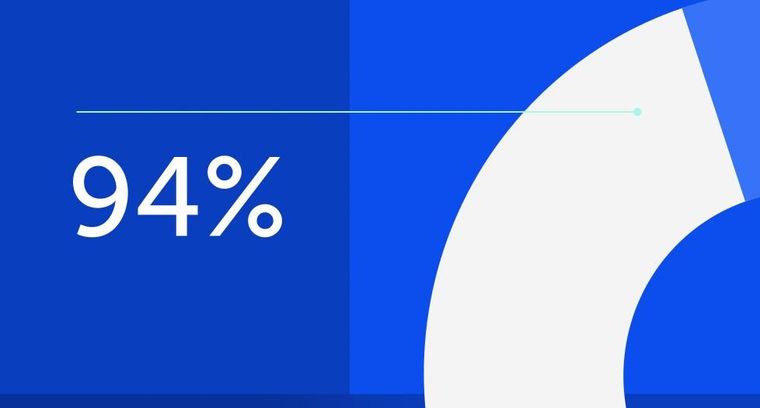
94% of researchers rate our articles as excellent or good
Learn more about the work of our research integrity team to safeguard the quality of each article we publish.
Find out more
REVIEW article
Front. Comput. Sci., 21 June 2023
Sec. Computer Vision
Volume 5 - 2023 | https://doi.org/10.3389/fcomp.2023.1136987
This article is part of the Research TopicPerceptual Organization in Computer and Biological VisionView all 14 articles
Image understanding is often conceived as a hierarchical process with many levels, where complexity and invariance of object representation gradually increase with level in the hierarchy. In contrast, neurophysiological studies have shown that figure-ground organization and border ownership coding, which imply understanding of the object structure of an image, occur at levels as low as V1 and V2 of the visual cortex. This cannot be the result of back-projections from object recognition centers because border-ownership signals appear well-before shape selective responses emerge in inferotemporal cortex. Ultra-fast border-ownership signals have been found not only for simple figure displays, but also for complex natural scenes. In this paper I review neurophysiological evidence for the hypothesis that the brain uses dedicated grouping mechanisms early on to link elementary features to larger entities we might call “proto-objects”, a process that is pre-attentive and does not rely on object recognition. The proto-object structures enable the system to individuate objects and provide permanence, to track moving objects and cope with the displacements caused by eye movements, and to select one object out of many and scrutinize the selected object. I sketch a novel experimental paradigm for identifying grouping circuits, describe a first application targeting area V4, which yielded negative results, and suggest targets for future applications of this paradigm.
We take it for granted that we see a world full of objects. But the images taken in by the eyes are just arrays of millions of pixels, and detecting objects from these arrays is a formidable task. It seems that the visual brain effortlessly provides us a representation of objects. Looking at Figure 1A, for example, we can easily answer questions like, what is the number of objects? how many corners has the green object? what is the color of the squares? which object is in the back? We can also compare two objects, or scrutinize a large complex object with multiple fixations. And when the display of Figure 1A is followed by the display of Figure 1B, we know that one object has moved from left to right. We have no doubt that it was one of the blue squares, although it is now neither blue nor a square. Complex natural images are certainly more difficult to process than the displays of Figure 1, but to understand vision, it seems to me, we should first understand how the visual brain enables us to make those assertions from such simple displays. What are the mechanisms that allow the brain to individuate objects from the stream of pixels, and how do they preserve their identity when the objects move? How do they achieve perceptual stability across eye movements, and how do they enable selective attention? This paper reviews studies that tries to answer those questions.
Figure 1. Object individuation. See text for further explanation. When (A, B) are displayed in sequence, one of the blue squares appears to move and at the same time change shape and color.
How does the visual cortex individuate objects? Since Hubel and Wiesel discovered the feature selectivity of simple, complex, and hypercomplex cells, early stages of visual cortex were thought to transform the pixel array into a representation of local image features like lines, edges, corners etc., which would then be assembled to larger entities that can be recognized as objects in inferior temporal cortex. This “hierarchical” scheme was questioned when a study showed that low-level cortical neurons that were supposed to signal lines and edges responded also to displays in which humans perceive illusory contours (von der Heydt et al., 1984). Contours are more than just edges and lines, they outline objects. At that time, illusory contours were commonly called “cognitive contours” because they appeared to be the result of a high-level, cognitive process, the system inferring a shape (like a triangle). Claiming that such contours are represented in a cortical area as low as V2 was to many a shock.
But the tide of vision sciences then had washed up the Fourier analyzer theory and neurophysiologists looked at the visual cortex as banks of spatial frequency filters. While this had the advantage of the convenient formalism of linear filtering, there were other indications (besides illusory contours) that cortical processing is highly non-linear from the beginning. In primary visual cortex it is not uncommon to find cells that respond to lines, but not to a grating of lines, and cells that respond vigorously to a sinusoidal grating of certain spatial frequency, but are totally unresponsive to the same grating when present as the 3rd harmonic component in a square wave grating (von der Heydt et al., 1992).
It took more than a decade until another perceptual phenomenon was found to have a correlate in visual cortex: figure-ground organization. Neurons in primary visual cortex respond to a texture in a “figure” region more strongly than to the same texture in a “ground” region (Lamme, 1995). Apparently, neurons at this low level already “know” what in the image is a figure, something that might be an object. But the tide of vision science then had surfaced another theory: coherent oscillations of neural firing were proposed to be the glue that holds the local features together as objects. Selective attention was thought to increase coherent oscillations which would lead to conscious perception. And the idea of the hierarchical scheme lives on in today's deep convolutional neural networks.
Neurophysiology led to another surprising discovery, neural selectivity for “border ownership” (Zhou et al., 2000). Figure 2 shows the basic finding. The responses of edge selective neurons, including the “simple” and “complex” types of Hubel and Wiesel, depend on how an edge is a feature of an object. The neuron illustrated responds strongly to the upper right edge of a square, and much less to the lower left edge. That is, the neuron responds to the identical local pattern differently, depending on whether it is an edge of an object to the bottom left of the receptive field, or an edge of an object to the top right. Indeed, for any location and orientation of receptive fields, there are two populations of neurons, those that “prefer” the object on one side of the receptive field, and those that prefer the object on the other side. Some respond also to lines, but many are strictly edge selective.
Figure 2. Border ownership selectivity. (A) The edge of a square figure is presented in the receptive field (oval) of a V2 neuron with the figure located either to the lower left or to the upper right. Note that the contrast was reversed between the two displays so as to compare locally identical stimulus conditions. Each trial started with a uniform field, and figure color and background color were both changed symmetrically at stimulus onset. The graphic depicts only tests with light-dark edges, but displays with reversed contrast were also tested, resulting in four basic conditions. Raster plots show the responses of the neuron, the curves show the time course of the mean firing rates. (B) Dashed line, time course of the difference between responses to preferred and non-preferred sides of figure—the “border ownership signal”—averaged across the neurons with significant effect of border ownership from one animal (left ordinate). Shading indicates standard error of the mean. Thin solid line, time course of responses (mean over the two figure locations, right ordinate).
Zhou et al. termed this selectivity for “border ownership”, adopting a term from the classic study by Nakayama et al. (1989) for the phenomenon that stereoscopic cues that change the way a border is perceptually assigned also affect object recognition: recognition of partly occluded objects is little impaired if the borders between occluded and occluding regions are stereoscopically assigned to the occluding regions (rendering them foreground objects), but is strongly impaired if these borders are stereoscopically assigned to the visible regions of the object.
The bottom of Figure 2A shows the time course of the neuron's mean firing rates. Because for each neuron with a border ownership preference one can find another neuron with the opposite preference, the two raster plots and the corresponding red and blue curves can be conceived as the simultaneous responses of a pair of neurons of opposite border ownership preferences. We also refer to the difference between the two as the “border ownership signal” (Figure 2B, dashed line, shading indicates SEM; from Zhang and von der Heydt, 2010). The border ownership signal is delayed by only about 15 ms relative to the mean response (thin line). These are responses from V2 neurons; border ownership signals of V1 have a similar time course. Note that Lamme's figure enhancement effect (where neurons respond to texture elements inside a figure) emerges later, about 50 ms after the response onset (Lamme, 1995).
The neuron of Figure 2 and the border ownership data to be reviewed below were recorded in rhesus macaques, but there is no doubt that the human visual cortex also represents contours by pairs of neurons of opposite border ownership preferences. A powerful paradigm for revealing selective neural coding is to demonstrate an adaptation aftereffect, which is based on the fact that cortical neurons exhibit short-term depression. Sure enough, it turned out that the classic tilt aftereffect is border-ownership selective. After adapting to a tilted edge that is owned by a figure on one side, a negative tilt aftereffect appears when the adapted location is tested with edges of figures on the same side, but not when tested with figures on the other side. And by alternating both, side-of-figure and tilt, during adaptation, one can produce two simultaneous tilt aftereffects in opposite directions at the same location. Thus, there are two populations of neurons that can be adapted separately (von der Heydt et al., 2005).
Are experiments with simple geometrical figures conclusive? The system may not need sophisticated algorithms to detect an isolated figure as in the displays of Figure 2. Other configurations that have been used in the early border ownership studies, like two overlapping figures, are also relatively simple compared to the complexity of natural scenes. Would neurons in V2 or V1 signal border ownership in natural scenes? Jonathan Williford tested neurons with large numbers of natural scenes (Williford and von der Heydt, 2016a). Using images from the Berkeley Segmentation Dataset (Martin et al., 2001) he selected many points on occluding contours for testing neurons (examples in Figure 3A). In the experiments, a fixation target for the monkey was embedded so that the selected points would be centered in the receptive field of the recorded neuron, and the image was rotated so that the contour matched the preferred orientation of the neuron. As in the standard border ownership test with squares, four conditions were tested: border ownership was controlled by rotating the image 180°, edge contrast was controlled by inverting the colors of the image so as to flip the colors between the regions adjacent to the contour. The data of this study are publicly available (Williford and von der Heydt, 2016b).
