- 1CNRS, LIS, Aix Marseille Univ, Université de Toulon, Marseille, France
- 2CNRS, ISM, Aix Marseille Univ, Université de Toulon, Marseille, France
- 3CNRS, LPL, Aix Marseille Univ, Université de Toulon, Marseille, France
In this article, we present a new multimodal annotated corpus of forum theater scenes on discrimination awareness. The collected interactions include scenes of ordinary sexism and racism situations played out by different actors in different contexts. The corpus also contains scenes of interactions between an author of discriminatory behavior and a witness trying to make the discriminatory actor aware of their behavior. These confrontations scenes have been played considering different social attitudes (denial, aggressive, and conciliatory). The experimental setup, including motion capture and audio-visual recordings, has been specifically designed to allow the semi-automatic annotation of the corpus and a fine-grained analysis of the multimodal cues associated with social behaviors.
1. Introduction
In the field of training, a growing interest has emerged in “training through simulation” based on virtual environments. Several studies have been carried out on devices that simulate a social interaction with an embodied conversational agent (ECA) to train individuals' social skills (“Virtual Agents for Social Skills Training,” Bruijnes et al., 2019). These studies have shown that ECAs can improve an individual's social skills, for example, in job interview training (Anderson et al., 2013), cultural awareness (Hall et al., 2011), public speaking (Chollet et al., 2018), or even when training doctors to break bad news to patients (Ochs et al., 2019). To date, however, the research in this area remains limited, and several application fields remain unexplored: for example, training to prevent social discrimination.
According to the latest figures from a European survey conducted in 2019, 60% of women experience some form of sexism at work. Most still react with resignation. In France, 30% of employees have been victims or witnesses of sexism and 28% of racism.1 Discrimination can take very different forms, ranging from inappropriate comments or behavior to harassment. This behavior has a direct impact on the wellbeing of individuals in a company but also on their professional integration and performance (Dardenne et al., 2007). Fighting against social discrimination, first of all, requires employees (who may be victims or witnesses) become aware of the different forms of discrimination; in particular, so-called ordinary sexism and racism. Ordinary sexism is defined as “stereotypes and collective representations that translate into words, gestures, behaviors or actions that exclude, marginalize or inferiorize women” (Grésy, 2009); for example, sexist remarks and jokes or devious seduction. Ordinary racism is expressed “in all those prejudiced phrases and attitudes that we hear or observe on a daily basis. They are not legally actionable, but are nevertheless microaggressions” (Diallo and Sassoon, 2015). Beyond awareness, it is essential to train individuals to react when they witness these forms of discrimination. However, reacting as a witness to a form of sexism or racism in the workplace is a difficult task.
The final objective of our research project is to design and deploy a virtual reality training tool to raise awareness of situations involving social discrimination (ethnic and gendered) and to train individuals to react when they witness such situations. The tool is inspired by a method of interactive theater called the forum theater technique. The forum theater is an interactive theater method created by Augusto Boal in the 1960s (Boal, 1972). Considered a popular education method, the forum theater is nowadays used to raise awareness of societal issues (e.g., discrimination and violence). This method is based on the staging of a problematic situation played by actors and then the participation of spectators who are invited to take the role of an actor in a scene to try to build an alternative to the story. Several theater companies and training firms now offer this method to sensitize individuals in the public and professional spheres to the issue of social discrimination (ethnic, gender, disability, etc.). The forum theater is not limited to raising awareness on a particular subject but allows individuals to develop and simulate concrete strategies that they can then use it in their daily lives to deal with these types of situations. A number of studies have shown the significant benefits of this method for awareness and subsequent behavioral changes (Yves, 1999; Gret, 2012; Assencio, 2016; Bélier, 2019). The final tool of our project will expose the user to a scene involving discrimination simulated by autonomous virtual characters through a virtual reality headset. The user will be instructed to converse with the virtual actor displaying discriminating behavior to make the virtual actor aware of their behavior. Different interactive situations will be simulated involving different types of social discrimination (ordinary sexism and ordinary racism) in different social contexts (e.g., hierarchical relations, opposite genders) and through various socio-emotional behaviors of the virtual actors (conciliatory or aggressive attitudes, etc.). The virtual actors will be integrated into a VR platform that simulates social interactions using natural language. The goal is to deploy a tool that can be used on a large scale to make individuals aware of discriminating social behaviors and train them to deal with such situations.
