- 1Aix-Marseille Université, CNRS, LPL, Marseille, France
- 2Aix Marseille Université, CNRS, LIS, Marseille, France
Understanding children's conversational skills is crucial for understanding their social, cognitive, and linguistic development, with important applications in health and education. To develop theories based on quantitative studies of conversational development, we need (i) data recorded in naturalistic contexts (e.g., child-caregiver dyads talking in their daily environment) where children are more likely to show much of their conversational competencies, as opposed to controlled laboratory contexts which typically involve talking to a stranger (e.g., the experimenter); (ii) data that allows for clear access to children's multimodal behavior in face-to-face conversations; and (iii) data whose acquisition method is cost-effective with the potential of being deployed at a large scale to capture individual and cultural variability. The current work is a first step to achieving this goal. We built a corpus of video chats involving children in middle childhood (6–12 years old) and their caregivers using a weakly structured word-guessing game to prompt spontaneous conversation. The manual annotations of these recordings have shown a similarity in the frequency distribution of multimodal communicative signals from both children and caregivers. As a case study, we capitalize on this rich behavioral data to study how verbal and non-verbal cues contribute to the children's conversational coordination. In particular, we looked at how children learn to engage in coordinated conversations, not only as speakers but also as listeners, by analyzing children's use of backchannel signaling (e.g., verbal “mh” or head nods) during these conversations. Contrary to results from previous in-lab studies, our use of a more spontaneous conversational setting (as well as more adequate controls) revealed that school-age children are strikingly close to adult-level mastery in many measures of backchanneling. Our work demonstrates the usefulness of recent technology in video calling for acquiring quality data that can be used for research on children's conversational development in the wild.
1. Introduction
Conversation is a ubiquitous and complex social activity in our lives. Cognitive scientists consider it as a hallmark of human cognition as it relies on a sophisticated ability for coordination and shared attention (e.g., Tomasello, 1999; Laland and Seed, 2021). Prominent computer scientists have sometimes described it as the ultimate test for Artificial Intelligence (Turing, 1950). Its role in healthy development is also crucial: When conversational skills are not well developed, they can negatively impact our ability to learn from others and to maintain relationships (Hale and Tager-Flusberg, 2005; Nadig et al., 2010; Murphy et al., 2014). Thus, the scientific study of how conversational skills develop in childhood is of utmost importance to understand what makes human cognition so special, to design better (child-oriented) conversational AI, and to allow more targeted and efficient clinical interventions (e.g., autistic individuals).
Conversation involves a variety of skills such as turn-taking management, negotiating shared understanding with the interlocutor, and the ability for a coherent/contingent exchange (e.g., Sacks et al., 1974; Clark, 1996; Fusaroli et al., 2014; Pickering and Garrod, 2021; Nikolaus and Fourtassi, 2023). We know little about how these skills manifest in face-to-face conversations, mainly because conversation has two characteristics that have made it difficult to fully characterize using only traditional research methods in developmental psychology. First, conversation is inherently spontaneous, i.e., it cannot be scripted, making it crucial to study in its natural environment. Second, conversation relies on collaborative multimodal signaling, involving, e.g., gesture, eye gaze, facial expressions, as well as intonation and linguistic content (e.g., Levinson and Holler, 2014; Özyürek, 2018; Krason et al., 2022). While controlled in-lab studies have generally fallen short of the ecological validity (that is, the first characteristic), observational studies in the wild (typically in the context of child-caregiver interaction), though very insightful, have fallen short of accounting for the multimodal dynamics, especially the role of non-verbal communicative abilities that are crucial for a coordinated conversation (e.g., Clark, 2018).
1.1. Video calls as a scalable data acquisition method
Recent technological advances in Natural Language Processing and Computer Vision allow us, in theory, to go beyond the limitations of traditional research methods as they provide tools to study complex multimodal dynamics while scaling up to more naturalistic data. Nevertheless, work in this direction has been slowed down by the lack of data on child conversations that allow the study of their multimodal communicative signals. Indeed, most existing video data of child-caregiver interaction either use a third-point-view camera (CHILDES) (MacWhinney, 2014) or head-mounted cameras (Sullivan et al., 2022). Neither of these recording methods allows clear access to the interlocutor's facial expressions and head gestures.
The current work is a step toward filling this gap. More precisely, we introduce a data acquisition method based on online child-caregiver online video calls. Using this method, we build and manually annotate a corpus of child-caregiver multimodal conversations. While communication takes place through a computer screen, making it only an approximation of direct face-to-face conversation, the big advantage of this data acquisition method is that it provides much clearer data of facial expressions and head gestures than commonly used datasets of child-caregiver conversations. Other advantages of this setting include cost-effectiveness—facilitating large-scale data collection (including across different countries)—and its naturalness in terms of the recording context (as opposed to in-lab controlled studies).
To prompt conversations between children and their caregivers, we use a novel conversational task (a word-guessing game) that is very intuitive and weakly structured, allowing conversations to flow spontaneously. The goal is to approximate a conversational activity that children could do with their caregivers at home, eliciting as much as possible the children's natural conversational skills. Unlike other—more classic—semi-structured tasks used in the study of adult-adult conversations (e.g., the map task Anderson et al., 1993), the word-guessing game does not require looking at a prompt (e.g., a map), thus optimizing children's non-verbal signaling behavior while interacting with the caregiver (we return to this point in the Task sub-section).
Finally, to evaluate children's conversational skills compared to adult-level mastery, it may not be enough to consider the caregiver as the only “end-state” reference. The reason is that research has shown that caregivers tend to adapt to children's linguistic and conversational competencies (e.g., Snow, 1977; Misiek et al., 2020; Fusaroli et al., 2021; Leung et al., 2021; Foushee et al., 2022; Misiek and Fourtassi, 2022). Thus, in addition to child-caregiver conversations, we must study how adults behave in similar interactive situations involving other adults. We collected similar data involving the same caregiver talking either to another family member or to a non-family member, the former situation being closer to the child-caregiver social context.
1.2. Case study: Backchannel signaling
In addition to introducing a new data acquisition method for child-caregiver conversation, we also illustrate its usefulness in the study of face-to-face communicative development by analyzing how children compare to adults in terms of Backchannel signaling (hereafter BC). We chose BC as a case study since it is an important conversational skill that has received—surprisingly—little attention in the language development literature and because it relies heavily on multimodal signaling.
BC (Yngve, 1970) is a communicative feedback that the listener provides to the speaker in a non-intrusive fashion such as short vocalizations like “yeah” and “uh-huh,” and/or nonverbal cues such as head nods and smiles. Despite not having necessarily a narrative content, BC is a crucial element in successfully coordinated conversations, signaling, e.g., attention, understanding, and agreement (or lack thereof) while allowing the speaker to make the necessary adjustments toward achieving mutual understanding or “communicative grounding” (Clark, 1996).
What about the development of BC? While some studies have documented early signs of children's ability to both interpret and provide BC feedback in the preschool period (e.g., Shatz and Gelman, 1973; Peterson, 1990; Park et al., 2017), a few have pointed out that this skill continues developing well into middle childhood. For example, Dittmann (1972) analyzed conversations of 6 children between the ages of 7 and 12 in a laboratory setting where children conversed with adults and other children as well as children interacting with each other at school. The results found there to be fewer BC signals produced by young children in this age range compared to the older group (between 14 and 35 years old). Following Dittmann (1972)'s study and Hess and Johnston (1988) aimed at providing a more detailed developmental account of BC behaviors in middle childhood using a task where children listened to board game instructions from an experimenter. In particular, the authors analyzed children's BC production in various speaker cues such as pauses >400 ms, the speaker's eye gaze toward the listener, and the speaker's clause boundaries. Hess and Johnston found that younger children produced less BC compared to older ones.
While these previous studies (e.g., Dittmann, 1972; Hess and Johnston, 1988) provided important insight into BC development, they both analyzed BC when children were engaged in conversations that may not be the ideal context to elicit and characterize children's full conversational competence. Some conversations were recorded with strangers, in a laboratory, and/or with non-spontaneous predesigned scripts. We argue that children's conversational skills could have been underestimated in these previous studies because they focus on contexts that are unnatural to the child and are not similar to how they communicate spontaneously in daily life. Indeed, research has shown that context can influence the nature of conversational behavior more generally (e.g., Dideriksen et al., 2019). We hypothesize that recording children in a more natural context using methods such as ours would allow them to show more of their natural conversational skills, namely in terms of BC. More specifically, we expect children to produce more BC (relative to adults' production) compared to previous work. Furthermore, we predict that children's BC behavior would be closer to that found in the adult family dyads (than to adult non-family dyads) because it is supposed to provide a more similar social context to the child-caregiver context.
1.3. Related work and novelty of our study
The novelty of the current effort compared to previous work based on available child-caregiver conversational data (e.g., MacWhinney, 2014; Sullivan et al., 2022) is threefold:
1) We aim at capturing the multimodal aspects of children's conversational skills, especially facial expressions, gaze, and head gestures which are crucial to face-to-face conversations. While much of previous work has focused on verbal or vocal signals (e.g., Snow, 1977; Warlaumont et al., 2014; Hazan et al., 2017; Clark, 2018), a comprehensive study of conversational skills requires that we also investigate how children learn to coordinate with the interlocutor using visual signals. It is worth mentioning that some previous studies have proposed procedures to collect and analyze videos of school-age children (e.g., Roffo et al., 2019; Vo et al., 2020; Rooksby et al., 2021), however, these studies do not provide a method to collect face-to-face conversational data. Rather, they focus on clinical tasks such as the Manchester Child Attachment Story Task.