Figure 3. Neurons signal border ownership consistently across natural images. (A) Examples of images tested. Red circles show points where border ownership signals were measured (“scene points”). (B) Border ownership signals of an example V2 neuron normalized to the maximum and sorted. The signals were consistent for 74% of the 177 scene points tested. (C) Performance of a computational model on the 2,205 scene points tested in the neurons. The model was consistent for 69% of the points. (D) Distribution of the percentage of consistent signals of 65 V2 neurons with significant (p < 0.01) border ownership selectivity. Each neuron was tested with between 10 and 177 scene points (mean 43).
The first question was, can V2 neurons consistently signal border ownership under natural conditions? Each neuron was tested on many scene points (43 on average). The graph in Figure 3B shows the border ownership signals of an example neuron that was tested on 177 scene points. In seventy-nine percent of the cases the signals were consistent (plotted as positive in the graph). Consistency varied between neurons (Figure 3D). Out of 65, thirteen were over 80% consistent. In light of the hierarchical model of cortical processing, which is still widely accepted, the finding of consistent border ownership signaling in an area as low as V2 is highly surprising.
The burning question is now, could border ownership modulation at this low level be the result of top-down projections from higher-level object recognition areas? Figure 4A shows a summary of the neuronal latencies (the time from stimulus onset to the beginning of responses) that have been reported for the various visual areas (after Bullier et al., 2001). One can see that neurons in object recognition areas in inferior temporal cortex (including IT, TEx, TPO) respond relatively late. Of these, posterior IT (TPO) has the shortest latencies. To derive a prediction I use here the paper by Brincat and Connor (2006) who studied neuronal shape selectivity in the awake behaving conditions similar to those of the border ownership studies. Their study found that the response latencies in TPO depend on the type of responses within the area, with non-linear (shape selective) neurons having longer latencies than linear (unselective) neurons. The mean response for the shape selective group (green curve in their Figure 2B) reaches half-maximal strength at 130 ms. Thus, if border ownership selectivity in V2 depended on object recognition, the signal for natural scenes would reach half-maximal strength only at 130 ms (or later, depending on delays added by the projection down to V2). Figure 4B “Prediction” shows how the earliest border ownership signals would then look like for natural scenes (red line) compared to the signals for displays of squares (dashed black line, half-max strength at 68 ms according to Zhou et al., 2000). What the experiment actually showed was that the border ownership signals for the two kinds of displays rise simultaneously (Figure 4B, Data) (Williford and von der Heydt, 2016a). We conclude that the cognitive explanation is untenable. The border ownership signals are faster than shape recognition in IT. This is the beauty of neurophysiology: it can easily rule out alternative hypotheses that would be difficult to discriminate with psychological or computational arguments.
Figure 4. Selectivity of V2 neurons for border ownership in natural scenes cannot be the result of back-projections from object recognition centers in the inferotemporal cortex (“cognitive explanation”) because it appears well-before shape selective responses emerge in inferotemporal cortex. (A) Summary of visual latency data across brain areas in monkey. Shape selectivity occurs first in posterior temporal cortex (TPo); arrow shows time of half-maximal strength of mean response of shape selective cells from a study in behaving monkey. (B) The border ownership signals for squares (black dashed lines) and natural scenes (red solid lines), as predicted, and as observed.
Attentive enhancement might be a plausible explanation for the figure-enhancement effect. When a figure pops up, it automatically attracts attention. But if a neuron responds more to a figure when it pops up here than when it pops up there, that difference cannot be the result of attention. The two displays in Figure 2 both contain a figure, the figure in the bottom display being flipped about the edge in the receptive field relative to the top display, and some neurons preferred one location, while others preferred the other location. It's a property of the neurons. Qiu et al. (2007) showed that border ownership and attentional modulation are separable aspects of neuronal function, and discovered an interesting correlation.
When the display contained several separate figures, and the monkey attended to one or another, border ownership modulation was found whether the figure at the receptive field was attended or ignored; there was only a slight difference in strength of modulation (Figure 5A).
Figure 5. The influence of attention on border ownership signaling. (A) When objects are isolated, border ownership signals are similar whether the object at the receptive field is attended (red line) or ignored (blue line). (B) When objects overlap, side of attention and side of border ownership modulate the responses. Raster plots show responses of an example neuron. Dashed oval, receptive field. Lightening symbols indicate side of attention. Scatter plot shows the relation between attentional modulation and border ownership modulation across neurons of area V2. Red dots represent neurons that were influenced by both, attention and border ownership in form of significant main effects and/or significant interaction (p < 0.01).
And yet, attention does modulate the responses in displays in which objects partially occlude one another, and it interacts with border ownership in an interesting way. Figure 5B shows at the top the responses of an example neuron to the occluding contour. The two border ownership configurations are represented left and right, and side of attention in top and bottom rows. One can see that left was the preferred side of border ownership, and that the responses were enhanced when attention was on the left-hand object, compared to the right-hand object, for both border ownership conditions. Thus, attention on the neuron's preferred border ownership side enhanced the responses relative to attention on the non-preferred side, irrespective of the direction occlusion. But attention did not override the border ownership signal. The results of Figure 5B, while showing the responses of one neuron to the two directions of border ownership, can be interpreted as the responses of two neurons with opposite border ownership preferences, which shows that, whether attention is on the left-hand object (top row) or on the right-hand object (bottom row), responses are stronger when the left-hand object owns the border.
Like in this neuron, the rule is that attentive enhancement is on the preferred side of border ownership, as shown by the scatter plot at the bottom of Figure 5B. The two factors were roughly additive, but there was a small but significant positive interaction. That is, attention enhanced responses more on the foreground object than on the background object.
The reader can experience the attention effect when looking at pictures in which border ownership is ambiguous. Figure 6 shows an artist's depiction of Napoleon's tomb on St. Helena. And not only his tomb, also his ghost, standing beside the tomb. To see him, direct your attention to the space between the trees!—The shape pops out because, when you first look at the picture, the neurons representing the borders between trees and sky are biased so that those assigning ownership to the tree regions prevail (smaller regions produce stronger border ownership signals than larger regions; the Gestalt Law of Proximity). But when their opponent neurons are enhanced by attention, ownership shifts to the sky region, and you can perceive its shape: the ghost.
I do not see the practical value of having the attention mechanism interfere with border ownership coding—besides the ability to see ghosts—but the linkage between attention effect and ownership preference helps in identifying the mechanism of border ownership selectivity. This linkage was a surprise because selective attention effects are usually phrased in terms of regions (left vs. right hemifield, figure vs. ground region) rather than borders.
How does a neuron of V1 or V2 know that the edge stimulating its receptive field is part of a figure? Could it be that border ownership selective neurons in V1/V2 are just Hubel and Wiesel's simple and complex cells that receive an additional modulating input from cells with large receptive fields that sense the presence of a big shape that might be an object? And that this modulating circuit is also used in top-down selective attention? That might explain why the attention effect is asymmetric about the receptive field, producing enhancement of responses when the attended object is on the preferred side of ownership.
The receptive fields of the neurons studied were near-foveal and typically about 0.5 deg in diameter, whereas the squares used to demonstrate border ownership selectivity measured 4 deg on a side or more. The neurons must be sensitive to the context far beyond the classical receptive field. Figure 7 illustrates an experiment in which the context influence was explored (Zhang and von der Heydt, 2010). The little gray specks left and right of the calibration mark show the classical receptive field of the neuron studied, and the vertical lines through the receptive fields depict the edges of the square stimuli, separately for the figure-left and figure-right conditions (the plot combines the results of two experiments, one with a 4° square, and one with a 7° square). To demonstrate the context effect, the figures were fragmented into eight pieces which were presented in random combinations, one combination per trial.
Figure 7. The extent of context integration in border ownership selectivity. This experiment probed locations where the responses of a border ownership selective neuron to a local stimulus could be modulated by contextual contours. First, the “classical receptive field” was mapped with a small probe stimulus. The map (Gray specks) is depicted twice to allow graphic representation of the two border ownership conditions. The contours of a square figure were fragmented into eight pieces (four edges and four corners) that were presented in random combinations, one combination at a time. One edge of the square was centered in the receptive field to stimulate the neuron, the other “contextual” fragments were presented outside the receptive field, as depicted in red and blue (they appeared either to the left or to the right of the receptive field, depending on the border ownership condition). The graph combines the results of two experiments, with 4° and 7° squares, respectively. The contextual fragments modulated the responses as indicated by the color of the fragments, red for facilitation, blue for suppression. One can see that, despite the tiny size of the classical receptive field, the contextual stimuli modulated the responses almost everywhere, those on the left producing facilitation and those on the right producing suppression. The bottom graph shows the result of control trials in which the edge in the receptive field was absent: none of the contextual fragments produced a response by itself.
The top plot corresponds to the trials in which the various combinations of the contextual fragments were presented in addition to the edge fragment in the receptive field (the “center edge” for short). The bottom plot shows the trials in which the same contextual fragments were presented without the center edge. The effect of each context fragment is indicated by color, red meaning enhancement of responses relative to the response to the center edge alone, blue meaning suppression. One can see that, for both figure sizes, the fragments to the left of the receptive field enhanced the center edge response, while the fragments to the right suppressed it. The bottom plot shows that the contextual fragments alone (without the center edge) did not evoke any responses.
