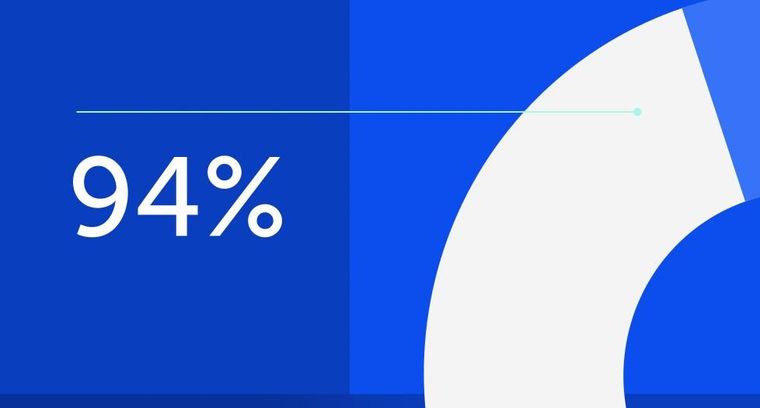
94% of researchers rate our articles as excellent or good
Learn more about the work of our research integrity team to safeguard the quality of each article we publish.
Find out more
REVIEW article
Front. Comput. Sci., 14 August 2023
Sec. Digital Education
Volume 5 - 2023 | https://doi.org/10.3389/fcomp.2023.1141649
The development of intelligent interactive learning approaches has received a lot of attention in recent years due to the trend of incorporating intelligent algorithms. Intelligent interactive education tools have a significant positive impact on user engagement, motivation, and social outcomes. This study attempts to explore the existing models, methods and technologies of intelligent interactive learning including virtual and augmented reality. The method of the current study was a semi-systematic literature review. This study is expected to make a substantial contribution to research on the use of intelligent interactive learning applications in e-learning. It supports earlier research and suggests a wide range of pertinent subject areas that might be investigated to progress the field. These findings lead to recommendations for future research on intelligent interactive learning techniques in e-learning.
The fundamental characteristic of interactive education is its emphasis on training students who require particular and distinctive instructional practices to aid in their understanding. The necessity of student group cooperation and support is emphasized in interactive education during instruction (Hui and Cong, 2022). Learning strategies are combined with intelligent components to create intelligent learning which helps to increase the effectiveness of teaching (Guo et al., 2021). Such technological advancements have already had a huge impact on the educational landscape, giving students new abilities, and fostering a collaborative learning environment in higher education that will have far-reaching effects soon (Kuleto et al., 2021). Intelligent education is currently undergoing rapid progress. Therefore, intelligent interactive learning (IIL) generally pertains to a learning approach where knowledge is acquired through interactive and adaptive methods, frequently employing artificial intelligence (AI) techniques. It encompasses systems capable of dynamically responding to user input, adjusting their behavior based on user feedback, and autonomously enhancing their performance as time progresses (Hu et al., 2022). Development of technology provides a strong support for the construction and application of intelligent learning environment and promotes the corresponding changes of learning and teaching methods (da Silva et al., 2021). One of them is through the Internet of things technology (IoT) which allows learning environments to become situational aware (Kassab et al., 2020). By improving connectivity, interaction, and personalization, IoT can have a significant positive impact on the field of e-learning (Qureshi et al., 2021). Moreover, IoT may cause a paradigm shift by making sustainable smart schools possible (Zeeshan et al., 2022). Another one is AI technology which identifies the learning environment, diagnoses pre-school learning situations, intelligently pushes learning resources, and provides personalized learning services (Chen et al., 2020). One opportunity for society to understand more about the state of AI is the public publication of OpenAI's GPT-3 model in December 2022 (FIRAT, 2023). Cloud computing (CC) technology can provide rich learning resources and enabling resources to be digitalized; rich media technology improves learners' interaction, participation, and learning experience (Ali, 2022). The original goal of CC was to lower computational costs while boosting system availability and reliability (Alam A., 2020; Alam T., 2020). These objectives have since changed to those of CC. Last but not least, big data and learning analysis technology can collect and mine big data related to teaching activities in the intelligent learning environment by using learning analysis technology (Huang, 2020; Huang X. et al., 2023).
Moreover, mobile terminals, such as smartphones and tablets, are becoming more popular and developed in education due to their mobility, adaptability, and accessibility (Hartley and Andújar, 2022). Consequently, the combination of the traditional Internet and mobile communication networks gives birth to mobile Internet (Yu, 2021). Due to mobile communication technology, mobile terminal equipment is able to communicate wirelessly anywhere and at any time (Gao et al., 2021). As a result of this technology, we are able to implement all kinds of teaching mode innovations in the intelligent learning environment using intelligent equipment.
A wide range of IIL methods have been examined in numerous studies on intelligent education (Hu et al., 2022). The purpose of this study is to undertake an information technology-based evaluation of the literature that is already accessible and to highlight the alleged benefits and limitations of current applications of IIL.
A literature review is an examination of academic sources on a certain topic (McCombes, 2019). In order to identify pertinent study hypotheses, methodologies, and gaps, it presents a summary of current knowledge. The literature review involves choosing, evaluating, and examining publications relevant to this research such as articles. The current study used a methodology of semi-systematic literature review to identify and compare the benefits and difficulties of IIL techniques. The semi-systematic or narrative review approach is designed for topics that have been conceptualized differently and studied by various groups of researchers within diverse disciplines and that hinder a full systematic review process (Wong et al., 2013). That is, to review every single article that could be relevant to the topic is simply not possible, so a different strategy must be developed. The review seeks to identify and understand all potentially relevant research traditions that have implications for the studied topic and to synthesize these using meta-narratives instead of by measuring effect size (Wong et al., 2013).
Offering earlier material through the literature review allowed for the collection of research findings related to the researcher's topic, the discovery of unfilled research gaps, and the designation of those gaps as the researcher's top priority for more research. For the data gathered, the current study used the qualitative methodology. The term “intelligent interactive learning” (IIL) often refers to the development of tailored and flexible learning experiences using cutting-edge technologies like artificial intelligence (AI), machine learning (ML), and natural language processing (NLP) (Ouyang et al., 2022). Therefore, “intelligent interactive learning” was chosen as the main study keyword. The review period covered by the current study is only from 2018 to 2023. To gain a worldwide perspective, the research was carried out using the following recommended electronic sources: Google Scholar and IEEE Explore, Web of science, Science Direct, Hindawi and Springer. The selection of linked articles was based on the requisites shown in Figure 1.
A total of 17,300 papers were initially found in the mentioned databases for the chosen date range with the main study keyword. However, more than 11,000 of them were peer-reviewed articles of journals, among which 351 were selected for further studies.
The results of the semi-systematic review can be analyzed and summarized using a variety of techniques. These techniques frequently resemble those applied in qualitative research in general. For instance, a frequently employed technique known as a thematic or content analysis can be broadly characterized as a way of locating, examining, and summarizing patterns in the form of themes inside a text (Braun and Clarke, 2006). Therefore, the authors have identified key areas presented in this paper according to five groups which are created by analyzing existing models, methods and technologies of teaching in the literature. Because this kind of analysis can be beneficial for observing trends, theoretical viewpoints, or shared problems within a particular research area or approach or for spotting elements of a theoretical notion (Ward et al., 2009). For this reason, the authors have provided some trends of approaches through about 100 research papers to remove duplicates of ways to improve the methods of IIL.
After all, due to the pace of development of information technologies, future research should choose a specific direction for the development of an IIL. Because the purpose of the authors' research is to improve IIL, the result of this article is to determine the direction that will solve the problem of the lack of educational resources and educational assessment services aimed at the modern student.
The authors have chosen approaches that are most compatible with their study goals and capable of producing reliable and valid results by weighing the benefits and drawbacks of the methods they have described. This review's objective was to determine how IIL affected e-learning. Details on the review outcomes are provided in the following subsections.