In order to create such a training tool, our first step was to collect a corpus of the forum theater on discrimination. The objective is then to use this corpus for two purposes: (1) to re-simulate discrimination scenes with virtual actors and (2) to model the discriminatory actor's behavior to be able to replay this behavior using a virtual actor interacting with a user. As far as we know, a corpus of the forum theater on discrimination does not exist. In this article, we present the forum theater corpus for discrimination awareness. We focus particularly on two types of discrimination : The ordinary sexism and racism. The corpus has been specifically collected in order to easily re-simulate the actors' behaviors on virtual ones and also to enable automatic annotations for multimodal behavior analysis.
The article is organized as follows. In the next section, we present the different scenarios developed for discrimination awareness. In Section 3, we present the experimental design. Section 4 is dedicated to the presentation of the annotations in the corpus. In Section 5, we illustrate how the corpus is used in order to create a virtual reality forum theater. We conclude Section 6.
2. Scenarios for discrimination awareness
A forum theater performance is composed of two stages. First, the forum theater begins with a short performance, either rehearsed or improvised, to highlight a social or a political problem. In our context, this short performance corresponds to a scene of ordinary racism or sexism between two actors. This stage is called in our corpus the discrimination scenes. Then, in the second stage, in a forum theater, a spectator can take the role of an actor in the scene to try to build an alternative to the story. In our context, this stage corresponds to the situation where another actor simulates a witness trying to make the discriminatory actor aware of his behavior. These scenes are called the confrontation scenes in our corpus.
A total of six professional actors (three women and three men) from the company NextLevel were recruited for the forum theater simulation to provide a variety of situations and behaviors. The recruited actors have strong experience in the forum theater providing training with this technique in several companies for discrimination awareness. Two scenarios of ordinary sexism and one of the ordinary racism have been defined with the company NextLevel to vary the types of discrimination, the hierarchical relations, and the gender of the actors.
2.1. The discrimination scenes
The context of the scenarios is professional situations. Concerning ordinary sexism scenarios, they have been written based on the description of ordinary sexism in professional situations (Grésy, 2009, 2015). They include the common sexists' behaviors: the condescension and paternalism (e.g., “my sweetheart” or “my lovely”); the denigration (e.g., “ you don't have the capacity for this job”); the indirect seduction (reminders to behave according to an agreed-upon female model); the maternity offense (“it is not the good time to have another child”); the part-time work (“You're lucky you're not doing anything tomorrow, Wednesday”); and the sexist remarks and jokes. The first sexism scenario consists of a conversation between a male supervisor and his female employee about an important job to achieve. The supervisor wants to entrust this job to her but shows uncertainty concerning her capacity to manage a team to realize this job. The second sexism scenario is related to a discussion between a female supervisor and her female employee. The female employee is just returning from a maternity leave. Her supervisor reduced her employee's workload during her absence, whereas the employee did not want to change her workload. The third racism scenario is a job interview. The candidate is of Algerian origin. Ordinary racism behavior is defined based on real examples described in Diallo and Sassoon (2015). It includes several remarks from the interviewers on the origins of the candidate and associated stereotypes. Each discrimination scene lasts in average of 4 min.
2.2. The confrontation scenes
For each discrimination scene, the discriminator was then confronted by a third actor five times. The third actor was instructed to try to make her/his interlocutor aware of her/his discriminating behavior (played during the discrimination scenes). During these scenes, the actor playing the discriminator was instructed to simulate a specific social attitude: two scenes with an aggressive attitude, two scenes with a conciliatory attitude, and one scene with a denial attitude. Each scene lasted approximately 7 min and was performed by different actors. For these confrontation scenes, the actors have improvised with only the instruction on the social attitude to adopt for the actor playing the discriminator. We have collected different confrontations scenes for the same discrimination scene in order to have variability for these situations since our final objective is to be able to simulate an autonomous virtual discriminator with different social attitudes that interact in natural language with a user.
3. Experimental design
The collected corpus is an audio–video corpus recorded at the Technosport of Aix Marseille University in Luminy. The audio–video records consist of 18 face-to-face interactions lasting between 4 and 9 min each.
3.1. Image recording
The experimental design is described in Figure 1.