The results from this kind of experiment show that, while neurons respond only to features within their small classical receptive fields, their responses can be modulated by the image context in a range that is much larger than the classical receptive field.
Nan R. Zhang also explored the context influence in the case of overlapping squares in which the border between the two squares (which perceptually belongs to the overlaying square) was placed in the receptive field. This situation is different in that there are figures on either side of the receptive field and mechanisms that simply detect the presence of a shape on one side would not work. The results showed that in this case the presence or absence of T-junctions, L-junctions (corners), and orthogonal edges, outside the receptive field modulated the responses to the center edge (von der Heydt and Zhang, 2018).
In area V4, where neurons are often selective for local contour features, Anitha Pasupathy and coworkers discovered that neurons that respond selectively to cusps are suppressed when the cusps are not object features, but accidental features produced by occlusion (Bushnell et al., 2011). Border ownership also affected the responses of shape selective neurons in infero-temporal cortex (Baylis and Driver, 2001).
The studies summarized so far led to the hypothesis that border ownership selectivity involves “grouping cells” that sum responses of feature neurons (including simple and complex types) and, via back projection, facilitate the responses of the same feature neurons, as sketched in Figure 8. Craft et al. (2007) designed a computational model in which grouping cells have fuzzy annular summation templates that are selective for oriented feature signals of roughly co-circular configuration. The summation of feature signals is linear, the feedback to the feature neurons is multiplicative. For example, the blue G cell in Figure 8 sums the responses of orientation selective neurons with receptive fields depicted in blue, and enhances their responses by feedback (“Facilitation”). This feedback makes those neurons border-ownership selective, as indicated by arrows on the receptive field symbols. Grouping cells also sum signals that do not correspond object features but indicate the layout of objects in depth, such as stereoscopic depth and accidental features produced by interposition, and the neurons providing these signals do not receive modulatory feedback. For example, T-junctions, and termination of lines at the contour, and orthogonal edges which contribute to border ownership (von der Heydt and Zhang, 2018).
Figure 8. The grouping cell hypothesis. Schematic depiction of cortical activation by two objects (black outlines). The object contours stimulate receptive fields of edge selective feature neurons (ovals) which send their signals to specific processing centers at higher levels, and, via collaterals, also excite grouping cells G. Grouping cells project back to the same feature neurons, facilitating their responses. To select an object, top-down attention signals excite the corresponding G cell (lightning symbol) thereby enhancing the feature signals of the object for further processing. Grouping cells are thought to sum also signals that are not evoked by object features but by background structures cut off by occlusion, such as line terminations, L-junctions, and T-junctions. These inputs are not depicted here for clarity.
Note that each piece of contour is represented by two groups of feature neurons for the two directions of border ownership, as illustrated in Figure 8 by the red and blue receptive field symbols in the center. Of the two objects depicted in black, the one to the left will activate the blue G cell, the one to the right, the red G cell. Selective attention, which consists in top-down activation of G cells (yellow lightning shapes), can enhance either the feature signals of the left-hand object (Figure 8, bottom) or those of the right-hand object (Figure 8, top). I have previously suggested that activation of a G cell (bottom-up or top-down) represents a “proto-object” (von der Heydt, 2015). This term had already been used in psychological studies, implying a preliminary object representation that may later be completed. The steep onset and early peak of border ownership signals do not indicate gradual completion but a one-shot process. But inspecting an object with multiple fixations seems to accumulate information about the details of an object in some central representation, which looks like gradual completion of an object representation. So, the emerging border ownership modulation and the enhanced feature signals might well be called a “proto-object”. Where the completion occurs in the brain, and how, are questions that are worthwhile investigating.
The grouping cell hypothesis proposes that G cells come with summation templates of different sizes to accommodate the variety of objects. There must be a gamut of template sizes, and templates of each size must cover the visual field densely. The numbers of G cells required might raise concerns, but that number is actually quite small, much smaller than the number of feature cells. This is because G cell templates only have low spatial resolution, the “resolution of attention” (Intriligator and Cavanagh, 2001), which is about 20 times lower than the feature resolution of the system. This means that, for the smallest template size, covering the visual field densely requires 400 times fewer G cells that feature cells. And the numbers of G cells with larger templates decreases in inverse proportion to square of size.
Besides the size of their summation template and the preference for co-circular signals, the hypothetical G cells are not particularly selective. The summation templates are fuzzy. Thus, round shapes, squares, and triangles, would all activate the G cells about as much as the potato shapes depicted in Figure 8. G cells are not “grandmother cells”. Detecting a grandmother requires selectivity for conjunctions; not every person with white hair is a grandmother. By contrast, summing of features in G cells is disjunctive. Changing just one little T junction can flip foreground and background. In the case of partially overlapping squares, the border ownership signals for the occluding contour were found to grow with the number of indicative features, but saturate early: on average, one single feature (any T junction, L junction, or orthogonal edge) already produced half maximal signal strength (von der Heydt and Zhang, 2018). Selectivity was also found for border ownership defined by stereoscopic cues (Qiu and von der Heydt, 2005), motion parallax (von der Heydt et al., 2003), transparent overlay (Qiu and von der Heydt, 2007), and display history (O'Herron and von der Heydt, 2011). In the spirit of the grandmother cells terminology, G cells might be termed “TSA cells”: “if you see something, say something.”1
What characterizes an object are its feature signals. By targeting one G cell, the top-down attention mechanism can simultaneously enhance a large number of feature signals that characterize the exact shape, color, etc. of the target object. The G cells are not in the object processing stream, they serve only as handles to pick objects and allow attentive selection to route feature information of individual objects to higher processing centers, like those in inferior temporal cortex. For example, to read out the color of one of the squares in Figure 1, attention would boost the activity of a G cell according to location, while activating at the same time a color processing center downstream. From the feature neurons that are enhanced by the G cell, which include many color-coded edge selective neurons (Friedman et al., 2003), the color processor will compute the color of that square. Similarly, activating other processing areas will identify object shape and other object attributes.
As mentioned, every border between image regions activates pairs of border ownership selective neurons with opposite preferences. One such pair is depicted in Figure 8, the pair with receptive fields on the border between the two objects. This is to illustrate a specific prediction of the hypothesis, namely that attention to one side only facilitates the neuron that prefers that side of ownership. Thus, the grouping cell hypothesis predicts the correlation that was experimentally observed (Figure 5B). It predicts a hundred percent, whereas the actual correlation was lower, which is most likely due to the presence of basic spatial attention mechanisms in addition to the grouping cell mechanism. Attention may involve the grouping cell mechanism only in situations where simple spatial selection is not feasible, such as situations of partial occlusion, where the occluding contour should not be conflated with features of the background object.
Computational modeling shows the advantage of grouping cells in selective attention (Mihalas et al., 2011). Different from spatial attention models, the grouping cell model automatically localizes and “zooms in” on structures likely to be objects. The top-down attention signal only needs to enhance the G cell activity broadly in the region to be attended, and the network will direct the activity to potential objects in that region and focus activity on the size of G cell templates that fit each object best. The model replicates findings of perceptual studies showing that “objectness” guides and captures attention.
The above models (Craft et al., 2007; Mihalas et al., 2011) work on synthetic images of simple geometric shapes. A fully image computable model of the grouping mechanism was created by Hu et al. (2019a) and applied to natural images. The model produced contours as well as border ownership. Although it has no free parameters, Hu et al. found its performance to be overall comparable to state-of-the-art computer vision approaches that achieved their performance through extensive training on thousands of labeled images, fitting large numbers of free parameters.
The Hu et al. model has three layers of cells with retinotopic receptive fields, Simple cells (S), Border-ownership cells (B), and Grouping cells (G). Each S cell excites pairs of B cells for the two possible directions of border ownership. B cells thus inherit their receptive field selectivity from the S cells. G cells sum B cell responses according to fuzzy annular templates selectively for “co-circularity”. The model works in an iterative manner. A given G cell sums the responses of one of the two B cells from each position and orientation, and facilitates the same B cells by modulatory feedback (see Figure 8) and suppresses the partner B cells by inhibitory feedback. This is motivated by neurophysiological results showing that image fragments placed outside the classical receptive field of a border ownership neuron can cause enhancement of the neuron's activity when placed on its preferred side, and suppression if placed on its non-preferred side (see Figure 7; the suppression is not depicted in Figure 8 for clarity). The model uses a scale pyramid of G cell template sizes, and pools information across different scales in a coarse-to-fine manner, with information from coarser scales first being upsampled to the resolution of the finer scale before being combined additively. A logistic function enforces competition between B cells such that their total activity was conserved.
Comparing with the neurophysiological data on the 2205 scene points tested in Williford and von der Heydt (2016a), Hu et al. found that their model achieved 69% consistent border ownership assignment, which was typical for V2 neurons (Figure 3). But the neurons varied, and many were actually more consistent. The neuron tested with the most images was 79% consistent across 177 scene points, and some were >90% consistent. This is no surprise because the Hu et al. model is simple. As Craft et al. (2007) observed, having grouping cells sum co-circular edge signals alone will not assign border ownership correctly for overlapping figures, which the neurons do resolve. In Craft's model, grouping cell summation also included T-junction signals. As we saw above, the neurophysiology of border ownership coding suggests that grouping cells integrate a variety of different features as figure-ground indicators (von der Heydt and Zhang, 2018), and there seems to be a diversity of grouping cells, each using a subset of potential indicators. As a result, the consistency of border ownership coding across images varies from cell to cell (Figure 3). As Hu et al. (2019a) show, there was little similarity between two neurons when comparing their border ownership signals on a common set of scene points. Even highly consistent neurons are not entirely consistent with each other.