2) We focus on middle childhood (i.e., 6–12 years old), an age range that has received little attention compared to the preschool period, although middle childhood is supposed to witness important developmental changes in conversational skills, e.g., in turn-taking management (Maroni et al., 2008), conversational grounding (e.g., backchannels, Hess and Johnston, 1988), and the ability to engage in coherent/contingent exchange (Dorval and Eckerman, 1984; Baines and Howe, 2010). Another—perhaps more pragmatic—reason we focus on middle childhood is that children in this period have already mastered much of formal language (e.g., phonology and syntax), allowing us to minimize the interference of language processing- and production-related issues with the measurement of “pure” conversational skills.
3) Regarding the case study of BC in middle childhood, the novelty of our work is that it provides a socially more natural context where children could show a more spontaneous use of their conversational skills. Indeed, in our new corpus, the children talk one-on-one with their caregivers (as opposed to a stranger/experimenter), are at home (as opposed to the lab or school), and converse to play a fun, easy, and natural game (word guessing) that many children are already familiar with, as opposed to scripted turns (e.g., Hess and Johnston, 1988) or complex conversational games such as the map task (e.g., Anderson et al., 1991).
The paper is organized into three parts: Corpus, Annotation, and the case study of BC. In the first part, we present the methodology for Corpus building. In the second, we present the coding scheme we used to annotate the video call recordings for several—potentially communicative—visual features as well as the inter-rater reliability for each feature. In the third, we present the results of the BC analyses comparing child-caregiver dyads to caregiver-adult dyads. Finally, we discuss the findings, impact, and limitations of this new data acquisition method.
2. The corpus
The Corpus consists of online video chat recordings. In what follows, we provide details about the participants, the recording setup, the task we used to elicit conversational data, and the recording procedure.
2.1. Participants
We recorded 20 dyads. Among these dyads, 10 involved children and their caregivers (the condition of interest) and 10 involved the same caregivers with other adults (the control condition). In the BC case study, below, we also analyzed this control condition by whether or not the caregiver and adult were family members: We had 5 family dyads and 5 non-family dyads (more detail is provided in Section 4). In total, we collected 20 conversations or N = 40 individual videos (i.e., of individual interlocutors). Each pair of videos lasted around 15 min for a total of 5 h and 49 min across both conditions. The children were 6–11 years old (M = 8.5, SD = 1.37). All 10 children were native French speakers and half were bilinguals. Children did not have any communicative or developmental disorders except for one child who had mild autism. One caregiver participated twice as they had two children in our sample. The caregivers were recruited among our university colleagues.
2.2. Recording setup
We did the recording using the online video chat system “Zoom” (Zoom Video Communications Inc., 2021). The setup required that the caregiver and child use different devices (e.g., two laptops or a laptop and a tablet) and that they communicate from different rooms (if they record from the same house) in order to avoid issues due to echo. We also required that the caregiver wore a headset microphone during the recording for better audio quality.1
2.3. The task
The task consists in playing a word-guessing game in which one of the participants thinks of a word and the other tries to find it by asking questions. After a word has been guessed, the interlocutors alternate their roles. The participants were told that they can finish the game after around 10 min. The caregivers were provided with a list of words to use during the interaction with the child whereas the children were free to choose the words they wanted. Detailed task instructions can be found in the Appendix below.
We chose this weekly-structured task over free conversations in order to correct for the asymmetrical aspect of child-caregiver interaction. In fact, our piloting of free conversation has led to rather unbalanced exchanges whereby the caregiver ends up playing a dominant role in initiating and keeping the conversation alive. We also piloted other, semi-structured tasks that have been used in previous studies to elicit spontaneous exchange between adults such as the map task (Anderson et al., 1993) and variants of the “spot the difference” task (Van Engen et al., 2010). Nevertheless, in both of these tasks, we observed that the child tends to overly fixate on the prompt (the prompt being the map in the first task and the pair of pictures in the second) which hindered a natural multimodal signaling dynamics with the interlocutor. We ended up picking the word-guessing game as (i) it allowed for a balanced exchange between children and caregiver and (ii) it does not involve a prompt, thus, optimizing multimodal signaling behavior during the entire interaction.
2.4. Procedure
We recorded a three-way call involving 1) the experimenter (doing the recording), 2) the caregiver, and 3) either the child (in the condition of interest) or another adult (in the control condition). The experimenter was muted during the entire interaction and used a black profile picture in order not to distract the participants. The experimenter was able to record both interlocutors by pinning their profiles side-by-side on her local machine (Figure 1). Furthermore, the participants were instructed to hide “self-view” and to pin only their interlocutors. The procedure consisted of three phases (all were included in the recordings). First, the caregiver (in both conditions) explains the task to the child, then the pair does the task for around 10 min, and lastly, the caregiver initiates a free conversation with the child (or adult) to chat about how the task went (for about 5 min).
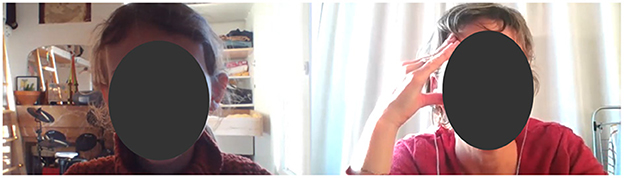
Figure 1. A snapshot of one of the recording sessions involving a child and her caregiver communicating through an online video chat system.
2.5. Video call software: Zoom
We used Zoom for video calls since our participants were familiar with this software. It is worth mentioning that Zoom has built-in audio-enhancing features that can be problematic for the study of some conversational phenomena, especially BC. In particular, one feature consists in giving the stage to one speaker while suppressing background noise that may come from other participants' microphones. We made sure, in preliminary testing, that BC was not suppressed as “background noise,” at least in our case where there were only two active participants in the Zoom session.
3. Annotation
We manually annotated the entire N = 40 individual recordings in our corpus for several visual—and potentially communicative—signals.2 The annotation was performed using a custom-developed template on ELAN. The annotated features were: gaze direction (looking at interlocutor vs. looking away), head movements (head nod and head shake), eyebrow movements (frowning and eyebrow-raising), mouth displays (smiles and laughter), and posture change (leaning forward or backward). In what follows, we elaborate on each of these annotations followed by an explanation of the annotation methods.
3.1. Annotated features
3.1.1. Gaze direction
Previous studies (e.g., Kendon, 1967) suggest that eye gaze can be used to control the flow of conversation, e.g., by regulating the turn-taking behavior: Speakers tend to look away from their partners when they begin talking and look back at them when they are about to finish their conversational turn. Gaze direction can also serve as a predictor of gestural feedback (e.g., Morency et al., 2010), signaling to the listener that the speaker is waiting for feedback. Thus, we annotated the occasions where the target participant was looking directly at the screen, which we took as an indication that the gaze was directed at the interlocutor.
3.1.2. Head movements
Head movements have various functions in human face-to-face communication such as giving feedback to—and eliciting feedback from—the interlocutor (Allwood et al., 2005; Paggio and Navarretta, 2013). We annotated two head movements that may play different communicative roles: head nods and head shakes.
3.1.3. Eyebrow displays
Eyebrow movements are among the most commonly employed facial expressions, they can communicate surprise, anger, and confusion. In our annotation scheme, we have two categories for eyebrow movements: raised (lifted eyebrows) or frown (the contraction of eyebrows and movement toward the nose).
3.1.4. Mouth displays
Smiles and laughs are common sources of backchannel communication between participants. They can, e.g., signal to the interlocutor that they are being understood (Brunner, 1979). They can also signal attention when combined with a head nod (Dittmann and Llewellyn, 1968). We annotated two categories of mouth display: smile and laughter.
3.1.5. Posture
Posture also plays a communicative role. In particular, leaning forward can indicate attention and/or positive feedback (Park et al., 2017) and leaning backward may occur as a turn-yielding signal (Allwood et al., 2005). We annotated posture by taking the starting point as the neutral position and any movement from there was tagged either forward or backward.
3.2. Annotation method and inter-rater reliability
The annotation task was structured in the following way: (1) Detecting that a target non-verbal event has occurred during the time course of the conversation; (2) Once the event has been detected, tagging its time interval (i.e., when it begins and ends) and; (3) tagging its category (e.g., smile, head nod, or leaning backward).3 The entire corpus was annotated by the first author. Additionally, a subset of 8 recordings (i.e., 20% of the data) including 4 children and 4 adults were independently annotated by another person in order to estimate inter-annotation agreement.
Estimating the degree of agreement between annotators for time-dependent events is not an easy task as it requires comparing not only agreement on the classification (whether an event is a laugh or a smile) but also agreement on the time segmentation of this event. Mathet et al. (2015) introduced a holistic measure, γ, that takes into account both classification and segmentation, accounting for some phenomena that are not well captured by other existing methods such as Krippendorff's αu (Krippendorff et al., 2016). Such phenomena include when segments overlap in time (e.g., when an interlocutor nods and smiles at the same time) which occur frequently in face-to-face data such as ours. Thus, it is the γ measure that we report in the current study using a Python implementation by Titeux and Riad (2021).