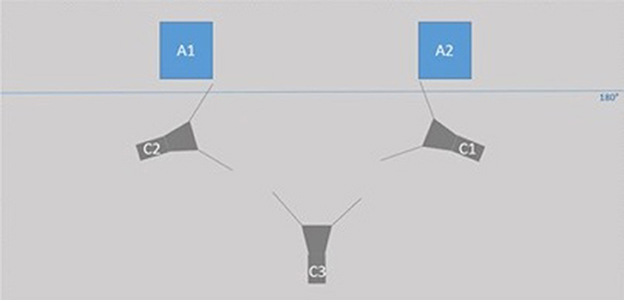
Figure 1. Experimental design. A1: actor 1 recorded with C1, A2: actor 2 recorded with C2; C1: camera 1 directed on A1 (close shot), C2: camera 2 directed on A2 (close shot), and C3: camera 3 (medium-long shot).
The data acquisition took place in a large room with high ceiling equipped for motion capture (Figure 2).
Our challenge was to the capture movements of the two actors and to make an audio–video record of their conversational face-to-face exchanges. The actors wore black suits equipped with motion sensors used by the Qualisys system. They sat on a chair without a backrest face to face.
3.2. Motion capture setup
The kinematic trajectories of 42 passive markers were monitored at 200 Hz by 16 Qualisys 700 infrared cameras (Qualisys, Gothenburg, Sweden) placed around the actors' dialogue area. The passive markers were placed on all actors according to the Animation Marker Set proposed by the Qualisys software (Figure 3). This marker placement allowed the use of the Skeleton Solver function of Qualisys and, in particular, to obtain the kinematic data of each body segment [position and orientation (pitch, yaw, and roll)]. A skeleton was calibrated and used for each of the different actors who took part in the experiment.
3.3. Video recordings
To record the conversational sequence, we used 2 cameras (C1 and C2), Canon XF105 (Figure 1). In front of each actor (A1 and A2), we installed the cameras while respecting 180 degree rule, which means that we installed them on the same side of the scene. By putting the two videos of each of the actors side by side, this setup allows keeping the coherence and understanding that the two actors discuss while being face to face, as illustrated in Figure 4. With the cameras 1 and 2, we filmed the actors in close shot format to focus on the actors' faces. Note that we choose the close shot since the motion capture system enables us to record all the body movements. The two cameras were synchronized. We also used a third camera (C3) to control the experimental design. The medium-long shot of the camera 3 is illustrated in Figure 3.
3.4. Audio recording
We recorded the two actors with headset microphones directional, Sennheiser HSP 4EW3, of flesh color, optimally positioned in order to not hide their face. The audio streams were synchronized with the two video streams. We used an audio interface, the RME Fireface UC, to control the audio signals and thus ensure good quality audio streams recorded with the two headset microphones. The audio setup is illustrated in Figure 5.
3.5. Synchronization
The synchronization of the experimental data (motion capture data, sound, and video) is very important. For this purpose, the synchronization of the two video streams was made due to a coaxial cable connecting the two cameras through their GenLock input. The synchronization of the two audio streams with two video streams was ensured by connecting microphones to XLR inputs of each camera. Thus, for each camera, we obtain the image (video stream) and the voice of the actor (audio stream) perfectly synchronized. To facilitate the control of the synchronization between all the streams, we used clap (Figure 6). The clap has the advantage of being identifiable on the audio signal of the video and viewable on the video.
3.6. Video editing
For each session, we delivered five files exported with Adobe Premiere Pro software. The five files exported are as follows: three video files (one from each camera), one containing two views synchronized (format H.264, image size of 1,920 x 1,080, 16/9 rate, 25 ips, with .MP4 extension), and two audio files (one per microphone: format Wavefom Audio, in 16 bits, 48 kHz, with .WAV extension). All these files are synchronized: They start at the same time and they have the same duration.
The experimental design with the camera focused on the actors' faces, the headset microphones with flesh color and the motion capture system have been set up in order to facilitate the semi-automatic annotation of the corpus. We present the annotation process in the next section.
4. Semi-automatic annotation of the corpus
4.1. The annotation process
In total, the collected corpus, in French, is composed of 38 videos composed of 19 interactions between two actors. Each video corresponds to one actor in the scene. The corpus is composed of two types of scenes: three discrimination scenes (six videos, one per actor) and 15 confrontation scenes (30 videos, one per actor). In total, the duration of the corpus is 120, 96 min (2h01 min).