The computation of border ownership in natural scenes might be improved by having grouping cells include also local figure-ground indicators, similarly as the Craft et al. model included T-junction signals in dealing with simple geometrical figures.
Do grouping cells exist? The observations of border ownership selectivity and attentive selection could also be explained by other hypotheses, for example, by propagating convexity signals along contours (Zhaoping, 2005), or by feedback projections in the cortical hierarchy from high-level areas with large receptive fields down to low levels with small receptive fields (Jehee et al., 2007), or simply by the magic of coherent oscillations. But there is one specific prediction of the grouping cell hypothesis: the top-down facilitation of feature neurons should lead to spiking synchrony, because all feature neurons that receive input from the same grouping cell (or cells) receive the identical spike trains. More specifically, synchrony should occur only between border ownership selective neurons when responding to the same object (Bound condition, Figure 9A); and only between pairs of neurons with “consistent” border ownership preferences (red dashed lines in Figure 9A), but not between “inconsistent” pairs (gray dashed lines). The hypothesis further predicts that synchrony will be found between neurons that are widely separated in cortex, because the grouping cells must be able to encompass the images of extended objects represented retinotopically in visual cortex.
Figure 9. Spiking synchrony between border ownership selective neurons. (A) According to the hypothesis a single grouping cell G contacts many V1/V2 neurons via recurrent projections (dashed arrows). These neurons receive identical spike trains when the grouping cell fires, which should lead to spike synchronization. Because the grouping input produces border ownership preference, the hypothesis predicts synchrony between neurons whose border ownership preferences point toward the activating object (consistent pairs, indicated by red dashed lines between receptive field symbols), but only when they respond to the same object (Bound), and not when they respond to different objects (Unbound). (B) Curves show the covariograms between spike trains of pairs of neurons under the experimental conditions shown schematically above: dot, fixation point; red ovals, receptive fields; yellow asterisk, focus of attention (ovals and asterisks were not part of the display). Consistent pairs produced a sharp peak at zero (coincidence) when stimulated by the same object, whether the object was attended or ignored (yellow and black heavy lines), in contrast to stimulation with different objects which did not produce a peak (thin line). Inconsistent pairs produced rather flat covariograms. Bar graphs show the frequency of coincidences within 40 ms for the two kinds of pairs under the three experimental conditions. There was a significant difference between Bound and Unbound for consistent pairs, and a highly significant interaction between pair type and binding condition (This figure shows the data from the quartile of trials with the fastest behavioral responses; results for all trials combined were similar except for an additional effect of attention, see Martin and von der Heydt, 2015). (C) Synchrony (frequency of 5-ms coincidences) as a function of the distance in cortex between the neurons of each pair (left-hand plot), and as a function of the difference between their preferred orientations (right-hand plot). Dashed lines, Mean. Neurons separated as widely as 13 mm fired synchronous spikes, as did neurons with different preferred orientations, indicating that individual grouping cells contact neurons representing distant features and features of different orientations.
Anne Martin tested these predictions, which was a difficult task. First, it required simultaneous stable recordings from two distant neurons, both of which had to be border ownership selective. Second, the objects had to be shaped according to the positions and orientations of the receptive fields of the two neurons encountered (sometimes it was impossible to construct a simple figure that would stimulate both neurons).
The main results are shown in Figure 9B (Martin and von der Heydt, 2015). The three different display- and attention conditions are depicted schematically at the top. While the subject fixated gaze on a fixation target (black dot), three figures were presented so that the two receptive fields (red ovals) were either stimulated by the same figure (Bound) or by different figures (Unbound). Additionally, attention was controlled (asterisk) by having the subject detect the moment of a subtle modification of shape that occurred predictably in one of the figures. Below, the frequency of spike coincidences is plotted as a function of lag time, after correcting for random coincidences (a cross-correlation function called “covariogram”). There is a sharp peak at zero lag in the Bound condition, but not the Unbound condition; and for Consistent pairs, but not for Inconsistent pairs. The bar graphs to the right show the frequency of coincidences within 40 ms and the significance of the differences (the results were similar for 5-ms coincidences and the differences were also significant). This is exactly as predicted: in neurons that receive projections from a common grouping cell (i.e., neurons whose directions of border ownership preference are consistent) spiking synchrony increases when that grouping cell is activated (i.e., when both neurons are stimulated by a common object). I think the experiment cannot distinguish whether synchrony is due to single grouping cells or pools of such cells, but the sharp peak at zero lag of the covariograms in Figure 9B indicates coincidences of individual spikes.
Attention had little effect on synchrony (just as it produced little enhancement of responses, Figure 5A).
Spiking synchrony between neurons in primary visual cortex has generally been found to fall off rapidly with distance between neurons, reaching zero at 4 mm, which is approximately the maximum length of horizontal fibers in V1, and to be specific to neurons with like orientations (Smith and Kohn, 2008). The grouping hypothesis predicts the opposite: to be flexible, the grouping mechanisms must encompass neurons with widely separated receptive fields and a variety of orientation preferences. And indeed, in the above experiment, neurons separated by as much as 13 mm showed tight (5 ms) synchrony, and finding synchrony did not depend on similarity of preferred orientations (Figure 9C).
Is grouping behaviorally relevant? The task in the experiment of Figure 9 required detection of a small shape change produced by counterphase movements of the edges in the two receptive fields; the behavioral response depended on grouping these edges to one object. Thus, the hypothesis predicts that, if the strength of the grouping feedback fluctuates from trial to trial, stronger synchrony should be followed by a faster behavioral response. Anne Martin discovered that the response time correlated negatively with synchrony in consistent pairs in the “Bound” condition, whereas inconsistent pairs showed no such correlation. In the quartile of trials with the strongest synchrony the mean response time was 8 ms shorter than in the quartile with the weakest synchrony. Thus, the behavioral responses were fastest when neural grouping was strongest, as predicted.
One question we glanced over above is, how can modulatory common input produce synchrony? Spiking synchrony is generally observed when two neurons are activated by a common spike train, but, according to the theory, grouping cell feedback to feature neurons does not activate, but only modulates existing activity (see example in Figure 7 showing that context features alone do not activate). Nobuhiko Wagatsuma and Ernst Niebur explored synchrony between pairs of feature neurons with a spiking model. They modeled the afferent inputs by independent spike trains activating AMPA receptors, and the modulatory grouping cell input by a common spike train activating NMDA receptors (using a standard computational model for generic NMDA receptors). Surprisingly, this model produced synchrony, and even the exact shape of the experimental covariograms and the observed synchrony at millisecond precision (Wagatsuma et al., 2016).
As we have seen, experiments and modeling confirm a critical prediction of the grouping cell theory: that pairs of border ownership selective cells with consistent direction preferences, when activated by a common object, exhibit spike train synchrony with a cross-correlation function whose shape is characteristic for common modulatory input. Next, we will consider another critical prediction of the theory, persistence.
It has been argued that vision—in contrast to audition—does not need short-term memory because the visual information is continuously available so that attention can always pick what is needed. But I argue that vision needs a short-term memory too. What would be the use of grouping features to objects if that would all be lost in a blink?
O'Herron and von der Heydt (2009) devised experiments to test if border ownership signals persist. The idea was to present an edge in the receptive field that is owned by a figure on one side, as in the standard test of Figure 2, and then, keeping the edge in the receptive field, switch to a display in which ownership of the edge is ambiguous. This simple paradigm has produced amazing results. Figure 10, top, shows the sequence schematically for ownership-left (the corresponding displays for ownership-right were also tested to measure the border ownership signal). Below, the red curve shows the average time course of the signal. It rises steeply and stays high during the figure phase, as in the standard test, but in the ambiguous phase it declines only slowly. For comparison, when the figure was flipped to the other side keeping the edge contrast (Figure 10, 2nd row of insets from top), the signal changed quickly to negative values (blue curve). The difference between the time constants was 20-fold. Thus, border ownership signals persist for a second or more. This experiment also shows that the persistence is not due to inherent persistence of responses in the recorded neurons, because in the “flip” condition their responses change rapidly.
Figure 10. Persistence of border ownership signals. The edge of a square was presented in the receptive field for 0.5 s and then switched to an ambiguous edge (in other trials, side of figure and contrast were reversed, like in the standard test). In the other condition, the square was flipped to the other side. Red line shows the time course of the border ownership signal when the edge is made ambiguous: The signal decays slowly. Blue line, time course of the signal when the square was flipped to the other side: The signal reverses quickly.
The paradigm of Figure 10 is somewhat artificial in that it does not have a simple interpretation in terms of objects with natural continuity. In the top display sequence, the initially presented object disappears and a bipartite field appears, and in the sequence below, the initial object disappears, and a different object appears on the opposite side. To study persistence of border ownership signals in a more natural situation, Philip O'Herron designed an ingenious display sequence in which objects maintain continuity. He presented two partially overlapping figures and recorded responses to the common border (the occluding contour) when the occlusion cues reversed while the two figures were continuously displayed. The result was that the initial border assignment persisted for 2 s or more before reversing sign (O'Herron and von der Heydt, 2011). Control conditions showed that, when the final configuration of overlapping figures was presented without history, the signal assumed the final value quickly; and when a single figure was presented on one side and was then replaced by a figure on the opposite side, as in Figure 10 Figure flip, the signal also reversed quickly. These results are summarized schematically in Figure 11, where pairs of adjacent frames represent two object locations, O1 and O2 denote two objects, and the red arrow indicates direction of border ownership. Abrupt-onset and object-flip result in fast signal changes, whereas reversal of occlusion cues in the presence of both objects results in retarded reversal of the signal. It seems that object continuity includes continuity of depth relations. More generally, we hypothesize that the system represents location in space as an object attribute which has continuity unless there is an abrupt image event like onset or offset.