In a nutshell, this method estimates agreement by finding the optimal alignment in time between segments obtained from different annotators. The alignment minimizes both dissimilarity in time correspondence between the segments as well as dissimilarity in their categorization. The optimal alignment is characterized by a disorder value: δ. The measure γ takes into account chance by sampling N random annotations and computing their average disorder: δrandom. Finally, the chance-adjusted γ measure is computed as: . The closer the value to 1, the stronger the agreement between the annotators.4
While γ allows us to obtain a global score for the annotation. We were also interested in the finer-grained analysis of agreement comparing segmentation vs. categorization for each annotated feature. To obtain scores for categorization only, we use the γcat measure introduced by Mathet (2017) which is computed after one finds the optimal alignment using the original γ as described above. To analyze agreement in segmentation, we computed γ for each category separately, thus eliminating all ambiguities in terms of categorization and letting γ deal with agreement in segmentation only.
Regarding agreement in our data, we found an average γav = 0.56 [0.49, 0.66] for children and an average γav = 0.65 [0.59, 0.78] for adults, where ranges correspond to the lowest and highest γ obtained in the four videos we double-annotated in each age group. How to interpret these numbers? Since γ is a new measure, it has not been thoroughly benchmarked with data similar to ours, as compared to more known measures (which, however, are less adequate for our data) such as Cohen's Kappa or Krippendorff's alpha. That said, γ is generally more conservative than other existing measures (Mathet et al., 2015). In addition, γ tends to (over-)penalize several aspects of disagreement in segmentation (see below). Thus, we can consider that the global scores obtained above reflect, overall, good agreement as can be seen in Table 1.
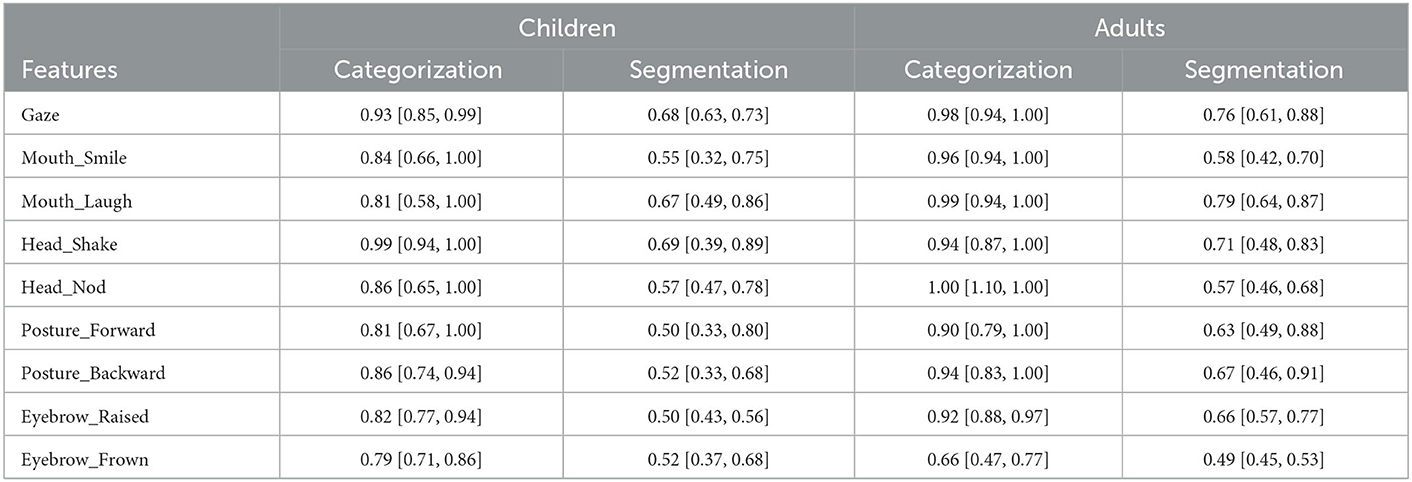
Table 1. Average gamma scores quantifying inter-rater reliability between two annotators using 20% of the corpus. Ranges the indicate lowest and largest gamma in the videos annotated in each age group.
Table 1 shows the detailed scores for each feature. We found that the scores for categorization were very high across all features and in both age groups, indicating that our non-verbal features are highly distinguishable from each other. The scores for segmentation, though overall quite decent (they are overall larger than 0.5 for all features), are much lower compared to categorization. Segmentation is harder because there are several ways disagreement can occur and for which it is penalized by γ. Part of this disagreement is quite relevant and should indeed be penalized such as when only one annotator detects a given event at a given time or when there is a mismatch between annotators in terms of the start and/or the end of the event. However, some aspects of disagreement need not necessarily be penalized such as when one annotator considers that an event is better characterized as a long continuous segment and the other annotator considers, instead, that it is better characterized as a sequence of smaller segments (e.g., a long continuous segment involving multiple consecutive nods vs. several consecutive but discrete segments representing, each, a single nod).
We found some features to be less ambiguous (to human annotators) than others, especially concerning their segmentation. For example, gaze switch, laughter, and head shake were among the least ambiguous for both children and adults. Posture change and eyebrow movement were the most ambiguous in children. Smiles and head nods had an intermediate level of ambiguity in both age groups. Finally, we noted that the agreement scores were overall higher for adults compared to children, indicating that adults' non-verbal behavior was generally less ambiguous to our annotators than children's.
3.3. Distribution of non-verbal signals
Based on our annotations, we quantified non-verbal communication in child-caregiver multimodal conversation compared to the control condition of adult-caregiver conversation. Figure 2 shows the average number of target non-verbal behaviors (e.g., gaze switch, head nod, or smile) per minute in both conditions and for each speaker. We can observe that the frequency distribution is strikingly similar across all speakers. This finding suggests that non-verbal behavior data is quite balanced across children and adults. Thus, our corpus provides a good basis for future studies of how these cues are used to manage conversations and for comparison with how adults behave in similar conversational contexts. The following section is an instance of how this data can be leveraged to focus on one conversational skill, i.e., backchannel signaling.
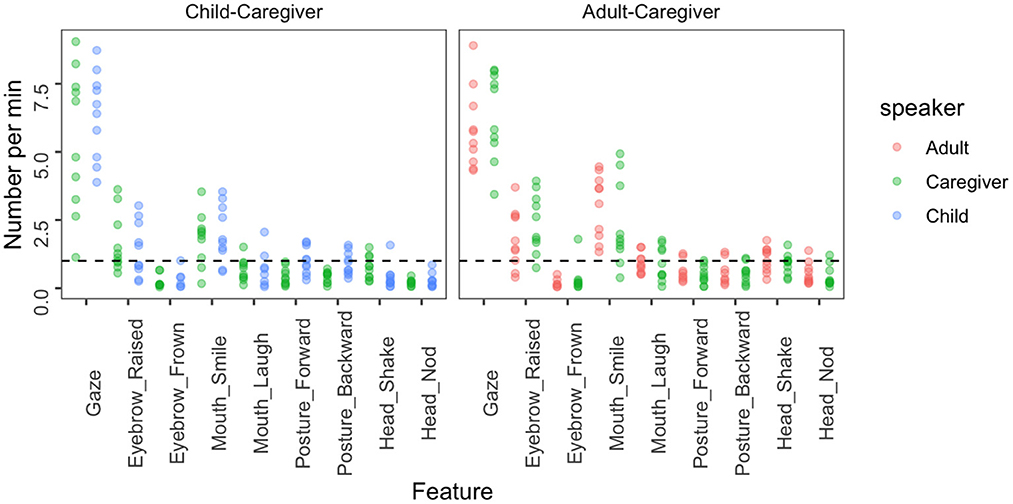
Figure 2. The frequency of use of non-verbal features for each participant in our corpus. The frequency was normalized by the total length (in minutes) of the conversation. The black dotted line represents a frequency of 1 per minute; data above this line can be understood as indicating relatively frequent behavior.
4. Case study: Backchannel signaling
This section provides a case study (illustrated by the examples in Table 2) of how the—rather general purpose—annotated corpus we presented above can be utilized to study specific conversational phenomena. We focus on BC signaling in middle childhood, providing a fresh perspective on the development of this skill compared to previous in-lab, rather controlled studies. In what follows, we present the methods we used to adapt the above-annotated corpus to the needs of this case study, then we present the results of the analyses comparing the child-caregiver dyads to adult-caregivers dyads both in terms of the rate of the listener's BC production and in terms of the distribution of this production across the speaker's contextual cues.
