- 1Department of Computer Science, Humantech Institute, University of Applied Sciences and Arts Western Switzerland, Fribourg, Switzerland
- 2School of Management, University of Applied Sciences and Arts Western Switzerland, Fribourg, Switzerland
- 3Department of Computer Science, Human-IST Institute, University of Fribourg, Fribourg, Switzerland
Introduction: The use of multiple interfaces may improve the perception of a stronger relationship between a conversational virtual coach and older adults. The purpose of this paper is to show the effect of output combinations [single-interface (chatbot, tangible coach), multi-interface (assignment, redundant-complementary)] of two distinct conversational agent interfaces (chatbot and tangible coach) on the eCoach-user relationship (closeness, commitment, complementarity) and the older adults' feeling of social presence of the eCoach.
Methods: Our study was conducted with two different study settings: an online web survey and a face to face experiment.
Results: Our online study with 59 seniors shows that the output modes in multi-interface redundant-complementary manner significantly improves the eCoach-user relationship and social presence of the eCoach compared to only using single-interfaces outputs. Whereas in our face to face experiment with 15 seniors, significant results were found only in terms of higher social presence of multi-interface redundant complementary manner compared to chatbot only.
Discussion: We also investigated the effect of each study design on our results, using both quantitative and qualitative methods.
1. Introduction
According to a recent systematic review on virtual coaches for older adults' wellbeing, conversational agents (CAs) have been increasingly used to deliver health interventions since 2016 (El Kamali et al., 2020a). In fact, since language is the primary modality used to establish human relationships, and with the growing capabilities of voice services and natural language understanding, CAs may be appealing as an intervention interface for e-coaching. CAs for coaching older adults can be embodied in different interfaces, such as a desktop application (Bickmore et al., 2005), robots (Bickmore et al., 2013; Black et al., 2014), smart speakers (Blusi et al., 2018), or virtual avatars (Callejas et al., 2014). Each of these interfaces can have different interaction modalities (image, speech, text, buttons, lights, etc.) to coach the user. Because of the variety of interfaces and modalities, several research questions arise for understanding how to combine them into an optimal interaction experience. For instance, combining different interfaces (using a conversational agent in a phone and an embodied/tangible conversational agent in a physical body) might improve the ecoach-user relationship and social presence of the ecoach.
Nestore is a virtual coach (or eCoach) designed for older adults' wellbeing in five different domains, namely, nutritional, physical, social, cognitive, and mental wellbeing (Andreoni and Mambretti, 2021). Nestore is designed to be a coach and a companion through its two conversational interfaces as follows:
• A chatbot, which is a text-based messaging app integrated in a mobile app. The chatbot is referred as a virtual agent since it is integrated in a mobile app and the user can interact only by text messaging.
• A tangible coach (TC) which is a physical device with vocal and tangible capabilities (El Kamali et al., 2020b). The tangible coach possesses both vocal and tangible capabilities. Through speech-to-text and text-to-speech services, it can listen to the user and respond verbally. Additionally, users can physically interact with the tangible coach, altering its status (such as TC asleep vs. TC awake) by manipulating its physical orientation (face-down or face-up). The tangible coach is also referred to as an embodied conversational agent (ECA) since it is embodied inside a dedicated physical object (other than a multi-purpose phone).
Previous study (Sidner et al., 2018a) has shown that isolated older adults appreciated more an embodied agent in a physical device rather than a virtual agent in the phone to provide company. However, the authors added that the virtual agent in the phone is essential, since it can be used outside the home to accompany them in different contexts. Regarding modalities (e.g., text and speech), (El Kamali et al., 2020a) concluded from their review that some implementations of behavior change techniques that help in the coaching interventions (e.g., calendar for action planning and charts for self-reflection, which rely on visual channels) may be easier to use in the smartphone or in web interfaces, while encouragement messages could be more effective through a vocal assistant, i.e., an agent embodied in a physical device.
In fact, some previous studies explored the factors influencing the choice among different modalities such as speech, text, and gestures. Factors such as efficiency, accuracy, privacy and security (Himmelsbach et al., 2015), user characteristics (Dumas et al., 2009; Ghosh and Joshi, 2013; Schüssel et al., 2013; Schaffer et al., 2015), attitudes toward technology, and quality perceptions and personality factors (Wechsung, 2014) have been shown to impact modality selection. Jian et al. (2013) demonstrated the potential of multimodal interaction (interacting with multiple modalities such as text and speech) for older adults. In their investigation, multimodal touch and speech contact outperformed unimodal interaction in terms of efficiency, effectiveness, and preferences. Hence, using multiple interfaces might also affect the user experience toward older adults.
Multimodal interaction frameworks, such as CASE (Dumas et al., 2009), CARE (Nigay and Coutaz, 1997), or Vernier and Nigay's (Vernier and Nigay, 2000) that defined combinations on different aspects such as the semantic aspect (combination based on meaning), can help interaction designers to investigate different ways of combining modalities. For instance, one can combine these different interfaces in a way that the same output message can be sent to all the interfaces (redundancy) or the output can be assigned to the most suitable interface (assignment) or the two interfaces can complement the information with each other in order to send one meaning to the user (complementary). In a previous study, we integrated CASE properties in the interaction design of the Nestore eCoach interfaces and compared the user experience of different output modes. Output modes refer to different way of using interfaces: using interfaces alone or combining interfaces together. The results showed that users liked receiving information from the eCoach from both interfaces (chatbot and tangible coach) together in a complementary manner, where one interface complement the other in terms of output message (El Kamali et al., 2020c). The study was conducted via an online survey with videotaped interaction scenarios due to COVID-19 pandemic.
In this article, we refined our modes of outputs investigating also the redundant complementary mode. In fact, we defined an output mode model to compare single-interface, where only one interface is used, and multi-interface, where multiple interfaces are combined together. In terms of multi-interface, we used the assignment case from the CARE model where both interfaces exist, but each output message is assigned to one interface. We also took inspiration from the study by Vernier and Nigay (2000) who defined the redundancy and complementary of the semantic combination aspect, which means that interfaces are combined in way that it gives users more information in terms of meaning and information richness. Such combination can help enriching the user experience as it maximizes the benefits of each interface. We used the chatbot and tangible coach from the Nestore eCoach and integrated them into the output mode model. Then, we studied the perception of the coach-user relationship (commitment, closeness, complementarity; Jowett and Ntoumanis, 2004) and the social presence of the virtual coach with respect to these modes based on one scenario. Good relationships are vital and have been proven to influence health outcomes in human relationships (Chipidza et al., 2015). Moreover, it is shown that the combination of modalities is effective on closeness with the ECA (Loveys et al., 2020). Hence, in this study, we would like to investigate the effect of different output modes of interfaces on older adults' relationship and social presence toward an eCoach. We defined five research questions as follows:
• RQ1: What is the effect of output modes on user's perceptions toward the user-virtual coach relationship?
• RQ2: What is the effect of output modes on user's perception of the virtual coach's social presence?
• RQ3: Which output mode improve the perception of the agent as a coach?
• RQ4: Which output mode allow the agent to provide users with companionship?
• RQ5: Which output mode is preferred by users?
Due to COVID, the study was conducted at the beginning online and then replicated via a face to face experiment. Section 2 explains the related work. Section 3 present the methods of both study settings (online and face to face). Section 3 presents the results of the 2 studies. Section 4 discusses the results and presents some limitations. Finally, we conclude the article with some ideas and future studies.
2. Related work
2.1. Multimodality in an eCoach
The effectiveness of eCoaches in supporting older adults' wellbeing and establishing meaningful relationships depends on various factors, including the modality of interaction. El Kamali et al. (2020a) investigated how an eCoach for older adults' wellbeing was defined in the literature. They concluded that an eCoach is not only a coach for wellbeing but also a companion. They also suggested that combining multimodal interfaces of a an eCoach could play a role in the relationship with older adults. To deepen the understanding of this relationship, Jowett and Ntoumanis (2004) explored the dynamics between a coach and a user using three key constructs: closeness, complementarity, and commitment. These constructs highlight how the modality of interaction can influence the emotional closeness between the user and the eCoach, the complementary roles they play, and the level of commitment to the coaching process. Shin and Choo (2011) further emphasized that the presence of social engagement enhances the perceived utility and positive attitudes toward robotic interactions. Social presence, akin to companionship, involves the sensation of being accompanied by the eCoach (Lombard and Ditton, 1997). Recent studies have also delved into the effects of different design features on the quality of relationships and social perceptions in the context of ECAs. Loveys et al. (2020) conducted a systematic review, concluding that an embodied agent with voice capability significantly improves rapport between older adults and ECAs in healthcare settings. Furthermore, combining multiple modalities in ECAs has demonstrated a positive impact on establishing a sense of closeness with the system.
2.2. Combination of multiple multimodal conversational agents
While research has underscored the significance of employing embodied conversational agents alongside virtual agents (chatbots) for interactive experiences (Steuer, 1992; Sidner et al., 2018b), empirical investigations supporting these claims have been limited. Although the literature suggests a user preference for embodied conversational agents over virtual agents, the potential benefits of combining both modalities have been consistently highlighted. Bartneck (2002) discovered the impact of physical embodiment on social presence. Participants interacting with an embodied robot exhibited higher engagement and rated the physically present characteristic as more socially present, demonstrating the potential influence of sensory richness on social presence perception. This observation aligns with primary findings (Merrill et al., 2022) that indicate increased perceived usefulness of socially present embodied AI companions.
Regarding the combination of two interfaces, pioneering work such as the ITAKO system (Imai et al., 1999) introduced the concept of “agent migration.” This concept enabled a personal agent for transition from a mobile device to a physical robot, facilitating continuous interaction. Tejwani (2020) further advanced this notion with a Migratable AI system, enabling seamless transitions across interfaces while maintaining context and identity. Their findings emphasized the positive impact of identity and information migration on trust, competence, and social presence.
Ogawa and Ono's study (Ogawa and Ono, 2005) focused on migrating from a wearable computer to a desk map. Users interact with the agent through daily interaction. When the user leaves the house, the agent moves to their wearable computer and follows them to their destination. The test results revealed that the participants' attachment to the media, as well as their relationship, was carried on through the media by agent migration. The study by Gomes et al. (2014) involved migrating an agent through the physical robot and its virtual mobile applications. Their research looked into the user experience of agent migration and how natural the process was for users. Cuba (2010) studied the agent migration by counting the number of agents the user perceived while interacting with the iCAT agent on different platforms. Sinoo et al. (2018) showed that the more children perceived the robot and its avatar as the same agency, the stronger is their friendship with the avatar and the higher is the motivation to play with and the usability. Luria et al. (2019) tested the user experience toward combining two social presences in one body (co-embody) or migrating one social presence from one body to another (re-embody). Re-embodying was observed as more comfortable by users that created more seamless and efficient experiences. To sum up, migrating information and social presence across embodiments are essential to increase the user experience with virtual agents.