Figure 11. Schematic of display sequences producing fast and slow changes of border ownership signals. First and Second are two phases of stimulation. Outlines represent object locations; O1 and O2, objects; red arrow, direction of border ownership. When overlapping objects appear suddenly, or when the object on one side disappears and another object appears on the opposite side, the signal changes fast. But when two objects are continuously present and ownership of the common border is reversed, the signal follows only slowly.
O'Herron also showed that the persistent ownership signals “remap” across saccades, a result that will be reviewed below. The persistence of border ownership signals is another example of the power of neurophysiology in providing clear answers to questions that are difficult to answer with psychological methods.
How is it possible that neural signals rise fast and decay slowly? Neurons in low-level visual areas must be able respond fast to the afferent signals from the retina which change swiftly with new information arriving after a fraction of a second. The memory-like behavior shown in Figure 10 is a puzzle for neural network theory. Traditional positive feedback models show attractor dynamics, with transient perturbations resulting in a quasi-permanent change of system state, whereas the responses of Figure 10 return to the original state after a transient. This is a question of very general interest because short-term memory underlies many kinds of behavior. Grant Gillary discovered that short-term depression, which is ubiquitous among cortical neurons, can create short-term persistence in derivative feedback circuits. If short-term depression acts differentially on positive and negative feedback projections between two coupled neurons, they can change their time constant dynamically, allowing for fast onset and slow decay (Gillary et al., 2017).
We see by moving our eyes. The eyes fixate, producing stable images for a moment, and then move rapidly to fixate another part of the scene. Each time, the images are displaced in the eyes. Humans as well as monkeys move fixation continually about 3–4 times per second. The reason why primates do this is obviously to be able to scrutinize different parts of a scene with the high-resolution center region of the retina and its corresponding processing apparatus in the brain. The system then synthesizes information from multiple fixations to represent complex objects and combines object representations to scene representations. From the computational standpoint this seems horribly complicated. At least I don't know of any technical system that would bounce a camera around four times per second. How our brain deals with this confusing input is a puzzle. One question is, why don't we perceive and are not disturbed by the frequent movements of the retinal image, but rather perceive a stable world. But the subjective stability is a minor issue compared to the question of how the system integrates object information across the eye movements.
To explain cross-saccadic integration, van Holst proposed the reafference principle (von Holst and Mittelstaedt, 1950). When the brain creates a signal that commands the eyes to move, he thought, it also produces an associated signal that tells the visual system about the impending eye movement and informs it about the direction and size of the image movement to expect. He called the change of retinal signals caused by the eye movement the “afference”, and the associated brain signal to the visual system the “reafference”. To create continuity the brain would have to correct the afference by the reafference, that is, to shift the image representation so as to cancel the image movement and thus achieve a stable internal representation (Figure 12A). The problem with this theory is that a shifter circuit that could remap the image representations would have to be huge. V1 and V2 each consist of over 100 million neurons and there is no other structure in the visual brain that could hold so much information.
Figure 12. The problem of dealing with image displacements caused by eye movements. (A) Reafference principle. When the eye movement control center sends a command to the eye muscles, it also sends a copy to the visual system indicating the impending eye movement. A shifter circuit shifts the visual representation accordingly to compensate for the image displacement caused by the eye movement. (B) Object pointer hypothesis. The visual system works with the moving image representation, but uses object pointers that are updated at each eye movement by a shifter circuit.
An alternative solution would be to work with image representations that move with every eye movement, and remap the object structure accordingly (Figure 12B). Instead of requiring a stabilized image representation, object-based attention would then only need object pointers that are updated with every eye movement. Zhu et al. (2020) conjectured that top-down attention signals activate object pointer cells whose signals are fed via a shifter circuit to grouping cells. This scheme would reduce the stabilization task from remapping millions of image signals to remapping a few object pointer signals. Assuming the system can maintain a number of object pointers, top-down attention could select to which object to attend, and the remapping would preserve its identity and enable the attention mechanism to keep focused on it, that is, keep enhancing the feature signals of that object across eye movements, or deliberately choose to focus on another object.
The hypothesis of object pointer remapping implies that the activation of grouping cells is being remapped to a new location with each eye movement. When an object appears, a grouping cell responds and will activate an object pointer. This activity persists and, by feedback, reinforces the activity of the grouping cell. When the eyes then make a saccade that moves the image of that object to the receptive field of another grouping cell, the shifter circuit will reroute the object pointer accordingly and its activity will flow down to the new grouping cell. Thus, the grouping cell in the new location will become active immediately. The result will be that border ownership is remapped, that is, the feature neurons that respond to the object in the new location will be biased immediately, without the need for new context processing.
O'Herron and von der Heydt (2013) tested this prediction as illustrated in Figure 13. Recording from a feature neuron they presented a figure so that its edges were outside the receptive field (Phase 1) and then replaced the figure with an ambiguous edge that coincided with one of the figure edges (Phase 2). After a while, the fixation point was moved, inducing the monkey to make a saccade that brought the receptive field onto the edge (Phase 3). The prediction was that the neuron's responses will reflect the previous ownership despite the absence of a figure. The graph at the bottom shows the population border ownership signal. There are no responses in Phases 1 and 2, as expected, because the receptive fields are in a blank region. During Phase 2, the fixation point moves (black arrow on time axis) eliciting a saccade that brings the receptive fields onto the edge. The neurons respond, and a border ownership signal emerges as predicted. This is about half a second after the figure was removed; border ownership is produced from memory.
Figure 13. Remapping of border ownership signals across saccades. Like in the memory experiment of Figure 10, a figure was presented (Phase 1) and then replaced by an ambiguous edge (Phase 2), but in this experiment, the neuron was not stimulated because the edges were outside its receptive field (dashed oval). Finally, a saccade was elicited that brought the receptive field onto the ambiguous edge (red arrow, Phase 3). Curve shows the mean border ownership signal (47 neurons). Black arrow on time axis, movement of fixation point, vertical dotted line with horizontal bracket, mean time of saccade with standard deviation. The border-ownership signal is close to zero during phases 1 and 2 because the neurons are not stimulated. But shortly after the saccade, when the edge stimulates the neurons, a signal emerges although border ownership is ambiguous. The neurons signal how the edge was owned before the saccade.
The results described so far are all based on variants of the border ownership paradigm and on recordings from V1, V2, and V4, and together they constitute strong evidence for the grouping cell theory. But the one crucial prediction of the theory, the existence of grouping cells has not yet been confirmed. Grouping cells might live in another brain region. In fact, finding persistence of the border ownership signal in areas like V1 and V2, where neuronal responses rise and fall fast, makes it seem unlikely to find grouping cells there.
Identifying grouping cells, to my knowledge, has only been attempted in one candidate area, V4, an area where some neurons have larger, fuzzy receptive fields and that has strong back projections to V2 and V1. Also, V4 is connected to both, the What and the Where pathways (Ungerleider and Mishkin, 1982; Ungerleider et al., 2008), and the function of grouping cells is just to pull out what is where.
Searching for grouping cells needs a different paradigm. The distinctive feature to look for is obviously the persistence of responses when an activating object disappears from view, as in O'Herron's demonstration of persistence of border ownership where an edge is substituted for a square. But we do not expect grouping cells to respond to edges; rather, they should respond best when an object is centered on their summation template.
Alex Zhang and Shude Zhu developed a new paradigm motivated by the phenomenon that objects persist perceptually when they are transiently occluded, a phenomenon called “object permanence”. When an object is occluded by another object passing in front of it and then reappears, we perceive it as the same object. We would be surprised if it had vanished, or if there were now two objects instead of one. The visual system holds the representations for a certain time even when the objects are invisible.
Figure 14 illustrates the new paradigm. The stratagem was to present an array of objects for visual search and, while the observing subject is scanning the array, transiently occlude some of the objects.
Figure 14. Searching for grouping cells—a novel paradigm. An array of test figures is presented, and monkey is instructed to scan the array in search for a figure that, when fixated, leads to reward. While monkey is scanning, opaque strips drift across the array (gray bars, depicted transparent for illustration only). For control of attention two shapes of figures are displayed, and a cue figure presented at the beginning of each trial (dotted outline) tells monkey which shape to look for. Line trace, eye movement trace of an example trial. Green circles, position of an example receptive field for each fixation.
To control top-down attention, the objects were of two different shapes and, before the array appeared, a cue object was displayed (dashed outline) that specified which shape to look for. The cue object disappeared when the array came on.
Figure 14 also shows the example of an eye movement trace of a trial in which a trapezoidal shape was cued. The monkey made four fixations, and four green circles indicate where the receptive field of an example neuron would be in each case (the circles are only for illustration, they were not part of the display). In fact, the array was constructed for each neuron being recorded so that, when one of the objects was fixated, another object would fall on the neuron's receptive field in most trials, and in other trials, a blank region. In the example, two fixations brought objects into the receptive field, one a trapezoid, and the other a square, while in two other fixations the receptive field landed on a blank region.