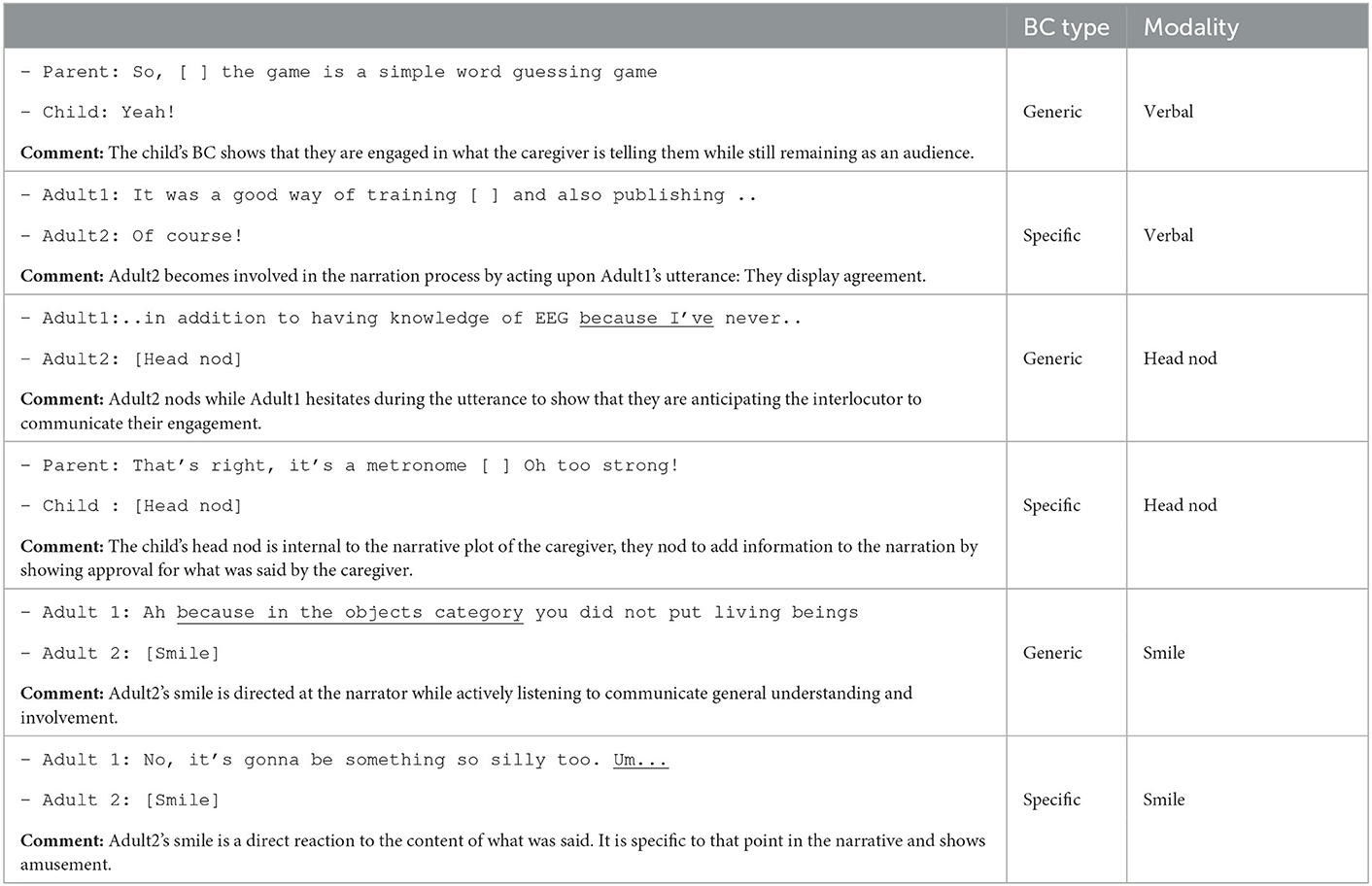
Table 2. Excerpts from conversations in our corpus (translated from French) exemplifying both types of BC in each of the three modalities we consider in this study. BC either occurs during a pause “[ ]” in the speaker's turn or it overlaps with a segment of the speaker's speech (the underlined part). The comment explains the decision to classify the BC as generic or specific, following the distinctions made in Bavelas et al. (2000).
4.1. Methods
4.1.1. Generic vs. specific BC
We adopt the distinction that Bavelas et al. (2000) has established experimentally between two types of BC: “generic” vs. “specific.”5 As its name indicates, specific BC is a reaction to the content of the speaker's utterance. It might indicate the listener's agreement/disagreement, surprise, fear, etc. As for generic BC, it is performed to show that the listener is paying attention to the speaker and keeping up with the conversation without conveying narrative content (see examples in Table 2). It is important to note that, while generic BC does not target the narrative content, it does not mean that it is used randomly. Both generic and specific BCs should be timed precisely and appropriately so as to signal proper attentive listening to the speaker. Otherwise, they can be counter-productive and perceived, rather, as distracting and interrupting, even by young children (e.g., Park et al., 2017). In that sense, both specific and generic BC are expected by interlocutors to be used in a collaborative fashion starting from their early development in childhood.
4.1.2. Family vs. non-family conditions
Previous work has pointed out differences in BC dynamics depending on the degree of familiarity between interlocutors (e.g., Tickle-Degnen and Rosenthal, 1990; Cassell et al., 2007), so we distinguished in the control caregiver-adult conversations between family members (5 dyads) and non-family members (5 dyads). Since interlocutors in the caregiver-adult condition are both mature speakers/users of the language, we did not separate them here based on whether they were the caregiver or adult, but based on whether they belong to family or non-family dyads. Thus, both the family and non-family conditions contain, each, N = 10 individuals, similar to the number of caregivers and children in the child-caregiver condition, making these four categories comparable in our analysis.
4.1.3. Listener's BC
While a multitude of signals can convey some form of specific active listening (surprise, amusement, puzzlement, etc.), here, we made this question more manageable by focusing on a subset of behaviors that can, a priori, be used for both specific BC and generic BC depending on the context of use. This subset is a good test for children's pragmatic ability to encode and decode specific vs. generic BC even when both are expressed by the same behavior. For example, a smile can be both an accommodating gesture (generic) or an expression of amusement (specific) depending on the context (see Table 2).
Based on examination of the set of annotated non-verbal signals in our corpus, we found head nods and smiles to be used by children and adults both as specific and generic BC. Other non-verbal signals (e.g., head shakes, eyebrow displays, and laughs) were almost always specific, and therefore—as we indicated above—were not included in the analysis. Further, and in addition to non-verbal signals, BC can also be expressed verbally as short vocalizations (e.g., “yeah,” “m-hm”). We obtained these signals automatically using the SPPAS software (Bigi, 2015) which segments the audio input into Inter-Pausal Units (IPU) defined as pause-free units of speech. We operationalized a short vocalization as—at least—a 150 ms-long IPU. This threshold was the minimal duration of a verbal BC based on our preliminary trials and hand-checking of a few examples. The automatically detected units were then verified manually, keeping only the real instances of BC.
A second round of annotation concerned the classification of these verbal and non-verbal signals into specific vs. generic BC. The first author annotated the entire set of these signals into specific vs. generic BC. In order to estimate inter-rater reliability, around 20% of the data were annotated independently by a second annotator. We obtained a Cohen Kappa value of κ = 0.66, indicating “moderate” agreement (McHugh, 2012). Table 3 shows some average statistics of Generic vs. Specific BC produced per participant in each modality. It shows that children and adults do produce both types of BC in each of the modalities we consider. Given our limited sample size and in order to optimize statistical power, all subsequent analyses were done by collapsing BC across all these three modalities.
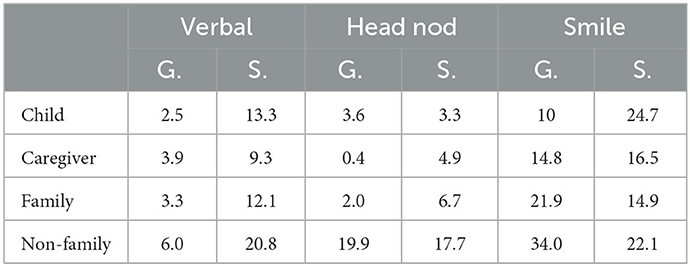
Table 3. Average number of generic vs. specific BC produced per participant in each modality. Note that here the numbers for “Caregiver” only concern their interaction with children. Family and non-Family groups include only participants from the caregiver-adult condition.
4.1.4. Speaker's contextual cues
We also needed annotations of the speaker's contextual cues, i.e., the context of speaking where the listeners produce a BC. We considered two broad speaking contextual cues: a) overlap with speech, that is, when the listener produces a verbal or non-verbal BC without interrupting the speaker's ongoing voice activity, and b) during a pause, i.e., when the listener produces a BC after the speaker pauses for a minimum of 400 ms (following Hess and Johnston, 1988). In addition, we study the subset of cases where these two contexts overlap with the speaker's eye gaze, that is, when the listener produces a BC while the speaker is looking at them vs. while the speaker is looking away. The speaker's continuous segments of speech (i.e., IPUs) vs. pauses were annotated automatically using the Voice Activity Detection of the SPASS software (Bigi, 2012, 2015; Bigi and Meunier, 2018). As for the speaker's gaze (looking at the listener vs. looking away), it was annotated manually (see Section 3 above).