2.3. Multimodal interaction frameworks
Several frameworks have addressed the issue of relationships between modalities. The CARE model (Nigay and Coutaz, 1997) focuses on the interaction between the user and the machine. The model has four properties, namely, complementary, assignment, redundancy, and equivalence. When multiple complementing modalities are required to grasp the intended meaning, complementary is used. In the assignment case, only one modality can lead to the required meaning. Redundancy denotes a number of modalities that, even when used concurrently, can be used independently to achieve the desired meaning. Finally, equivalence entails a variety of modalities, all of which can lead to the desired meaning, but only one is used at a time (e.g., speech or keyboard can be used to write a text).
Vernier and Nigay (2000) propose a framework for analyzing output multimodal user interfaces. They identify a variety of modality combinations and their characteristics. This framework aids in the selection of the most appropriate output combinations for effective multimodal presentations. The combination space includes the CARE and the discovery of new attributes. The first axis is comprised of four components, namely, time, space, interaction language, and semantics. The second axis spans a set of combination schema derived from the five Allen relationships (possible relations between intervals such as equals, overlaps, touches, starts, and finishes) (James, 1983) in order to give a mechanism of combining several modalities into a composite modality. The semantic combination in which the meaning of the information transmitted is considered along the modalities. The most common are complementary and redundant combinations. The terms complementary and redundant have the same definition as in the CARE design space above (Vernier and Nigay, 2000). The redundant-complementary combinations convey information that is partially redundant and complementary. These combinations are useful for creating design rules, classifying existing output systems, and evaluating the usability of a system (Vernier and Nigay, 2000). For instance, in the multimodal Nestore eCoach, the chatbot can send instructions via written text, and the tangible coach can send the same instructions in a vocal form (redundancy). The chatbot can also add images and videos of the exercise (complementary), while the tangible coach can add lights to communicate at which stage of the exercise the user is (complementary).
In the context of the Nestore project (Angelini et al., 2022), multiple interfaces were introduced to older adults, including a chatbot and a tangible vocal coach. However, the independent use of each interface led to certain information being available exclusively on one platform. This setup raised questions about the potential benefits of combining these interfaces in a redundant-complementary manner. This prompts the exploration of whether such an integration can enhance the user experience, influence the relationship with the eCoach, and shape the perception of the system's social presence. Despite the extensive investigations into agent migration, the specific aspect of redundant-complementary combinations, entailing the parallel use of output modalities and interfaces for conveying richer meaning, remains uncharted territory in prior studies. While migrating information and social presence across embodiments is recognized as pivotal, the investigation into these particular combinations has been notably absent. There is no previous work found in the literature that states that redundant-complementary combination is better than only the assignment output which could be beneficial to increase the user-coach relationship. Hence, this study seeks to address this gap by delving into the unexplored realm of redundant-complementary modalities in the context of multimodal conversational agents. It also seek to investigate the impact of different output combinations on older users' perceptions of an eCoach as both a coach and a companion. By examining how various interfaces contribute to the user-eCoach interaction, we aim to enhance the understanding of the role of modality effects in eCoaching for older adults.
3. Methods
3.1. Usage scenario
We conducted two workshops involving HCI experts, with 6 participants in the first and 8 participants in the second. These workshops were aimed at defining scenarios and usage contexts related to the diverse interfaces within Nestore, designed for coaching and companionship purposes. The outcomes of these workshops were instrumental in constructing the comprehensive scenario outlined in this article and subsequently integrating it into the four distinct output modes.
The scenario unfolded as follows: an elderly individual awakens from sleep and proceeds to the living room. With the intention of engaging in a physical activity—specifically squats—the senior interacts with Nestore. To be precise, the senior asks three questions to Nestore (or questions of a similar nature):
1. What are my instructions?
2. Please start the exercise.
3. What is my overall score?
3.2. Output modes
Inspired by the study of Vernier and Nigay (2000) about combining output modes, the scenario was integrated in the four output modes. To enhance the readabality of the article, the terms are defined as follows: “Output mode” refers to our combined model encompassing both single-interface and multi-interface cases. “Modalities” encompass various modes, such as speech, images, and text. “Interfaces” pertain to either chatbots or tangible coaches.
• Single-Interface: The user is only given one interface (either a chatbot or a tangible coach). Because of its simplicity and ease of use, single-interface is essential for assessing older adults' perception of an eCoach. Figure 1 shows an example of the single-interface output.
- Chatbot: Nestore is a chatbot. The user engages in a direct one-to-one conversation with the chatbot. The interaction sequence unfolds as follows. Initially, the chatbot sends physical instructions to the user using textual descriptions and accompanying images. Subsequently, the chatbot initiates a counting process through animated GIFs. Finally, the chatbot concludes the interaction by conveying a summary of the user's score, employing both textual content and visual imagery.
- Tangible Coach (TC): Nestore is a tangible coach. The user engages in a one-to-one conversation with the tangible coach, leading to the following sequence of interactions. Initially, the TC sends physical instructions to the user audibly. Following this, the TC starts a counting process directed at the user, utilizing vocal prompts. Finally, the TC concludes the exchange by conveying a summary of the user's score, utilizing both vocal cues and illuminating lights.
• Multi- Interface: This output mode combines interfaces together. It may show that richer interactions can affect the relationship and social presence. Older adults are more exposed to the virtual coach due to its combined response which means that both interfaces can convey an information. The more the interaction is richer, the more it may deepen the sense of coaching and companionship. Two output modes are explored in this case as follows:
- Assignment: The user is provided with all interfaces. The system's information is assigned to one of the existing interfaces. This mode promotes the best interface based on the request type. Depending on the type of information, Nestore assigns it to one of the existing interfaces. Nestore interacts through a chatbot or a TC. In the case of providing instructions, Nestore employs the chatbot interface, utilizing text and images for conveying detailed information. For tasks that involve free-hand interaction, Nestore employs the TC interface, utilizing voice communication. When it comes to presenting scores, Nestore utilizes the chatbot interface, utilizing text and images to enhance the visual representation. Figure 2 shows an example of the multi-interface assignment output mode.
- Redundant-Complementary (Red-comp): Several interfaces transmit the same piece of information in a partially redundant and complementary manner. The information is supplemented by various modalities available in each interface in order to provide the user with more information. Nestore is both a chatbot and a tangible coach. In the case of instructions, counting, and scoring, Nestore responds with the same information via the chatbot and the TC, where each interface sends the information with all existing modalities in each interface. Figure 3 shows an example of the multi-interface red-comp output mode.
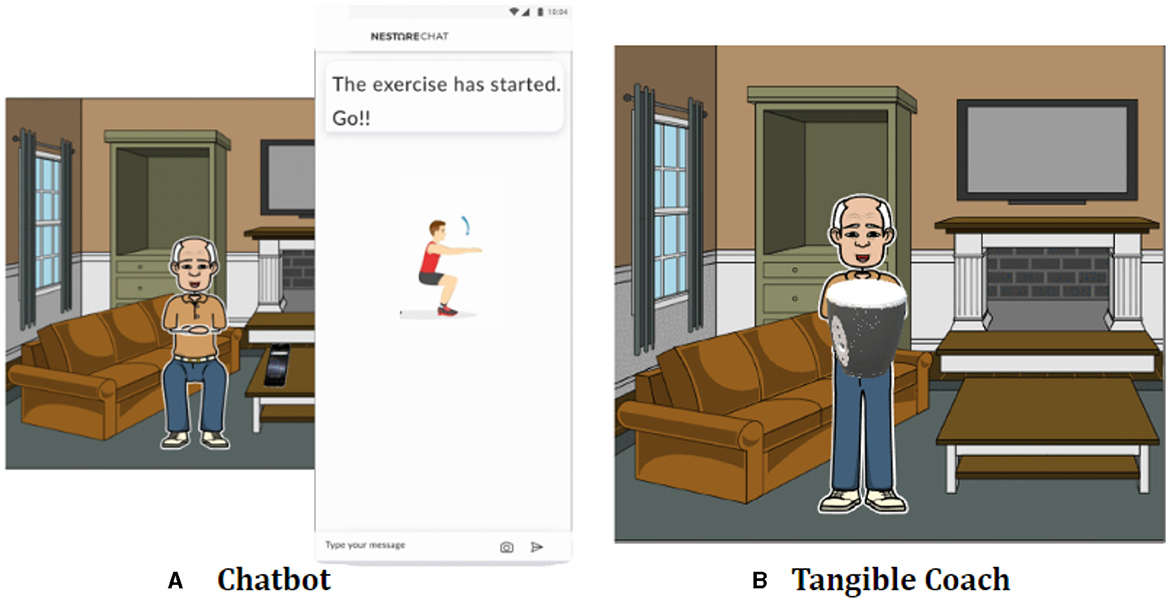
Figure 1. Single-interface output mode: (A) interaction with chatbot only and (B) interaction with tangible coach only. Created with Storyboard That.
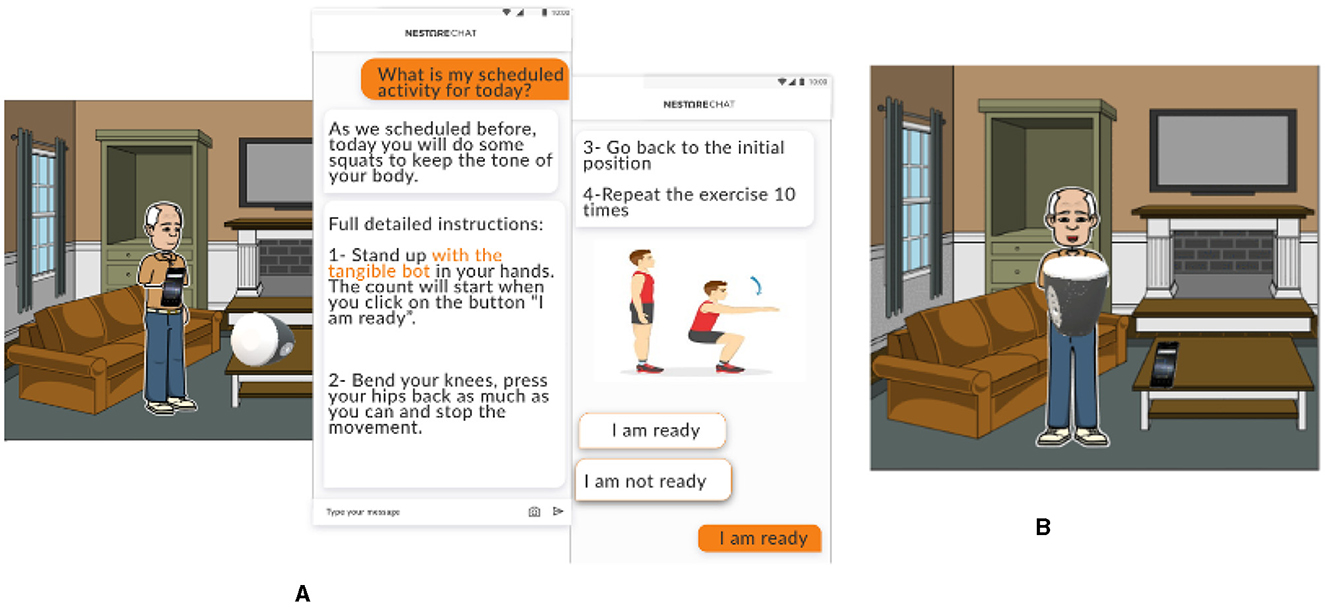
Figure 2. Multi-interface output mode. Interaction with assignment case. Based on the output message (instruction, count or score), only one interface will deliver the answer; (A) in case of instructions, the chatbot delivers the message; (B) in case of counting, the tangible coach delivers the message. Created with Storyboard That.
