- Human-Computer Interaction Center, RWTH Aachen University, Aachen, Germany
Introduction: Artificial Intelligence (AI) has become ubiquitous in medicine, business, manufacturing and transportation, and is entering our personal lives. Public perceptions of AI are often shaped either by admiration for its benefits and possibilities, or by uncertainties, potential threats and fears about this opaque and perceived as mysterious technology. Understanding the public perception of AI, as well as its requirements and attributions, is essential for responsible research and innovation and enables aligning the development and governance of future AI systems with individual and societal needs.
Methods: To contribute to this understanding, we asked 122 participants in Germany how they perceived 38 statements about artificial intelligence in different contexts (personal, economic, industrial, social, cultural, health). We assessed their personal evaluation and the perceived likelihood of these aspects becoming reality.
Results: We visualized the responses in a criticality map that allows the identification of issues that require particular attention from research and policy-making. The results show that the perceived evaluation and the perceived expectations differ considerably between the domains. The aspect perceived as most critical is the fear of cybersecurity threats, which is seen as highly likely and least liked.
Discussion: The diversity of users influenced the evaluation: People with lower trust rated the impact of AI as more positive but less likely. Compared to people with higher trust, they consider certain features and consequences of AI to be more desirable, but they think the impact of AI will be smaller. We conclude that AI is still a “black box” for many. Neither the opportunities nor the risks can yet be adequately assessed, which can lead to biased and irrational control beliefs in the public perception of AI. The article concludes with guidelines for promoting AI literacy to facilitate informed decision-making.
1. Introduction
Artifical Intelligence (AI), Deep Neural Networks (DNN) and Machine Learning (ML) are the buzzwords of the moment. Although the origins of AI and ML date back decades, they have received a tremendous boost in recent years due to increased computing power, more available digital data, improved algorithms and a substantial increase in funding (Lecun et al., 2015; Statista, 2022).
While we are still a long way from Artificial General Intelligence (AGI) (“strong AI”)—referring to an AI that matches human intelligence, and can adapt as well as transfer learning to new tasks (Grace et al., 2018)—it is undeniable that even “weak AI” and ML that focus on narrow tasks already have a huge impact on individuals, organizations and our societies (West, 2018). While the former, aims at recreating human-like intelligence and behavior, the latter is applied to solve specific and narrowly defined tasks, such as image recognition, medical diagnosis, weather forecasts, or automated driving (Flowers, 2019). Interestingly, recent advancements in AI and its resulting increase in media coverage, can be explained by the progress in the domain of weak AI, like faster and more reliable image recognition, translation, text comprehension through DNNs and their sub types (Vaishya et al., 2020; Statista, 2022), as well as image or text generation (Brown et al., 2020). Despite the tremendous progress in weak AI in the recent years, AI still has considerable difficulties in transferring its capabilities to other problems (Binz and Schulz, 2023). However, public perceptions of AI are often shaped by science fiction characters portrayed as having strong AI, such as Marvin from The Hitchhiker's Guide to the Galaxy, Star Trek's Commander Data, the Terminator, or HAL 9000 from Space Odyssey (Gunkel, 2012; Gibson, 2019; Hick and Ziefle, 2022). These depictions can influence the public discourse on AI and skew it into an either overly expectant or unwarranted pessimistic narrative (Cugurullo and Acheampong, 2023; Hirsch-Kreinsen, 2023).
Much research has been done on developing improved algorithms, generating data, labeling for supervised learning, and studying the economic impact of AI on organizations (Makridakis, 2017; Lin, 2023), the workforce (Acemoglu and Restrepo, 2017; Brynjolfsson and Mitchell, 2017), and society (Wolff et al., 2020; Floridi and Cowls, 2022; Jovanovic et al., 2022). However, despite an increased interest in the public perception of AI (Zuiderwijk et al., 2021), it is essential to regularly update these academic insights. Understanding the individual perspective plays a central part since the adoption and diffusion of new technologies such as AI and ML can be driven by greater acceptance or significantly delayed by perceived barriers (Young et al., 2021).
In this article, we present a study in which we measured novices' expectations and evaluations of AI. Participants assessed the likelihood that certain AI related developments will occur and whether their feelings about these developments are positive or negative. In this way, we identify areas where expectations and evaluations are aligned, as well as areas where there are greater differences and potential for conflict. Since areas of greater disparity can hinder social acceptance (Slovic, 1987; Kelly et al., 2023), they need to be publicly discussed. Based on accessible and transparent information about AI and a societal discourse about its risks and benefits, these discrepancies can either be reduced or regulatory guidelines for AI can be developed.
A result of this study is a spatial criticality map for AI-based technologies that 1) can guide developers and implementers of AI technology with respect to socially critical aspects, 2) can guide policy making regarding specific areas in need of regulation, 3) inform researchers about areas that could be addressed to increase social acceptance, and 4) identify relevant points for school and university curricula to inform future generations about AI.
The article is structured as follows: Section 2 defines our understanding of AI and reviews recent developments and current projections on AI. Section 3 presents our approach to measuring people's perceptions of AI and the sample of our study. Section 4 presents the results of the study and concludes with a criticality map of AI technology. Finally, Section 5 discusses the findings, the limitations of this work, and concludes with suggestions on how our findings can be used by others.
2. Related work
This section first presents some of the most commonly used definitions of AI and elaborates on related concepts. It then presents studies in the field of AI perception and identifies research gaps.
2.1. Overview on AI
Definitions of Artifical Intelligence (AI) are as diverse as research on AI. The term AI was coined during the “Dartmouth workshop on Artificial Intelligence” in 1955. During that year's summer, the proposed definition of Artifical Intelligence (AI) was that “every aspect of learning or any other feature of intelligence can in principle be so precisely described that a machine can be made to simulate it” (McCarthy et al., 2006). In the year 1955—almost 70 years ago—researchers were convinced that—within a 2 month period—these machines would understand language, use abstract concepts, and could improve themselves. It was an ambitious goal that was followed by even more ambitious research directions and working definitions for AI.
AI is a branch of computer science that deals with the creation of intelligent machines that can perform tasks that typically require human intelligence, such as visual perception, speech recognition, decision-making, and language translation (Russell and Norvig, 2009; Marcus and Davis, 2019). ML, conversely, is a subset of AI that focuses on the development of algorithms and statistical models that enable machines to improve their performance on a specific task over time by learning from data, without being explicitly programmed.
A central introductory textbook on AI by Russel and Norvig defines it as “the designing and building of intelligent agents that receive percepts from the environment and take actions that affect that environment” (Russell and Norvig, 2009). The Cambridge Dictionary takes a somewhat different angle by defining AI as “the study of how to produce computers that have some of the qualities of the human mind, such as the ability to understand language, recognize pictures, solve problems, and learn” or as “computer technology that allows something to be done in a way that is similar to the way a human would do it” (Cambridge Dictionary, 2022). This kind of AI approximates the human mind and is built into a computer which is then used to solve some form of complex problem. On the one hand, this approach serves us with a well-defined line of events: We have a problem, develop a solution and, hopefully, will be able to solve the initial problem. The machine's job, or more precisely, an AI's job would be to find a solution, that is, give an answer to our question. On the other hand, this approach is rather narrow in scope. As Pablo Picasso famously commented in an interview for the Paris Review in 1964 “[Computers1] are useless. They can only give you answers.” Picasso wanted to convey that a computer, or an AI for that matter, can only present outputs specific to an input, i.e., a specific answer to a specific question. However, it is currently beyond the capabilities of any AI algorithm to transfer its “knowledge” to any previously unseen problem and excel at solving it (Binz and Schulz, 2023). This is why there are many algorithms and many AI models, one for each particular problem. Going back to definitions—at least today—there is no single universal definition that captures the essence of AI.
Current AI research focuses on automating cognitive work that is often repetitive or tiring (Fosso Wamba et al., 2021). Its aim is to provide technological solutions to an otherwise inefficient or less efficient way of working. However, there are many other areas of (potential) AI applications that are merely an extension of what the human mind can do, such as creativity. In a recent example, a AI-based art generator won a prestigious art competition in the USA. In this case, the piece of art was entitled The death of art and received a mixed reception on Twitter, with some people fearing for their jobs, which may soon be replaced by a machine (Jumalon, 2022).