Occlusion was added by having a series of opaque gray strips drift across the array that occluded half of it at any time (the strips are depicted as transparent in the Figure just for illustration; in fact, display items that we call “occluded” were physically absent). Surprisingly, the subjects had no difficulty in dealing with that complication. Once they mastered the task without occlusions, they rapidly adjusted to the occlusions in just one session. This of course confirms the power of perceptual permanence.
In the new paradigm neurons respond to static objects brought into their receptive fields by eye movements, much like in natural viewing, which is fundamentally different from the traditional neurophysiological paradigms in which neurons respond to objects that are being switched on and off. A technical complication here is that “stimulus onset” is not controlled by the experimenter, but by the subject's eye movements, which means that the neural responses are timed by onset and offset of fixation. Thus, the phases of visibility and occlusion of individual objects, which are programmed by the experimenter, need to be related to the recorded eye movements. But this complication is greatly outweighed by the opportunity to study neuronal activity under quasi natural viewing conditions which makes this an enormously powerful paradigm.
Studying V4 neurons with this paradigm Zhu et al. (2020) indeed found a “response” to the invisible objects in the mean firing rate, corresponding to the predicted top-down activation of grouping cells (their Figure 10 which shows the averaged responses of 87 V4 neurons). But the authors rejected this result as evidence for grouping cells in V4, suggesting an alternative explanation for the “responses” to invisible objects, because of another result that was not consistent with the predictions: While top-down attention and saccade planning clearly produced response enhancement for visible objects, they did not so for occluded objects (Figure 15).
Figure 15. Attending and saccading to invisible objects. (A) Theory of object pointers. Gray bars with ovals represent receptive fields of feature cells in retinal space. G, grouping cell layer, SH shifter circuit, OP object pointer cells. Presentation of an object excites a number of feature cells and a grouping cell, and, through SH, an object pointer cell. OP cells sustain activity once excited. Top panel. Two objects (squares) have activated two OPs before the left-hand object was occluded by another object (blue outline). Attention on right-hand object (yellow asterisk), which is currently fixated, is enhancing corresponding OP. Middle panel. Planning of a saccade to left-hand object re-allocates attention, enhancing left-hand OP whose activity flows down to G1 (red arrow), and a signal is recorded (microelectrode symbol) even though the object is no longer visible. Bottom panel. The saccade has moved the receptive fields, and SH has compensated for the movement by re-routing the connections to G3 and G5, as indicated by yellow arrows, thus keeping left-hand OP connected to the feature cells of left-hand object. (B) The mean time course of activity recorded from 87 V4 neurons at the end of a fixation period; zero on abscissa marks time of saccade. Note that new visual input does not affect responses until ~50 ms, the latency of V4. Red and brown traces, responses to visible objects; blue and cyan traces, responses to occluded objects; solid lines, when attended; dashed lines, when ignored; red and blue, when goal of next saccade. Responses were enhanced by attention (solid vs. dashed), and further enhanced when object was goal of planned saccade (red vs. brown), but only for visible objects. Had the recordings been from a G cell, enhancements would also be found for occluded objects.
Neurophysiology can be hard to understand if one just looks at what the various individual neurons do; only a theory can relate the neural signals to visual experience or the performance of a vision algorithm. Figure 15A illustrates the prediction of the theory when fixation is on one object (square marked by yellow asterisk) and a saccade is planned to another object (dashed square) that is momentarily occluded by a larger object (blue outline rectangle). According to the theory, there are three layers of cells, the feature cells with receptive fields in retinal space (ovals on gray bars), a grouping cell layer G with fixed connections to the feature cells, and a number of object pointer cells OP that are connected with grouping cells through the shifter circuit SH.
The top panel of Figure 15A illustrates the fixation before the saccade: top-down attention enhances the OP cell that is momentarily connected to grouping cell G3. Grouping cell G1 (assumed to be the recorded cell) is not active because the object in its receptive field is occluded.
When the saccade to the other object is planned, as shown in the middle panel, top-down attention moves to the OP cell that is momentarily connected with G1, and the OP activity flows down to G1 (red arrow). This is the predicted activity that will be recorded despite absence of afference from the retina.
And when the saccade is executed, as shown in the bottom panel, SH reroutes the connections to G3 and G5 as indicated by yellow arrows. Thus, while the left-hand object activates other feature cells after the saccade, it is again connected to the left-hand OP cell.
Figure 15B shows the time course of the mean firing rates at the end of a fixation period, that is, at the moment when the brain initiates a new saccade. The curves represent the activity from before the saccade until 50 ms after the saccade. Because 50 ms is the latency of visual responses in V4, visual information from the next fixation did not influence this activity.
The top three curves show the responses to visible objects, red line for responses when the object in the receptive field was the goal of the next saccade, and brown lines when another object was the goal; solid lines, when the object was a target, and dashed line when it was a distracter. These curves show that the responses were enhanced by attention (solid brown vs. dashed brown) and further enhanced when the attended object was the goal of the next saccade (solid red vs. solid brown). But planning a saccade to an occluded object did not produce the activity predicted by the red arrow in Figure 15A (blue vs. cyan curves) and occluded targets were not represented by enhanced activity compared to occluded distracters (solid cyan vs. dashed cyan). This means that the recorded neurons were activated by visual afference, but not by top-down activity from object pointer cells.
To conclude this section, previous experiments had shown that border ownership signals in neurons of V1/V2 persist after the object that produced these signals has been removed (Figure 10), and that they even persist across a half second of display of a blank field that completely silences the activity of these neurons (O'Herron and von der Heydt, 2009, their Figure 7). These findings suggests that border-ownership selective neurons must be modulated by an external signal, by activity that we do not see in V1/V2. And the results of the new experiment, summarized in Figure 15B, show that this signal does not come from V4.
Since figure-ground organization was discovered by the Gestalt psychologists it has stimulated theories about the underlying brain activity unlike few other phenomena in perception, and the interest in modeling it has grown since neurophysiologists discovered neural activity related to illusory contours (von der Heydt et al., 1984), figure ground segregation (Lamme, 1995), object-based attention (Roelfsema et al., 1998), and border ownership (Zhou et al., 2000).
Among the various models of perceptual organization that have been proposed (Grossberg and Mingolla, 1985; Zhaoping, 2005; Jehee et al., 2007; Kogo et al., 2010; Jeurissen et al., 2016), the grouping cell model discussed here is distinct in that it makes the highly specific prediction that pairs of border-ownership selective neurons with consistent side-of-figure preferences, when stimulated by a common object, show spiking synchrony. And experiments have shown exactly this. Other neural models do not predict synchrony because the neurons representing the distributed features of an object are not supposed to receive input from common spike trains. Only the models by Jehee et al. (2007) and Jeurissen et al. (2016) propose neurons with receptive fields large enough to encompass objects. However, the coarse-to-fine processing in their model is relayed through a cascade of neurons down through the hierarchy of visual areas from TEO to V1, and the relays do not preserve spike timing.
Models that rely on lateral signal propagation (Grossberg and Mingolla, 1985; Zhaoping, 2005; Kogo et al., 2010) are not physiologically plausible because the conduction velocity of horizontal fibers in cortex is too slow. Based on published conduction velocity data, Craft et al. (2007) estimated that lateral propagation would delay the border ownership signal for the 8 deg square by at least 70 ms relative to the edge responses, in addition to processing delays, whereas only 30 ms has been found. Sugihara et al. (2011) calculated the latencies of border ownership signals for two conditions in which the relevant context information was located at different distances from the receptive field and compared the latency difference with the difference predicted from horizontal signal propagation. The prediction was based on the increase in cortical distance computed from mapping of the actual test stimuli onto the cortex and the known conduction velocities of horizontal fibers. The actual latencies increased with cortical distance, but much less than predicted by the horizontal propagation hypothesis. Probability calculations showed that an explanation of the context influence by lateral signal propagation is highly unlikely.
In contrast, mechanisms involving back projections from other extrastriate areas or subcortical structures (Craft et al., 2007; Jehee et al., 2007; Jeurissen et al., 2016) are plausible because they use white-matter fibers which are an order of magnitude faster than horizontal fibers. Context information for the 8 deg square that might take over 70 ms if conducted through horizontal fibers in V2 would take perhaps 10 ms if sent up to V4 and back.
Kogo et al. (2010), who base their model on perceptual observations of illusory figures akin to the Kanizsa triangle, state that “most of the many attempts to mimic the Kanizsa illusory phenomenon in neurocomputational models have been inspired by the borderline-completion scheme driven by the collinear alignment of the contours of the Pac Man shapes”—which is not true. In fact, all models since the mid 1980ies were inspired by the discovery of illusory contour responses in the visual cortex which included responses to stimuli that do not entail collinear alignment. When I began recording from area V2, I was surprised to find orientation selective neurons that responded to patterns consisting of lines orthogonal to their preferred orientation: lines that terminated along a virtual line through the receptive field at the preferred orientation (von der Heydt et al., 1984). Neurons that were sharply selective for a certain orientation responded vigorously to stimuli that had no line or edge of that orientation at all, and no energy for that orientation in the Fourier spectrum (von der Heydt and Peterhans, 1989). These stimuli also produce illusory contours in perception. A striking example of an illusory contour that is not a collinear completion of given features is the Ehrenstein illusion, in which a circular contour is produced by radial lines (Kogo et al. do not mention this illusion).
Also architects of artificial neural nets that do not claim physiological plausibility should take note that about 30% of the orientation selective cells in monkey V2 respond to a virtual line defined by line terminations as if it were a real line. V2 is a large area (in humans V2 is even larger than V1). Thus, 30% means a huge number of cells. There must be an advantage of having so many cells capable of signaling illusory contours. These cells seem to respond simply to the line of discontinuity, perhaps because it is indicative of an occluding contour. Their responses grow with the number of aligned terminations, but they do not require evidence for border ownership—the stimulus can be symmetric about the contour and does not need to have a closed contour or something that suggests a figure. V2 is an early stage in the process, and those responses appear with short latency.