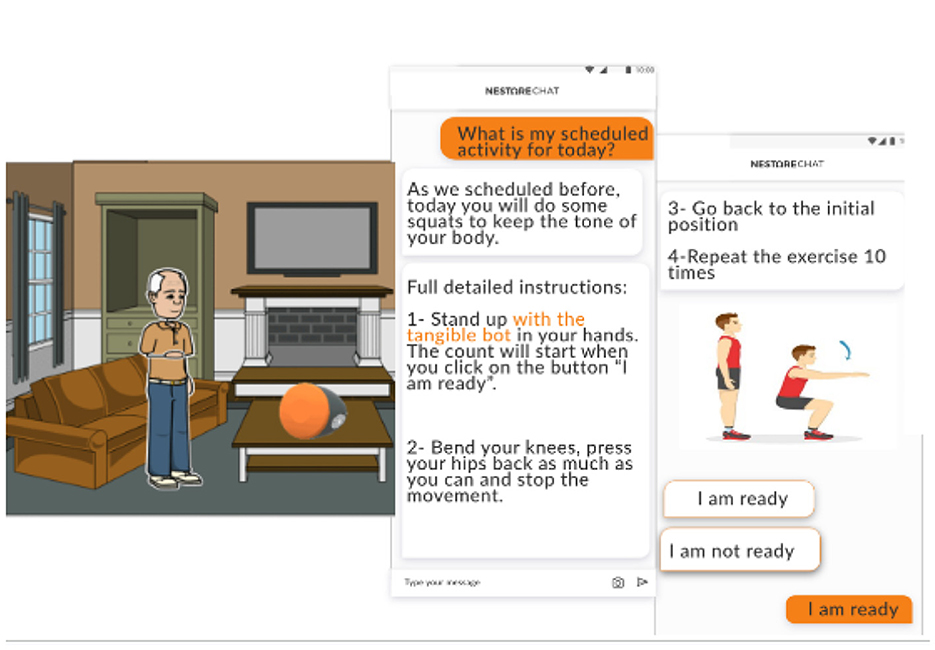
Figure 3. Multi-interface output mode. Interaction with Red-Comp case. Both interfaces deliver the output message in a redundant way but also in a complementary way because of its various modalities such as images and lights. Created with Storyboard That.
3.3. User study
To answer our research questions, we conducted a within-subject study aimed at evaluating the perception of older adults regarding different output modes with a virtual coach named Nestore. The work consisted of two studies: an online web-based survey and a face-to-face experiment. The online web survey was chosen due to the constraints imposed by the COVID-19 pandemic, enabling data collection while adhering to safety measures. The face-to-face experiment aimed to provide participants with a direct interaction experience with Nestore. In both study phases, participants were introduced to Nestore's capabilities. They watched a video of older adults interacting with Nestore to gain insight into the modalities. Next, participants evaluated Nestore's output modes based on one scenario (Section 3.2). We ensured that participants engaged with each output mode in a randomly chosen sequence. This randomization aimed to minimize any potential bias or order effects. They provided qualitative and quantitative feedbacks, enabling us to comprehensively understand their perceptions. The storyboards used in the online study effectively replicated the interactions facilitated in the face-to-face experiment. This allowed for a consistent assessment of both study settings (web survey vs. face to face). Figure 4 shows an example of the online web survey storyboard vs. face-to-face experiment. Notably, the voice of the physical coach is opposite to the user's gender preference, as indicated in a study mentioned in the reference (Angelini et al., 2021). In that study, users expressed a preference for a coach with a gender different from their own. In contrast, Nestore adapts its responses and wording based on the user's gender; for example, if the user is a woman, Nestore will respond accordingly. The study was implemented in English and French.
3.3.1. Online study
During the COVID-19 pandemic, we leveraged an online web survey as a rapid and convenient means of data collection. The survey was designed using platforms such as Unipark (Startsteite, 2022), which facilitated the inclusion of various media elements such as videos, GIFs, and diverse question types. The survey's content was carefully constructed to ensure logical alignment with the scenario and maintain consistency with the face-to-face experiment.
To bridge the gap between online and in-person interactions, we utilized interactive storyboards. Storyboarding enabled the depiction of interactions between older adults and Nestore's various output modes. Participants were immersed in scenarios, wherein an older adult sought to enhance wellbeing through interactions with Nestore. Additionally, we integrated techniques to gauge participant engagement and seriousness (Aust et al., 2013).
3.3.2. Face-to-face study
The face-to-face experiment took place within the premises of the University of Applied Sciences and Arts Western Switzerland, Fribourg. Participants engaged directly with Nestore, offering them a tangible experience. An experimenter guided participants through the study, emphasizing the presence of an operator to assist during the process. Each participant interacted with Nestore, both the tangible coach and the chatbot. Notably, the experiment involved a degree of semi-autonomy, where the tangible coach's interaction was facilitated by a human operator. Participants were only informed of this at the study's conclusion. Notably, those who did not give their consent for photography were excluded from having their pictures taken, while those who granted consent had their pictures taken.
3.3.3. Participants
For the online study, we recruited 59 older adults (28 men and 31 women) aged 65–85 years with mean 75, primarily through the Prolific platform and local university channels. Most participants are from the UK, Switzerland, or Italy. The average completion time for the online survey was approximately 18.24 min. In total, 88% use a smartphone everyday and rarely to never use chatbots or smart speakers. A significant portion expressed motivation for maintaining a healthy lifestyle.
For the face-to-face study, we recruited 15 seniors (10 men and 5 women) aged 65–85 years wih mean 77 participated. Most participants are from Switzerland or Italy. These individuals were selected from both a senior home association or word of mouth. Participants exhibited engagement with technology and a willingness to stay updated with its advancements. In total, 90% of participants use a smartphone everyday and rarely to never use chatbots or smart speakers.
3.4. Analysis procedure
We evaluated the perception of older adults based on qualitative and quantitative analyses for both evaluation study settings. The user study is based on the evaluation of the output modes in order to understand how the user could combine the interfaces of the virtual coach (tangible and chatbot) to be perceived as a coach and friend/companion. The goal of these output modes was to simplify the user-coach relationship while making the experience more engaging. The involvement of various modalities and interfaces can have an impact on the relationship. Relationships and social presence may be used to assess the users' perception of Nestore as a coach or companion. CART-Q (Jowett and Ntoumanis, 2004) and social presence (Toader et al., 2019) questionnaires were used to assess the relationship between the eCoach and the user. The CART-Q was chosen because it can assess the relationship between cognitive (commitment), affective (closeness), and behavioral (complementarity) dimensions. Their findings support the multifaceted nature of the coach–athlete relationship. Thus, the measured relationship can demonstrate not only the perception of a coach but also the perception of a companion. To shorten the questionnaire, the items were chosen based on the highest item-total correlation, as suggested in the study by Eisinga et al. (2013). Concerning social presence, Toader et al.'s scale (Toader et al., 2019) is used. It contains constructs such as human warmth, human contact, sociability, source of comfort, and sense of support. These constructs are also related to the users' perception of the eCoach as a companion. Both questionnaires use a seven-point Likert scale. Participants were also asked to rank the different output modes according to how much they perceived Nestore as a coach and companion and according to which they preferred overall. In this context, “WS” denotes online web surveys and “FF” denotes face-to-face experiment. In the face-to-face experiment, user experience of each output mode is also assessed with the UEQ-S questionnaire (Schrepp et al., 2017) because users actively participate in testing. Finally, two open-ended questions were asked at the end about the positive aspects they found in their first choices and the negative aspects they found in their last choices in the ranking questions. The analysis was conducted by focusing on both the positive and negative aspects of the highest and lowest rated output modes. In this context, “P#” denotes the participant number, “ws#” denotes online web surveys, and ff# denotes face to face. As an example, “Pws2” represents an excerpt from the interview of participant 2 in the web survey, Pff2 represents an excerpt from the interview of participant 2 in the face-to-face experiment. Table 1 describes the questionnaires used.
4. Results
4.1. Quantitative results
Table 2 summarizes the results of web survey, and Table 3 summarizes the results of the face-to-face experiment. Only significant results between pairs of output modes are shown in these tables.
4.1.1. Likert scale analysis
Because the data were not normally distributed, a Friedman test was used for data analysis. Conover's post-hoc test was employed to compare pairs of output modes and determine the presence of statistically significant differences between them.
Participants in both the web survey and face-to-face interactions consistently reported relatively low mean scores for both Nestore's relationship (CART-Q) and social presence, indicating an overall less favorable perception in respect to all output modes. Notably, the chatbot consistently received lower scores compared with other output modes.
However, in the case of face-to-face interactions, it is noteworthy that all median values were equal to or greater than 4 (except for the perception of a chatbot as socially present). This suggests that there is room for improvement. The overall median perception of the coach-user relationship and social presence is equal to or a bit greater than half, indicating a more positive trend.
4.1.1.1. CART-Q
In the web survey, significant differences in senior responses in terms of commitment and closeness were found in the case of the coach-user relationship (RQ1). Seniors were able to significantly perceive commitment toward Nestore [ = 7.87, p = 0.05], as well as closeness toward Nestore [ = 10.26, p = 0.017], whereas complementarity results were not significant [ = 1.51, p = 0.68]. Conover's post-hoc tests revealed that older adults perceive to be more committed when using the red-comp mode (M = 3.68, SD = 1.84) compared with receiving a response only from the chatbot (M = 3.32, SD = 1.66) with t-stat = 2.72 and p = 0.007. They perceive a closer connection when using the red-comp mode (M = 4.034, SD = 1.89) vs. receiving a response only from the tangible coach (M = 3.81, SD = 1.84) with T-stat = 2.89, p = 0.004.
In the case of face-to-face, in the case of coach-user relationship, there was no significant differences in terms of commitment, closeness, and complementarity. CHI-squared revealed that seniors were not able to significantly perceive differences between different output modes [COM (=2.032, p = 0.556), COMP ( = 3.87, p = 0.28), and CLO (=3.37, p = 0.34)].
