagung Fahrerassistenz und integrierte Sicherheit eds. GmbH, VDI Wissensforum (VDI Verlag).
Lau, M., Jipp, M., and Oehl, M. (2021b). “Investigating the Interplay between eHMI and dHMI for Automated Buses: How Do Contradictory Signals Influence a Pedestrian's Willingness to Cross?” in 13th International Conference on Automotive User Interfaces and Interactive Vehicular Applications (New York, NY, USA: ACM) 152–155. doi: 10.1145/3473682.3480284
Lau, M., Jipp, M., and Oehl, M. (2022). One solution fits all? Evaluating different communication strategies of a light-based external human-machine interface for differently sized automated vehicles from a pedestrian's perspective. Accid. Analy. Prevent. 171, 106641. doi: 10.1016/j.aap.2022.106641
Lau, M., Le, D. H., and Oehl, M. (2021a). “Design of External Human-Machine Interfaces for Different Automated Vehicle Types for the Interaction with Pedestrians on a Shared Space,” in Lecture Notes in Networks and Systems. Proceedings of the 21st Congress of the International Ergonomics Association (IEA 2021) eds. N. L. Black, W. P. Neumann, and I. Noy (Cham: Springer International Publishing) 710–717. doi: 10.1007/978-3-030-74608-7_87
Lee, J. D., and See, K. A. (2004). Trust in automation: designing for appropriate reliance. Hum. Fact. 46, 50–80. doi: 10.1518/hfes.46.1.50.30392
Leiner, D. J. (2019). Too fast, too straight, too weird: non-reactive indicators for meaningless data in internet surveys. Surv. Res. Methods. 13, 229–248.
Lewis, J. D., and Weigert, A. (1985). Trust as a social reality. Soc. Forc. 63, 967–985. doi: 10.2307/2578601
Marsh, S., and Dibben, M. R. (2003). The role of trust in information science and technology. Ann. Rev. Inf. Sci. Technol. 37, 465–498. doi: 10.1002/aris.1440370111
Merat, N., Louw, T., Madigan, R., Wilbrink, M., and Schieben, A. (2018). What externally presented information do VRUs require when interacting with fully Automated Road Transport Systems in shared space? Accid. Analy. Prevent. 118, 244–252. doi: 10.1016/j.aap.2018.03.018
Mirnig, A. G., Gärtner, M., Wallner, V., Gafert, M., Braun, H., Fröhlich, P., and Tscheligi, M. (2021). “Stop or go? Let me know!” in 13th International Conference on Automotive User Interfaces and Interactive Vehicular Applications (New York, NY, USA: ACM) 287–295. doi: 10.1145/3409118.3475131
Morgan, P. L., Williams, C., Flower, J., Alford, C., and Parkin, J. (2019). “Trust in an autonomously driven simulator and vehicle performing maneuvers at a T-junction with and without other vehicles,” in Advances in Intelligent Systems and Computing. Advances in Human Aspects of Transportation eds. N. Stanton (Cham: Springer International Publishing) 363–375. doi: 10.1007/978-3-319-93885-1_33
Muir, B. M. (1987). Trust between humans and machines, and the design of decision aids. Int. J. Man-Mach. Stud. 27, 527–539. doi: 10.1016/S0020-7373(87)80013-5
Nyberg, T., Pek, C., Dal Col, L., Noren, C., and Tumova, J. (2021). “Risk-aware motion planning for autonomous vehicles with safety specifications,” in 2021 IEEE Intelligent Vehicles Symposium (IV) (IEEE) 1016–1023. doi: 10.1109/IV48863.2021.9575928
Paddeu, D., Parkhurst, G., and Shergold, I. (2020). Passenger comfort and trust on first-time use of a shared autonomous shuttle vehicle. Transp. Res. C. 115, 102604. doi: 10.1016/j.trc.2020.02.026
Pagano, R. R. (2013). Understanding Statistics in the Behavioral Sciences (10. ed. International ed.). Australia: Wadsworth Cengage.
Powelleit, M., Winkler, S., and Vollrath, M. (2018). “Cooperation through communication-Using headlight technologies to improve traffic climate,” in Proceedings of the Human Factors and Ergonomics Society Europe 149–160.
Raats, K., Fors, V., and Pink, S. (2019). “Understanding Trust in Automated Vehicles,” in Proceedings of the 31st Australian Conference on Human-Computer-Interaction (New York, NY, USA: ACM) 352–358. doi: 10.1145/3369457.3369493
Reschka, A. (2016). “Safety concept for autonomous vehicles,” in Autonomous Driving eds. M. Maurer, J. C. Gerdes, B. Lenz, and H. Winner (Berlin, Heidelberg: Springer Berlin Heidelberg) 473–496. doi: 10.1007/978-3-662-48847-8_23
Risto, M., Emmenegger, C., Vinkhuyzen, E., Cefkin, M., and Hollan, J. (2017). “Human-vehicle interfaces: the power of vehicle movement gestures in human road user coordination,” in Proceedings of the 9th International Driving Symposium on Human Factors in Driver Assessment, Training, and Vehicle Design: driving assessment 2017 (Iowa City, Iowa: University of Iowa) 186–192. Available online at: https://drivingassessment.uiowa.edu/sites/drivingassessment.uiowa.edu/files/wysiwyg_uploads/29.pdf (accessed July 24, 2023).