Many research articles focus on workers' perceptions of machine labor and its potential to replace some aspect of their work (Harari, 2017). In most cases, the machine is not a replacement, but rather an addition to the workforce (Topol, 2019). However, fear of replacement still exists among people working in jobs that are particularly easy to automate, such as assembly line work, customer service or administrative tasks (Smith and Anderson, 2014). A recent study found that workers' level of fear of being replaced did not significantly affect their level of preparation for this potential replacement, such as acquiring new skills. Furthermore, appreciation of the new technology and perceived opportunity positively influenced workers' attitudes toward automation (Rodriguez-Bustelo et al., 2020). This is just one example of the importance of perception of e.g., a new technology, and consequently its understanding, to accurately judge its implications.
Some form of AI is now used in almost all areas of technology, and it will continue to spread throughout society (Grace et al., 2018; Almars et al., 2022). Current application areas include voice assistants, automatic speech recognition, translation, and generation that can exceed the human performance (Corea, 2019), automated driving and flying (Klos et al., 2020; Kulida and Lebedev, 2020), and medical technologies (areas where AI could touch our personal lives) (Klos et al., 2020; Jovanovic et al., 2022), as well as production control (Brauner et al., 2022), robotics and human-robot interaction (Onnasch and Roesler, 2020; Robb et al., 2020), human resource management, and prescriptive machine maintenance (areas where AI could touch our professional lives).
We suspect that the perception of the benefits and potential risks of AI is influenced by the application domain and thus that the evaluation of AI cannot be separated from its context. For example, AI-based image recognition is used to evaluate medical images for cancer diagnosis (Litjens et al., 2017) or to provide autonomously driving cars with a model of their surroundings (Rao and Frtunikj, 2018). Therefore, people's perception of AI and its implications will depend less on the underlying algorithms and more on contextual factors.
2.2. Studies on human perception of AI
As outlined in the section above, perceptions of AI can be influenced not only by the diversity of end users (Taherdoost, 2018; Sindermann et al., 2021), but also by contextual influences. As an example from the context of automated driving, Awad et al. used an instance of Foot's Trolly dilemma (Foot, 1967) to study how people would prefer a AI-controlled car to react in the event of an unavoidable crash (Awad et al., 2018). In a series of decision tasks, participants had to decide if the car should rather kill a varying number of involved pedestrians or its car passengers. The results show that, for example, sparing people is preferred to sparing animals, sparing more people is preferred over sparing fewer people and, to a lesser extend, pedestrians are preferred to passengers. The article concludes that consideration of people's perceptions and preferences, combined with ethical principles, should guide the behavior of these autonomous machines.
In a different study (Araujo et al., 2020) examined the perceived usefulness of AI in three contexts (media, health, and law). As opposed to the automated driving example, their findings suggest that people are generally concerned about the risks of AI and question its fairness and usefulness for society. This means that in order to achieve appropriate and widespread adoption of AI technology, end-user perceptions and risk assessments should be taken into account at both the individual and societal levels.
In line with this claim, another study has investigated whether people assign different levels of trust to human, robotic or AI-based agents (Oksanen et al., 2020). In this study, the researchers investigated the extent to which participants would trust either an AI-based agent or a robot with their fictitious money during a so-called trust game, and whether the name of the AI-based agent or robot would have an influence on this amount of money. The results showed that the most trusted agent was a robot with a non-human name, and the least trusted i.e., the agents was given the least amount of money, was an unspecified control (meaning that it was not indicated if it was human or not) named Michael. The researchers concluded that people would trust a sophisticated technology more in a context where this technology had to be reliable in terms of cognitive performance and fairness. They also concluded that, from the Big Five personality model (McCrae and Costa, 1987), the dimension Openness was positively, and Conscientiousness negatively related to the attributed trust. The study provided support for the theory that higher levels of education, previous exposure to robots, or higher levels of self-efficacy in interacting with robots may influence levels of trust in these technologies.
In addition to this angle, the domain of implementation of AI, i.e., the role it takes on in a given context, was explored (Philipsen et al., 2022). Here, the researchers investigated what the roles of an AI are and how an AI has to be designed in order to fulfill the expected roles. On the one hand, the results show that people do not want to have a personal relationship with an AI, e.g., an AI as a friend or partner. On the other hand, the diversity of the users influenced the evaluation of the AI. That is, the higher the trust in an AI's handling of data, the more likely personal roles of AI were seen as an option. Preference for subordinate roles, such as an AI as a servant, was associated with general acceptance of technology and a belief in a dangerous world. Thus, subordinate roles were preferred when participants believed that the world we live in is more dangerous than it is not. However, the attribution of roles was independent of the intention to use AI. Semantic perceptions of AI also differed only slightly from perceptions of human intelligence, e.g., in terms of morality and control. This supports our claim that initial perceptions of e.g., AI can influence subsequent evaluations and both, potentially and ultimately, AI adoption.
With AI becoming an integral part of lives as personal assistants (Alexa, Siri, …) (Burbach et al., 2019), large language models (ChatGPT, LaMDA, …), smart shopping lists, and the smart home (Rashidi and Mihailidis, 2013), end-user perception and evaluation fo these technologies becomes increasingly important (Wilkowska et al., 2018; Kelly et al., 2023). This is also evident in professional contexts, where AI is used—for example—in medical diagnosis (Kulkarni et al., 2020), health care (Oden and Witt, 2020; Jovanovic et al., 2022), aviation (Klos et al., 2020; Kulida and Lebedev, 2020), and production control (Brauner et al., 2022). The continued development of increasingly sophisticated AI can lead to profound changes for individuals, organizations and society as a whole (Bughin et al., 2018; Liu et al., 2021; Strich et al., 2021).
However, the assessment of the societal impact of a technology in general, and the assessment of AI in particular, is a typical case of the Collingridge (1982): These are developments that are either difficult to predict if they do not exist, or difficult to manage and regulate if they are already ubiquitous. On the one hand, if the technology is sufficiently developed and available, it can be well evaluated, but by then it is often too late to regulate the development. On the other hand, if the technology is new and not yet pervasive in our lives, it is difficult to assess its perception and potential impact, but it is easier to manage its development and use. Responsible research and innovation requires us to constantly update our understanding of the societal evaluations and implications as technologies develop (Burget et al., 2017; Owen and Pansera, 2019). Here, we aim to update our understanding of the social acceptability of AI and to identify any need for action (Owen et al., 2012).
3. Method
Above, we briefly introduced the term AI, showed that AI currently involves numerous areas of our personal and professional lives, and outlined studies on the perception of AI. The present study is concerned with laypersons' perceptions, their assessment of an AI development and its expected likelihood of actually happening. Thus, our approach is similar to the Delphi method, where (expert) participants are asked to make projections about future developments (Dalkey and Helmer, 1963), by aggregating impartial reflections of current perceptions into insights about technology adoption and technology foresight.
To assess perceptions of AI, we used a two-stage research model. In the first stage of our research, topics were identified in an expert workshop to get an accurate list. Then, these topics were rated by a convenient sample in the manner described above. This approach for studying laypeople's perception of AI will be further discussed later in the article.
3.1. Identification of the topics
To develop the list of topics we conducted a three-stage expert workshop with four experts in the field of technology development and technology forecasting. In the first stage, we brainstormed possible topics. In the second stage, similar topics were grouped and then the most relevant topics were selected, resulting 38 topics. In the third and final stage, the labels of the 38 defined topics were reworded so that they could be easily understood by the participants in the survey which followed.