4.1.1.2. Social Presence (SP)
Among the 4 output modes that users tested and experimented them participants perceived the tangible coach [WS:(M = 2.885, SD = 1.8), FF:(M = 5, SD = 2.03)] more socially present than chatbot only [WS: (M = 2.51, SD = 1.56), FF: (M = 3.79, SD = 1.6)] with [WS: t-stat = 2.94 and p = 0.004, FF: t-stat = 2.383, p = 0.022]. The red-comp [WS: (M = 2.99, SD = 1.77) , FF: (M = 4.44, SD = 1.59)] was perceived more socially present to only chatbot (WS:t-stat = 1.16, p < 0.001, FF: t-stat = 2.15, p = 0.04).
In the case of web survey, CHI-squared revealed that seniors perceived Nestore's social presence differently depending on the output modes [ = 14.12, p = 0.003]. Conover's post-hoc tests revealed that seniors perceive the tangible coach (M = 2.885, SD = 1.8) to be more socially present than the chatbot alone (M = 2.51, SD = 1.56) with T-stat = 2.94 and p = 0.004, and that seniors perceive the red-comp mode (M = 2.99, SD = 1.77) to be more socially present than interacting only with a chatbot (M = 2.51, SD = 1.56) with T-stat = 1.16, p < 0.001.
In the case of face-to-face, CHI-squared revealed that participants were able to significantly perceive differences between different output modes in terms of social presence[= 8.881, p = 0.031]. The tangible coach (M = 5, SD = 2.03) was perceived more socially present compared with only chatbot (M = 3.79, SD = 1.6) with t-stat = 2.383 and p = 0.022, and Red-comp (M = 4.44, SD = 1.59) was perceived more socially present to only chatbot with t-stat = 2.15 and p = 0.04. Moreover, assignment (M = 4.093, SD = 1.88) was perceived more socially present than tangible coach (M = 4.41, SD = 1.65) but with a value very close to be significant with t-stat = 1.2 and p = 0.05.
Because the data were not normalized, the Mann-Whitney test was used. All subscales differ significantly between web survey and face-to-face. Figure 5 shows that the web survey produced significantly lower results than the face-to-face experiment. In person, each subscale is greater than 4, indicating a stronger relationship and social presence, compared with the web survey.
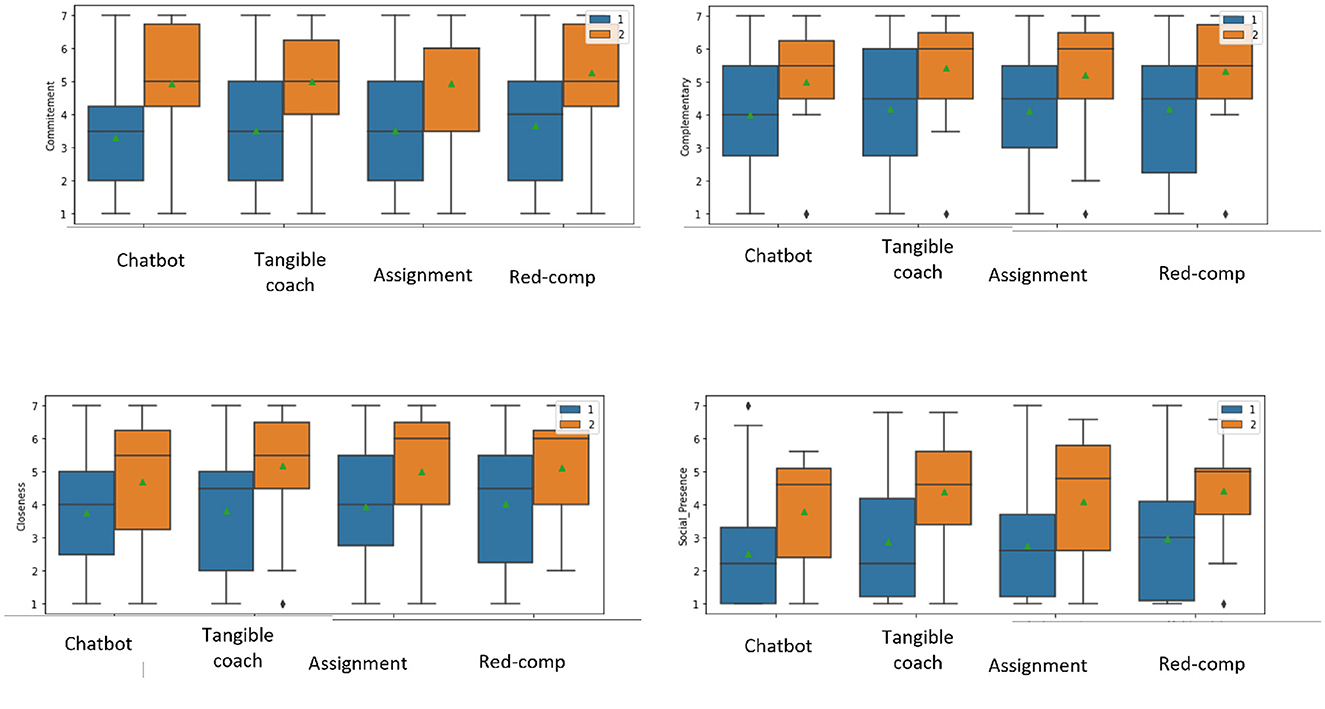
Figure 5. Difference of Likert scale results between web survey study setting (1) and face-to-face study setting (2).
In terms of differences between each output mode, as demonstrated by the web survey, the tangible coach and assignment and Red-comp have significantly higher results when compared with the chatbot in terms of commitment and social presence. To summarize, similar results are shown when comparing different output modes in both study settings. When the results were significant in both study settings, it was usually the chatbot who scored the lowest.
4.1.2. Ranking analysis
Three ranking questions were asked: one for coach perception, one for companionship, and one for preference. The percentage was calculated to determine how many participants chose the first mode to be perceived as a coach and provide with companionship and is most preferred.
The Wilcoxon ranking test was used to determine whether there were any significant differences between output modes. To note, if the mean is lower, it means the rank is highest. The results were mostly found between the chatbot and the other output modes.
In terms of coaching, the assignment output mode was consistently perceived as a coach, outperforming the chatbot alone with (WS: p < 0.001, FF: p = 0.03) in terms of coaching capabilities.
The results also show that the chatbot was consistently less liked in terms of companionship when compared with other output modes. Older adults favored companionship from the tangible coach (WS: p = 0.008, FF: p = 0.008) and the assignment mode (WS:p = 0.001, FF: p = 0.006) and the red-comp over the chatbot (WS: p = 0.003, FF:p = 0.016). In terms of preference, the multi-interface was chosen over the chatbot. The Red-Comp was favored over the chatbot with (WS: p = 0.001, FF: p = 0.039) and the same for the assignment case with (WS: P < 0.01 and FF: p = 0.006). No results were found between Red-comp and assignment case.
In the case of web survey, one participant have mentioned in the qualitative data that their ranking was not significant “I had to choose because there was no choice to say none. I have no reason to think this would work for me (Pws92)”. Hence, his or her data were deleted for the ranking.
In terms of coach rank, in particular, 46.55% of seniors rated the red-comp as top 1. In terms of companion rank, 43.10% of seniors rated the red-comp top 1. In terms of ranking the preference, 44.83 % of seniors rated red-comp as top 1.
In terms of ranking the output combinations as a coach (RQ3), the tangible coach (M = 2.78, SD = 0.93) edged out the chatbot (M = 3.14, SD = 1.15) with p = 0.051. The assignment (M = 1.95, SD = 0.66) ranked significantly higher than the chatbot (p < 0.001). Similarly, the red-comp mode (M = 2.138, SD = 1.24) was found to be significantly perceived more as a coach than the chatbot with p < 0.001. The assignment outperformed the tangible coach (p < 0.001), while the red-comp mode outperformed the tangible coach (p < 0.006).
In terms of ranking companionship (RQ4), the chatbot (M = 3.07, SD = 1.23) was significantly less perceived as a companion by older adults than the other output modes: tangible coach (M = 2.48, SD = 0.96), p = 0.008, assignment (M = 2.24, SD = 0.8) p = 0.001, and red-comp (M = 2.21, SD = 1.24) p = 0.003.
When it comes to ranking the output mode that older adults prefer to interact with (RQ5), seniors ranked the chatbot (M = 3.86, SD = 1.25) lower than the assignment mode (M = 2.05, SD = 0.69) with (p < 0.001) and lower than the red-comp mode (M = 2.12, SD = 1.22) with p = 0.001. They also ranked the tangible coach (M = 2.74, SD = 0.9) lower than the assignment (M = 2.05, SD = 0.69) with p < 0.001 and lower than the red-comp mode (M = 2.12, SD = 1.22) with p = 0.003.
In the case of face-to-face, in terms of ranking as a coach, 46.67 % seniors rated the red-comp mode as top 1. In terms of ranking as a companion, 46.67 % of seniors rated the tangible coach as top 1. In terms of ranking as a preference, 46.67% seniors rated the multi-interface mode as top 1, as 40% of seniors rated the tangible coach as top 1.
The assignment output mode perceived more as a coach compared with chatbot only (p = 0.03), while the red-comp was perceived higher compared with only chatbot (p = 0.052). In terms of companionship, the tangible coach outranked the chatbot (p = 0.008) and the assignment outranked the chatbot (p = 0.005). The red-comp case outranked the chatbot (p = 0.016). In terms of general preference, the tangible coach was preferred to the chatbot (p = 0.018), and the assignment was preferred to the chatbot (p = 0.006). Finally, the multi-interface red-comp was preferred to the chatbot(p = 0.039).
The chi-square test was used to determine whether there are any significant differences between the two modes of evaluation. Except in some cases, the chi-square test revealed no significance. Significant differences between the two study settings was shown for ranking the chatbot as a coach [ = 14.62, p = 0.002] and ranking the assignment as a coach [ = 12.955, p = 0.005]. The ranking as a companion showed also significant differences for the chatbot [ = 10.94, p = 0.01].
In terms of ranking questions, the top 1 differed in terms of companionship. In the face-to-face experiment, it was the tangible coach. In the web survey, however, the tangible coach was ranked third. In fact, participants felt more of the social presence of the tangible coach in person, enjoyed interacting with it, and found it very easy to use. In the face-to-face experiment, participants stated that the tangible coach could provide enough companionship through its vocal capability. According to the comments, unlike in the web survey, participants were unable to have this experience with the tangible coach.
4.1.3. UEQ for face to face experiment
User experience was evaluated. Figure 6 shows the mean of UEQ results. Table 4 shows the significant results in terms of overall pragmatic and hedonic subscales.