Rouchitsas, A., and Alm, H. (2019). External human-machine interfaces for autonomous vehicle-to-pedestrian communication: a review of empirical work. Front. Psychol. 10, 2757. doi: 10.3389/fpsyg.2019.02757
Rouchitsas, A., and Alm, H. (2022). Ghost on the windshield: employing a virtual human character to communicate pedestrian acknowledgement and vehicle intention. Information, 13, 420. doi: 10.3390/info13090420
Rouchitsas, A., and Alm, H. (2023). Smiles and angry faces vs. nods and head shakes: facial expressions at the service of autonomous vehicles. Multim. Technol. Inter. 7, 10. doi: 10.3390/mti7020010
SAE. (2021a). J3016C: Taxonomy and Definitions for Terms Related to Driving Automation Systems for On-Road Motor Vehicle. Available online at: https://www.sae.org/standards/content/j3016_202104/ (accessed July 24, 2023).
SAE. (2021b). Levels of Driving Automation. Available online at: https://www.sae.org/site/blog/sae-j3016-update (accessed July 24, 2023).
Scherer, S., Schubert, D., Dettmann, A., Hartwich, F., and Bullinger, A. C. (2016). Wie will der “Fahrer” automatisiert gefahren werden? Überprüfung verschiedener Fahrstile hinsichtlich des Komforterlebens [How does the “driver” want to be driven in an automated way? Examination of different driving styles with regard to comfort experience]. Tagungsband 32. VDI/VW-Gemeinschaftstagung Fahrerassistenzsysteme automatisiertes Fahren, 8, 2016. doi: 10.51202/9783181022887-299
Schieben, A., Wilbrink, M., Dietrich, A., Ruenz, J., Portouli, E., Amditis, A., et al. (2020). “Designing cooperative interaction of automated vehicles in mixed traffic environments: Insights from the interACT project,” in Proceedings of 8th Transport Research Arena.
Schieben, A., Wilbrink, M., Kettwich, C., Madigan, R., Louw, T., and Merat, N. (2019). Designing the interaction of automated vehicles with other traffic participants: design considerations based on human needs and expectations. Cogn. Technol. Work. 21, 69–85. doi: 10.1007/s10111-018-0521-z
Schindler, J., Herbig, D. L., Lau, M., and Oehl, M. (2020a). “Communicating issues in automated driving to surrounding traffic - how should an automated vehicle communicate a minimum risk maneuver via eHMI and/or dHMI?” in Communications in Computer and Information Science. HCI International 2020 – Late Breaking Posters eds. C. Stephanidis, M. Antona, and S. Ntoa (Cham: Springer International Publishing) 619–626. doi: 10.1007/978-3-030-60703-6_79
Schindler, J., Markowski, R., Wesemeyer, D., Coll-Perales, B., Boker, C., and Khan, S. (2020b). “Infrastructure supported automated driving in transition areas – a prototypic implementation,” in 2020 IEEE 3rd Connected and Automated Vehicles Symposium (CAVS) (IEEE) 1–6. doi: 10.1109/CAVS51000.2020.9334555
Schrepp, M., Hinderks, A., and Thomaschewski, J. (2017). Design and evaluation of a short version of the user experience questionnaire (UEQ-S). Int. J. Inter. Multim. Artif. Intell. 4, 103. doi: 10.9781/ijimai.2017.09.001
Siebert, F. W., Oehl, M., and Pfister, H.-R. (2014). The influence of time headway on subjective driver states in adaptive cruise control. Transport. Res. F. 25, 65–73. doi: 10.1016/j.trf.2014.05.005
Sucha, M., Dostal, D., and Risser, R. (2017). Pedestrian-driver communication and decision strategies at marked crossings. Accid. Analy. Prevent. 102, 41–50. doi: 10.1016/j.aap.2017.02.018
Tulving, E., and Kroll, N. (1995). Novelty assessment in the brain and long-term memory encoding. Psychon. Bull. Rev. 2, 387–390. doi: 10.3758/BF03210977
UNO. (1968). Convention on road signs and signals (with annexes). Available online at: https://treaties.un.org/doc/Publication/UNTS/Volume%201091/volume-1091-I-16743-English.pdf (accessed July 24, 2023).
Weber, F., Sorokin, L., Schmidt, E., Schieben, A., Wilbrink, M., Kettwich, C., et al. (2019). interACT D. 4.2. Final Interaction Strategies for the interACT Automated Vehicles [Project deliverable]. Available online at: https://www.interact-roadautomation.eu/wp-content/uploads/interACT_WP4_D4.2_Final_Human_Vehicle_Interaction_Strategies_v1.1_uploadWebsiteApproved.pdf (accessed July 24, 2023).
Keywords: highly automated vehicles, minimal risk maneuver, external human-machine interface (eHMI), dynamic human-machine interface (dHMI), following driver
Citation: Hub F, Hess S, Lau M, Wilbrink M and Oehl M (2023) Promoting trust in HAVs of following manual drivers through implicit and explicit communication during minimal risk maneuvers. Front. Comput. Sci. 5:1154476. doi: 10.3389/fcomp.2023.1154476
Received: 30 January 2023; Accepted: 14 July 2023;
Published: 02 August 2023.
Edited by:
Yannick Forster, BMW, GermanyReviewed by:
Alexandros Rouchitsas, Luleå University of Technology, SwedenJeremy Dillmann, BMW, Germany
Copyright © 2023 Hub, Hess, Lau, Wilbrink and Oehl. This is an open-access article distributed under the terms of the Creative Commons Attribution License (CC BY). The use, distribution or reproduction in other forums is permitted, provided the original author(s) and the copyright owner(s) are credited and that the original publication in this journal is cited, in accordance with accepted academic practice. No use, distribution or reproduction is permitted which does not comply with these terms.
*Correspondence: Fabian Hub, ZmFiaWFuLmh1YkBkbHIuZGU=