Cloud computing is one of the IIL techniques that offers a strong foundation for the development and application of intelligent learning environments. This model has developed as a cutting-edge technology in recent years, accelerating the pace of technological advancement in the computing industry. Application development and Internet access have been revolutionized by cloud computing. The services offered by this technology are delivered online. Due to cloud computing's convenience of economics and scalability, the upkeep of IT infrastructure and management becomes less necessary; it supports business without oppressing it (Alshwaier et al., 2012).
Siddiqui et al. (2019) evaluated the top five tools now available along with their benefits and drawbacks, noting that some platforms are overly complex. This paper suggests that platform “Easy generator” is a terrific alternative for students looking for an excellent education with the least amount of hassle. “Ruzuku” is a straightforward authoring tool that supports link sharing or embedding. The study (Wu and Plakhtii, 2021) demonstrates that the integration of cloud computing into the educational process results in improvements in the quality of the educational content and can significantly increase training efficiency due to updated learning technologies, concepts, and tools that produce new teaching content, models, and methods.
Wang and Zhao (2021) points out that the application of online teaching platform based on cloud computing and big data technology is a comfortable teaching method for educators as well as students with richer teaching resources. It also services as the students' personalized, diverse, and interactive learning need for learning. This kind of learning platform is based on the “cloud” resource network and mobile Internet and multimedia technologies, enabling learners to carry out quickly, self-contained, sharing, and IIL platforms through mobile terminals. By the testing and analysis of the platform, the feasibility of using the platform functions is obtained. According to the results, the number of students using mobile devices to access the cloud learning platform is considerably higher than the number of people using the computer web version of the platform. Due to the portability, real-time and interactive features of mobile devices, it is more suitable for students to learn, and it is more able to arouse students' interest in learning. However, experienced methods to analyse results could not show all of significance level of using this platform.
To improve the effect of English teaching (Hui and Cong, 2022), suggests combination of the intelligent algorithm to construct an intelligent interactive English teaching system for engineering education. This kind of interactive education is a way to achieve equal communication and independent interaction on a free educational platform under the macro educational situation, through teachers' teaching and students' learning around a certain issue or topic as interaction is one of the most basic characteristics of classroom teaching. Through simulation teaching combined with the expert evaluation method, it is verified that the intelligent interactive English teaching system for engineering education has certain effects.
Xin (2022) proposes the discrete data modeling and simulation of smart interactive English teaching based on mobile APP, expecting to explore the effective teaching strategies for deep integration of mobile APP technology and smart interactive English teaching. Using this kind of learning software, students can easily comprehend the knowledge as well as master the knowledge by practicing skillfully the learning software in a class, and exchange and inspect the works of other students to reach the level of application and insight understanding in comprehension learning. The author believes that at this point the learner has reached the highest level of understanding, i.e., self-awareness. In terms of discrete point parameterization, an improved discrete point parameterization method is obtained by using known normal vector information, and the accuracy is greatly improved compared with previous methods. In the node vector determination, the dominant point selection method and the algorithm stopping condition are modified with the help of normal vector information, which makes the method require fewer dominant points, and more accurate dominant point selection, and the required computation time is greatly reduced. This method combines the normal vector fitting error to improve the characteristic fitting approximation model. Compared with previous regularization methods, the results obtained in this study can better maintain the overall shape of the characteristic. How effectively mobile APP technology can assist teaching and learning depends mainly on the users. Because students with low willpower do not get the maximum learning benefit. Therefore, it is important for teachers to emphasize their leading position in teaching and supervise students to learn on their own.
Rafiq et al. (2021) investigates the potential for deaggregating query/question information in online lectures or course recommendations for e-learning systems. Reading, processing, and categorizing inquiry and question messages are all parts of information arrangement. A type of shallow content processing is data extraction. It searches the feature language archives for a preset collection of relevant content and uses artificial intelligence techniques to analyze common language. The creation of query optimization systems is of major significance to the assessment of difficulties in online lectures as numerous problems surfaced in the listeners' minds during the lectures. The findings demonstrate that the suggested approach enhances action verb classification to a higher level of accuracy.
AI is used to automate learning procedures such developing curriculum, training, assessing student performance, and using existing teaching methods. In higher education and business, AI is the newest e-learning trend. Kaplan and Haenlein (2019) explains AI in terms of its capacity to independently comprehend and learn from external data in order to achieve specified objectives through flexible adaptation, which is a more elaborate and maybe more detailed characterization. With the use of AI, data analytics can be used to make personalized judgments, which improves education for more individualized instruction and more efficient learning (Gao et al., 2021). According to Murtaza et al. (2022), tailored e-learning systems based on AI algorithms choose the right content for each student depending on their level of comprehension and preferred learning styles. As a result, the framework described in this paper is intended to serve as an illustration of an intelligent e-learning system that integrates complementary components to first learn to determine a student's comprehension levels before suggesting learning resources in accordance with the identified student level. Moreover, Huang A. Y. et al. (2023) focuses on how AI was incorporated into language teaching. The functions of AI in empirical research, the algorithms employed in empirical research, and the results and consequences generated by empirical research are all examined in Ouyang et al. (2022). In contrast to the more cognitively focused—human level AI where the complexities of human thinking and feeling have yet to be effectively translated, the use of big data has allowed algorithms to deliver excellent performance for specific tasks (robotic vehicles, game playing, autonomous scheduling, etc.) and a more pragmatic application of AI (Dwivedi et al., 2021). Findings from Ali et al. (2023) demonstrated that ChatGPT generally inspires students to improve their reading and writing abilities. Moreover, Huang A. Y. et al. (2023) outcomes showed that students with a moderate level of motivation might learn more effectively and interact in class more when given AI-enabled customized video recommendations. This study (Bag et al., 2022) empirically investigates and confirms the relationship between user engagement and conversion and the deployment of AI technologies. Because user engagement is essential as it encourages efficient learning, improves retention, and builds a favorable attitude toward education (Dwivedi et al., 2023). Alhazmi et al. (2023) offers insight to practitioners and researchers to improve AI's contribution to education.
One of the examples of an intelligent interactive learning system is ChatGPT. It is intended to have conversational interactions with users, comprehend their input, and produce pertinent responses (Dwivedi et al., 2023). For dynamic and intelligent interactions, ChatGPT uses machine learning and natural language processing methods. In order to provide some possible advantages of ChatGPT in boosting teaching and learning, this review (Baidoo-Anu and Owusu Ansah, 2023) synthesizes recent existing literature. It is important to note that despite numerous controversies and ethical questions (Ray, 2023), ChatGPT has in a very short period of time gained amazing attention from academics, research, and enterprises. The experts (Dwivedi et al., 2023) recognize ChatGPT's potential to increase productivity and contend that these gains are likely to be substantial for the banking, travel, and tourism, information technology, and commercial activities including management and marketing.
Intelligent learning systems that mimic human speech are called chatbots. They are capable of interacting with users by text or speech, responding to inquiries, disseminating knowledge, and even having more in-depth dialogues. The results of Kuhail et al. (2023) show that most chatbots followed a predetermined conversational path, and more than a quarter used a personalized learning approach that catered to students' learning needs. Other chatbots also used experiential learning and collaborative learning in addition to other design principles.
In this study (Bhaskaran et al., 2021), a hybrid intelligent recommender that adapts to the learner's learning preferences and personality was created. In comparison to the current collaborative filtering, the proposed sequential pattern clustering, pruning, and recommender algorithms delivered improved results. The suggested cluster-based recommender was found to boost performance experimentally, as evidenced by the fact that learners in the simulation cluster completed more lessons than those in the no-recommender cluster category. The simulation results show that, in comparison to several well-known approaches, this recommender minimized the mean absolute error measure for the various clusters.