4.2. Results
4.2.1. Rate of BC production
We computed the total number of specific and generic BC produced by each interlocutor, then we divided this number by the length of the conversation in each case to have a measure of the rate of production per minute. Results are shown in Figure 3. When comparing children to caregivers, we found what seems to be a developmental effect regarding the rate of production of specific vs. generic BC. More precisely, children produced specific BC at a higher rate than generic BC. This difference was larger for children than for caregivers. Statistical analysis confirmed this observation: A mixed-effect model predicting only children's rate as a function of BC type6 yielded an effect of β = 1.47 (SE = 0.17, p < 0.001), meaning there was a difference between specific and generic BC rate in children. A second model predicting the rate of production (by both children and caregiver) as a function of both BC type and interlocutor (child or caregiver) 7 showed there to be an interaction β = 0.81 (SE = 0.37, p < 0.05), meaning that the difference between BC types in children is larger than it is in caregivers. As can be seen in Figure 3, and although children produce slightly fewer generic BC, the developmental difference can be largely attributed to children producing more specific BC compared to caregivers.
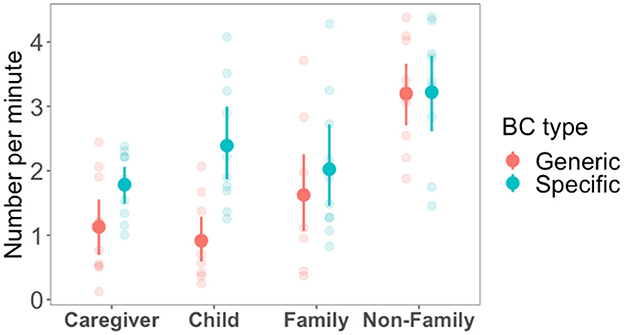
Figure 3. The rate of BC production per minute for both the child-caregiver condition and family vs. non-family in the caregiver-adult condition. Each data point represents a participant and ranges represent 95% confidence intervals.
In the same Figure 3, we also have the rates of production in the Adult-Adult control conditions. While these controls were meant to be contrasted with the child-caregiver dyads, here we noticed an interesting difference among them: Both BC types were higher in the non-family dyads than in the family dyads. Using a mixed-effects model predicting the rate of BC production as a function of type and family membership, 8 we found a main effect of family membership: β = 0.81 (SE = 1.57, p = 0.01) but there was no effect of BC type nor an interaction. By comparing the child-caregiver condition to family vs. non-family of the adult-adult conditions in Figure 3, we make the following observations. Caregivers, when talking to children, produced BC at a rate roughly similar to the one used among adults in the family dyads. The same thing can be said about children: Although they tend to produce slightly more specific BC and slightly fewer generic BC than adults, these differences were small and not statistically significant. This comparison suggests that, overall, children are not less prolific in terms of BC production than adults, at least when adults converse in a family context.
4.2.2. Distribution of BC over speaker's contextual cues
4.2.2.1. Child-Caregiver condition
While the results shown in Figure 3 inform us about the overall rate of BC production, they do not show the speaker's contextual cues where this production occurs. As explained in the Methods subsection, we examined the nature of BC production of the listener during the speaker's speech vs. pause and in the subset of cases where these cues overlap with the speaker's gaze (Kendon, 1967; Kjellmer, 2009; Morency et al., 2010). In Figure 4 we show the rate of BC production of children and caregivers across these cues. The main observation is that children and caregivers produce BC across speaker's cues in roughly similar proportions. Another observation is that, for both interlocutors, while BC in speech almost always coincided with the speaker's gaze at the listener (though this is likely due to a floor effect), this was not the case for BC produced during pauses where part of this production did not coincide with the speaker's gaze (in other words, listeners also provided BC when the speaker paused and looked away). One minor difference between children and caregivers is the following. While their rate of BC during speech was generally low, this rate was slightly higher for children (while almost totally absent for caregivers). The origin of this small difference is unclear: it could be due to children providing fewer BC opportunities for adults to capitalize on, or to adults not providing BC despite such opportunities. Another difference is that caregivers seem to produce slightly more generic BC after pauses than children do (though this difference was not statistically significant).
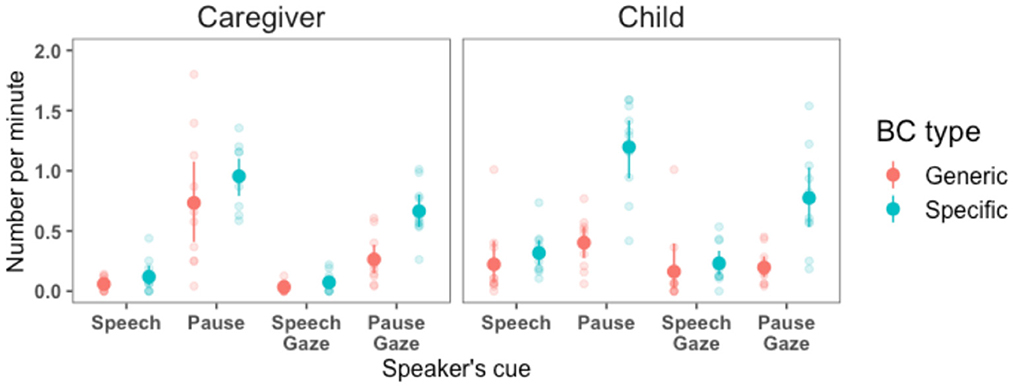
Figure 4. The rate of listener's BC production of children and caregivers across the speaker's speech and pauses (mutually exclusive). We also show the subset of cases where these cues overlap with the speaker's gaze at the listener. Each data point represents a participant and ranges represent 95% confidence intervals.
4.2.2.2. Adult-adult (control) conditions
Next, we examined how BC production varied across the speaker's cues between family and non-family dyads in the adult-adult control conversations. The results are shown in Figure 5. Unsurprisingly, and in line with findings in Figure 3, we observe a general increase in BC production rate among non-family dyads relative to family dyads. However, this increase was–interestingly–not similar across the speaker's cues. In particular, while the average BC production rate remained similar during the speaker's pauses, we observed a striking increase in generic BC produced by non-family listeners during the speaker's speech, going from almost zero to around 1 BC per minute. Indeed, a mixed-effects model predicting the rate of generic BC as a function of family membership showed there to be a strong difference: β = 0.84 (SE = 0.2, p < 0.001). Another observation is that, for both family and non-family dyads, only part of the BCs co-occurred with the speaker's eye gaze toward the listener in both speaker's speech and pause, i.e., many BC occurred when the speaker was looking away. By comparing Figures 4, 5, we conclude that patterns of BC distribution (across speaker's cues) of children and caregivers are not only largely similar to each other, but also similar to the patterns of BC distribution of adult-adult conversations in the family context. In fact, we observed much more differences due to family membership between adults than differences due to developmental age.
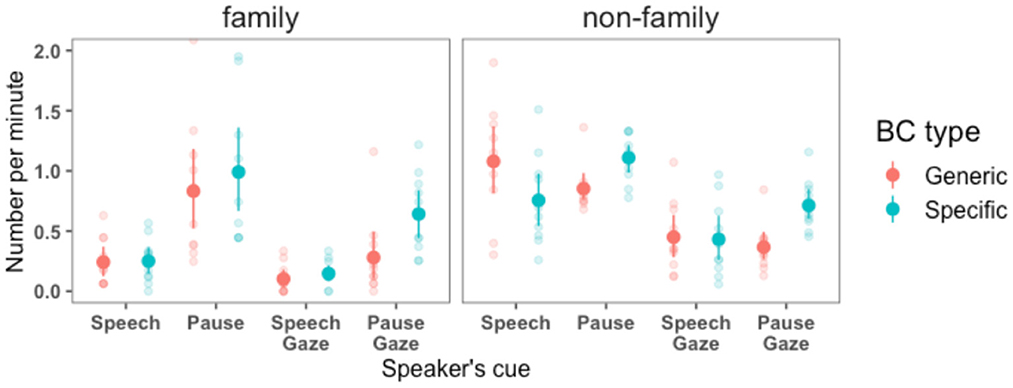
Figure 5. The rate of listener's BC production in family and non-family adult dyads across the speaker's speech and pauses (mutually exclusive). We also show the subset of cases where these cues overlap with the speaker's gaze at the listener. Each data point represents a participant and ranges represent 95% confidence intervals.
4.3. Discussion
This case study focused on BC in child-caregiver conversations and compared children's behavior to adult-level mastery in family and non-family contexts. While previous work (e.g., Dittmann, 1972; Hess and Johnston, 1988) found BC to be still relatively infrequent in middle childhood, here we found that children in the same age range produced BC at a similar rate as in adult-adult conversations when the adults were family members, an arguably more pertinent control condition than when adults are not family members. In the latter, the rate of production of BC was much higher.
An alternative interpretation of children's adult-level BC production is the fact that caregivers may provide children with more BC opportunities than what they would have received otherwise, thus “scaffolding” their BC production. While it is difficult to quantify exhaustively and precisely all BC opportunities, we can have an approximation by counting all pauses of at least 400 ms between two successive sound segments (or IPUs) of the same speaker. Using this rough estimate9, Figure 6 shows that, indeed, children are offered slightly more opportunities by the caregiver than the other way around. However, the number of BC opportunities is higher in adult-adult conversations. Thus, the number of BC opportunities alone does not explain why children still produce BC at a similar rate as adults in the family dyads.