3.2. Survey
We designed an online survey to assess non-experts' perceptions of AI. It consisted of two main parts: First, we asked about the participant's demographics and additional explanatory factors (see below). Second, we asked participants about numerous aspects and whether they thought the given development was likely to occur (i.e., Will this development happen?), and, as a measure of acceptability (Kelly et al., 2023), how they personally evaluated this development (i.e., Do you think this development is good?). Overall, we asked about the expectation (likelihood) and evaluation (valence) of 38 different aspects, ranging from the influence on the personal and professional life, to the perceived impact of AI on the economy, healthcare, and culture, as well as wider societal implications. The questionnaire was administered in German and the items were subsequently translated into English for this article. Figure 1 illustrates the research approach and the structure of the survey. Table 2 lists all statements from the AI scenarios.
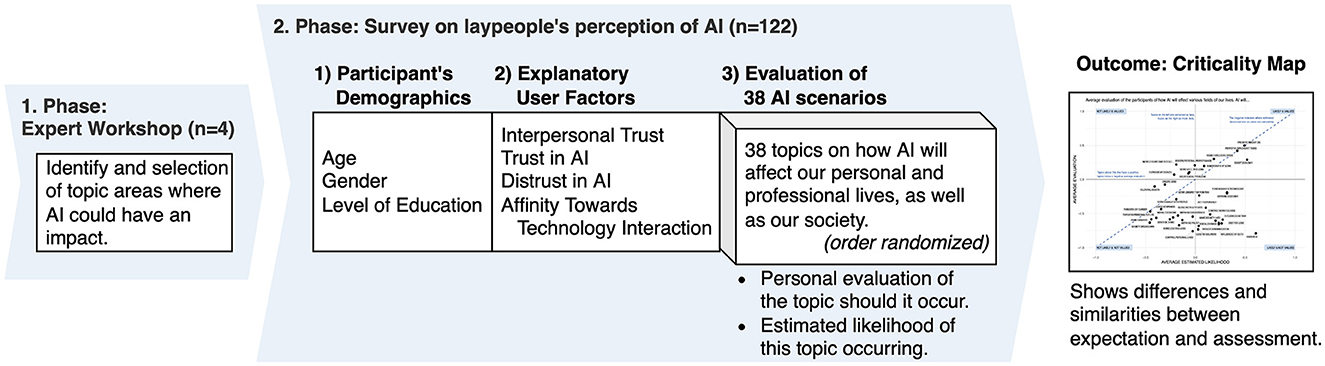
Figure 1. Multi-stage research design of this study with expert workshop and subsequent survey study. The questionnaire captures demographics, exploratory user factors and the evaluation of the 38 AI-related scenarios.
3.3. Demographics and explanatory user factors
In order to investigate possible influences of user factors (demographics, attitudes) on the expectation and evaluation of the scenarios, the survey started with a block asking for demographic information and attitudes of the participants. Specifically, we asked participants about their age in years, their gender and their highest level of education. We then asked about the following explanatory user factors that influenced the perception and evaluation of technology in previous studies. We used 6-point Likert-scales to capture the explanatory user factors (ranging from 1 to 6). Internal reliability was tested using Cronbach's alpha (Cronbach, 1951).
Affinity for Technology Interaction refers to a person's “tendency to actively engage in intensive technology interaction” (Franke et al., 2019) and is associated with a positive basic attitude toward various technologies and presumably also toward AI. We used five items with the highest item-total-correlation. The scale achieved excellent internal reliability (α = 0.804, n = 122, 5 items).
Trust is an important prerequisite for human coexistence and cooperation (Mc Knight et al., 2002; Hoff and Bashir, 2015). Mayer et al. (1995) defined trust as “the willingness of a party to be vulnerable to another party.” As technology is perceived as social actor (Reeves and Nass, 1996), trust is also relevant to the acceptance and use of digital products and services. We used three scales to measure trust: First, we measured interpersonal trust using the psychometrically well validated KUSIV3 short scale with three items (excellent internal reliability, α = 0.829) (Beierlein, 2014). The scale measures the respondent's trust in other people. Secondly and thirdly, we developed two short scales with three items each to specifically model trust in AI and distrust in AI. Both scales achieved an acceptable internal reliability of α = 0.629 (trust in AI) and α = 0.634 (distrust in AI).
3.4. Perception of artificial intelligence
We asked about various topics in which AI already plays or could play a role in the future. The broader domains ranged from implications for the individual, over economical and societal changes, to questions of governance. Some of the topics were more straightforward and others rather far-flung.
For each of the 38 topics, we asked the participants whether this development is likely or not (likelihood) and if they evaluate this development as positive or negative (evaluation). Table 3 presents these topics that ranged from “AI will promote innovation,” over “AI will create significant cultural assets,” to “AI will lead to the downfall of society.”
The questionnaire displayed the items in three columns: The item text on the left and two Likert scales to query the participants' expected likelihood and evaluation should the development come true on the right. The order of the items was randomized across the participants to compensate for question order biases. We used 4-point Likert scales to measure the expected likelihood of occurrence and evaluation of the given statements.
3.5. Survey distribution and data analysis
The link to the survey was distributed via email, messaging services, and social-networks. We checked that none of the user factors examined were correlated with not completing the survey and found no systematic bias. We therefore consider the dataset of 122 samples in the following.
We examined the dataset using the social sciences portfolio of methods (Dienes, 2008). To assess the association between the variables, we analyzed the data using non-parametric (Spearman's ρ) and parametric correlations (Pearson's r), setting the significance level at 5% (α = 0.05). We used Cronbach's α to test the internal consistency of the explanatory user factors and, where permitted, calculated the corresponding scales. As there is no canonical order for the statements on the AI developments, we did not recode the values. We calculated mean scores (M) and the standard deviation (SD) for likelihood and evaluation for both the 38 developments (individually for each topic across all participants; vertical in the dataset) and for each participant (individually for each participant across all topics; horizontal in the dataset). The former gives the sample's average assessment of each topic, while the latter is an individual measure of how likely and how positive the participants consider the questioned developments are in general.
3.6. Description of the sample
In total, 122 people participated in the survey. Forty one identified themselves as men, 81 as women, and no one stated “diverse” or refused to answer. The age ranged from 18 to 69 years (M = 33.9, SD = 12.8). In the sample, age was neither associated with Affinity Toward Technology Interaction, nor with any of the three trust measures (p > 0.05). Gender was associated to Affinity Toward Technology Interaction (r = −0.381, p < 0.001), with men, on average, reporting higher attitudes toward interacting with technology. Interpersonal Trust is associated to higher Trust in AI (r = 0.214, p = 0.018), but not to higher distrust in AI (p = 0.379). Not surprisingly, there is a negative relationship between trust and distrust in AI (r = −0.386, p < 0.01. People who have more trust in AI report less distrust and vice versa. Finally, Affinity Toward Technology Interaction is related to both trust in AI (r = 0.288, p = 0.001 and (negatively) to distrust in AI (r = −0.280, p = 0.002). Table 1 shows the correlations between the (explanatory) user factors in the sample.
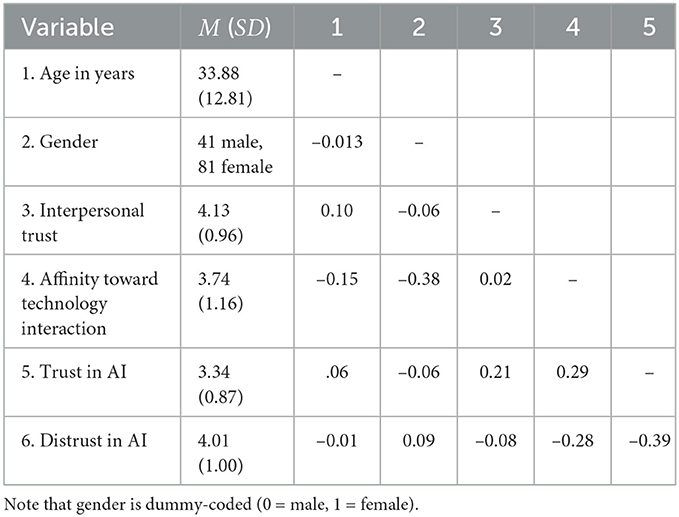
Table 1. Descriptive statistics and correlations of the (explanatory) user factors in the sample of 122 participants.