The research (Bekmanova et al., 2021) has developed a personalized, intelligent learning model with standardized content for the management of blended and distance learning throughout life. This model includes a learner model, a model of the learning process, and an implementation of a learning interface. The potential for widespread usage of the proposed paradigm, its comparatively simple implementation, compliance with user expectations through personalisation, and the intellectual assessment of students' knowledge are the work's main contributions.
It is claimed that e-learning has become more of a focus as a source of knowledge for its users. Using IoT and smart learning, e-learning techniques can also be used to illustrate and demonstrate smart processes (Soni, 2019). A new smart e-learning paradigm was put forth by Zaguia et al. (2021) that emphasizes synchronous e-learning for students. This framework suggests a brand-new method of distance education where the teacher has more control over the students. IoT, AI, and VR tools are coupled to create a more powerful system that aids the teacher in keeping an eye on the students while delivering lessons and during tests. Numerous researchers (Farahani et al., 2018; Magrabi et al., 2018; Mershad and Wakim, 2018; Shyr et al., 2018; Tan et al., 2018) used the IoT in their other works as well as in the field of education.
Massive open online courses (MOOCs) have proliferated quickly and changed the landscape of higher education. More diversified empirical research has recently been done on MOOC design. Hew (2018), for instance, identified five course design elements that were most commonly noted in the comments of 4,466 participants on 10 highly rated MOOCs in order to engage MOOC participants. Namely, resources offered by the course, peer interaction, the availability and enthusiasm of the instructor, active learning, and issue learning. However, there is still little general understanding about the extent to which research-proven instructional design approaches have been incorporated into the creation of MOOCs.
By investigating the application of e-learning principles to MOOCs, Oh et al. (2020) improves the body of research (Colvin and Mayer, 2011) on the instructional quality of MOOCs. The study also provides insight into the areas that need improvement and suggests prospective directions for educational improvements in MOOCs.
Advanced self-directed learners should consider MOOCs as an appropriate environment (Rafiq et al., 2019). However, students with inadequately developed self-directed learning abilities may run into issues like not knowing what to do in MOOCs, not knowing where to start studying, and not receiving the proper assistance when they require it. While participating in activities in MOOCs, students are frequently not properly supervised and informed (Julia and Marco, 2021). Student engagement with the content is the main pedagogical tenet of MOOCs. But in the systems that are in place, this engagement is still relatively limited. To enhance the engagement between students and the curriculum, the content must offer thorough interactions and feedback to the students (Barthakur et al., 2021). These difficulties that MOOC participants encounter can lead to dropouts, a reduction in motivation, and unsatisfactory academic outcomes (Borrella et al., 2022). In order to solve the issues with MOOCs, Yilmaz et al. (2022) has suggested the idea of how to make systems such as MOOCs smarter. For this reason, a smart MOOC system is modeled that incorporates components of machine learning, intelligent tutoring, learning analytics, recommendation systems, adaptive mastery testing, dynamic evaluation, and adaptive learning. The goal of this framework is to assist students in their quest for knowledge and creative problem-solving. It is created to interact with students during their learning processes using educational data mining and learning analytics. However, as part of the example course, the pilot application studies of the developed system were conducted on undergraduate students. As a result, the study's findings are only applicable to this course and its target audience of students.
This study (Huiying and Qiang, 2021) improves and analyzes classic MOOC, cleans up traditional algorithms in accordance with the actual needs of MOOC teaching, and presents a new enhanced model in order to increase the effectiveness of college English cross-cultural teaching. Moreover, functional modules are created up in this document using requirements analysis. This research also designs control experiments in accordance with actual teaching in order to validate and examine the model performance from experimental teaching and the impact of luring students to online learning. The study's findings demonstrate the model's strong performance and its potential to significantly increase the effectiveness of cross-cultural English instruction.
Yang (2020) suggests a paradigm of an intelligent teaching system (ITS). The approach could be viewed as a platform or a system to implement various educational methods, including MOOCs, flipped classrooms, and others. ITS's hardware and software components will then be modeled utilizing the agent-based approach. With a focus on function specification and scene description, the modeling and simulation method works from whole to parts. The opportunity exists for the modeling of ITS to transition from conceptual design in the lab to application products on the market through evaluation.
This study (Hamal, 2022) offers an intelligent system capable of assisting the learner by providing answers to all his questions on the subjects covered in the MOOC. The architecture of our system is based on new advances in artificial intelligence, in particular the applications of deep learning in the field of natural language processing. Another study (Dhaiouir et al., 2022) suggests a method for classifying and categorizing learner profiles using an intelligent, autonomous system built on the foundation of neural networks, specifically the self-organizing map (SOM). We were able to create homogeneous groups using this methodology, which is based on the traceability of learners, and lead them toward MOOCs that suit their requirements and characteristics. But the primary problem with MOOCs that is impeding their development now is their high dropout rates. This research (Fu et al., 2021) recommends a pipeline model called CLSA to forecast the dropout rate based on learner behavior data in order to address this issue. This model was compared to four baseline models, and tests using varied time matrix specifications and ablation experiments based on the attention mechanism were also carried out. The experimental findings demonstrate that the model performs better when given more data.
In order to hasten the process of educational reform, a number of interactive online learning platforms integrating multimedia computer technology and network technology have evolved. However, these teaching platforms still cannot meet students' demands for experiments, practical exercises, and training, and they cannot realize real-time interaction between teachers and students, and teachers cannot perform the function of guidance and supervision, which will reduce students' participation in the teaching process. In light of this circumstance, virtual reality (VR), a new technology that is rapidly developing, has entered the sphere of education. VR technology works on the fundamental tenet of creating a corresponding three-dimensional virtual space with the aid of computer graphics rendering technology and other technologies to provide the user with sensory perceptions, primarily visual, auditory, and tactile, so the user can feel and interact with the data from the VR world as if someone were in a virtual environment. Additionally, the user can sense and engage with data from the VR world. The term “VR technology” actually refers to a variety of technologies, including data communication technology, voice recognition, stereo sound, network technology, and sensing technology (Jiang, 2021).
In essence, Rajo (2022) has introduced a full educational platform for experiencing the actual functions of this education, as well as a platform with visual and auditory all-around autonomy. It also introduced platform hardware selection, Unity-3D-based software development of virtual reality system, interaction between Bluetooth handle and virtual environment, and platform interaction between real and virtual environments. The platform is a low-cost construction and has a large student coverage, to solve the lack of teaching resources, trailing equipment conditions, outdated course content, professors and students unable to communicate in real-time, and a number of other issues.
Face recognition technology is used in the student monitoring system to determine different states of students in the classroom, such as raising hands, doing exercises, listening to class, and speaking. The teacher can directly grasp the differences in the different states of the students at each stage of the entire learning life using the assistance of the AI teaching aid system, which automatically generates the relevant learning report of each student based on changes in the classroom state and the students' facial emotions. To improve the effectiveness of intelligent education and teaching, (Hu et al., 2022) combines interactive visual computer vision analysis algorithms to build an intelligent education assistance system based on machine vision. The user, terminal interface, terminal processing, web server, and database are the five tiers that make up the entire system. The fundamental operating premise of the system described in this paper is to identify students in intelligent education using visual computer vision technology, assess the students' learning progress, and assist teachers in changing the lesson plan as needed to increase teaching effectiveness. The system's student classroom recognition module, which is based on the interactive visualization computer vision algorithm, is one of its proposed units. Positive, negative, and surprised facial micro-expressions can all be detected by this approach. The image data will be delivered to the facial recognition model every 20 facial image frames, and the results will be shown in the screen display area after the recognition model has finished processing the image data. The virtual avatar in the scene will reply appropriately to a command sent to Unity3D based on the evaluation of the real-time micro-expression. According to the findings of the experimental investigation, this intelligent education system has a specific practical impact.