Heitger et al. (1998) modeled the illusory contour neurons by combining two inputs, one that detects edges, and a second input that integrates termination features along the receptive field axis. They suggested that termination features are signaled by end-stopped cells (Heitger et al., 1992). Indeed, the neural illusory-contour responses had opened eyes for an important role of orthogonal features in the definition of contours. This model reproduced all the neural illusory contour responses and also produced the circular shapes of the Ehrenstein illusion. It achieved all this with a semi-local image operator.
As explained above, Craft et al. (2007) showed that integrating co-circular edge signals alone is not sufficient to reproduce the neural border ownership signals in configurations of partially occluding figures, and therefore included integration of T-junction signals, and von der Heydt and Zhang (2018) explicitly showed the influence of contextual T-junctions, L-junctions, and orthogonal edges in modulating the neural responses. Craft et al. adopted the two-input scheme of Heitger et al. (1992) and showed that it explains the data on neural responses to geometrical figures completely. I think there are good reasons to expect that an image-computable model that combines integration of co-circular edge signals as in Hu et al. (2019a) with integration of end-stopped signals as in Heitger et al. would improve the consistency of border ownership assignment, perhaps from the 69% score of Hu et al. to over 90%, as found in some neurons.
The notion that border ownership coding appears at low levels of the hierarchy and early in the process runs counter to current trends in machine vision. In convolutional nets one expects such context-sensitive coding only at higher levels, and late in the process. In fact, Hu et al. (2019b) found that the convolutional nets that represent figure-ground organization show it only at the higher levels.
As said, area V4 is but one of many candidate regions in the search for grouping cells. In a way, the negative result in this visual area makes sense because representing objectness may require comprehensive action at multiple cortical levels. In fact, border ownership modulates responses in V1, V2, and V4, and shape selectivity of neurons in infero-temporal cortex also depends on border ownership. And for effective object-based attention, grouping cells should target neurons not only in V2, but at various levels of the visual object processing pathways in parallel, including V1, V2, V4, and IT. Indeed, recordings from different levels of the visual pathways have shown that attentional modulation tends to get stronger at higher levels, suggesting that the modulatory effects accumulate from stage to stage. Thus, grouping cells might not be found within the feature processing visual pathways, but rather in a structure “on the side” as sketched in Figure 8 (a similar architecture was proposed by Wolfe and Horowitz, 2004 for guidance in visual search, suggesting that “the ‘guiding representation' … is not, itself, part of the pathway”). This idea also explains the finding that border ownership signals in V4 have similar or even shorter latencies than those of V2 (Bushnell et al., 2011; Franken and Reynolds, 2021).
Moreover, for dealing with objects, grouping cells should also receive and target neurons in other modalities like touch, proprioception, audition, taste, and smell. The model sketched in Figure 8 could be extended across modalities. The feed forward pathway activates grouping cells which provides handles for selective attention: The sound of a dropping coin directs visual attention to the site where the coin fell. Through back projections, grouping cells facilitate feature signals for the computation of object attributes: Say, an object has been identified visually. When the hand grasps the object, grouping cells selectively facilitate feature signals from skin and tendon receptors informing about haptic qualities and hand conformation, signals from which further processing may compute shape, weight, and other attributes of the object.
An important function of grouping cells and object pointers is in representing the layout of objects in a scene for reaching. When we reach for a pawn on a chess board, the hand easily grasps the pawn without knocking over other pieces on the board. This cannot be based on object recognition—all pawns look the same. Also selectively attending just to the target would not be successful. Grouping cells indicate object locations in retinal space, and object pointers track their locations in real space thus representing the layout of the objects.
Considering all these aspects it becomes clear that object representation needs a brain structure bigger than area V4. It must be large enough to be able to coordinate spatial information coming in through senses as diverse as vision, audition, and touch. Auditory space sense depends on head orientation, and so does vision, with the extra complication of eye movements, and tactile perception of 3D objects involves hand conformation. To combine these requires massive computations in real time. And we are looking for a structure that has connections to a range of cortical areas.
The pulvinar might be able to meet these requirements. The pulvinar is enlarged in primates which use hands for grasping and handling objects, compared to rats and cats which lack hands. It synchronizes activity between interconnected cortical areas according to attentional allocation (Saalmann et al., 2012). In humans, damage to the pulvinar often produces neglect (Ohye, 2002; Furman, 2014) which suggests a deficiency of grouping cells because grouping cells provide objects with “handles” for selective attention, and without these handles the system may not be able to disentangle objects in the visual representations even though the feature representations are intact.
The deficits expected from a loss of grouping cells are subtle; problems with visual attention to objects, visual guidance of grasping movements and saccades in cluttered scenes, e.g., situations where objects are partially occluded. Also deficits in object permanence and in maintaining object identity across object movements and saccades are to be expected.
Clearly, using object permanence as the criterion in the search for grouping cells is but one of many possible strategies. But it seems to me that permanence is the most decisive evidence for object-based perceptual organization. Grouping cells are a hypothesis of modeling, and a computational model is merely an existence proof. It shows that an algorithm exists that can perform a given task. Whether such cells really exist we do not know, they are imaginary. But persistence of border ownership signals is real.
The author confirms being the sole contributor of this work and has approved it for publication.
I wish to thank Ernst Niebur for complementing my neurophysiology with computational neuroscience; Fangtu T. Qiu for creating a powerful and versatile system for visual stimulus generation, behavioral control, and recording; and Ofelia Garalde who as an animal lab technician contributed a lot to the experimental success of the 14 neurophysiological studies reviewed here.
The author declares that the research was conducted in the absence of any commercial or financial relationships that could be construed as a potential conflict of interest.
All claims expressed in this article are solely those of the authors and do not necessarily represent those of their affiliated organizations, or those of the publisher, the editors and the reviewers. Any product that may be evaluated in this article, or claim that may be made by its manufacturer, is not guaranteed or endorsed by the publisher.
1. ^Slogan of the Transport Security Agency.
Baylis, G. C., and Driver, J. (2001). Shape-coding in IT cells generalizes over contrast and mirror reversal, but not figure-ground reversal. Nat. Neurosci. 4, 937–942. doi: 10.1038/nn0901-937
Brincat, S. L., and Connor, C. E. (2006). Dynamic shape synthesis in posterior inferotemporal cortex. Neuron 49, 17–24. doi: 10.1016/j.neuron.2005.11.026
Bullier, J., Hupe, J. M., James, A. C., and Girard, P. (2001). the role of feedback connections in shaping the responses of visual cortical neurons. Prog. Brain Res. 134, 193–204. doi: 10.1016/S0079-6123(01)34014-1
Bushnell, B. N., Harding, P. J., Kosai, Y., and Pasupathy, A. (2011). Partial occlusion modulates contour-based shape encoding in primate area V4. J. Neurosci. 31, 4012–4024. doi: 10.1523/JNEUROSCI.4766-10.2011
Craft, E., Schütze, H., Niebur, E., and von der Heydt, R. (2007). A neural model of figure-ground organization. J. Neurophysiol. 97, 4310–4326. doi: 10.1152/jn.00203.2007
Franken, T., and Reynolds, J. (2021). Columnar processing of border ownership in primate visual cortex. Elife 10, e72573. doi: 10.7554/eLife.72573.sa2
Friedman, H. S., Zhou, H., and von der Heydt, R. (2003). The coding of uniform color figures in monkey visual cortex. J. Physiol. 548, 593–613. doi: 10.1113/jphysiol.2002.033555
Furman, M. (2014). “Chapter 19 - visual network,” in Neuronal Networks in Brain Function, CNS Disorders, and Therapeutics, eds L. Carl Faingold, and H. Blumenfeld (San Diego, CA: Academic Press), 247–259.