4. Results
First, we analyse how participants evaluate the different statements on AI and map these statements spatially. Figure 2 shows a scatter plot of the participants' average estimated probability of occurrence and their average rating for each of the 38 topics in the survey. Each individual point in the figure represents the evaluation of one topic. The position of the points on the horizontal axis represents the estimated likelihood of occurrence, with topics rated as more likely to occur further to the right of the figure. The position on the vertical axis shows the rating of the statement, with topics rated as more positive appearing higher on the graph. Table 2 shows the individual statements and their ratings.
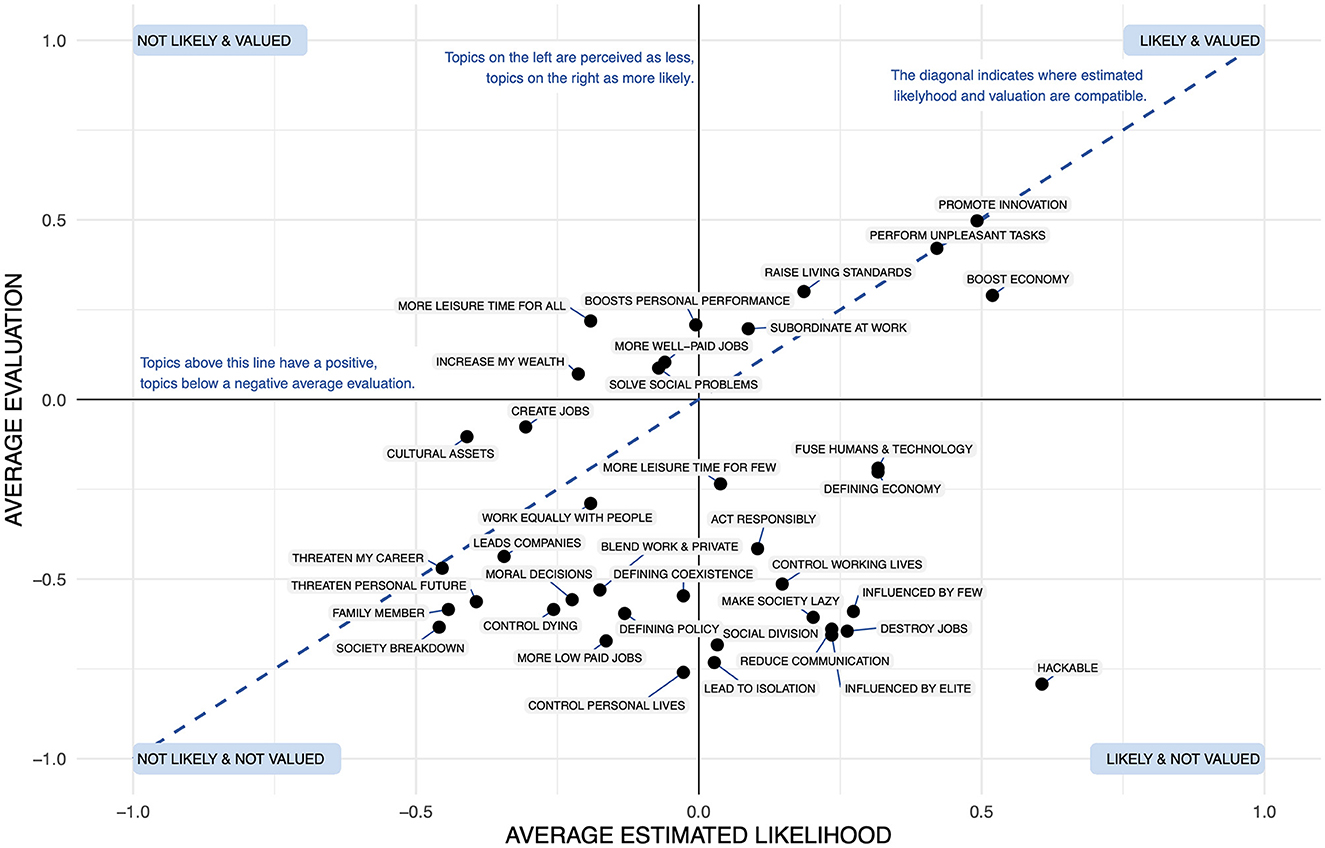
Figure 2. Criticality map showing the relationship between estimated likelihood and evaluations for the AI predictions.
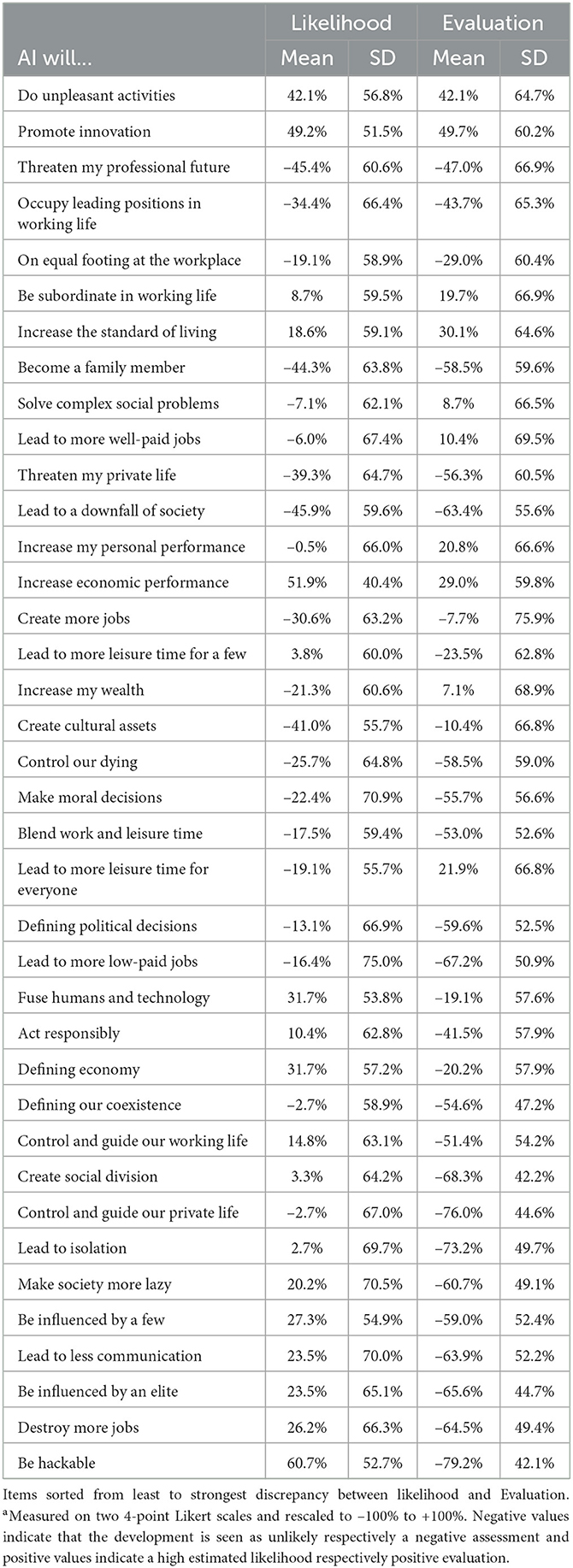
Table 2. The participants' estimated likelihood (Likelihood) of occurrence and subjective assessment (Evaluation) of the various consequences AI could have on our lives and worka.
The resulting graph can be interpreted as a criticality map and read as follows: In the upper left corner are those aspects that were rated as positive but unlikely. The upper right corner shows statements that were rated as both positive and likely. The lower right corner contains statements that were rated as negative but likely. Finally, the lower right corner contains statements that were perceived as both negative and unlikely. Second, dots on or near the diagonal represent aspects where the perceived occurrence is consistent with the personal rating of the aspect: These aspects are either perceived as likely and positive (e.g., “promote innovation” or “do unpleasant activities” or as unlikely and negative (e.g., “occupy leading positions in working life” or “threaten my professional future”). On the other hand, for points off the diagonal, expectations and evaluations diverge. The future is either seen as probable and negative (e.g., “be hackable” or “be influenced by a few”), or as unlikely and positive (e.g., “create cultural assets” or “lead to more leisure time for everyone”).