Ke et al. (2020) findings showed that the VR-based learning environment encouraged participants to engage in interactive teaching and demonstrative education, and trained them to pay attention to and notice students' actions and emotions while teaching. There is evidence to support the idea that the participants are able to process and make use of the environment-situated learning support throughout their teaching activities, hence displaying a better comprehension or application of the embedded teaching knowledge. These results supported the educational benefits of the VR environment for training active teaching, which were in line with previous research reports such as Theelen et al. (2019). This study also offered preliminary evidence in favor of the deployment of non-player student agents in an open-source VR setting, which permits a potential teacher to practice and master instruction on their own schedule without coordinating with peers or other users. Moreover, it highlights a functional constraint of the current VR environment and the potential strategies or directions for the future design and implementation efforts. The study's conclusions propose that future research should integrate the analyses of learning experience, environmental affordance, and learner characteristics in planning and designing a VR-supported learning program.
The objective of Lamb et al. (2022) is to investigate if neurocognitive data obtained using functional near-infrared spectroscopy can be used to more quickly and accurately produce predictive models of student outcomes when utilizing a synthetic adaptive learning environment. This study specifically looks at the usefulness of developing student response prediction on a science content test using neurocognitive data. Three conditions were presented to study participants such as video, no content and VR. Deoxyribonucleic acid replication is described in the video lesson and VR lesson. Neurocognitive responses that were noticed throughout each condition were recorded and utilized to forecast how well students will respond to questions on an exam. The findings of this study provide evidence to support the use of neurocognitive data for adaptation of digitally presented content as well as how machine learning and AI may be utilized to classify student data in real-time as students interact with content. The development of artificially intelligent tutors and the advancement of student-based learning analytics may both benefit from these discoveries. It does have some restrictions, though. These prediction models may have issues when used to predict test results for students who exhibit greater neurocognitive diversity than is typical in a general education classroom. Furthermore, even though a challenging task was used, it's possible that these assessments don't fully account for the variety of cognitive actions that might be important for scientific education or other subject areas.
Jiang (2021) uses VR to create an interactive education platform. The main VR technologies are covered in this study, together with the pertinent theoretical underpinnings and a detailed description of the most widely used VR gadgets today, along with a thorough examination of their benefits and drawbacks. From the standpoint of instructional design, the design of a human-computer interaction (HCI) teaching process using virtual reality (VR) technology to create learning scenes is investigated. 3D modeling techniques, model construction, the creation of picture and text panels, the creation of video materials, and the establishment of 3D virtual tour guide scenes are all introduced. The purpose of this paper is to develop a 3D vision for 3D views of educational requirements for designing high-quality photos and video animation utilizing the 3DMAX and unity 3D engines. Additionally, it introduces the creation of a basic interactive teaching platform using Bluetooth wireless handles, entry-level VR spectacles, and smartphones running Android or iOS. Furthermore, the platform has a cheap cost of construction and student coverage, which helps to solve a number of issues like a lack of teaching resources, outdated equipment, aging curriculum content, and teachers and students who are unable to communicate in real time. However, a mobile phone is chosen to be installed in the glasses case as a display device, which may be used to calculate, using gyroscopes and other devices, the posture angle of the human head. Another phone should be included as well to complete the interaction with the telephone through the VR glasses. Not everyone finds it simple or comfortable to use the two phones in different directions. Furthermore, this paper (Gong, 2021) covers the use of VR as a teaching tool for the development of digital art, which helps to diversify the tools available for artistic creation and raise the standard of the process.
VR technology allows everyone to gain more technical knowledge by visualizing the theoretical information in abstract sports, whereas traditional sports courses at stadiums have some restrictions due to safety concerns and difficulties providing accurate and scientific teaching and learning. The research (Wang, 2021; Zhou, 2021) mainly explore physical education teaching assisted by AI-based VR technology. Last but not least, Zhu and Kou (2021) indicates three-dimensional simulation of swimming training based on Android mobile system and VR technology which its results provide evidence for the mechanism of thrust generation, and ambient speed and vorticity fields and swimming training show excellent effects in sports. It's crucial to note that VR is employed in many different sectors of education (Rojas-Sánchez et al., 2023), but that, up until the outbreak of the pandemic, student use of this so-called “disruptive process” predominated. Institutions were slow to adapt and integrate VR into teaching and learning processes. The use of virtual reality for learning is still somewhat challenging (Jessica et al., 2023) due to inadequate facilities caused by a lack of sufficient funding.
Digital information is incorporated into the user's actual environment by using technology of augmented reality (AR). It presents a fresh strategy for medical treatments and instruction. AR assists in the planning of operations, patient care, and the explanation of difficult medical problems to patients and their loved ones. According to the research (Eckert et al., 2019), disciplines that exploited display technology appeared to be explored more than other medical fields. The technical readiness level revealed that AR technology follows a rough bell curve from levels 4 to 7. More frequently than training situations, the current AR technology is used in therapy scenarios. There are yet no clinical trials demonstrating the efficiency of applied AR technologies.
The study (Jang et al., 2021) investigates teachers' openness to using AR and VR for teaching and learning practices. Therefore, it has used an extended Technology Acceptance Model (eTAM) to examine how teachers' intentions to use technology are influenced by their technical pedagogical and content knowledge, social norms, and motivational support. As a consequence, it implies the importance of providing technology professional development and support for teachers to promote the use of AR and VR in classrooms.
A overview of the literature on the use of VR and AR in oral and maxillofacial surgery was provided by the study (Ayoub and Pulijala, 2019). Maxillofacial surgery training and planning have benefited from VR and AR. A few papers (Farronato et al., 2019; Joda et al., 2019; Towers et al., 2019) have emphasized the significance of this technology in raising the standard of patient care. There aren't enough prospective randomized trials contrasting VR's effectiveness with that of more traditional techniques of teaching oral surgery. There are advantages to looking at possibilities for fresh and efficient delivery methods because there are increasing demands on the amount of knowledge that students must study within a medical and health sciences program. Learning in physiology and anatomy, where students need a three-dimensional understanding of human organ systems and architecture, has the potential to be improved by AR technology. Moro et al. (2021) explores the potential of AR as a tool for teaching and advocates for its adoption in postsecondary institutions. Additionally, it offers the HoloLens and AR for mobile devices as cutting-edge and fact-based means of teaching health sciences and medicine. Pathania et al. (2023) highlights the advantages of AR in comparison to more conventional technical platforms (such e-learning, MOOCs, and VR) and educational methods (chalk and talk and old-style books). The advantages of using AR technology in e-learning contexts include, but are not limited to, the improvement of kinesthetic and collaborative learning, the facilitation of high-risk e-learning in real-time, as well as visualizations, the support of real-world simulations with interactive objects, and the increase in learners' motivation, satisfaction, attention, and content retention (Fitria, 2023). The research literature on AR (Alzahrani, 2020) also revealed obstacles to its adoption and implementation, including its technological, pedagogical, and learning-related problems. Despite the difficulties, training and ongoing instruction were seen as practical answers to the main problems with AR adoption in e-learning contexts, even though the field still depends on technology developments in this area.
Gamification focuses on delivering effective instruction that emphasizes the value of engaging in social and global interactions while learning (York and Dehaan, 2018). Furthermore, gamification in education has enormous positive benefits on user engagement, motivation, and social dynamics. E-learning has used gamified aspects like points, badges, feedback, levels, awards, challenges, etc. According to the findings (Saleem et al., 2022), gamification is becoming more and more popular as a strategy for creating engaging learning environments. Students are encouraged and supported to participate in a gamification system via additional components (Mohamad et al., 2018).
The systematic review (Oliveira et al., 2021) of the literature which included a content analysis of 130 publications listed in the Web of Science database, has made identifications such as gamification-inspired behavioral attitudes, key game mechanics and their interrelationships. The main game elements and behavioral attitudes are combined in this study for the first time to encourage behavioral attitude change and ensure the efficacy of the educational process. Growing evidence that using gamification as a teaching tool can assist create engaging learning environments is pointing to the benefits of this approach (Zainuddin et al., 2020).