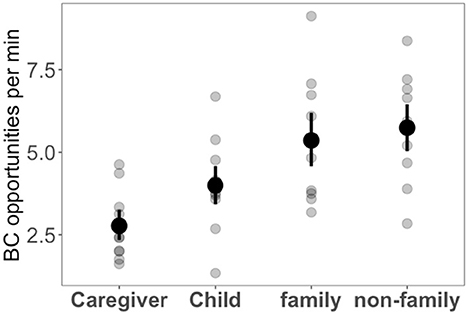
Figure 6. The rate of BC (received) opportunities. Opportunities were defined roughly as pauses of at least 400 ms between two successive sound segments (or IPUs) of the speaker.
Regarding the distinction between specific vs. generic BC, we found that children used verbal and non-verbal behavior to signal fewer generic BC compared to specific BC. However, this result does not necessarily mean children find generic BC harder. Indeed, the rate of generic BC was very low for both children and adults in family dyads. Findings from the non-family control condition suggest a better interpretation of this result. In this condition, adults provided a much higher rate of generic BC. We speculate that the participants used more generic BC (e.g., smiles) to establish social rapport with a stranger. In family dyads (and regardless of the interlocutor's ages), however, social rapport is already established, requiring less explicit accommodating signals (see also Tickle-Degnen and Rosenthal, 1990; Cassell et al., 2007).
5. Conclusions
This paper introduced a new data acquisition method for the study of children's face-to-face conversational skills. It consists in using online video calls to record children with their caregivers at home. Compared to existing datasets of spontaneous, multimodal child-caregiver interactions in the wild (e.g., MacWhinney, 2014; Sullivan et al., 2022), our method allows much clearer access to the interlocutor's facial expressions and head gestures, thus facilitating the study of conversational development. We collected an initial corpus using this data acquisition method which we hand-annotated for various multimodal communicative signals. The analysis of these annotations revealed that children in middle childhood use non-verbal cues in conversation almost as frequently as adults do. In our corpus, interlocutors played a word-guessing game instead of chatting about random topics. This game was used here to elicit balanced conversational exchange in an interactive context where such a criterion is difficult to meet due to obvious social and developmental asymmetries between the interlocutors. That said, the word-guessing game remains highly flexible and useful for investigating a wide range of research questions.
We illustrated the usefulness of such data by focusing on a specific conversational skill: Backchannel signaling, which—despite its importance for coordinated communication (Clark, 1996)—has received little attention in the developmental literature. The findings from this case study have confirmed our prediction that using a new data acquisition method, which improves the naturalness of the exchange (i.e., conversation with a caregiver, at home, and using a fun/easy game), allows us to capture more of children's conversational competencies. In particular, here we found, by contrast to previous in-lab studies, that school-age children's rate and context of BC production is strikingly close to adult-level mastery.
Backchannel signaling is only an illustration; many other aspects of conversational development can be studied with these data. In particular, ongoing research aims at characterizing children's multimodal alignment, a phenomenon whereby interlocutors tend to repeat each other's verbal and non-verbal behavior. Alignment is believed to be associated with the collaborative process of building mutual understanding and, thus, communicative success more generally (Brennan and Clark, 1996; Pickering and Garrod, 2006; Rasenberg et al., 2020). For example, Mazzocconi et al. (2023) used our corpus to compare laughter alignment/mimicry in child-caregiver and caregiver-adult interactions. They found that, although laughter occurrences were comparable between children and adults (as we report here in Figure 2), laughter mimicry was overall significantly less frequent in child-caregiver interactions in comparison to caregiver-adult interactions, the latter being similar to what was observed in previous studies of adult face-to-face interactions (e.g., Mazzocconi et al., 2020).
Finally, a major advantage of the zoom-based data acquisition method is its cost-effectiveness, allowing large-scale data collection including from different cultures. In the current paper, however, our goal was not only to collect data but also to provide manual annotation for various communicative signals, a labor-intensive task. Thus, we settled on a manageable sample size. That said, progress in the automatic annotation of children's multimodal data (Sagae et al., 2007; Nikolaus et al., 2021; Rooksby et al., 2021; Erel et al., 2022; Long et al., 2022) should alleviate the constraint on large-scale data collection in future research. The current work also contributes to this effort by providing substantial hand-annotated data that can be used for the automatic models' training and/or validation.
5.1. Limitations
We used video calls as a way to collect face-to-face conversational data in a naturalistic context (e.g., at home instead of the lab). However, this method involves introducing a medium (i.e., a screen) that has obvious constraints and the participants may be adapting to—or influenced by—these constraints. For example, in the case of BC, we noticed some differences with previous work that has studied adult BC in direct face-to-face conversations in the same culture/country (i.e., France) (Prévot et al., 2017; Boudin et al., 2021). In particular, our rate of BC production in adults was overall lower. Besides, our number of verbal BC compared to non-verbal BC was also lower (see Table 3).10 Nevertheless, our goal is generally to compare children and adults; the constraints due to introducing a medium of communication apply equally to both children and adults, thus the comparison remains valid, albeit in this specific, mediated conversational context.
BC behavior can also be influenced by internet issues such as time lags (Boland et al., 2021), possibly disturbing the appropriate timing and anticipation of conversational moves. That said, our preliminary testing (and, then, the full annotation of the data) has shown that, if there were lags, they must have been minimal compared to the time scale of BC dynamics. The production of BC did not seem to be disrupted nor disruptive (to the interlocutor). However, further research is required to precisely quantify the potential effect that online video call systems might have on BC and conversational coordination more generally.
Author's note
The manuscript is based on one work presented in the “2021 International Workshop on Corpora And Tools for Social skills Annotation” and a second work presented (as a non-archival proceeding) in the “2022 Annual Meeting of the Cognitive Science Society.”
Data availability statement
The original contributions presented in the study are included in the article/supplementary material, further inquiries can be directed to the corresponding author.
Ethics statement
The studies involving human participants were reviewed and approved by Aix-Marseille University. Written informed consent to participate in this study was provided by the participants' legal guardian/next of kin.
Author contributions
KB, MN, LP, and AF designed research and wrote the paper. KB performed research. KB and AF analyzed data. All authors contributed to the article and approved the submitted version.
Funding
This research was supported by grants ANR-16-CONV-0002 (ILCB), ANR-11-LABX-0036 (BLRI), ANR-21-CE28-0005-01 (MACOMIC), AMX-19-IET-009 (Archimedes Institute), ED356, and the Excellence Initiative of Aix-Marseille University (A*MIDEX).
Acknowledgments
We would like to thank Fatima Kassim for help with the annotation. We also thank Auriane Boudin, Philippe Blache, Noël Nguyen, Roxane Bertrand, Christine Meunier, and Stéphane Rauzy for useful discussion. Finally, we thank all families that have volunteered to participate in data collection.
Conflict of interest
The authors declare that the research was conducted in the absence of any commercial or financial relationships that could be construed as a potential conflict of interest.
Publisher's note
All claims expressed in this article are solely those of the authors and do not necessarily represent those of their affiliated organizations, or those of the publisher, the editors and the reviewers. Any product that may be evaluated in this article, or claim that may be made by its manufacturer, is not guaranteed or endorsed by the publisher.
Footnotes
1. ^If neither of the interlocutors wears a headphone, Zoom tends to automatically cut the sound of one speaker when the speakers happen to talk over each other, which is an undesirable feature for the purpose of our data collection, and in particular for the study of BC.
2. ^The corpus was also fully transcribed manually. Here we focus on the more challenging task of coding the non-verbal signals.
3. ^Note that similar visual events may vary in their precise communicative functions. For example, a head nod can be used either as a non-verbal answer to a yes-no question or as a BC, indicating attention/understanding without necessarily signaling agreement. Such distinctions have not been made at this stage of this annotation (but see the case study on BC, below, for further specifications of some communicative moves). Here we annotated the above-described features regardless of their precise communicative role in the conversation.
4. ^N is chosen so that γ has a default precision level of 2%, we did not change this default value in the current work.
5. ^A roughly similar distinction has been proposed in Conversational Analysis literature (Schegloff, 1982; Goodwin, 1986), using the terms “continuers” and “assessment.”
6. ^Specified as Rate ~ BC_type + (1 | dyad).
7. ^Specified as Rate ~ BC_type*Interlocutor + (1 | dyad).
8. ^Specified as Rate ~ BC_type*Family + (1 | dyad).
9. ^Note that this measure approximates BC opportunities only in the context of the speaker's pauses, not opportunities for BCs that overlap with the speaker's speech. Estimating the latter requires investigating finer-grained cues within speech such as intonation and clause boundaries.
10. ^That said, there are other factors that may have caused these differences, namely, our use of a new elicitation task, i.e., the word-guessing game.
References
Allwood, J., Cerrato, L., Dybkjaer, L., Jokinen, K., Navarretta, C., and Paggio, P. (2005). “The MUMIN multimodal coding scheme,” in NorFA Yearbook, 129–157.
Anderson, A., Thompson, H. S., Bard, E. G., Doherty-Sneddon, G., Newlands, A., and Sotillo, C. (1993). “The HCRC map task corpus: natural dialogue for speech recognition,” in Proceedings of the Workshop on Human Language Technology, HLT '93 (Plainsboro, NJ: Association for Computational Linguistics), 25–30.