Accordingly, three sets of points deserve particular attention. Firstly, the points in the bottom half of the graph, as these are seen as negative by the participants. This is where future research and development should take people's concerns into account. Secondly, the points in the upper left quadrant of the graph, as these are considered positive but unlikely. These points provide insight into where participants perceive research and implementation of AI to fall short of what they want. Finally, all items where there is a large discrepancy between the likelihood of occurrence and the assessment (off the diagonal), as these items are likely to lead to greater uncertainty in the population.
As the figure shows, for some of the statements the estimated likelihood of occurrence is in line with the participants' personal assessment, while for others there is a strong divergence. The statements with the highest agreement were that AI will support the performance of unpleasant activities (positive expectation and evaluation), that it will promote innovation (also positive expectation and evaluation), that it will threaten the professional future of participants (both low evaluation and low expectation), and that AI will occupy leading positions in working life (again, both low evaluation and low expectation). In contrast, the statements with the largest difference share the pattern that they are expected to become reality and are viewed negatively by the participants. The statements were that the development and use of AI will be influenced by a few, that the use of AI will lead to less communication, that AI will be influenced by an elite, that it will destroy more jobs than it creates, and finally that it will be hackable.
4.1. Are the estimated likelihood of occurrence and the evaluation correlated?
Next, we analyse whether the expected likelihood and perceived valence ratings are correlated. To do this, we calculated Pearson's correlation coefficient between the average ratings of the 38 AI-related topics. The test showed a weak association of (r = 0.215), but this is not significant (p = 0.196 > .05). This means that expectations of potential developments are not related to people's evaluations of them. Thus, our sample does not provide evidence that the perceived likelihood and valence of AI's impact on society, personal and professional life are related.
4.2. Does user diversity influence the technology foresight?
Finally, we examined whether the explanatory user factors influenced the evaluation and estimated likelihood of the different AI topics. To do this, we calculated an average score for the two target dimensions for each participant. A correlation analysis shows that both the mean likelihood and the mean evaluations of the topics are influenced by user diversity and the explanatory user factors. Table 3 shows the results of the analyses. Across the participants the mean valence is weakly and negatively related to trust (r = −0.253, p = 0.005) and positively related to distrust in AI (r = 0.221, p = 0.014). Thus, participants with higher distrust in AI rated the potential scenarios as slightly more favorable, while higher trust is associated with slightly lower evaluations.
The mean estimated likelihood of occurrence is related with distrust in AI (r = −0.336, p < 0.001), Affinity Toward Technology Interaction (r = 0.310, p < 0.001), trust in AI (r = 0.203, p = 0.025), as well as to interpersonal trust (r = 0.183, p = 0.043). Higher distrust in AI is associated with a lower estimated likelihood, while all other variables are associated with a higher estimated likelihood. It appears that distrust in AI is associated with a lower estimated likelihood.
5. Discussion
This article presents the results of a survey of people's expectations and evaluations of various statements about the impact AI might have on their lives and society. Overall, participants in our study associated AI with both positive and negative evaluations, and also considered certain developments to be more or less likely. Thus, AI and its implications are not perceived as either black or white, but participants had a nuanced view of how AI will affect their lives. From the perspective of social acceptance, issues of divergence between the two dimensions of expectation and evaluation deserve particular attention.
We analyzed the participants' subjective assessments of the developments. While this gives an insight into their beliefs and mental models, some of the assessments are likely to be challenged by other research. A critical point here is certainly the assessment of how AI will affect the labor market and individual employment opportunities. Our study participants are not very concerned about their professional future or the labor market as a whole. While a significant shift away from jobs with defined inputs and outputs (tasks perfectly suited for automation by AI) is predicted, which could lead to either lower employment or lower wages (Acemoglu and Restrepo, 2017; Brynjolfsson and Mitchell, 2017), participants in our sample do not feel personally affected by this development. They see a clearly positive effect on the overall economic performance and that in the context of AI it is likely that few new jobs will be created (and that jobs will rather be cut), but they do not see their individual future prospects as being at risk. This may be due to their qualifications or to an overestimation of their own market value in times of AI. Unfortunately, our research approach does not allow us to answer this question. However, comparing personal expectations, individual skills and future employment opportunities in the age of AI is an exciting research prospect.
Rather than examining the influence of individual differences, our study design focused on mapping expectations toward AI. However, this more explanatory analysis still revealed insights that deserve attention in research and policy making. Our results suggest that people with a lower general disposition to trust AI will, on average, evaluate the different statements more positively than people with a lower disposition to distrust. Similarly, a higher disposition to trust AI is associated with a lower average valence. When it comes to expectations for the future, the picture is reversed. A high disposition to trust is associated with a higher probability that the statements will come true, whereas a high disposition to distrust is associated with a lower expected probability. As a result, people with less trust rate the impact of AI as more positive but less likely. Thus, for this group, certain features and consequences of AI seem desirable, but there is a lack of conviction that this will happen in such a positive way. Future research should further differentiate the concept of trust in this context: On the one hand, trust that the technology is reliable and not harmful, and on the other hand, trust that the technology can deliver what is promised to oneself or by others, i.e., trust as opposed to confidence.
In our explanatory analysis, we examined whether the expected likelihood was related to the valuation. However, this relationship was not confirmed, although the (non-significant) correlation was quite large. We refrain from making a final assessment and suggest that the correlation between valence and expected likelihood of occurrence should be re-examined with a larger sample and a more precise measurement of the target dimensions. This would provide a deeper understanding of whether there is a systematic bias between these two dimensions and at the same time allow, if possible, to derive distinguishable expectation profiles to compare user characteristics, e.g., between groups that are rather pessimistic about AI development, groups that have exaggerated expectations or naive ideas about the possibilities of AI, or groups that reject AI but fear that it will nevertheless permeate life.
5.1. Implications
As discussed above, AI is at the center of attention when it comes to innovative and “new” technology. Huge promises have been made about the impact, both positive and negative, that AI could have on society as a whole, but also on an individual level (Brynjolfsson and Mitchell, 2017; Ikkatai et al., 2022). This development has led to a shift in public attention and attitudes toward AI. Therefore, it is necessary to elaborate on this perception and attitude in order to find future research directions and possible educational approaches to increase people's literacy about AI and AI-based technologies. This discussion should also include a discourse on ethical implications, i.e., possible moral principles that should guide the way we research and develop AI. These principles should include individual, organizational and societal values as well as expert judgements about the context in which AI is appropriate or not (Awad et al., 2018; Liehner et al., 2021).
Previous research on this approach shows that cynical distrust of AI, i.e., the attitude that AI cannot be trusted per se, is a different construct from the same kind of distrust toward humans (Bochniarz et al., 2022). This implies that although AI is thought to be close to the human mind—at least in some circumstances—it is not confused with the human traits of hostility or emotionality. Importantly, according to Kolasinka et al., people have different evaluations of AI depending on the context (Kolasinska et al., 2019): When asked in which field of AI research they would invest an unlimited amount of money, people chose the fields of medicine and cybersecurity. There seems to be an overlap between the context in which AI is placed and the level of trust required in that specific context. For example, most people are not necessarily experts in cybersecurity or medicine. However, because of the trust placed in an IT expert, a doctor or any other expert, people generally do not question the integrity of these experts. AI is a similar matter, as people do not usually attribute emotionality to it, but rather objectivity, so they tend to trust its accuracy and disregard its potential for error (Cismariu and Gherhes, 2019; Liu and Tao, 2022).
Despite the benefits of AI, an accurate knowledge of its potential and limitations is necessary for a balanced and useful use of AI-based technology (Hick and Ziefle, 2022). Therefore, educational programmes for the general public and non-experts in the field of AI seems appropriate to provide a tool with which people can evaluate for themselves the benefits and barriers of this technology (Olari and Romeike, 2021). More research is needed to find out what are the most important and essential aspects of such an educational programme, but the map presented here may be a suitable starting point starting point to identify crucial topics.