The results of gamified in education, which are based on observational data from recent research, seek to support the benefits of splitting in favor of its ability to inspire, engage, and have an impact on social dynamics while enabling students to fully engage in experiential learning (Lopez and Tucker, 2019; Zainuddin et al., 2020). It's critical to measure the impact of gamification on student learning in order to fully characterize the gamification of education. Even though gamification is popular, there is no consensus on how to raise academic performance. Language learners, teachers, and researchers are paying more and more attention to digital games that help students learn vocabulary. According to research conducted by Zou et al. (2021), ten different types of digital games, including simulation, tutorial, role-playing, motion-sensing, 3D virtual, adventure, card, board, and serious games, as well as gamified digital books, have positive effects on both short- and long-term vocabulary learning. From the perspective of creating digital games for vocabulary learning, it is stated that cognitive engagement, specific learning goals, learning techniques that take into account cognitive load, scaffolding, and intuitive game design are crucial elements for games to be successful. According to the Wu et al. (2020) findings, the main benefits of digital game based learning over conventional e-learning strategies are improved emotional health and enhanced attentiveness. Another paper Sukirman et al. (2022) has suggested a conceptual framework to develop a strategy for teaching computational thinking through game-based in VR contexts based on the findings and discussions from the 15 reviewed studies. In the conceptual framework, authors place more emphasis on the fact that computational thinking is intended to be the learning outcome.
Chan et al. (2022) presents the Urban Tourist Scenario Game platform, which was developed to improve the teaching-learning process of tourism geography and planning through a scenario-based game of an imaginary city undergoing urban tourism development. It is important to investigate the effects of gamification on children's self-regulated learning because it has been widely employed in elementary education to support children's motivation and engagement in their learning. Through the use of a gamified e-learning system, Li et al. (2022) explored the role of gamification on children's English learning. Regarding the use of gamified learning to improve students' self-regulated learning and second language learning, its findings have significance for e-learning designers and educators.
To deliver medical education online and through mobile apps, health researchers and providers have begun employing gamification apps (Garett and Young, 2019). The data in favor of game-based learning in undergraduate and graduate medical education, as well as in neurology education, is reviewed in Sandrone and Carlson (2021). Additionally, it has covered potential and problems for implementing neurologic curricula and has explored applications in clinical settings. Applications used in neurology education by Garcin et al. (2019) and Weaver and Roche (2019) were well received by the students and created a stress-free learning environment. Some experimental studies attempt to adapt psychological and educational theories available in the literature as gamification approaches, the resulting systems are not very clear, and there is no rationale for choosing certain game elements over others (Khaldi et al., 2023). Consequently, it can be concluded that these gamification approaches cannot strongly assist designers and practitioners in gamifying their learning systems.
Researchers can improve methodology, prevent traps, choose appropriate approaches, improve validity and reliability, advance knowledge, and enlighten conversations and interpretations by recognizing the benefits and limitations of various methods for a particular study issue. It is necessary for carrying out thorough and worthwhile study. That's why we have highlighted the claimed advantages (Table 1) and limitations (Table 2) of the present implementations of IIL in comparison to provide support for prior studies and makes a wide range of applicable suggestions for future research to advance the discipline. By evaluating the strengths and weaknesses of mentioned methods, the authors have made informed decisions about which method aligns best with their research objectives.
The development of technology and the spread of the internet and smart devices have positively reflected all areas. Especially in education, in this context, many modern technology tools, platforms, and advanced concepts have been harnessed in the curriculum to give education value across the web (distance, synchronize, blended, electronic, or online learning) is a unified concept for web-based learning. As studies have shown, e-learning platforms are advancing actively in almost all stages of the research.
This paper has reviewed the different models of intelligent interactive learning, which can be used to create an education tool for e-learning. The findings of this study are anticipated to have a significant impact on the field of study examining the usage of intelligent interactive learning applications in online learning. It provides support for prior studies and makes a wide range of applicable suggestions for future research to advance the discipline. Recommendations are made based on these findings for additional study into intelligent interactive learning methods in e-learning.
All of the aforementioned works strive to raise educational standards through enhancing e-learning techniques or technological advancements. Nevertheless, this study focused on providing a comprehensive overview of the current state of IIL while another study which was published very recently, presents a previous review to understand the implementation of one of the methods of IIL. The limitations of the research are chosen methods that are most appropriate for the authors' research objectives and capable of generating accurate results. Moreover, the criteria used to select the studies involved specifying the type of sources considered such as peer-reviewed articles of chosen databases which hosts a huge collection of peer-reviewed publications across various scientific areas, providing academics with access to a vast array of high-quality scholarly articles.
In general, AI techniques contribute to the success of the overall model rather than being the sole foundation. When AI has pertained to a particular domain, such as natural language processing, computer vision, or autonomous vehicles, it has held more validity (Dwivedi et al., 2021; Huang A. Y. et al., 2023). The techniques may serve as components within a larger framework or be integrated with traditional statistical models or rule-based systems (Murtaza et al., 2022). However, the authors have chosen an approach such as modeling the intelligent teaching system that can produce reliable and valid results by weighing the benefits and drawbacks of the methods they have described. According to the simulation (Yang, 2020), this approach is an effective technique to raise teaching quality and boost learning outcomes, and the system has the potential to be sold as an application product. Future research can focus on using one of the models to design and develop an intelligent learning system with the elements of VR technology. In view of the high pace of development of the digitalization of society, including the rapid development of the digitalization of education, there is a need for intelligent learning systems with interactive content. Such systems in the future will solve the problem of the lack of modern student-oriented educational resources, not tied to the time and place of classes, knowledge assessment services.
AU contributed to the writing and layout of the article, methodology, and research on the review of the methods of intelligent interactive learning. GB was engaged in the development of the research methodology and contributed to the conceptualization, observation, and visualization of the article. All authors have read and agreed to the published version of the manuscript.
The authors declare that the research was conducted in the absence of any commercial or financial relationships that could be construed as a potential conflict of interest.
All claims expressed in this article are solely those of the authors and do not necessarily represent those of their affiliated organizations, or those of the publisher, the editors and the reviewers. Any product that may be evaluated in this article, or claim that may be made by its manufacturer, is not guaranteed or endorsed by the publisher.
Alam, A. (2020). Challenges and possibilities in teaching and learning of calculus: A case study of India. J. Educ. Gifted Young Scient. 8, 407–433. doi: 10.17478/jegys.660201
Alam, T. (2020). Cloud Computing and its role in the Information Technology. IAIC Trans. Sustain. Digital Innov. 1, 108–115. doi: 10.34306/itsdi.v1i2.103
Alhazmi, A. K., Alhammadi, F., Zain, A. A., Kaed, E., and Ahmed, B. (2023). “AI's role and application in education: Systematic review,” in Intelligent Sustainable Systems. Lecture Notes in Networks and Systems, Vol. 578, eds A. K. Nagar, D. Singh Jat, D. K. Mishra, and A. Joshi (Singapore: Springer). doi: 10.1007/978-981-19-7660-5_1
Ali, A. (2022). An overview of cloud computing for the advancement of the e-learning process. J. Theor. Appl. Inf. Technol. 100, 847–855.