Anderson, A. H., Bader, M., Bard, E. G., Boyle, E., Doherty, G., Garrod, S., et al. (1991). The hcrc map task corpus. Lang. Speech 34, 351–366. doi: 10.1177/002383099103400404
Baines, E., and Howe, C. (2010). Discourse topic management and discussion skills in middle childhood: the effects of age and task. First Lang. 30, 508–534. doi: 10.1177/0142723710370538
Bavelas, J. B., Coates, L., and Johnson, T. (2000). Listeners as co-narrators. J. Pers. Soc. Psychol. 79, 941. doi: 10.1037/0022-3514.79.6.941
Bigi, B. (2012). “SPPAS: a tool for the phonetic segmentations of speech,” in The Eighth International Conference on Language Resources and Evaluation (Istanbul), 1748–1755.
Bigi, B. (2015). SPPAS-multi-lingual approaches to the automatic annotation of speech. the phonetician. J. Int. Soc. Phonetic Sci. 111, 54–69.
Bigi, B., and Meunier, C. (2018). Automatic segmentation of spontaneous speech. Revista de Estudos da Linguagem 26, 1530. doi: 10.17851/2237-2083.26.4.1489-1530
Boland, J. E., Fonseca, P., Mermelstein, I., and Williamson, M. (2021). Zoom disrupts the rhythm of conversation. J. Exp. Psychol. Gen. 151, 1272–1282. doi: 10.1037/xge0001150
Boudin, A., Bertrand, R., Rauzy, S., Ochs, M., and Blache, P. (2021). “A multimodal model for predicting conversational feedbacks,” in International Conference on Text, Speech, and Dialogue (Olomouc: Springer), 537–549.
Brennan, S. E., and Clark, H. H. (1996). Conceptual pacts and lexical choice in conversation. J. Exp. Psychol. Learn. Mem. Cogn. 22, 1482. doi: 10.1037/0278-7393.22.6.1482
Brunner, L. J. (1979). Smiles can be backchannels. J. Pers. Soc. Psychol. 37, 728. doi: 10.1037/0022-3514.37.5.728
Cassell, J., Gill, A., and Tepper, P. (2007). “Coordination in conversation and rapport,” in Proceedings of the Workshop on Embodied Language Processing (Prague: Association for Computational Linguistics), 41–50.
Clark, E. V. (2018). Conversation and language acquisition: a pragmatic approach. Lang. Learn. Dev. 14, 170–185. doi: 10.1080/15475441.2017.1340843
Dideriksen, C., Fusaroli, R., Tylén, K., Dingemanse, M., and Christiansen, M. H. (2019). “Contextualizing conversational strategies: backchannel, repair and linguistic alignment in spontaneous and task-oriented conversations,” in CogSci'19 (Montreal, QC: Cognitive Science Society), 261–267.
Dittmann, A. T. (1972). Developmental factors in conversational behavior. J. Commun. 22, 404–423. doi: 10.1111/j.1460-2466.1972.tb00165.x
Dittmann, A. T., and Llewellyn, L. G. (1968). Relationship between vocalizations and head nods as listener responses. J. Pers. Soc. Psychol. 9, 79. doi: 10.1037/h0025722
Dorval, B., and Eckerman, C. O. (1984). Developmental trends in the quality of conversation achieved by small groups of acquainted peers. Monogr. Soc. Res. Child Dev. 49, 1–72. doi: 10.2307/1165872
Erel, Y., Potter, C. E., Jaffe-Dax, S., Lew-Williams, C., and Bermano, A. H. (2022). iCatcher: a neural network approach for automated coding of young children's eye movements. Infancy 27, 765–779. doi: 10.1111/infa.12468
Foushee, R., Byrne, D., Casillas, M., and Goldin-Meadow, S. (2022). “Getting to the root of linguistic alignment: Testing the predictions of interactive alignment across developmental and biological variation in language skill,” in Proceedings of the 44th Annual Meeting of the Cognitive Science Society (Toronto, ON).
Fusaroli, R., Ra̧czaszek-Leonardi, J., and Tylén, K. (2014). Dialog as interpersonal synergy. New Ideas Psychol. 32, 147–157. doi: 10.1016/j.newideapsych.2013.03.005
Fusaroli, R., Weed, E., Fein, D., and Naigles, L. (2021). Caregiver linguistic alignment to autistic and typically developing children. PsyArXiv [Preprint]. doi: 10.31234/osf.io/ysjec
Goodwin, C. (1986). Between and within: alternative sequential treatments of continuers and assessments. Hum. Stud. 9, 205–217. doi: 10.1007/BF00148127
Hale, C. M., and Tager-Flusberg, H. (2005). Social communication in children with autism: the relationship between theory of mind and discourse development. Autism 9, 157–178. doi: 10.1177/1362361305051395
Hazan, V., Pettinato, M., and Tuomainen, O. (2017). kidlucid: London ucl children's clear speech in interaction database.
Hess, L., and Johnston, J. (1988). Acquisition of backchannel listener responses to adequate messages. Commun. Sci. Disord. Faculty Publications 11, 319–335. doi: 10.1080/01638538809544706
Kendon, A. (1967). Some functions of gaze-direction in social interaction. Acta Psychol. 26, 22–63. doi: 10.1016/0001-6918(67)90005-4
Kjellmer, G. (2009). Where do we backchannel?: on the use of mm, mhm, uh huh and such like. Int. J. Corpus Linguist. 14, 81–112. doi: 10.1075/ijcl.14.1.05kje
Krason, A., Fenton, R., Varley, R., and Vigliocco, G. (2022). The role of iconic gestures and mouth movements in face-to-face communication. Psychonomic Bull. Rev. 29, 600–612. doi: 10.3758/s13423-021-02009-5
Krippendorff, K., Mathet, Y., Bouvry, S., and Widlöcher, A. (2016). On the reliability of unitizing textual continua: Further developments. Quality Quantity 50, 2347–2364. doi: 10.1007/s11135-015-0266-1
Laland, K., and Seed, A. (2021). Understanding human cognitive uniqueness. Annu. Rev. Psychol. 72, 689–716. doi: 10.1146/annurev-psych-062220-051256
Leung, A., Tunkel, A., and Yurovsky, D. (2021). Parents fine-tune their speech to children's vocabulary knowledge. Psychol. Sci. 32, 975–984. doi: 10.1177/0956797621993104
Levinson, S. C., and Holler, J. (2014). The origin of human multi-modal communication. Philos. Trans. R. Soc. B Biol. Sci. 369, 20130302. doi: 10.1098/rstb.2013.0302
Long, B. L., Kachergis, G., Agrawal, K., and Frank, M. C. (2022). A longitudinal analysis of the social information in infants' naturalistic visual experience using automated detections. Dev. Psychol. 2022, 1414. doi: 10.1037/dev0001414
MacWhinney, B. (2014). The CHILDES Project: Tools for Analyzing talk, Volume II: The Database. Psychology Press.
Maroni, B., Gnisci, A., and Pontecorvo, C. (2008). Turn-taking in classroom interactions: Overlapping, interruptions and pauses in primary school. Euro. J. Psychol. Educ. 23, 59–76.
Mathet, Y. (2017). The agreement measure γ cat a complement to γ focused on categorization of a continuum. Comput. Linguist. 43, 661–681. doi: 10.1162/COLI_a_00296
Mathet, Y., Widlöcher, A., and Métivier, J.-P. (2015). The unified and holistic method Gamma (γ) for inter-annotator agreement measure and alignment. Comput. Linguist. 41, 437–479. doi: 10.1162/COLI_a_00227
Mazzocconi, C., Haddad, K. E., O'Brien, B., Bodur, K., and Fourtassi, A. (2023). “Laughter mimicry in parent-child and parent-adult interaction,” in International Multimodal Communication Symposium (MMSYM) (Barcelona).
Mazzocconi, C., Tian, Y., and Ginzburg, J. (2020). What's your laughter doing there? a taxonomy of the pragmatic functions of laughter. IEEE Trans. Affect. Comput. 13, 1302–1321. doi: 10.1109/TAFFC.2020.2994533
McHugh, M. L. (2012). Interrater reliability: the Kappa statistic. Biochem. Med. 22, 276–282. doi: 10.11613/BM.2012.031
Misiek, T., Favre, B., and Fourtassi, A. (2020). “Development of multi-level linguistic alignment in child-adult conversations,” in Proceedings of the Workshop on Cognitive Modeling and Computational Linguistics (Punta Cana), 54–58.
Misiek, T., and Fourtassi, A. (2022). “Caregivers exaggerate their lexical alignment to young children across several cultures,” in Proceedings of the 26th Workshop on the Semantics and Pragmatics of Dialogue (Dublin).
Morency, L.-P., de Kok, I., and Gratch, J. (2010). A probabilistic multimodal approach for predicting listener backchannels. Auton. Agent Multi Agent Syst. 20, 70–84. doi: 10.1007/s10458-009-9092-y
Murphy, S. M., Faulkner, D. M., and Farley, L. R. (2014). The behaviour of young children with social communication disorders during dyadic interaction with peers. J. Abnorm. Child Psychol. 42, 277–289. doi: 10.1007/s10802-013-9772-6
Nadig, A., Lee, I., Singh, L., Bosshart, K., and Ozonoff, S. (2010). How does the topic of conversation affect verbal exchange and eye gaze? A comparison between typical development and high-functioning autism. Neuropsychologia 48, 2730–2739. doi: 10.1016/j.neuropsychologia.2010.05.020
Nikolaus, M., and Fourtassi, A. (2023). Communicative feedback in language acquisition. New Ideas Psychol. 68, 100985. doi: 10.1016/j.newideapsych.2022.100985
Nikolaus, M., Maes, J., Auguste, J., Prevot, L., and Fourtassi, A. (2021). “Large-scale study of speech acts' development using automatic labelling,” in Proceedings of the 43rd Annual Meeting of the Cognitive Science Society.