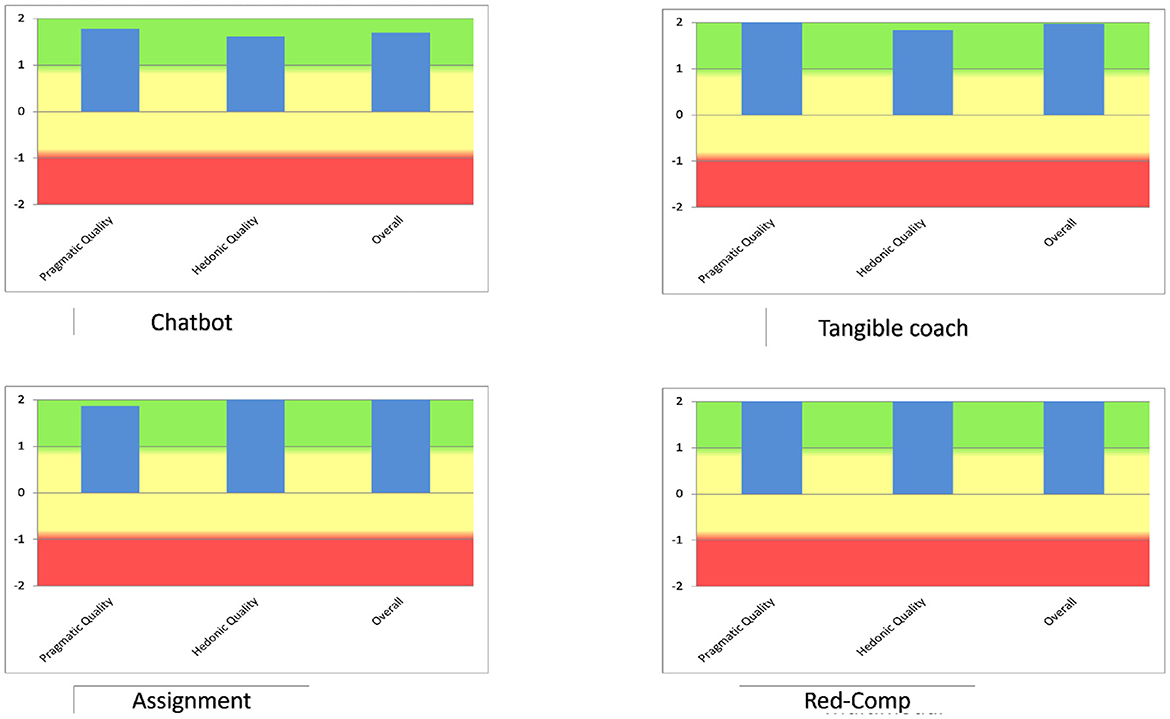
Figure 6. UEQ results. Red color indicates negative sentiment or unfavorable responses, yellow color corresponds to neutral sentiments, and green color represents positive sentiment or favorable reactions.
Significant results were obtained. In terms of pragmatic results, the chatbot was less pragmatic and less hedonic (and overall providing and inferior user experience) compared with the other four output modes (p < 0.05). Furthermore, the tangible coach is perceived less pragmatic (t = 2.66, p = 0.01) than the assignment case. Moreover, the tangible coach is perceived less pragmatic than the red-comp (t = 3.65, p < 0.001), and the tangible coach is perceived less pragmatic than the assignment. The tangible coach received a significantly lower overall score than the assignment (t = 2.24, p = 0.03) and the red-comp (t = 3.22, p = 0.002). There were no significant differences between assignment and red-comp.
4.2. Qualitative results
While the questions pertained to the first two and last two output modes, participants predominantly provided insights into only the first and last modes. Most qualitative responses revolved around giving feedback regarding the positive aspects of their initial choice. To uncover recurring patterns across datasets, a thematic analysis approach was employed. Thematic analysis was selected due to its capacity to explore explanatory conceptual themes related to older adults' engagement with four distinct modes of interaction.
When presenting qualitative results, it is possible to observe a higher number in a category such as “Pws98,” even though there were only 59 participants for, e.g., in the web survey (same thing for the face-to-face study). This discrepancy can be attributed to the inclusion of adults in the overall testing. However, it is important to note that the primary focus of this article is on older adults. As a result, the increased number in the “Pws98” category reflects a combined response from both the limited pool of participants and the broader group, which includes adults.
Older people are a diverse group, with unique perspectives and experiences with technology. As a result, participants' reactions and perspectives on the four designs ranged from excitement and curiosity to hesitancy, uncertainty, and even refusal to all of them.
Many participants refused these types of technologies upon entering the room, but after the experiment, some became more open about it and began to see the value in Nestore: “it is more pleasant than what I thought at first, it is quite human and better than the general silence” (Pff14), “I didn't like the technology but you almost converted me” (Pff14).
Some older adults have said that this type of technology is required for older people who feel isolated: “There seems a misconception that people above 60 are old and in need of an electronic gadget to keep them company 2- Really old people >80 tend to be not familiar with electronic gadgets 3- If successful it needs to be looked into with real elderly people that are alone” (Pws1)
Then, through their comments, participants appeared to recognize, appreciate, and enjoy the various benefits that each of the four output modes provide. The findings revealed some critical factors to consider when combining a chatbot and a tangible coach. The following sections contain reports on how participants' use and perception of being a coach and a companion for the different output modes differ across interactions in the web survey and the face-to-face experiment.
• Simplicity, clearness and ease of use, non-intrusive: The chatbot garnered the bulk of comments regarding its simplicity. Among the four output modes, in the web survey, older adults found the chatbot to be the simplest. One could posit that this stems from their unfamiliarity with speaking with physical devices (such as smart speakers) and the other output modes involving more devices, which might be perceived as more challenging. A majority of participants appreciated the notion of a straightforward and uncomplicated interaction with the chatbot: “(...) Instructions were clear (...)” (Pws64). In contrast, in the face-to-face, participants noted that interacting with the tangible coach was highly straightforward. On the other hand, some participants were not fond of the chatbot due to its complexity, especially the challenge of typing or pressing buttons that were too small for certain participants' fingers. Interestingly, almost all participants (with the exception one) chose to utilize the tangible coach to submit their question in the red-comp and assignment case, citing speed and ease of use as reasons. Those who attempted to input text did so mainly for experimental purposes. One participant regarded the chatbot as the most favorable option, praising its automatic nature and accessibility and stating “The responses are automatic and accessible(Pff1).” Additionally, one participant regarded the multi-interface mode red-comp case as an easy interaction: “Combining reading, listening, and watching makes it easier to interact and follow instructions (...)” (Pws11). Finally, some participants refused all four options, stating: “I found it all too complicated and impersonal” (Pws83).
• Social Presence (Sense of Humanness): Comments suggest that older adults value the sense of humanity in their interactions. Different output modes yielded distinct outcomes. Most comments indicated that both the chatbot and the assignment case felt overly automated for seniors. For instance, the chatbot's automated and impersonal nature was highlighted: “It sounds very monotone” (Pws30). Similarly, the assignment case was criticized for its lack of personal touch: “(...) It feels automated and lacks personalization” (Pws94). While a few participants found the tangible coach to lack personal connection (“I find this contact impersonal”—Pws26), the majority of comments favored the tangible coach. It was perceived as socially present due to its ability to vocalize and impart a sense of warmth: “Hearing a voice makes it feel more personal and warm” (Pws97). In fact, participants expressed a strong preference for speech-based interactions. They found the option to hear a voice comforting and more akin to interacting with a human. Some participants even stated a preference for speaking to the coach over writing to it (“the option to hear a voice gives me a sense of interacting with a human although this is only a sense” (Pws5), “I like the sound of the voice as it sounds more like a real instructor.” (Pws9), “the voice interaction is more than enough for me.” (Pff2), “I prefer voice interaction”) (Pff11). This positive feedback suggests that speech-based interactions were well received and considered more natural by users. Some participants expressed concern about how the quality of the voice-based technology used could affect the overall experience: “I did not find the voice of the tangible device very pleasant(...) (Pws61).” Moreover, although the goal of the study was to focus on how participants want Nestore to respond to them, many participants mentioned that they prefer speaking to the coach more than writing to the coach. Furthermore, the red-comp mode was also deemed and socially present due to its dual interfaces: “It also gives the impression that the coach is present to assist and enhance the exercise experience” (Pws11). Finally, some participants did not accept Nestore, in general, to be able to give any kind of human warmth or social presence: “when I see a guy who takes a picture to see his calories for me it's crazy, to have a camera to tell him that” (Pff15), “Getting encouragement from a machine is not good” (Pff13).
• Cooperation: When performing an activity, participants expressed cooperation between the user and the coach in some of the output modes. One participant, for example, complained that the chatbot was inconvenient when performing a physical activity. This implies that he or she has a lot on his or her plate: “Cumbersome to interact with if you do physical workouts(Pws51)”. Other participants explained that the assignment case gives them a sense of purpose since it seems that there is a way to do the exercise and the system is adapting to it. The red-comp was described as cooperative from most participants due to its ability to enhance task comprehension, especially for new exercises, as mentioned by one participant: “ability to understand the task better, especially for a new exercise (Pff3).” Additionally, the chatbot's capability to provide written documentation led to a higher cooperative assessment from another participant: “The chatbot has a written record (Pff5),” and they added, “If I forget the exercise, I can go back and check the instructions. It's particularly beneficial for unfamiliar exercises.”, “I prefer to have spoken instructions backed up by written instructions. (Pws98)”
• Commitment: The majority of comments indicated that a screen-based interface can enhance commitment to the activity, but a combination of both approaches could provide additional encouragement to commit. As stated by one participant, utilizing a screen-based interface helped foster a sense of purpose, potentially leading to heightened dedication to the activity: “I felt like the chatbot was akin to having an instructor. The exercise was clear with this output mode and it gave me a sense of purpose and something to look forward to.” (Pws64), “I like Nestore to decide for me” (Pff14), and another mentioned being “used to doing exactly what was asked (Pff15).” Another participant highlighted the red-comp mode as a means to motivate a broader audience to engage in the exercise: “(...) I believe this would be the option that would motivate me the most (...)(Pws56)”, “an incentive to prompt me to do it when reopening the tablet (Pff5).” On the other hand, some participants highlighted the tangible coach's ability to assign tasks in real-time, which they found effective in ensuring completion. One participant stated, “I like the tangible coach because I can listen and do the exercise (Pff9).”
• Complementarity: Comments highlighted how Nestore's capabilities position it as an effective coach for seniors. Numerous remarks underscored the value of screen-based exercises, allowing users to better visualize both the exercises and their outcomes: “The chatbot provides more opportunities to comprehend the instructions I received.” (Pws61). Conversely, many comments emphasized the importance of utilizing both approaches to create an immersive experience and foster comprehensive interaction. Considering their distinct capabilities, several participants discussed the complementary nature of these interface modes. Most suggestions revolved around instruction and encouragement, envisioning the chatbot as a coach and the tangible interface as a means to motivate users: “I believe the chatbot and tangible coach together complement each other, enabling a fully immersive experience for maximizing the benefits of the interaction” (Pws57). On the other hand, a few participants mentioned redundancy in the web survey when both devices confirm each other's actions: “Having speech interaction is great, but the repeated confirmation messages feel redundant.” (Pws69). Whereas in the face-to-face, participants generally found redundancy less appealing and considered it acceptable primarily for educational purposes, especially for getting coaching instructions for a new exercise at the beginning. As one participant expressed, “So if it provides a different answer, that's okay, but I dislike redundancy. Nevertheless, it is preferable to the assignment or chatbot alone, since the assignment case can be confusing (Pff11).” Although participants did not rank the red-comp case last due to its multifunctionality in the face-to-face, many of them confirmed that they would not engage with both reading and listening simultaneously, deeming it superfluous information for them. On the other hand, the idea of complementary information was suggested, utilizing non-overlapping modalities such as images and voice.