Ali, J. K. M., Shamsan, M. A. A., Hezam, T. A., and Mohammed, A. A. (2023). Impact of ChatGPT on learning motivation: teachers and students' voices. J. English Stud. Arabia Felix 2, 41–49. doi: 10.56540/jesaf.v2i1.51
Alshwaier, A., Youssef, A., and Emam, A. (2012). A new trend for e-learning in KSA using educational clouds. Adv. Comput. 3, 81. doi: 10.5121/acij.2012.3107
Alzahrani, N. M. (2020). Augmented reality: A systematic review of its benefits and challenges in e-learning contexts. Appl. Sci. 10, 5660. doi: 10.3390/app10165660
Ayoub, A., and Pulijala, Y. (2019). The application of virtual reality and augmented reality in Oral and Maxillofacial Surgery. BMC Oral Health 19, 1–8. doi: 10.1186/s12903-019-0937-8
Bag, S., Srivastava, G., Bashir, M. M. A., Kumari, S., Giannakis, M., and Chowdhury, A. H. (2022). Journey of customers in this digital era: Understanding the role of artificial intelligence technologies in user engagement and conversion. Benchmark. Int. J. 29, 2074–2098. doi: 10.1108/BIJ-07-2021-0415
Baidoo-Anu, D., and Owusu Ansah, L. (2023). Education in the era of generative artificial intelligence (AI): Understanding the potential benefits of ChatGPT in promoting teaching and learning. Available at SSRN 4337484. doi: 10.2139/ssrn.4337484
Barthakur, A., Kovanovic, V., Joksimovic, S., Siemens, G., Richey, M., and Dawson, S. (2021). Assessing program-level learning strategies in MOOCs. Comput. Hum. Behav. 117, 106674. doi: 10.1016/j.chb.2020.106674
Bekmanova, G., Ongarbayev, Y., Somzhurek, B., and Mukatayev, N. (2021). Personalized training model for organizing blended and lifelong distance learning courses and its effectiveness in Higher Education. J. Comput. High. Educ. 33, 668–683. doi: 10.1007/s12528-021-09282-2
Bhaskaran, S., Marappan, R., and Santhi, B. (2021). Design and analysis of a cluster-based intelligent hybrid recommendation system for e-learning applications. Mathematics 9, 197. doi: 10.3390/math9020197
Borrella, I., Caballero-Caballero, S., and Ponce-Cueto, E. (2022). Taking action to reduce dropout in MOOCs: Tested interventions. Comput. Educ. 179, 104412. doi: 10.1016/j.compedu.2021.104412
Braun, V., and Clarke, V. (2006). Using thematic analysis in psychology. Qualit. Res. Psychol. 3, 77–101. doi: 10.1191/1478088706qp063oa
Chan, C. S., Yat-Hang, C., and Tsz Heung Agnes, F. (2022). Promoting game-based e-Learning through urban tourism scenario game from the evaluation of knowledge-attitude-usability effectiveness. J. Global Schol. Market. Sci. 32, 16–35. doi: 10.1080/21639159.2020.1808831
Chen, L., Chen, P., and Lin, Z. (2020). Artificial intelligence in education: A review. IEEE Access 8, 75264–75278. doi: 10.1109/ACCESS.2020.2988510
Colvin, R., and Mayer, R. E. (2011). E-Learning and the Science of Instruction: Proven Guidelines for Consumers and Designers of Multimedia Learning. Misenheimer, NC: Pfeiffer.
da Silva, L. M., Dias, L. P. S., Rigo, S., Barbosa, J. L. V., Leithardt, D. R., and Leithardt, V. R. Q. (2021). A literature review on intelligent services applied to distance learning. Educ. Sci. 11, 666. doi: 10.3390/educsci11110666
Dhaiouir, I., Ezziyyani, M., and Khaldi, M. (2022). Smart model for classification and orientation of learners in a MOOC. Int. J. Emerg. Technol. Learn. 17, 28153. doi: 10.3991/ijet.v17i05.28153
Dwivedi, Y. K., Hughes, L., Ismagilova, E., Aarts, G., Coombs, C., Crick, T., et al. (2021). Artificial Intelligence (AI): Multidisciplinary perspectives on emerging challenges, opportunities, and agenda for research, practice and policy. Int. J. Inf. Manag. 57, 101994. doi: 10.1016/j.ijinfomgt.2019.08.002
Dwivedi, Y. K., Kshetri, N., Hughes, L., Slade, E. L., Jeyaraj, A., Kar, A. K., et al. (2023). “So what if ChatGPT wrote it?” Multidisciplinary perspectives on opportunities, challenges and implications of generative conversational AI for research, practice and policy. Int. J. Inf. Manag. 71, 102642. doi: 10.1016/j.ijinfomgt.2023.102642
Eckert, M., Volmerg, J. S., and Friedrich, C. M. (2019). Augmented reality in medicine: systematic and bibliographic review. JMIR mHealth uHealth 7, e10967. doi: 10.2196/10967
Farahani, B., Firouzi, F., Chang, V., Badaroglu, M., Constant, N., and Mankodiya, K. (2018). Towards fog-driven IoT eHealth: Promises and challenges of IoT in medicine and healthcare. Fut. Gener. Comput. Syst. 78, 659–676. doi: 10.1016/j.future.2017.04.036
Farronato, M., Maspero, C., Lanteri, V., Fama, A., Ferrati, F., Pettenuzzo, A., et al. (2019). Current state of the art in the use of augmented reality in dentistry: A systematic review of the literature. BMC Oral Health 19, 1–15. doi: 10.1186/s12903-019-0808-3
FIRAT, M. (2023). Integrating AI applications into learning management systems to enhance e-learning. Instr. Technol. Lifelong Lear. 4, 1–14. doi: 10.52911/itall.1244453
Fitria, T. N. (2023). Augmented Reality (AR) and virtual reality (VR) technology in education: media of teaching and learning: a review. Int. J. Comput. Inf. Syst. (IJCIS) 4, 14–25.
Fu, Q., Gao, Z., Zhou, J., and Zheng, Y. (2021). CLSA: A novel deep learning model for MOOC dropout prediction. Comput. Electr. Eng. 94, 107315. doi: 10.1016/j.compeleceng.2021.107315
Gao, P., Li, J., and Liu, S. (2021). An introduction to key technology in artificial intelligence and big data driven e-learning and e-education. Mobile Netw. Applic. 26, 2123–2126. doi: 10.1007/s11036-021-01777-7
Garcin, B., Mariani, L. L., Méneret, A., Mongin, M., Delorme, C., Cormier, F., et al. (2019). The “neurological hat game”: a fun way to learn the neurological semiology. Rev. Neurol. 175, 528–533. doi: 10.1016/j.neurol.2019.01.395
Garett, R., and Young, S. D. (2019). Health care gamification: a study of game mechanics and elements. Technol. Knowl. Learn. 24, 341–353. doi: 10.1007/s10758-018-9353-4
Gong, Y. (2021). Application of virtual reality teaching method and artificial intelligence technology in digital media art creation. Ecol. Inform. 63, 101304. doi: 10.1016/j.ecoinf.2021.101304
Guo, L., Wang, D., Gu, F., Li, Y., Wang, Y., and Zhou, R. (2021). Evolution and trends in intelligent tutoring systems research: a multidisciplinary and scientometric view. Asia Pacific Educ. Rev. 22, 441–461. doi: 10.1007/s12564-021-09697-7
Hamal, O. (2022). Intelligent system using deep learning for answering learner questions in a MOOC. Int. J. Emer. Technol. Lear. 17, 32–42. doi: 10.3991/ijet.v17i02.26605
Hartley, K., and Andújar, A. (2022). Smartphones and learning: an extension of m-learning or a distinct area of inquiry. Educ. Sci. 12, 50. doi: 10.3390/educsci12010050
Hew, K. F. (2018). Unpacking the strategies of ten highly rated MOOCs: Implications for engaging students in large online courses. Teach. College Record. 120, 1–40. doi: 10.1177/016146811812000107
Hu, Y., Li, Q., and Hsu, S. W. (2022). Interactive visual computer vision analysis based on artificial intelligence technology in intelligent education. Neural Comput. Applic. 34, 9315–9333. doi: 10.1007/s00521-021-06285-z
Huang, A. Y., Lu, O. H., and Yang, S. J. (2023). Effects of artificial Intelligence–Enabled personalized recommendations on learners' learning engagement, motivation, and outcomes in a flipped classroom. Comput. Educ. 194, 104684. doi: 10.1016/j.compedu.2022.104684
Huang, X. (2020). “Construction and application of online course teaching in intelligent learning environment,” in Cyber Security Intelligence and Analytics. CSIA 2020. Advances in Intelligent Systems and Computing eds. Z., Xu, R., Parizi, M., Hammoudeh, O., Loyola-González (Cham: Springer).