6. Limitations and future work
Of course, this study is not without its limitations. First, the sample of 122 participants is not representative for the whole population of our country or even across countries. We therefore recommend that this method be used with a larger, more diverse sample, including participants of all ages, from different educational backgrounds and, ideally, from different countries and cultures. Nevertheless, the results presented here have their own relevance: Despite the relatively homogeneous young and educated sample, certain misconceptions about AI became apparent and imbalances in estimated likelihood and valuation could be identified. These could either be an obstacle to future technology development and adoption and/or are aspects that require societal debate and possibly regulation.
Second, participants responded to a short item on each topic and we refrained from explaining each idea in more detail. As a result, the answers to these items may have been shaped by affective rather than cognitively considered considerations. However, this is not necessarily a disadvantage. On the one hand, this approach made it easier to explore a wide range of possible ways in which AI might affect our future. On the other hand, and more importantly, we as humans are not rational agents, but most of our decisions and behavior are influenced by cognitive biases and our affect (i.e., “affect heuristic”) (Finucane et al., 2000; Slovic et al., 2002). In this respect, this study contributes to affective technology evaluation, which nonetheless influences evaluation and use.
From a methodological point of view, asking for ratings with only two single items leads to a high variance and makes it difficult to examine individual aspects in detail. Although this allowed us to address a variety of different issues, future work should select specific aspects and examine them in more detail. Consequently, future work may further integrate other concepts, such as the impact of AI on individual mobility, public safety, or even warfare through automation and control. However, the present approach allowed us to keep the survey reasonably short, which had a positive effect on response attention and unbiased dropout rates.
Finally, we propose the integration of expert judgement into this cartography. We suspect that there are considerable differences between expert and lay assessments, particularly in the assessment of the expected likelihood of the developments in question. Again, it is the differences between expert and lay expectations that are particularly relevant for informing researchers and policy makers.
7. Conclusion
The continuing and increasing pervasiveness of AI in almost all personal and professional contexts will reshape our future, how we interact with technology, and how we interact with others using technology. Responsible research and innovation on AI-based products and services requires us to balance technical advances and economic imperatives with individual, organizational, and societal values (Burget et al., 2017; Owen and Pansera, 2019).
This work suggests that the wide range of potential AI applications is assessed differently in terms of perceived likelihood and perceived valence as a measure of acceptability. The empirically derived criticality map makes this assessment visible and highlights issues with urgent potential for research, development, and governance and can thus contribute to responsible research and innovation of AI.
We also found individual differences in perceptions of AI that may threaten both people's ability to participate in societal debates about AI and to adequately adapt their future skill sets to compete with AI in the future of work. It is a political issue, not a technological one, in which areas AI can influence our lives and society, and to what extent. As a society, we need to discuss and debate the possibilities and limits of AI in a wide range of applications and define appropriate regulatory frameworks. For this to happen, we all need to have a basic understanding of AI so that we can participate in a democratic debate about its potential and its limits. Free online courses for adults such as “Elements of AI” and modern school curricula that teach the basics of digitalisation and AI are essential for this (Olari and Romeike, 2021; Marx et al., 2022).
Data availability statement
The datasets presented in this study can be found in online repositories. The names of the repository/repositories and accession number(s) can be found below: https://osf.io/f9ek6/.
Ethics statement
Ethical review and approval was not required for the study on human participants in accordance with the local legislation and institutional requirements. The patients/participants provided their written informed consent to participate in this study.
Author contributions
PB and RP designed the study. PB wrote the original draft of the manuscript and coordinated the analysis and writing, while RP and AH made substantial contributions to the motivation, related work, and discussion sections of the manuscript. MZ supervised the work and acquired the funding for this research. All authors contributed to the article and approved the submitted version.
Funding
This work was funded by the Deutsche Forschungsgemeinschaft (DFG, German Research Foundation) under Germany's Excellence Strategy—EXC-2023 Internet of Production—390621612 and the VisuAAL project “Privacy-Aware and Acceptable Video-Based Technologies and Services for Active and Assisted Living”, funded by the European Union's Horizon 2020 Research and Innovation Programme under the Marie Skłodowska-Curie Grant Agreement No. 861091.
Acknowledgments
We would particularly like to thank Isabell Busch for her support in recruiting participants, Mohamed Behery for his encouragement in writing this manuscript, Luca Liehner for feedback on the key figure, and Johannes Nakayama and Tim Schmeckel for their support in analysis and writing. Thank you to the referees and the editor for their valuable and constructive input. The writing of this article was partly supported by ChatGPT, DeepL, and DeepL Write.
Conflict of interest
The authors declare that the research was conducted in the absence of any commercial or financial relationships that could be construed as a potential conflict of interest.
Publisher's note
All claims expressed in this article are solely those of the authors and do not necessarily represent those of their affiliated organizations, or those of the publisher, the editors and the reviewers. Any product that may be evaluated in this article, or claim that may be made by its manufacturer, is not guaranteed or endorsed by the publisher.
Footnotes
1. ^Picasso was referring to mechanical calculation machines, nowadays called computers.
References
Acemoglu, D., and Restrepo, P. (2017). The Race Between Machine and Man. Am. Econ. Rev. 108, 1488–1542. doi: 10.3386/w22252
Almars, A. M., Gad, I., and Atlam, E.-S. (2022). “Applications of AI and IoT in COVID-19 vaccine and its impact on social life,” in Medical Informatics and Bioimaging Using Artificial Intelligence (Springer), 115–127.
Araujo, T., Helberger, N., Kruikemeier, S., and Vreese, C. H. de (2020). In AI we trust? Perceptions About Automated Decision-making by Artificial Intelligence. AI Society 35, 611–623. doi: 10.1007/s00146-019-00931-w
Awad, E., Dsouza, S., Kim, R., Schulz, J., Henrich, J., Shariff, A., et al. (2018). The moral machine experiment. Nature 563, 59–64. doi: 10.1038/s41586-018-0637-6
Beierlein, K. C. (2014). Interpersonales vertrauen (KUSIV3). Zusammenstellung sozialwissenschaftlicher Items und Skalen (ZIS). doi: 10.6102/zis37
Binz, M., and Schulz, E. (2023). Using cognitive psychology to understand GPT-3. Proc. Natl. Acad. Sci. U.S.A. 120, e2218523120. doi: 10.1073/pnas.2218523120
Bochniarz, K. T., Czerwiński, S. K., Sawicki, A., and Atroszko, P. A. (2022). Attitudes to AI among high school students: Understanding distrust towards humans will not help us understand distrust towards AI. Pers. Ind. Diff. 185, 111299. doi: 10.1016/j.paid.2021.11129
Brauner, P., Dalibor, M., Jarke, M., Kunze, I., Koren, I., Lakemeyer, G., et al. (2022). A computer science perspective on digital transformation in production. ACM Trans. Internet Things 3, 1–32. doi: 10.1145/3502265
Brown, T., Mann, B., Ryder, N., Subbiah, M., Kaplan, J. D., Dhariwal, P., et al. (2020). “Language models are few-shot learners,” in Advances in neural information processing systems, eds H. Larochelle, M. Ranzato, R. Hadsell, M. F. Balcan, and H. Lin (Curran Associates, Inc.), 1877–1901. Available online at: https://proceedings.neurips.cc/paper/2020/file/1457c0d6bfcb4967418bfb8ac142f64a-Paper.pdf
Brynjolfsson, E., and Mitchell, T. (2017). What can machine learning do? Workforce implications. Science 358, 1530–1534. doi: 10.1126/science.aap8062
Bughin, J., Seong, J., Manyika, J., Chui, M., and Joshi, R. (2018). Notes from the AI frontier: modeling the impact of AI on the world economy. McKinsey Glob. Inst. 4.
Burbach, L., Halbach, P., Plettenberg, N., Nakayama, J., Ziefle, M., and Calero Valdez, A. (2019). ““Hey, Siri”, “Ok, Google”, “Alexa”. Acceptance-relevant factors of virtual voice-assistants,” in 2019 IEEE International Professional Communication Conference (ProComm) (Aachen: IEEE), 101–111.