• General preference: Many people expressed a preference for interacting with a chatbot over a physical coach (tangible coach). The feedback was focused on specific modalities or the overall interface: “I don't like text based” (Pws57), “voice not my liking” (Pws67).
• Cognitive load: Participants mentioned how convenient it was to interact with it from a distance without using any tools for input or reading visual output. Participants appreciated that a tangible coach requires no physical interaction other than speaking and listening. The ability to operate the device using voice from a distance was recognized as a significant advantage over other devices, particularly for those who have physical declines, such as decreased mobility or vision loss typical of the natural aging process: “I do not always have my reading glasses with me so reading the chatbot could involve extra activity not required by the tangible coach.” (Pws60), “requires no attention” (Pff9). However, it was also considered stressful due to the need to concentrate on what the tangible coach was saying (“stressful, requires focus, attention, speaks fast”) (Pff16). On the other hand, the assignment was occasionally viewed as perplexing since participants could not determine the source of the answers, leading to confusion “Who chooses? That's confusing.” (Pff11). Finally, because users could revisit and review the chatbot's messages, it was perceived as imposing a lower cognitive burden “I can check my messages again, no need to ask and repeat the question.” (Pff5).
• Disability concerns: Some participants expressed a preference for single-interface cases due to disability concerns: “The chatbot alone does not meet the needs of people with visual and psychometric disabilities of the hands. The tangible alone does not meet the needs of people with hearing loss.” (Pws28). Other participants rated the red-comp the highest because it can solve problems such as disability concerns: “I have a hearing problem. Maybe if he can connect the device to his headphones” (Pff13), and “It could be useful for someone who has difficulty on one side” (Pff15).
• Richness: Users mentioned richness in terms of interaction richness, modality richness, and information richness. Some participants criticized the red-comp mode for having too many varieties and devices. Comments such as “too any interfaces for the user” (Pws8), or found this arrangement confusing and unnecessary “having more conversational agents is nice but there's no need for all of them to interact together all the time” (Pff3). Other participants, on the other hand, expressed an interest in combining these two interfaces, particularly in the form of a synergistic interaction that takes advantage of all of the benefits that they provide and compels them to engage all their senses to accomplish tasks.: “The red-comp case would give me the most extensive interaction (Pws51)”, “richer interaction” (Pff3), “appeal to the senses that are important” (Pff15).
• Sense of control: When participants ranked the assignment and red-comp highest, the majority of comments centered around the flexibility to select how their responses are delivered: “Having two interfaces provides the option to choose how the response is presented.” (Pws52). There were even suggestions that the assignment feature should allow users to pick the source of the response: “Having the ability to individually select the appropriate mode for each situation is convenient.” (Pws90). Interestingly, due to the absence of an interface choice, one participant rated the single interface option the lowest and the red-comp combination the highest: “No choice of interaction method” (Pws52). Some individuals leaned toward the red-comp combination because it offers the chance to receive both types of information simultaneously, granting them the freedom to choose where to access it: “We are provided with choices and not just predetermined responses.” (Pws30).
• Adaptivity: The assignment case was commonly perceived as the most adaptable and received positive ratings.
The majority of comments revolved around the desire for a system that could adjust to their preferences and context. Consequently, in this scenario, they assigned a higher rating to the assignment case, highlighting its adaptability to individual preferences and contextual requirements. For instance, one participant mentioned an appreciation for the ability to observe how the chatbot tailors its responses to their requests: “I observed the bot's reaction to my requests” (Pws6). other remarked “(...) depends on the person if they are visual or auditive (Pff2)”. Some participants suggested that while they prefer interacting with the tangible coach, they would like to receive responses from the chatbot, as expressed in this quote: “I prefer to speak to the tangible but receive the info on my tablet. Can I do that?” (Pff12). Additionally, they inquired about the possibility of customizing the system's adaptability to their needs, as one participant queried, “Can I change how I want the response to be, adapt it based on my needs” (Pff3)? They indicated that adaptability should be a determining factor. Some users might have assigned the lowest rating to the single interface option due to its lack of adaptability: “This strikes me as the least flexible” (Pws9).
• Enjoyment: Comments were also found regarding the level of enjoyment seniors experienced when interacting with the various output modes. They discovered that having two interfaces is more enjoyable, pleasant, and communicative: “Didn't enjoy just the one form of communication as much as the two together” (Pws78).
• Habits: Numerous participants indicated that their choice was influenced by habitual factors. A subset of participants assigned the highest rating to the chatbot due to their familiarity with smartphone usage and WhatsApp. One participant mentioned, “I am accustomed to having a smartphone” (Pff1). Another group of participants ranked the option of having two interfaces as their top choice while acknowledging the necessity of adapting and establishing a routine. One participant noted, “I need to get used to it (Pff2).”
• Transportability: Certain participants expressed their preference for the chatbot, citing its ability to accompany them wherever they desired: “i can take the phone wherever i want” (Pff1).
• Error prevention: Some participants expressed concerns about whether they would understand a typical exercise or not; hence, the red-comp was their best output mode: “if I don't understand the message from the first interface, I can reconfirm it from the other” (Pff12).
5. Discussion
These output modes were tested to understand how they influenced the perception of Nestore as a coach and companion and their overall preference. Social presence and the relationship between the user and the eCoach were measured. The results consistently demonstrated a preference for combining multi-interfaces over using single-interfaces. Participants appreciated the richness that multi-interface cases brought to their experience.
Below, we answer our research questions and discuss them based on our results.
• RQ1: What is the effect of output modes on user's perceptions toward the user-virtual coach relationship?
The first research question prompted us to delve into distinct trends. Our exploration revealed that exclusive interaction with a chatbot resulted in diminished levels of commitment and perceived social presence when contrasted with other output modes. Both interface options played a pivotal role in shaping these perceptions. Intriguingly, among older adults, the preference leaned significantly toward the red-comp setup over the chatbot when evaluating commitment. This observation underscores the pivotal role that engaging with both interfaces can play.
Furthermore, when assessing the closeness dimension, users displayed a marked preference for the red-comp mode over both the chatbot and the tangible coach. This preference suggests that the single-interface experience may be interpreted as less intimate. This insight underscores the essential nature of incorporating both tangible and chatbot elements for a well-rounded user experience.
Qualitative data provided additional depth to these findings. Participants often described the red-comp mode as cooperative, effectively delivering more information and enhancing complementarity. The distinct capabilities of each interface contributed to inspiring users to perform better. In contrast, while the CART-Q analysis did not yield statistically significant results, qualitative insights surfaced the prevailing sentiment among participants. Many suggested a transformation of the red-comp setup into two complementary conversational agents for coaching. There was a significant appreciation for its provision of complementary messages. This sentiment was observed in both study settings, underlining the effectiveness of this mode in delivering comprehensive information. In this proposed model, the chatbot was perceived as a supporting tool that aided users in adhering to exercise commitments. In contrast, the tangible coach assumed the role of the primary eCoach, vigilantly monitoring user compliance. Notably, a distaste for redundancy became apparent, with participants favoring a more complementary message delivery approach. Interestingly, the expectation that older adults would appreciate redundancy was not entirely confirmed. While redundancy was initially thought to be valued, many comments from the face-to-face experiment expressed negative sentiment toward redundant information, advocating for complementary messages to prevent repetition and cognitive load. This sentiment aligns with Reeves' design guidelines (Reeves and Nass, 1996), which advise against redundant information. However, participants acknowledged the potential need for redundancy during the learning phase, reconfirmation, and support, highlighting the complexity of this aspect.
• RQ2: What is the effect of output mode on user's perception of the social presence of virtual coach?
Distinct patterns emerged from social presence perception. Notably, participants consistently regarded the chatbot as embodying less social presence compared with all other output modes. The chatbot was perceived as less socially present and was often observed as demanding more cognitive effort, particularly evident in the face-to-face experiment. Participants proposed the potential role of the chatbot as a visual aid for reinforcing the coach's messages, suggesting its use outside the home or for visualization purposes. Specifically, the tangible coach garnered a stronger sense of social presence than the chatbot, with participants frequently citing the voice of the tangible coach as a factor that emanated warmth and a palpable sense of presence. This aligns with prior research indicating that embodied conversational agents (ECAs) can foster a sense of companionship, especially when compared with chatbots (Sidner et al., 2018a). This also resonates with findings from the study mentioned in the reference (Loveys et al., 2020), which suggests that an embodied agent with voice capabilities enhances rapport in healthcare settings. However, it is imperative to acknowledge that the quality of this voice played a pivotal role in this perception. Furthermore, participants from the web survey highlighted the multi-interface nature of the red-comp mode as a contributor to the feeling of presence, signifying the efficacy of combining different modes within the red-comp interface. This outcome reinforced the idea that multi-interface within the red-comp interface led to significantly enhanced results in comparison to single-interface interactions. In contrast, participants in the face-to-face experiment expressed that the presence of multiple interfaces at home was not an essential requirement to evoke a sense of presence. This sentiment led to the conclusion that the tangible coach alone was sufficiently adept at fostering a sense of presence among the senior participants when evaluating via face-to-face setting.
• RQ3:Which output modes improve the perception of the agent as a coach?
Ranking results shed light on participants' preferences. Red-comp emerged as the top choice, followed by the assignment, tangible coach, and chatbot, respectively. This ranking underscored the significance of multi-interfaces, with both red-comp and assignment outperforming single-interface interactions, and tangible coaches being favored over chatbots. Qualitative data provided further understanding to these rankings, revealing that the red-comp interface was lauded for its enhanced cooperation and complementarity. The assignment, on the other hand, was noted for its ability to foster commitment through its adaptability. They liked it because the coach has a specific way of conducting the exercise and takes on a specific role. Finally, user comments suggested that the tangible coach effectively reduced cognitive load.
• RQ4: Which output modes allow the agent to provide users with companionship?
Participants perceived the chatbot as a less effective companion. Qualitative data further elaborated on this sentiment, with participants expressing dissatisfaction over the chatbot's perceived monotony and automation. In contrast, the tangible coach was celebrated for providing social presence and emanating warmth. The tangible interaction and hands-free capabilities of tangible coach were highly valued by participants. Especially in the face-to-face experiment, seniors' preferences came to the forefront, ranking the tangible coach as their top choice. Qualitative insights highlighted the tangible coach's widespread appreciation for its tactile interaction and hands-free functionality. Many seniors found the tangible coach's interaction to be more intuitive and user-friendly compared with the chatbot. Interestingly, for one-on-one conversations, the use of two interfaces introduced complications, leading participants to perceive this setup as unnecessary. This observation underscores the importance of simplicity and seamless interaction, particularly in personalized settings.