Huang, X., Zou, D., Cheng, G., Chen, X., and Xie, H. (2023). Trends, research issues and applications of artificial intelligence in language education. Educ. Technol. Soc. 26, 112–131.
Hui, S., and Cong, S. (2022). Intelligent interactive english teaching system for engineering education. Adv. Multim. 2022, 4676776. doi: 10.1155/2022/4676776
Huiying, X., and Qiang, M. (2021). College English cross-cultural teaching based on cloud computing MOOC platform and artificial intelligence. J. Intell. Fuzzy Syst. 40, 7335–7345. doi: 10.3233/JIFS-189558
Jang, J., Ko, Y., Shin, W. S., and Han, I. (2021). Augmented reality and virtual reality for learning: An examination using an extended technology acceptance model. IEEE Access 9, 6798–6809. doi: 10.1109/ACCESS.2020.3048708
Jessica, P., Salim, S., Syahputra, M. E., and Suri, P. A. (2023). A Systematic literature review on implementation of virtual reality for learning. Proc. Comput. Sci. 216, 260–265. doi: 10.1016/j.procs.2022.12.135
Jiang, L. (2021). Virtual reality action interactive teaching artificial intelligence education system. Complexity 2021, 5553211. doi: 10.1155/2021/5553211
Joda, T., Gallucci, G. O., Wismeijer, D., and Zitzmann, N. U. (2019). Augmented and virtual reality in dental medicine: A systematic review. Comput. Biol. Med. 108, 93–100. doi: 10.1016/j.compbiomed.2019.03.012
Julia, K., and Marco, K. (2021). Educational scalability in MOOCs: Analysing instructional designs to find best practices. Comput. Educ. 161, 104054. doi: 10.1016/j.compedu.2020.104054
Kaplan, A., and Haenlein, M. (2019). Siri, Siri, in my hand: Who's the fairest in the land? On the interpretations, illustrations, and implications of artificial intelligence. Business Horizon. 62, 15–25. doi: 10.1016/j.bushor.2018.08.004
Kassab, M., DeFranco, J., and Laplante, P. (2020). A systematic literature review on Internet of things in education: Benefits and challenges. J. Comput. Assist. Lear. 36, 115–127. doi: 10.1111/jcal.12383
Ke, F., Pachman, M., and Dai, Z. (2020). Investigating educational affordances of virtual reality for simulation-based teaching training with graduate teaching assistants. J. Comput. Higher Educ. 32, 607–627. doi: 10.1007/s12528-020-09249-9
Khaldi, A., Bouzidi, R., and Nader, F. (2023). Gamification of e-learning in higher education: a systematic literature review. Smart Lear. Environ. 10, 10. doi: 10.1186/s40561-023-00227-z
Kuhail, M. A., Alturki, N., Alramlawi, S., and Alhejori, K. (2023). Interacting with educational chatbots: A systematic review. Educ. Inf. Technol. 28, 973–1018. doi: 10.1007/s10639-022-11177-3
Kuleto, V., Ilić, M., Dumangiu, M., Ranković, M., Martins, O. M., Păun, D., et al. (2021). Exploring opportunities and challenges of artificial intelligence and machine learning in higher education institutions. Sustainability 13, 10424. doi: 10.3390/su131810424
Lamb, R., Neumann, K., and Linder, K. A. (2022). Real-time prediction of science student learning outcomes using machine learning classification of hemodynamics during virtual reality and online learning sessions. Comput. Educ. 3, 100078. doi: 10.1016/j.caeai.2022.100078
Li, X., Xia, Q., Chu, S. K. W., and Yang, Y. (2022). Using gamification to facilitate students' self-regulation in e-learning: a case study on students' L2 english learning. Sustainability 14, 7008. doi: 10.3390/su14127008
Lopez, C. E., and Tucker, C. S. (2019). The effects of player type on performance: A gamification case study. Comput. Hum. Behav. 91, 333–345. doi: 10.1016/j.chb.2018.10.005
Magrabi, S. A. R., Pasha, M. I., and Pasha, M. Y. (2018). Classroom teaching to enhance critical thinking and problem-solving skills for developing IOT applications. J. Eng. Educ. Transform. 31, 152–157. doi: 10.16920/jeet/2018/v31i3/120785
McCombes, S. (2019). How to write a literature review. Available online at: https://www.scribber.com/dissertation/litrature-review (accessed September 24, 2020).
Mershad, K., and Wakim, P. (2018). A learning management system enhanced with internet of things applications. J. Educ. Lear. 7, 23–40. doi: 10.5539/jel.v7n3p23
Mohamad, S. N. M., Sazali, N. S. S., and Salleh, M. A. M. (2018). Gamification approach in education to increase learning engagement. Int. J. Human. Arts Soc. Sci. 4, 22–32. doi: 10.20469/ijhss.4.10003-1
Moro, C., Phelps, C., Redmond, P., and Stromberga, Z. (2021). HoloLens and mobile augmented reality in medical and health science education: A randomised controlled trial. Br. J. Educ. Technol. 52, 680–694. doi: 10.1111/bjet.13049
Murtaza, M., Ahmed, Y., Shamsi, J. A., Sherwani, F., and Usman, M. (2022). AI-based personalized e-learning systems: Issues, challenges, and solutions. IEEE Access. 10, 81323–81342. doi: 10.1109/ACCESS.2022.3193938
Oh, E. G., Chang, Y., and Park, S. W. (2020). Design review of MOOCs: Application of e-learning design principles. J. Comput. High. Educ. 32, 455–475. doi: 10.1007/s12528-019-09243-w
Oliveira, R. P., Souza, C. G. D., Reis, A. D. C., and Souza, W. M. D. (2021). Gamification in e-learning and sustainability: a theoretical framework. Sustainability 13, 11945. doi: 10.3390/su132111945
Ouyang, F., Zheng, L., and Jiao, P. (2022). Artificial intelligence in online higher education: A systematic review of empirical research from 2011 to 2020. Educ. Inf. Technol. 27, 7893–7925. doi: 10.1007/s10639-022-10925-9
Pathania, M., Mantri, A., Kaur, D. P., Singh, C. P., and Sharma, B. (2023). A chronological literature review of different augmented reality approaches in education. Technol. Knowl. Lear. 28, 329–346. doi: 10.1007/s10758-021-09558-7
Qureshi, K. N., Naveed, A., Kashif, Y., and Jeon, G. (2021). Internet of Things for education: A smart and secure system for schools monitoring and alerting. Comput. Electr. Eng. 93, 107275. doi: 10.1016/j.compeleceng.2021.107275
Rafiq, K. R. M., Hashim, H., and Yunus, M. M. (2019). MOOC for training: a review of the variations of MOOC. Int. J. Innov. Creat. Change. 5, 90–98.
Rafiq, M. S., Jianshe, X., Arif, M., and Barra, P. (2021). Intelligent query optimization and course recommendation during online lectures in E-learning system. J. Ambient Intell. Human. Comput. 12, 10375–10394. doi: 10.1007/s12652-020-02834-x
Rajo, T. (2022). Teaching artificial intelligence using virtual reality action interactive system of education for advanced educational outcomes in Hunan province of China. Hindawi Complexity 2022.