Burget, M., Bardone, E., and Pedaste, M. (2017). Definitions and conceptual dimensions of responsible research and innovation: a literature review. Sci. Eng. Ethics 23, 1–19. doi: 10.1007/s11948-016-9782-1
Cambridge Dictionary (2022). Cambridge dictionary. Artificial Intelligence. Available online at: https://dictionary.cambridge.org/dictionary/english/artificial-intelligence (accessed December 1, 2022).
Cismariu, L., and Gherhes, V. (2019). Artificial intelligence, between opportunity and challenge. Brain 10, 40–55. doi: 10.18662/brain/04
Collingridge, D. (1982). Social Control of Technology. Continuum International Publishing Group Ltd.
Corea, F. (2019). “AI knowledge map: How to classify AI technologies,” in An Introduction to Data. Studies in Big Data, Vol 50 (Cham: Springer). doi: 10.1007/978-3-030-04468-8_4
Cronbach, L. J. (1951). Coefficient alpha and the internal structure of tests. psychometrika 16, 297–334.
Cugurullo, F., and Acheampong, R. A. (2023). Fear of AI: An inquiry into the adoption of autonomous cars in spite of fear, and a theoretical framework for the study of artificial intelligence technology acceptance. AI & Society, 1–16. doi: 10.1007/s00146-022-01598-6
Dalkey, N., and Helmer, O. (1963). An experimental application of the delphi method to the use of experts. Manag. Sci. 9, 458–467. doi: 10.1287/mnsc.9.3.458
Dienes, Z. (2008). Understanding Psychology as a Science-An Introduction to Scientific and Statistical Inference, 1st Edn (London: Red Globe Press).
Finucane, M. L., Alhakami, A., Slovic, P., and Johnson, S. M. (2000). The affect heuristic in judgments of risks and benefits. J. Behav. Decis. Making 13, 1–17. doi: 10.1002/(SICI)1099-0771(200001/03)13:1<::AID-BDM333>.0.CO;2-S
Floridi, L., and Cowls, J. (2022). “A unified framework of five principles for AI in society,” in Machine Learning and the City: Applications in Architecture and Urban Design, 535–545.
Flowers, J. C. (2019). “Strong and weak AI: deweyan considerations,” in AAAI Spring Symposium: Towards Conscious AI Systems.
Foot, P. (1967). The Problem of Abortion and the Doctrine of the Double Effect. Oxford: Oxford Review.
Fosso Wamba, S., Bawack, R. E., Guthrie, C., Queiroz, M. M., and Carillo, K. D. A. (2021). Are we preparing for a good AI society? A bibliometric review and research agenda. Technol. Forecast. Soc. Change 164, 120482. doi: 10.1016/j.techfore.2020.120482
Franke, T., Attig, C., and Wessel, D. (2019). A personal resource for technology interaction: development and validation of the affinity for technology interaction (ATI) scale. Int. J. Human Comput. Interact. 35, 456–467. doi: 10.1080/10447318.2018.1456150
Gibson, R. (2019). Desire in the Age of Robots and AI: An Investigation in Science Fiction and Fact. Springer.
Grace, K., Salvatier, J., Dafoe, A., Zhang, B., and Evans, O. (2018). When will AI exceed human performance? Evidence from AI experts. J. Artif. Intell. Res. 62, 729–754. doi: 10.1613/jair.1.11222
Gunkel, D. J. (2012). The Machine Question: Critical Perspectives on AI, Robots, and Ethics. Cambridge, MA: MIT Press.
Hick, A., and Ziefle, M. (2022). “A qualitative approach to the public perception of AI,” in IJCI Conference Proceedings, eds D. C. Wyld et al., 01–17.
Hirsch-Kreinsen, H. (2023). Artificial intelligence: A “promising technology.” AI & Society 2023, 1–12. doi: 10.1007/s00146-023-01629-w
Hoff, K. A., and Bashir, M. (2015). Trust in automation: integrating empirical evidence on factors that influence trust. Human Factors 57, 407–434. doi: 10.1177/0018720814547570
Ikkatai, Y., Hartwig, T., Takanashi, N., and M., Y. H. (2022). Segmentation of ethics, legal, and social issues (ELSI) related to AI in Japan, the united states, and Germany. AI Ethics doi: 10.1007/s43681-022-00207-y
Jovanovic, M., Mitrov, G., Zdravevski, E., Lameski, P., Colantonio, S., Kampel, M., et al. (2022). Ambient assisted living: Scoping review of artificial intelligence models, domains, technology, and concerns. J. Med. Internet Res. 24, e36553. doi: 10.2196/36553
Jumalon, G. (2022). TL;DR–someone entered an art competition with an AI-generated piece and won the first prize. Available online at: https://twitter.com/GenelJumalon/status/1564651635602853889 (accessed November 28, 2022).
Kelly, S., Kaye, S.-A., and Oviedo-Trespalacios, O. (2023). What factors contribute to the acceptance of artificial intelligence? A systematic review. Telematics Inf. 77, 101925. doi: 10.1016/j.tele.2022.101925
Klos, A., Rosenbaum, M., and Schiffmann, W. (2020). “Emergency landing field identification based on a hierarchical ensemble transfer learning model,” in IEEE 8th international symposium on computing and networking (CANDAR) (Naha: IEEE), 49–58.
Kolasinska, A., Lauriola, I., and Quadrio, G. (2019). “Do people believe in artificial intelligence? A cross-topic multicultural study,” in Proceedings of the 5th EAI International Conference on Smart Objects and Technologies for social good GoodTechs '19. (New York, NY: Association for Computing Machinery), 31–36.
Kulida, E., and Lebedev, V. (2020). “About the use of artificial intelligence methods in aviation,” in 13th International Conference on Management of Large-Scale System Development (MLSD), 1–5. doi: 10.1109/MLSD49919.2020.9247822
Kulkarni, S., Seneviratne, N., Baig, M. S., and Khan, A. H. A. (2020). Artificial intelligence in medicine: where are we now? Acad. Radiol. 27, 62–70. doi: 10.1016/j.acra.2019.10.001
Lecun, Y., Bengio, Y., and Hinton, G. (2015). Deep Learning. Nature 521, 436–444. doi: 10.1038/nature14539
Liehner, G. L., Brauner, P., Schaar, A. K., and Ziefle, M. (2021). Delegation of moral tasks to automated agents The impact of risk and context on trusting a machine to perform a task. IEEE Trans. Technol. Soc. 3, 46–57. doi: 10.1109/TTS.2021.3118355
Lin, H.-Y. (2023). Standing on the shoulders of AI giants. Computer 56, 97–101. doi: 10.1109/MC.2022.3218176
Litjens, G., Kooi, T., Bejnordi, B. E., Setio, A. A. A., Ciompi, F., Ghafoorian, M., et al. (2017). A survey on deep learning in medical image analysis. Med. Image Anal. 42, 60–88. doi: 10.1016/j.media.2017.07.005
Liu, K., and Tao, D. (2022). The roles of trust, personalization, loss of privacy, and anthropomorphism in public acceptance of smart healthcare services. Comput. Human Behav. 127, 107026. doi: 10.1016/j.chb.2021.107026
Liu, R., Rizzo, S., Whipple, S., Pal, N., Pineda, A. L., Lu, M., et al. (2021). Evaluating eligibility criteria of oncology trials using real-world data and AI. Nature 592, 629–633. doi: 10.1038/s41586-021-03430-5
Makridakis, S. (2017). The forthcoming artificial intelligence (AI) revolution: its impact on society and firms. Futures 90, 46–60. doi: 10.1016/j.futures.2017.03.006
Marcus, G., and Davis, E. (2019). Rebooting AI: Building Artificial Intelligence We Can Trust. New York, NY: Pantheon Books.