• RQ5: Which output mode is preferred by users?
The rank question provided insightful outcomes. The chatbot exhibits several positive features, including its user-friendly interface, simplicity, and visual aids, enhancing the accessibility and engagement with information. However, it is also noted for its automated nature and time-consuming interactions. Like we saw in our results, some participants were not fond of the chatbot due to its complexity, especially the challenge of typing or pressing buttons that were too small for certain participants' fingers.
Notably, the tangible coach garnered preference over the chatbot, while red-comp and assignment interfaces surpassed their single-interface counterparts. However, the assignment case proved perplexing; users needed time to acclimate to it during face-to-face interactions. A previous study highlighted that the perceived effort required to learn new technology is a significant barrier for older adults' adoption (Kim and Choudhury, 2021). Although this output mode was sometimes confusing to user, a notable aspect was the seniors' expressed desire for control over the adaptation process, underscoring their need for customization and personalization. This insight further accentuates the significance of user control in the design of interfaces for older adults. Adaptive systems were appealing to users, yet older adults ultimately preferred control and the ability to choose, consistent with guidelines for designing embodied conversational agents (El Kamali et al., 2023).
Seniors prominently ranked red-comp as their top choice. This preference aligns with the multifaceted nature of the red-comp system, encompassing both devices and information. Seniors articulated that this configuration empowered them with the choice of information sources, reflecting their persistent desire to influence the agent's responses. Qualitative data delved deeper into this trend, with participants emphasizing the significance of having a sense of control when selecting their most preferred interfaces. This observation underscores the importance of user agency and control in the design of interfaces for older adults (Ghiani et al., 2016). Moreover, the qualitative narratives shed light on the attributes of red-comp, portraying it as not only more enjoyable but also adept at addressing specific disability concerns. These elements likely contribute to the heightened preference for multi-interfaces. However, it is worth noting that the tangible coach frequently stood out as well, especially in the face-to-face study setting, capturing attention due to its human-like attributes, including voice interaction, free hand capabilities, flexibility, and reduced cognitive load. This aligns with the findings by Kim and Choudhury (2021), who investigated vocal assistants' use among older adults and found them to be perceived as user-friendly.
5.1. Emergent findings: web Survey vs. face-to-face
In this section, we delve into the emergent findings that surfaced during the course of our study, shedding light on unanticipated insights and noteworthy observations.
The choice of study settings—online survey and face-to-face experiment—yielded distinct advantages and challenges. The online survey effortlessly attracted 53 participants, indicating its convenience and ability to reach a wider audience. Online platforms facilitated participant recruitment and data collection. In contrast, face-to-face experiments required more effort to find willing participants, with a smaller sample size of 15. The face-to-face experiment extended over a longer period and necessitated traveling of participant.
The nature of the two study settings also impacted the user experience and the quality of interaction. In the face-to-face experiment, participants engaged with tangible devices, fostering a more immersive experience that allowed them to directly perceive social presence and the depth of interaction. This likely influenced their responses, with higher Likert scale scores and a stronger perceived relationship and social presence compared with the online survey. Moreover, in the ranking questionnaire as a companion, we saw that in the face-to-face, the tangible coach was a top 1 choice, whereas we could not observe this result in the web survey. The tactile and tangible nature of the tangible coach enhanced its evaluation during face-to-face interactions, as participants could more easily grasp its usability and benefits.
Despite similar thematic outcomes in both methods, nuanced differences emerged in the evaluation of specific design output modes. Face-to-face interactions led participants to better comprehend the tangible coach's ease of use, conversational capabilities, and the role of vocal interaction, thereby resulting in a more favorable evaluation. The tangible presence of tangible coach seemed to resonate more powerfully in person. Conversely, online surveys lacked references to the importance of redundancy for the learning phase, an aspect highlighted by some face-to-face participants. Three additional themes emerging in the face-to-face sessions were found as well, namely, habits, portability, and error prevention. This divergence might be attributed to users physically engaging with the device, gaining insight into its functionality, portability, and the need for repetition in responses. The preference for complementary information from multiple devices and the desire for control over responses were consistently highlighted across both methods.
An intriguing difference between the two methods was the extent of personal insights shared by participants. While the online survey remained more focused on the study's context, face-to-face participants occasionally divulged personal circumstances that motivated their engagement, such as taking care of parents in need of entertainment. This contextual information, though limited, provided additional layers of understanding and empathy.
Both online surveys and face-to-face experiments offered valuable insights, but their nuances highlight the importance of selecting the appropriate study setting based on research goals, target audience, and resources. Online surveys excelled in terms of participant reach and efficiency, making them a suitable choice when gathering a large amount of data is essential. In contrast, face-to-face experiments facilitated more immersive interactions and deeper insights, making them preferable when a richer understanding of user experience is required. By carefully considering the pros and cons of each method, researchers can tailor their approach to best suit the objectives of their study.
5.2. Limitations and future directions
This study is not without its limitations, primarily stemming from the constraints of the experimental setup. The primary limitation lies in the fact that the experiments were confined to a single scenario. The prevailing circumstances of the COVID-19 pandemic necessitated this approach due to challenges in constructing multiple storyboards with varying scenarios. Expanding to diverse scenarios would have prolonged the survey duration and potentially led to higher dropout rates. During the course of the face-to-face experiment, it became evident that many users expressed a desire to explore different scenarios, particularly in the domain of nutrition. In future endeavors, it would be prudent to test the different output modes across a spectrum of scenarios with older adults.
Additionally, this study focuses on users' perception of output modes, thus excluding an in-depth examination of the architecture and implementation aspects, such as time synchronization and output coordination. Addressing these technical intricacies could offer a more comprehensive view and enhance the robustness of the study's architecture (Palumbo et al., 2020).
A further limitation surfaces in the context of the online web survey. Interpretation of user comments occasionally proved challenging, leading to potential ambiguity in their intended meaning.
To mitigate these limitations, future research could consider the integration of a more interactive storyboard, enabling users to engage with the conversational agent in a live setting. Platforms such as Genially () offer avenues to create interactive scenarios, potentially enhancing the experimental setup. Moreover, a tailored platform accommodating the tangible interactions of the tangible coach could yield more comprehensive results by encompassing touch interactions. This holistic approach would enable a more nuanced understanding of user-agent interactions and preferences.
Furthermore, Based on our results, implementing and assessing the automatic adaptation of interface output modes, such as user preferences and request types and the ability to control the choice of interface, could provide invaluable insights into its impact on user-agent relationships.
6. Conclusion
This study delved into the exploration of diverse output modes involving the tangible coach and the chatbot of Nestore eCoach, a design aimed at enhancing the wellbeing of older adults. Unlike seeking to determine a single superior output mode, the essence of this article resided in comprehending the influence that varying output modes wielded over users' perceptions of a conversational agent as both a coach and a companion. The study looked at different ways the system can work. One way is the “assignment” where the system chooses how to respond. The other is “Redundant-complementary,” where the system gives the same information to both parts and adds specific information for each part. Hence, an output mode model was defined where single-interfaces (using only a chatbot or only a tangible coach) and multi-interfaces (assignment and redundant-complementary) were defined and tested with older adults. The results showed that the ability to control the system and to have multiple interfaces that can complement each other are important factors to take into consideration when combining multiple interfaces. Plus, they are more appreciated than using a chatbot only. Furthermore, the design of multimodal output mode should prioritize user preference and response type. Understanding the unique qualities of each interface mode and their impact on user perception allows for the creation of more engaging and user-centric conversational agents. By carefully considering the balance between redundancy and complementary messages, and offering users the control to choose their preferred interface to interact with, designers can craft interactions that resonate positively with older adults. Expanding the exploration to encompass diverse scenarios within the aforementioned domains could yield a richer understanding of the system's efficacy across varied contexts. Overall, this investigation illuminates the intricate interplay between interface output modes and user perceptions, leading the way to better designs for older adults' wellbeing.
Data availability statement
The datasets presented in this article are not readily available because the dataset will not be generated. Requests to access the datasets should be directed to bWlyYS5lbGthbWFsaUBoZXMtc28uY2g=.
Ethics statement
Ethical approval was not required for the studies involving humans because the study focused on user experience and perception of using the different interfaces. We did not elicit emotions or asked any personal or sensitive questions. Nothing dangerous was done. The studies were conducted in accordance with the local legislation and institutional requirements. The participants provided their written informed consent to participate in this study. Written informed consent was obtained from the individual(s) for the publication of any potentially identifiable images or data included in this article.
Author contributions
All authors listed have made a substantial, direct, and intellectual contribution to the work and approved it for publication.
Funding
This project has been supported by the European Commission under the Horizon 2020 program, SC1-PM-15-2017 - Personalised Medicine topic, through the project Grant No. 769643.
Conflict of interest
The authors declare that the research was conducted in the absence of any commercial or financial relationships that could be construed as a potential conflict of interest.
The reviewer ZC declared a past co-authorship with the authors ME, LA to the handling editor.
Publisher's note
All claims expressed in this article are solely those of the authors and do not necessarily represent those of their affiliated organizations, or those of the publisher, the editors and the reviewers. Any product that may be evaluated in this article, or claim that may be made by its manufacturer, is not guaranteed or endorsed by the publisher.
References
(2022). Genially. Available online at: https://genial.ly/fr/
Andreoni, G., and Mambretti, C. (2021). Digital Health Technology for Better Aging. Germany: Springer.
Angelini, L., Caon, M., Michielan, E., Khaled, O. A., and Mugellini, E. (2021). “Seniors' perception of smart speakers: challenges and opportunities elicited in the silver&home living lab,” in Congress of the International Ergonomics Association (Cham: Springer), 137–144.
Angelini, L., El Kamali, M., Mugellini, E., Abou Khaled, O., Röcke, C., Porcelli, S., et al. (2022). The nestore e-coach: designing a multi-domain pathway to well-being in older age. Technologies 10, 50. doi: 10.3390/technologies10020050
Aust, F., Diedenhofen, B., Ullrich, S., and Musch, J. (2013). Seriousness checks are useful to improve data validity in online research. Behav. Res. Methods 45, 527–535. doi: 10.3758/s13428-012-0265-2
Bartneck, C. (2002). eMuu-an Embodied Emotional Character for the Ambient Intelligent Home. Eindhoven: Technische Universiteit Eindhoven.