Gillary, G., von der Heydt, R., and Niebur, E. (2017). Short-term depression and transient memory in sensory cortex. J. Comput. Neurosci. 43, 273–294. doi: 10.1007/s10827-017-0662-8
Grossberg, S., and Mingolla, E. (1985). Neural dynamics of form perception: boundary completion, illusory figures, and neon color spreading. Psychol. Rev. 92, 173–211. doi: 10.1037/0033-295X.92.2.173
Heitger, F., Rosenthaler, L., Von Der Heydt, R., Peterhans, E., and Kübler, O. (1992). Simulation of neural contour mechanisms: from simple to end-stopped cells. Vis. Res. 32, 963–981. doi: 10.1016/0042-6989(92)90039-L
Heitger, F., von der Heydt, R., Peterhans, E., Rosenthaler, L., and Kübler, O. (1998). Simulation of neural contour mechanisms: representing anomalous contours. Image Vis. Comp. Comput. Psychophys. Stud. Early Vis. 16, 407–421. doi: 10.1016/S0262-8856(97)00083-8
Hu, B., Khan, S., Niebur, E., and Tripp, B. (2019b). “Figure-ground representation in deep neural networks,” in 2019 53rd Annual Conference on Information Sciences and Systems (CISS) (Baltimore, MD), 1–6. doi: 10.1109/CISS.2019.8693039
Hu, B., von der Heydt, R., and Niebur, E. (2019a). Figure-ground organization in natural scenes: performance of a recurrent neural model compared with neurons of area V2. ENeuro 6, ENEURO.0479-18. doi: 10.1523/ENEURO.0479-18.2019
Intriligator, J., and Cavanagh, P. (2001). The spatial resolution of visual attention. Cognit. Psychol 43, 171–216. doi: 10.1006/cogp.2001.0755
Jehee, J. F., Lamme, V. A., and Roelfsema, P. R. (2007). Boundary assignment in a recurrent network architecture. Vis. Res. 47, 1153–1165. doi: 10.1016/j.visres.2006.12.018
Jeurissen, D., Self, M. W., and Roelfsema, P. R. (2016). Serial grouping of 2D-image regions with object-based attention in humans. Elife 5, e14320. doi: 10.7554/eLife.14320
Kogo, N., Strecha, C., Van Gool, L., and Wagemans, J. (2010). Surface construction by a 2-D differentiation-integration process: a neurocomputational model for perceived border ownership, depth, and lightness in Kanizsa Figures. Psychol. Rev. 117, 406–439. doi: 10.1037/a0019076
Lamme, V. A. F. (1995). The neurophysiology of figure-ground segregation in primary visual cortex. J. Neurosci. 15, 1605–1615. doi: 10.1523/JNEUROSCI.15-02-01605.1995
Martin, A. B., and von der Heydt, R. (2015). Spike synchrony reveals emergence of proto-objects in visual cortex. J. Neurosci. 35, 6860–6870. doi: 10.1523/JNEUROSCI.3590-14.2015
Martin, D., Fowlkes, C., Tal, D., and Malik, J. (2001). “A database of human segmented natural images and its application to evaluating segmentation algorithms and measuring ecological statistics,” in Proceedings of Eighth IEEE International Conference on Computer Vision (Vancouver, BC: IEEE), 416–423. doi: 10.1109/ICCV.2001.937655
Mihalas, S., Dong, Y., von der Heydt, R., and Niebur, E. (2011). Mechanisms of perceptual organization provide auto-zoom and auto-localization for attention to objects. Proc. Nat. Acad. Sci. U. S. A. 108, 7583–7588. doi: 10.1073/pnas.1014655108
Nakayama, K., Shimojo, S., and Silverman, G. H. (1989). Stereoscopic depth: its relation to image segmentation, grouping, and the recognition of occluded objects. Perception 18, 55–68. doi: 10.1068/p180055
O'Herron, P., and von der Heydt, R. (2009). Short-term memory for figure-ground organization in the visual cortex. Neuron 61, 801–809. doi: 10.1016/j.neuron.2009.01.014
O'Herron, P., and von der Heydt, R. (2011). Representation of object continuity in the visual cortex. J. Vis. 11, 12. doi: 10.1167/11.2.12
O'Herron, P., and von der Heydt, R. (2013). Remapping of border ownership in the visual cortex. J. Neurosci. 33, 1964–1974. doi: 10.1523/JNEUROSCI.2797-12.2013
Ohye, C. (2002). “Thalamus and thalamic damage,” in Encyclopedia of the Human Brain, eds V. S. Ramachandran (New York, NY: Academic Press), 575–597.
Qiu, F. T., Sugihara, T., and von der Heydt, R. (2007). Figure-ground mechanisms provide structure for selective attention. Nat. Neurosci. 10, 1492–1499. doi: 10.1038/nn1989
Qiu, F. T., and von der Heydt, R. (2005). Figure and ground in the visual cortex: v2 combines stereoscopic cues with gestalt rules. Neuron 47, 155–166. doi: 10.1016/j.neuron.2005.05.028
Qiu, F. T., and von der Heydt, R. (2007). Neural representation of transparent overlay. Nat. Neurosci. 10, 283–284. doi: 10.1038/nn1853
Roelfsema, P. R., Lamme, V. A., and Spekreijse, H. (1998). Object-based attention in the primary visual cortex of the macaque monkey. Nature. 395, 376–381. doi: 10.1038/26475
Saalmann, Y. B., Pinsk, M. A., Wang, L., Li, X., and Kastner, S. (2012). The pulvinar regulates information transmission between cortical areas based on attention demands. Science 337, 753–756. doi: 10.1126/science.1223082
Smith, M. A., and Kohn, A. (2008). Spatial and temporal scales of neuronal correlation in primary visual cortex. J. Neurosci. 28, 12591–12603. doi: 10.1523/JNEUROSCI.2929-08.2008
Sugihara, T., Qiu, F. T., and von der Heydt, R. (2011). The speed of context integration in the visual cortex. J. Neurophysiol. 106:374–385. doi: 10.1152/jn.00928.2010
Ungerleider, L. G., Galkin, T. W., Desimone, R., and Gattass, R. (2008). Cortical connections of area V4 in the Macaque. Cereb. Cortex 18, 477–499. doi: 10.1093/cercor/bhm061
Ungerleider, L. G., and Mishkin, M. (1982). “Two cortical visual systems,” in Analysis of Visual Behavior, eds D. J. Ingle, M. A. Goodale, and R. J. W. Mansfield (Cambridge: MIT Press), 549–586.
von der Heydt, R. (2015). Figure-ground organization and the emergence of proto-objects in the visual cortex. Front. Psychol. 6, 1695. doi: 10.3389/fpsyg.2015.01695
von der Heydt, R., Macuda, T. J., and Qiu, F. T. (2005). Border-ownership dependent tilt aftereffect. J. Opt. Soc. Am. Opt. A. 22, 2222–2229. doi: 10.1364/JOSAA.22.002222
von der Heydt, R., and Peterhans, E. (1989). Mechanisms of contour perception in monkey visual cortex. I. Lines of pattern discontinuity. J. Neurosci. 9, 1731–1748. doi: 10.1523/JNEUROSCI.09-05-01731.1989
von der Heydt, R., Peterhans, E., and Baumgartner, G. (1984). Illusory contours and cortical neuron responses. Science 224, 1260–1262. doi: 10.1126/science.6539501
von der Heydt, R., Peterhans, E., and Dürsteler, M. R. (1992). Periodic-pattern-selective cells in monkey visual cortex. J. Neurosci. 12, 1416–1434. doi: 10.1523/JNEUROSCI.12-04-01416.1992
von der Heydt, R., Qiu, F. T., and He, Z. J. (2003). Neural mechanisms in border ownership assignment: motion parallax and gestalt cues. J. Vis. 3/9, 666. doi: 10.1167/3.9.666
von der Heydt, R., and Zhang, N. R. (2018). Figure and ground: how the visual cortex integrates local cues for global organization. J. Neurophysiol. 120, 3085–3098. doi: 10.1152/jn.00125.2018
von Holst, E., and Mittelstaedt, H. (1950). Das Reafferenzprinzip. Wechselwirkungen Zwischen Zentralnervensystem Und Peripherie. Naturwissenschaften 37, 464–476. doi: 10.1007/BF00622503
Wagatsuma, N., von der Heydt, R., and Niebur, E. (2016). Spike synchrony generated by modulatory common input through NMDA-type synapses. J. Neurophysiol. 116, 1418–1433. doi: 10.1152/jn.01142.2015
Williford, J. R., and von der Heydt, R. (2016a). Figure-ground organization in visual cortex for natural scenes. ENeuro 3, ENEURO.0127–0116. doi: 10.1523/ENEURO.0127-16.2016
Williford, J. R., and von der Heydt, R. (2016b). Data Associated with Publication ‘Figure-Ground Organization in Visual Cortex for Natural Scenes,' Version 1. Johns Hopkins University Data Archive. doi: 10.7281/T1C8276W
Wolfe, J. M., and Horowitz, T. S. (2004). What attributes guide the deployment of visual attention and how do they do it? Nat. Rev. Neurosci. 5, 495–501. doi: 10.1038/nrn1411
Zhang, N. R., and von der Heydt, R. (2010). Analysis of the context integration mechanisms underlying figure-ground organization in the visual cortex. J. Neurosci. 30, 6482–6496. doi: 10.1523/JNEUROSCI.5168-09.2010
Zhaoping, L. (2005). Border ownership from intracortical interactions in visual area V2. Neuron 47, 147–153. doi: 10.1016/j.neuron.2005.04.005
Zhou, H., Friedman, H. S., and von der Heydt, R. (2000). Coding of border ownership in monkey visual cortex. J. Neurosci. 20, 6594–6611. doi: 10.1523/JNEUROSCI.20-17-06594.2000
Keywords: visual cortex, figure ground organization, neural mechanism, object individuation, object permanence, selective attention, spiking synchrony, computational model
Citation: von der Heydt R (2023) Visual cortical processing—From image to object representation. Front. Comput. Sci. 5:1136987. doi: 10.3389/fcomp.2023.1136987
Received: 03 January 2023; Accepted: 30 May 2023;
Published: 21 June 2023.
Edited by:
Mary Peterson, University of Arizona, United StatesReviewed by:
Jack Gallant, University of California, Berkeley, United StatesCopyright © 2023 von der Heydt. This is an open-access article distributed under the terms of the Creative Commons Attribution License (CC BY). The use, distribution or reproduction in other forums is permitted, provided the original author(s) and the copyright owner(s) are credited and that the original publication in this journal is cited, in accordance with accepted academic practice. No use, distribution or reproduction is permitted which does not comply with these terms.
*Correspondence: Rüdiger von der Heydt, cnVkaWdlcjhAZ21haWwuY29t
Disclaimer: All claims expressed in this article are solely those of the authors and do not necessarily represent those of their affiliated organizations, or those of the publisher, the editors and the reviewers. Any product that may be evaluated in this article or claim that may be made by its manufacturer is not guaranteed or endorsed by the publisher.
Research integrity at Frontiers
Learn more about the work of our research integrity team to safeguard the quality of each article we publish.