Marx, E., Leonhardt, T., Baberowski, D., and Bergner, N. (2022). “Using matchboxes to teach the basics of machine learning: An analysis of (possible) misconceptions,” in Proceedings of the Second Teaching Machine Learning and Artificial Intelligence Workshop Proceedings of Machine Learning Research, eds K. M. Kinnaird, P. Steinbach, and O. Guhr (PMLR), 25–29. Available online at: https://proceedings.mlr.press/v170/marx22a.html
Mayer, R. C., Davis, J. H., and Schoorman, F. D. (1995). An integrative model of organizational trust. Acad. Manag. Rev. 20, 709–734.
Mc Knight, D. H., Choudury, V., and Kacmar, C. (2002). Developing and validating trust measure for e-commerce: an integrative typology. Inf. Syst. Res. 13, 334–359. doi: 10.1287/isre.13.3.334.81
McCarthy, J., Minsky, M. L., Rochester, N., and Shannon, C. E. (2006). A proposal for the dartmouth summer research project on artificial intelligence (August 31, 1955). AI Mag. 27, 12–12. doi: 10.1609/aimag.v27i4.1904
McCrae, R. R., and Costa, P. T. (1987). Validation of the five-factor model of personality across instruments and observers. J. Pers. Soc. Psychol. 52, 81.
Oden, L., and Witt, T. (2020). “Fall-detection on a wearable micro controller using machine learning algorithms,” in IEEE International Conference on Smart Computing 2020 (SMARTCOMP) (Bologna: IEEE), 296–301.
Oksanen, A., Savela, N., Latikka, R., and Koivula, A. (2020). Trust toward robots and artificial intelligence: an experimental approach to human–technology interactions online. Front. Psychol. 11, 568256. doi: 10.3389/fpsyg.2020.568256
Olari, V., and Romeike, R. (2021). “Addressing AI and data literacy in teacher education: a review of existing educational frameworks,” in The 16th Workshop in Primary and Secondary Computing Education WiPSCE '21 (New York, NY: Association for Computing Machinery).
Onnasch, L., and Roesler, E. (2020). A taxonomy to structure and analyze human–robot interaction. Int. J. Soc. Rob. 13, 833–849. doi: 10.1007/s12369-020-00666-5
Owen, R., Macnaghten, P., and Stilgoe, J. (2012). Responsible research and innovation: from science in society to science for society, with society. Sci. Public Policy 39, 751–760. doi: 10.1093/scipol/scs093
Owen, R., and Pansera, M. (2019). Responsible innovation and responsible research and innovation. Handbook Sci. Public Policy, 26–48. doi: 10.4337/9781784715946.00010
Philipsen, R., Brauner, P., Biermann, H., and Ziefle, M. (2022). I am what i am–roles for artificial intelligence from the users' perspective. Artif. Intell. Soc. Comput. 28, 108–118. doi: 10.54941/ahfe1001453
Rao, Q., and Frtunikj, J. (2018). “Deep learning for self-driving cars: Chances and challenges,” in Proceedings of the 1st International Workshop on Software Engineering for AI in Autonomous Systems, 35–38. doi: 10.1145/3194085.3194087
Rashidi, P., and Mihailidis, A. (2013). A survey on ambient-assisted living tools for older adults. IEEE J. Biomed. Health Inform. 17, 579–590. doi: 10.1109/JBHI.2012.2234129
Reeves, B., and Nass, C. (1996). The Media Equation–How People Treat Computers, Television, and New Media Like Real People and Places. Cambridge: Cambridge University Press.
Robb, D. A., Ahmad, M. I., Tiseo, C., Aracri, S., McConnell, A. C., Page, V., et al. (2020). “Robots in the danger zone: Exploring public perception through engagement,” in Proceedings of the 2020 ACM/IEEE International Conference on Human-Robot Interaction HRI '20. (New York, NY: Association for Computing Machinery), 93–102.
Rodriguez-Bustelo, C., Batista-Foguet, J. M., and Serlavós, R. (2020). Debating the future of work: The perception and reaction of the spanish workforce to digitization and automation technologies. Front. Psychol. 11, 1965. doi: 10.3389/fpsyg.2020.01965
Russell, S., and Norvig, P. (2009). Artificial Intelligence: A Modern Approach, 3rd Edn. Hoboken, NJ: Prentice Hall Press.
Sindermann, C., Sha, P., Zhou, M., Wernicke, J., Schmitt, H. S., Li, M., et al. (2021). Assessing the attitude towards artificial intelligence: introduction of a short measure in german, chinese, and english language. KI-Künstliche Intelligenz 35, 109–118. doi: 10.1007/s13218-020-00689-0
Slovic, P., Finucane, M., Peters, E., and MacGregor, D. G. (2002). “The affect heuristic,” in Heuristics and Biases: The Psychology of Intuitive Judgment, eds T. Gilovich, D. Griffin, and D. Kahneman (Cambridge: Cambridge University Press), 397–420.
Smith, A., and Anderson, J. (2014). AI, robotics, and the future of jobs. Pew Res. Center 6, 51. Available online at: https://www.pewresearch.org/internet/2014/08/06/future-of-jobs/
Statista (2022). Artificial intelligence (AI) worldwide-statistics & facts. Available online at: https://www.statista.com/topics/3104/artificial-intelligence-ai-worldwide/#dossier-chapter1 (accessed November 28, 2022)
Strich, F., Mayer, A.-S., and Fiedler, M. (2021). What do i do in a world of artificial intelligence? Investigating the impact of substitutive decision-making AI systems on employees' professional role identity. J. Assoc. Inf. Syst. 22, 9. doi: 10.17705/1jais.00663
Taherdoost, H. (2018). A review of technology acceptance and adoption models and theories. Procedia Manufact. 22, 960–967. doi: 10.1016/j.promfg.2018.03.137
Topol, E. (2019). Deep Medicine: How Artificial Intelligence Can Make Healthcare Human Again. London: Hachette UK.
Vaishya, R., Javaid, M., Khan, I. H., and Haleem, A. (2020). Artificial intelligence (AI) applications for COVID-19 pandemic. Diabetes Metabolic Syndrome Clin. Res. Rev. 14, 337–339. doi: 10.1016/j.dsx.2020.04.012
West, D. M. (2018). The Future of Work: Robots, AI, and Automation. Washington, DC: Brookings Institution Press.
Wilkowska, W., Brauner, P., and Ziefle, M. (2018). “Rethinking technology development for older adults: a responsible research and innovation duty,” in Aging, Technology and Health, eds R. Pak and A. C. McLaughlin (Cambridge, MA: Academic Press), 1–30.
Wolff, J., Pauling, J., Keck, A., and Baumbach, J. (2020). The economic impact of artificial intelligence in health care: Systematic review. J. Med. Internet Res. 22, e16866. doi: 10.2196/16866
Young, A. T., Amara, D., Bhattacharya, A., and Wei, M. L. (2021). Patient and general public attitudes towards clinical artificial intelligence: a mixed methods systematic review. Lancet Digital Health 3, e599–e611. doi: 10.1016/S2589-7500(21)00132-1
Keywords: artificial intelligence, affect heuristic, public perception, user diversity, mental models, technology acceptance, responsible research and innovation (RRI), collingridge dilemma
Citation: Brauner P, Hick A, Philipsen R and Ziefle M (2023) What does the public think about artificial intelligence?—A criticality map to understand bias in the public perception of AI. Front. Comput. Sci. 5:1113903. doi: 10.3389/fcomp.2023.1113903
Received: 01 December 2022; Accepted: 28 February 2023;
Published: 16 March 2023.
Edited by:
Simona Aracri, National Research Council (CNR), ItalyReviewed by:
Galena Pisoni, University of Nice Sophia Antipolis, FranceMohammad Faiz Iqbal Faiz, Tezpur University, India
Copyright © 2023 Brauner, Hick, Philipsen and Ziefle. This is an open-access article distributed under the terms of the Creative Commons Attribution License (CC BY). The use, distribution or reproduction in other forums is permitted, provided the original author(s) and the copyright owner(s) are credited and that the original publication in this journal is cited, in accordance with accepted academic practice. No use, distribution or reproduction is permitted which does not comply with these terms.
*Correspondence: Philipp Brauner, brauner@comm.rwth-aachen.de