Özyürek, A. (2018). “Role of gesture in language processing: Toward a unified account for production and comprehension,” in Oxford Handbook of Psycholinguistics (Oxford: Oxford University Press), 592–607.
Paggio, P., and Navarretta, C. (2013). Head movements, facial expressions and feedback in conversations: empirical evidence from Danish multimodal data. J. Multimodal User Interfaces 7, 29–37. doi: 10.1007/s12193-012-0105-9
Park, H. W., Gelsomini, M., Lee, J. J., and Breazeal, C. (2017). “Telling stories to robots: The effect of backchanneling on a child's storytelling,” in Proceedings of the 2017 ACM. IEEE International Conference on Human-Robot Interaction (Singapore: IEEE), 2308–2314.
Peterson, C. (1990). The who, when and where of early narratives. J. Child Lang. 17, 433–455. doi: 10.1017/S0305000900013854
Pickering, M. J., and Garrod, S. (2006). Alignment as the basis for successful communication. Res. Lang. Comput. 4, 203–228. doi: 10.1007/s11168-006-9004-0
Pickering, M. J., and Garrod, S. (2021). Understanding Dialogue: Language use and Social Interaction. Cambridge: University Press.
Prévot, L., Gorisch, J., Bertrand, R., Gorene, E., and Bigi, B. (2017). “A sip of CoFee: a sample of interesting productions of conversational feedback,” in Proceedings of the 16th Annual Meeting of the Special Interest Group on Discourse and Dialogue (SIGdial), Prague, Czech Republic, 2-4 September 2015 (Prague: Association for Computational Linguistics), 149–153.
Rasenberg, M., Özyürek, A., and Dingemanse, M. (2020). Alignment in multimodal interaction: an integrative framework. Cogn. Sci. 44, e12911. doi: 10.1111/cogs.12911
Roffo, G., Vo, D.-B., Tayarani, M., Rooksby, M., Sorrentino, A., Di Folco, S., et al. (2019). “Automating the administration and analysis of psychiatric tests: the case of attachment in school age children,” in Proceedings of the 2019 CHI Conference on Human Factors in Computing Systems (Glasgow), 1–12.
Rooksby, M., Di Folco, S., Tayarani, M., Vo, D.-B., Huan, R., Vinciarelli, A., et al. (2021). The school attachment monitor–a novel computational tool for assessment of attachment in middle childhood. PLoS ONE 16, e0240277. doi: 10.1371/journal.pone.0240277
Sacks, H., Schegloff, E. A., and Jefferson, G. (1974). A simplest systematics for the organization of turn-taking for conversation. Language 50, 696–735. doi: 10.1353/lan.1974.0010
Sagae, K., Davis, E., Lavie, A., MacWhinney, B., and Wintner, S. (2007). “High-accuracy annotation and parsing of CHILDES transcripts,” in Proceedings of the Workshop on Cognitive Aspects of Computational Language Acquisition (Prague), 25–32.
Schegloff, E. A. (1982). Discourse as an interactional achievement: some uses of ‘uh-huh' and other things that come between sentences. Analyzing Discourse 71, 93.
Shatz, M., and Gelman, R. (1973). “The development of communication skills: modifications in the speech of young children as a function of listener,” in Monographs of the Society for Research in Child Development, 1–38.
Snow, C. E. (1977). The development of conversation between mothers and babies. J. Child Lang. 4, 1–22. doi: 10.1017/S0305000900000453
Sullivan, J., Mei, M., Perfors, A., Wojcik, E., and Frank, M. C. (2022). SAYCam: a large, longitudinal audiovisual dataset recorded from the infant's perspective. Open Mind 5, 20–29. doi: 10.1162/opmi_a_00039
Tickle-Degnen, L., and Rosenthal, R. (1990). The nature of rapport and its nonverbal correlates. Psychol. Inq. 1, 285–293. doi: 10.1207/s15327965pli0104_1
Titeux, H., and Riad, R. (2021). Pygamma-agreement: gamma γ measure for inter/intra-annotator agreement in Python. J. Open Source Software 6, 2989. doi: 10.21105/joss.02989
Tomasello, M. (1999). The Cultural Origins of Human Cognition. Cambridge, MA: Harvard University Press.
Turing, A. M. (1950). I.–computing machinery and intelligence. Mind LIX 433–460. doi: 10.1093/mind/LIX.236.433
Van Engen, K. J., Baese-Berk, M., Baker, R. E., Choi, A., Kim, M., and Bradlow, A. R. (2010). The wildcat corpus of native-and foreign-accented english: communicative efficiency across conversational dyads with varying language alignment profiles. Lang. Speech 53, 510–540. doi: 10.1177/0023830910372495
Vo, D. B., Brewster, S., and Vinciarelli, A. (2020). “Did the children behave? investigating the relationship between attachment condition and child computer interaction,” in Proceedings of the 2020 International Conference on Multimodal Interaction (Utrecht), 88–96.
Warlaumont, A. S., Richards, J. A., Gilkerson, J., and Oller, D. K. (2014). A social feedback loop for speech development and its reduction in autism. Psychol. Sci. 25, 1314–1324. doi: 10.1177/0956797614531023
Yngve, V. H. (1970). “On getting a word in edgewise,” in Chicago Linguistics Society, 6th Meeting, Vol. 1970 (Chicago, IL), 567–578.
Appendix: Instructions given to the caregivers
The following instructions were given to the caregivers in written format an hour before the recording as well as verbally right before the beginning of the recording. The caregivers were allowed to ask questions–if they had any–to the experimenter until the recording started.
First step: Connecting to Zoom
You'll need two computers to connect separately on Zoom (it is preferable for the quality of audio that they be in different rooms).
Make sure to use headphones for improved audio quality (not required for your child).
Follow the link sent by the experimenter in order to connect both computers to Zoom.
If Zoom is not installed on your computer, you can install it by following the link.
Once the installation is complete, you will be directed to a Zoom window. There you will see the experimenter who will be recording your interaction for the study.
Make sure to expand the zoom window to full screen and to pin your video on your child's screen (and his/hers on your screen).
Second step: The Word-Guessing Game
In this task, we ask you to play a “Guess the Word” game with your child for 10 minutes. The goal is to have an active conversation where one asks questions and the other answers them until the chosen word has been found. Try guessing as many as possible!
- You can ask any question (not only yes/no questions).
- You can give small hints if it gets too complicated.
1. The caregiver explains the task to the child.
2. The parent starts with a word that they can choose from a list of words that was provided to them prior to the recording (see the list below).
3. Once ready, indicate that you have your word so that the child could start asking questions about it.
4. The child asks his first question in order to find out the parent's first word.
5. The parent answers the questions while paying attention to not answering the questions that give away the word.
6. The pair continues interacting until the word is found.
7. Once the word is correctly guessed, it's the child's turn.
8. The child starts with a word of their choice and answers the questions about the word until the parent finds it out.
9. After each correctly guessed word, it's the other participant's turn.
10. They should try to guess as many words as possible in 10 minutes.
11. At the end of 10 minutes, the parent initiates a spontaneous conversation with the child. They can discuss how they did in this game, what they think of it etc. (this part is also recorded as part of the data).
List of words provided to the caregivers (only in the child-caregiver condition)
• Brosse à dents (toothbrush)
• Dauphin (dolphin)
• Fraise (strawberry)
• Policier (police officer)
• Vélo (bike)
• Lune (moon)
• Oreille (ear)
• Anniversaire (birthday)
Keywords: conversation, language acquisition, conversational development, cognitive development, nonverbal, backchannel
Citation: Bodur K, Nikolaus M, Prévot L and Fourtassi A (2023) Using video calls to study children's conversational development: The case of backchannel signaling. Front. Comput. Sci. 5:1088752. doi: 10.3389/fcomp.2023.1088752
Received: 03 November 2022; Accepted: 12 January 2023;
Published: 03 February 2023.
Edited by:
Mathieu Chollet, University of Glasgow, United KingdomReviewed by:
Dong Bach Vo, École Nationale de l'Aviation Civile (ENAC), FrancePatrizia Paggio, University of Copenhagen, Denmark
Copyright © 2023 Bodur, Nikolaus, Prévot and Fourtassi. This is an open-access article distributed under the terms of the Creative Commons Attribution License (CC BY). The use, distribution or reproduction in other forums is permitted, provided the original author(s) and the copyright owner(s) are credited and that the original publication in this journal is cited, in accordance with accepted academic practice. No use, distribution or reproduction is permitted which does not comply with these terms.
*Correspondence: Kübra Bodur, a3VicmEuYm9kdXImI3gwMDA0MDt1bml2LWFtdS5mcg==