Ray, P. P. (2023). ChatGPT: A comprehensive review on background, applications, key challenges, bias, ethics, limitations and future scope. Internet Things Cyber-Phys. Syst. 3, 121–154. doi: 10.1016/j.iotcps.2023.04.003
Rojas-Sánchez, M. A., Palos-Sánchez, P. R., and Folgado-Fernández, J. A. (2023). Systematic literature review and bibliometric analysis on virtual reality and education. Educ. Inf. Technol. 28, 155–192, doi: 10.1007/s10639-022-11167-5
Saleem, A. N., Noori, N. M., and Ozdamli, F. (2022). Gamification applications in E-learning: A literature review. Technol. Knowl. Lear. 27, 139–159. doi: 10.1007/s10758-020-09487-x
Sandrone, S., and Carlson, C. (2021). Gamification and game-based education in neurology and neuroscience: Applications, challenges, and opportunities. Brain Disor. 1, 100008. doi: 10.1016/j.dscb.2021.100008
Shyr, W. J., Zeng, L. W., Lin, C. K., Lin, C. M., and Hsieh, W. Y. (2018). Application of an energy management system via the internet of things on a university campus. EURASIA J. Mathem. Sci. Technol. Educ. 14, 1759–1766. doi: 10.12973/ejmste/80790
Siddiqui, S. T., Alam, S., Khan, Z. A., and Gupta, A. (2019). “Cloud-based e-learning: using cloud computing platform for an effective e-learning,” in Smart Innovations in Communication and Computational Sciences (Singapore: Springer) 335–346. doi: 10.1007/978-981-13-2414-7_31
Soni, V. D. (2019). IoT connected with e-learning. Int. J. Integr. Educ. 2, 273–277. doi: 10.31149/ijie.v2i5.496
Sukirman, S., Ibrahim, L. F. M., Said, C. S., and Murtiyasa, B. (2022). A strategy of learning computational thinking through game based in virtual reality: systematic review and conceptual framework. Inf. Educ. 21, 179–200. doi: 10.15388/infedu.2022.07
Tan, P., Wu, H., Li, P., and Xu, H. (2018). Teaching management system with applications of RFID and IoT technology. Educ. Sci. 8, 26, doi: 10.3390/educsci8010026
Theelen, H., Van den Beemt, A., and den Brok, P. (2019). Classroom simulations in teacher education to support preservice teachers' interpersonal competence: A systematic literature review. Comput. Educ. 129, 14–26. doi: 10.1016/j.compedu.2018.10.015
Towers, A., Field, J., Stokes, C., Maddock, S., and Martin, N. (2019). A scoping review of the use and application of virtual reality in pre-clinical dental education. Br. Dental J. 226, 358–366. doi: 10.1038/s41415-019-0041-0
Wang, J., and Zhao, B. (2021). Intelligent system for interactive online education based on cloud big data analytics. J. Intell. Fuzzy Syst. 40, 2839–2849. doi: 10.3233/JIFS-189324
Wang, Y. (2021). Physical education teaching in colleges and universities assisted by virtual reality technology based on artificial intelligence. Mathem. Probl. Eng. 2021, 5582716. doi: 10.1155/2021/5582716
Ward, V., House, A., and Hamer, S. (2009). Developing a framework for transferring knowledge into action: a thematic analysis of the literature. J. Health Serv. Res. Policy 14, 156–164. doi: 10.1258/jhsrp.2009.008120
Weaver, K., and Roche, C. C. (2019). Learning the cranial nerves: a low-tech gamified teaching strategy. J. Nurs. Educ. 58, 553–553. doi: 10.3928/01484834-20190819-12
Wong, G., Greenhalgh, T., Westhorp, G., Buckingham, J., and Pawson, R. (2013). RAMESES publication standards: Meta-narrative reviews. J. Adv. Nurs. 69, 987–1004. doi: 10.1111/jan.12092
Wu, C. H., Tzeng, Y. L., and Huang, Y. M. (2020). Measuring performance in leaning process of digital game-based learning and static E-learning. Educ. Technol. Res. Dev. 68, 2215–2237. doi: 10.1007/s11423-020-09765-6
Wu, W., and Plakhtii, A. (2021). E-learning based on cloud computing. Int. J. Emerg. Technol. Lear. 16, 4–17. doi: 10.3991/ijet.v16i10.18579
Xin, L. (2022). Intelligent interactive english teaching discrete data modeling and simulation. Scient. Progr. 2022, 3807762. doi: 10.1155/2022/3807762
Yang, Y. (2020). Research on modeling and simulation of agent-based intelligent teaching system. Sci. J. Educ. 8, 27–31. doi: 10.11648/j.sjedu.20200801.15
Yilmaz, R., Yurdugül, H., Yilmaz, F. G. K., Sahin, M., Sulak, S., Aydin, F., et al. (2022). Smart MOOC integrated with intelligent tutoring: A system architecture and framework model proposal. Comput. Educ. Artif. Intell. 3, 100092. doi: 10.1016/j.caeai.2022.100092
York, J., and Dehaan, J. (2018). A Constructivist approach to game-based language learning: Student perceptions in a beginner-level EFL context. Int. J. Game-Based Lear. 8, 19–40. doi: 10.4018/IJGBL.2018010102
Yu, H. (2021). Application analysis of new internet multimedia technology in optimizing the ideological and political education system of college students. Wirel. Commun. Mobile Comput. 2021, 1–12. doi: 10.1155/2021/5557343
Zaguia, A., Ameyed, D., Haddar, M., Cheikhrouhou, O., and Hamam, H. (2021). Cognitive IoT-based e-learning system: enabling context-aware remote schooling during the pandemic. J. Healthcare Eng. 2021, 7358874. doi: 10.1155/2021/7358874
Zainuddin, Z., Chu, S. K. W., Shujahat, M., and Perera, C. J. (2020). The impact of gamification on learning and instruction: A systematic review of empirical evidence. Educ. Res. Rev. 30, 100326. doi: 10.1016/j.edurev.2020.100326
Zeeshan, K., Hämäläinen, T., and Neittaanmäki, P. (2022). Internet of Things for sustainable smart education: An overview. Sustainability 14, 4293. doi: 10.3390/su14074293
Zhou, J. (2021). Virtual reality sports auxiliary training system based on embedded system and computer technology. Microproc. Microsyst. 82, 103944. doi: 10.1016/j.micpro.2021.103944
Zhu, X., and Kou, F. (2021). Three-dimensional simulation of swimming training based on android mobile system and virtual reality technology. Microproc. Microsyst. 82, 103908. doi: 10.1016/j.micpro.2021.103908
Keywords: e-learning, intelligent interactive system, MOOC, VR technology, AR technology, gamification
Citation: Ukenova A and Bekmanova G (2023) A review of intelligent interactive learning methods. Front. Comput. Sci. 5:1141649. doi: 10.3389/fcomp.2023.1141649
Received: 12 January 2023; Accepted: 17 July 2023;
Published: 14 August 2023.
Edited by:
Clifford A. Shaffer, Virginia Tech, United StatesReviewed by:
Shadi Aljawarneh, Jordan University of Science and Technology, JordanCopyright © 2023 Ukenova and Bekmanova. This is an open-access article distributed under the terms of the Creative Commons Attribution License (CC BY). The use, distribution or reproduction in other forums is permitted, provided the original author(s) and the copyright owner(s) are credited and that the original publication in this journal is cited, in accordance with accepted academic practice. No use, distribution or reproduction is permitted which does not comply with these terms.
*Correspondence: Aru Ukenova, dWtlbm92YWFydTA3QGdtYWlsLmNvbQ==
Disclaimer: All claims expressed in this article are solely those of the authors and do not necessarily represent those of their affiliated organizations, or those of the publisher, the editors and the reviewers. Any product that may be evaluated in this article or claim that may be made by its manufacturer is not guaranteed or endorsed by the publisher.
Research integrity at Frontiers
Learn more about the work of our research integrity team to safeguard the quality of each article we publish.