Bickmore, T. W., Caruso, L., Clough-Gorr, K., and Heeren, T. (2005). ‘It's just like you talk to a friend' relational agents for older adults. Interact. Comput. 17, 711–735. doi: 10.1016/j.intcom.2005.09.002
Bickmore, T. W., Silliman, R. A., Nelson, K., Cheng, D. M., Winter, M., Henault, L., et al. (2013). A randomized controlled trial of an automated exercise coach for older adults. J. Am. Geriatr. Soc. 61, 1676–1683. doi: 10.1111/jgs.12449
Black, J. T., Romano, P. S., Sadeghi, B., Auerbach, A. D., Ganiats, T. G., Greenfield, S., et al. (2014). A remote monitoring and telephone nurse coaching intervention to reduce readmissions among patients with heart failure: study protocol for the better effectiveness after transition-heart failure (beat-HF) randomized controlled trial. Trials 15, 1–11. doi: 10.1186/1745-6215-15-124
Blusi, M., Nilsson, I., and Lindgren, H. (2018). “Older adults co-creating meaningful individualized social activities online for healthy ageing,” in Building Continents of Knowledge in Oceans of Data: The Future of Co-Created eHealth (IOS Press), 775–779.
Callejas, Z., Griol, D., McTear, M. F., and López-Cózar, R. (2014). “A virtual coach for active ageing based on sentient computing and m-health,” in International Workshop on Ambient Assisted Living (Cham: Springer), 59–66.
Chipidza, F. E., Wallwork, R. S., and Stern, T. A. (2015). Impact of the doctor-patient relationship. Primary Care Comp. CNS Disord. 17, 27354. doi: 10.4088/PCC.15f01840
Cuba, P. F. Q. (2010). Migração de agentes entre corpos e plataformas (agent migration between bodies and platforms).
Dumas, B., Lalanne, D., and Oviatt, S. (2009). “Multimodal interfaces: a survey of principles, models and frameworks,” in Human Machine Interaction (Springer), 3–26.
Eisinga, R., Grotenhuis, M. t., and Pelzer, B. (2013). The reliability of a two-item scale: Pearson, cronbach, or spearman-brown? Int. J. Public Health 58, 637–642. doi: 10.1007/s00038-012-0416-3
El Kamali, M., Angelini, L., Caon, M., Carrino, F., Röcke, C., Guye, S., et al. (2020a). Virtual coaches for older adults' wellbeing: a systematic review. IEEE Access 8, 101884–101902. doi: 10.1109/ACCESS.2020.2996404
El Kamali, M., Angelini, L., Caon, M., Dulake, N., Chamberlain, P., Craig, C., et al. (2023). Co-designing an embodied e-coach with older adults: the tangible coach journey. Int. J. Hum. Comput. Interact. 1–24. doi: 10.1080/10447318.2023.2171332
El Kamali, M., Angelini, L., Caon, M., Lalanne, D., Abou Khaled, O., and Mugellini, E. (2020b). “An embodied and ubiquitous e-coach for accompanying older adults towards a better lifestyle,” in International Conference on Human-Computer Interaction (IEEE: Springer), 23–35.
El Kamali, M., Angelini, L., Lalanne, D., Abou Khaled, O., and Mugellini, E. (2020c). “Multimodal conversational agent for older adults' behavioral change,” in Companion Publication of the 2020 International Conference on Multimodal Interaction (New York, NY), 270–274.
Ghiani, G., Manca, M., Paternò, F., and Santoro, C. (2016). “End-user personalization of context-dependent applications in AAL scenarios,” in Proceedings of the 18th International Conference on Human-Computer Interaction with Mobile Devices and Services Adjunct (New York, NY), 1081–1084.
Ghosh, S., and Joshi, A. (2013). “Exploration of multimodal input interaction based on goals,” in Proceedings of the 11th Asia Pacific Conference on Computer Human Interaction (New York, NY), 83–92.
Gomes, P. F., Sardinha, A., Márquez Segura, E., Cramer, H., and Paiva, A. (2014). Migration between two embodiments of an artificial pet. Int. J. Human. Robot. 11, 1450001. doi: 10.1142/S0219843614500017
Himmelsbach, J., Garschall, M., Egger, S., Steffek, S., and Tscheligi, M. (2015). “Enabling accessibility through multimodality? interaction modality choices of older adults,” in Proceedings of the 14th International Conference on Mobile and Ubiquitous Multimedia (New York, NY), 195–199.
Imai, M., Ono, T., and Etani, T. (1999). “Agent migration: communications between a human and robot,” in IEEE SMC'99 Conference Proceedings. 1999 IEEE International Conference on Systems, Man, and Cybernetics (Cat. No. 99CH37028) (IEEE), 1044–1048.
Jian, C., Shi, H., Sasse, N., Rachuy, C., Schafmeister, F., Schmidt, H., et al. (2013). “Modality preference in multimodal interaction for elderly persons,” in International Joint Conference on Biomedical Engineering Systems and Technologies (Berlin; Heidelberg: Springer), 378–393.
Jowett, S., and Ntoumanis, N. (2004). The coach–athlete relationship questionnaire (CART-Q): development and initial validation. Scand. J. Med. Sci. Sports 14, 245–257. doi: 10.1111/j.1600-0838.2003.00338.x
Kim, S., and Choudhury, A. (2021). Exploring older adults' perception and use of smart speaker-based voice assistants: a longitudinal study. Comput. Hum. Behav. 124, 106914. doi: 10.1016/j.chb.2021.106914
Lombard, M., and Ditton, T. (1997). At the heart of it all: the concept of presence. J. Comput. Mediat. Commun. 3, JCMC321.
Loveys, K., Sebaratnam, G., Sagar, M., and Broadbent, E. (2020). The effect of design features on relationship quality with embodied conversational agents: a systematic review. Int. J. Soc. Robot. 12, 1293–1312. doi: 10.1007/s12369-020-00680-7
Luria, M., Reig, S., Tan, X. Z., Steinfeld, A., Forlizzi, J., and Zimmerman, J. (2019). “Re-embodiment and co-embodiment: exploration of social presence for robots and conversational agents,” in Proceedings of the 2019 on Designing Interactive Systems Conference (New York, NY), 633–644.
Merrill, K. Jr., Kim, J., and Collins, C. (2022). AI companions for lonely individuals and the role of social presence. Commun. Res. Rep. 39, 93–103. doi: 10.1080/08824096.2022.2045929
Nigay, L., and Coutaz, J. (1997). Multifeature systems: the care properties and their impact on software design. Intell. Multimodal. Multimedia Interfaces.
Ogawa, K., and Ono, T. (2005). “Ubiquitous cognition: mobile environment achieved by migratable agent,” in Proceedings of the 7th International Conference on Human Computer Interaction With Mobile Devices & Services (New York, NY), 337–338.
Palumbo, F., Crivello, A., Furfari, F., Girolami, M., Mastropietro, A., Manferdelli, G., et al. (2020). “Hi this is nestore, your personal assistant”: design of an integrated iot system for a personalized coach for healthy aging. Front. Digit. Health 2, 545949. doi: 10.3389/fdgth.2020.545949
Reeves, B., and Nass, C. (1996). The Media Equation: How People Treat Computers, Television, and New Media Like Real People. Cambridge, UK: Cambridge University Press.
Schaffer, S., Schleicher, R., and Möller, S. (2015). Modeling input modality choice in mobile graphical and speech interfaces. Int. J. Hum. Comput. Stud. 75, 21–34. doi: 10.1016/j.ijhcs.2014.11.004
Schrepp, M., Hinderks, A., and Thomaschewski, J. (2017). Design and evaluation of a short version of the user experience questionnaire (UEQ-S). Int. J. Interact. Multimedia Artif. Intell. 4, 103–108. doi: 10.9781/ijimai.2017.09.001
Schüssel, F., Honold, F., and Weber, M. (2013). Influencing factors on multimodal interaction during selection tasks. J. Multimodal User Interfaces 7, 299–310. doi: 10.1007/s12193-012-0117-5
Shin, D.-H., and Choo, H. (2011). Modeling the acceptance of socially interactive robotics: social presence in human–robot interaction. Interact. Stud. 12, 430–460. doi: 10.1075/is.12.3.04shi
Sidner, C. L., Bickmore, T., Nooraie, B., Rich, C., Ring, L., Shayganfar, M., et al. (2018a). Creating new technologies for companionable agents to support isolated older adults. ACM Trans. Interact. Intell. Syst. 8, 1–27. doi: 10.1145/3213050
Sidner, C. L., Bickmore, T., Nooraie, B., Rich, C., Ring, L., Shayganfar, M., et al. (2018b). Creating new technologies for companionable agents to support isolated older adults. ACM Trans. Interact. Intell. Syst. 8, 1–27.
Sinoo, C., van Der Pal, S., Henkemans, O. A. B., Keizer, A., Bierman, B. P., Looije, R., et al. (2018). Friendship with a robot: children's perception of similarity between a robot's physical and virtual embodiment that supports diabetes self-management. Patient Educ. Counsel. 101, 1248–1255. doi: 10.1016/j.pec.2018.02.008
Startsteite (2022). Unipark. Available online at: https://www.unipark.com/en/
Steuer, J. (1992). Defining virtual reality: dimensions determining telepresence. J. Commun. 42, 73–93.
Tejwani, R. (2020). Migratable AI (Ph.D. thesis). Massachusetts Institute of Technology, Cambridge, MA, United States.
Toader, D.-C., Boca, G., Toader, R., Măcelaru, M., Toader, C., Ighian, D., et al. (2019). The effect of social presence and chatbot errors on trust. Sustainability 12, 256. doi: 10.3390/su12010256
Vernier, F., and Nigay, L. (2000). “A framework for the combination and characterization of output modalities,” in International Workshop on Design, Specification, and Verification of Interactive Systems (Berlin, Heidelberg: Springer), 35–50.
Keywords: older adults, conversational agents, multimodal interaction, output modes, combination of interfaces, multiple interfaces
Citation: El Kamali M, Angelini L, Lalanne D, Abou Khaled O and Mugellini E (2023) Older adults' perspectives on multimodal interaction with a conversational virtual coach. Front. Comput. Sci. 5:1125895. doi: 10.3389/fcomp.2023.1125895
Received: 16 December 2022; Accepted: 25 September 2023;
Published: 07 November 2023.
Edited by:
Iulia Lefter, Delft University of Technology, NetherlandsReviewed by:
Zoraida Callejas, University of Granada, SpainSanjana Mendu, The Pennsylvania State University (PSU), United States
Janet Wessler, German Research Center for Artificial Intelligence (DFKI), Germany
Copyright © 2023 El Kamali, Angelini, Lalanne, Abou Khaled and Mugellini. This is an open-access article distributed under the terms of the Creative Commons Attribution License (CC BY). The use, distribution or reproduction in other forums is permitted, provided the original author(s) and the copyright owner(s) are credited and that the original publication in this journal is cited, in accordance with accepted academic practice. No use, distribution or reproduction is permitted which does not comply with these terms.
*Correspondence: Leonardo Angelini, bGVvbmFyZG8uYW5nZWxpbmlAaGVzLXNvLmNo