Different tools have been used in order to annotate the corpus. First, the corpus has been automatically transcribed using the BAS web service.2 The transcription has been manually corrected and enriched with different information (laughter, elisions, and specific pronunciation) based on the TOE (Blache et al., 2017). We have used SPPAS (Bigi, 2015) to automatically segment the corpus in IPU (Inter-Pausal Unit).3 We have then manually filled the IPU with the transcription (Figure 7). Using SPPAS, IPUs, tokens, phonemes, and syllables are segmented and time-synchronized on the audio signal. It allowed extracting automatically the IPUs duration and the number of tokens per IPU. Laughs are also automatically aligned on the signal (Bigi and Meunier, 2018).
The tool Marsatag (Rauzy et al., 2014) has been used to extract the POS tags and, in particular, the following morpho-syntactic categories: adjective, adverb, auxiliary, conjunction, determiner, interjection, noun, preposition, pronoun, and verb.
Concerning the non-verbal signals, the smiles, the laughs, and the nods of the actors have been annotated automatically using the SMAD tool (Rauzy and Amoyal, 2020). Gaze direction is also of great importance in conversation. For now, the annotation of gaze has been manually annotated. Annotators have annotated when the gaze is toward the speaker or when the gaze is elsewhere (Figure 8).
Gaze direction, as well as the corrections of the annotations on laughs and nods, have been performed by three annotators. In order to validate the annotation of the gaze, 13.36% of the corpus has been annotated by one more annotator. The inter-annotator agreement, using Cohen's kappa, was very strong (k = 0.91).
In Table 1, we present the descriptive statistics concerning the verbal and non-verbal annotations.
We can notice the richness of the corpus both concerning the verbal and the non-verbal signals with a high number of IPUs and tokens, as well as pauses, nods, and changes in gaze direction. Note that all the automatic smile annotations have not been manually corrected and then are not reported in the table. The number of laughs remains quite low which is not surprising given the context of the interaction and the scenarios. We are currently analyzing the motion capture files in order to automatically extract annotations for the gestures based on the multi-level body notation system proposed in Fourati et al. (2019).
4.2. A first analysis of the actors' behaviors during the confrontation scenes
In the corpus, we are particularly interested in analyzing the confrontation scenes. Indeed, the discrimination scenes are directly replicated on the virtual actors (Section 5), whereas the confrontation scenes are exploited to create an interactive ECA able to interact with users with different attitudes.
The confrontation corpus is composed of 15 scenes for a total of 107.2 min (approximately 1 h: 48 min). Each scene lasts an average of 7.2 min (± 50 s). For each scene, the behavior of the two actors has been annotated.
4.2.1. The impact of the role on the behavior
In each scene, the discriminator faces another person who has the task of trying to make the discriminator aware of her/his discriminating behavior (played during the discrimination scenes) (Section 2.2). Consequently, we consider two roles in a confrontation scene:
• The role of a discriminator who has previously discriminated a person in a discrimination scene;
• And the persuader who was a witness of the discrimination scene and who has the instruction to try to make the discriminator aware of her/his discriminating behavior. The witness of the confrontation scene tries not only to raise awareness but also to persuade her/his interlocutor that this latter has behaved in a discriminatory manner. As such, the witness/persuader is the main speaker (initiation of the action and the goal of the dialogue).
We have varied the gender of the discriminators and of the persuaders as described in Table 2.
Descriptive statistics have been computed in order to compare the behavior of the actors according to their role in the interaction (Table 3).
As the main speaker, the persuader is expected to produce more speech. The Table 3 shows that, in fact, the quantity of speech of the persuader is more important. IPUs and tokens are more frequent. Also, pauses are more frequent but smaller than in the discriminator's speech which talks less (less pauses but longer). This can be explained by the fact that the discriminator speaks less (less pauses but longer).
Head nods are also very important signals in conversation. They depend on the participants' roles: when produced by listeners (as feedback) they show attention or agreement to the discourse (Boudin et al., 2021) whereas when produced by main speakers, they can serve to check agreement or express emphasis (Heylen, 2005). In the corpus, the nods of persuaders are more frequent than those of the discriminators, that could be explained by her/his role to try to convince the interlocutor. As far as we know, no research has correlated the frequency and duration of nods to persuaders' behavior. A deeper analysis on other data should be performed to confirm this hypothesis.
Similarly to nods, eye gaze is important in conversation, and more particularly as a reliable cue for monitoring and regulating turn-taking. Eye-gaze can indeed change according to the different roles of the participants (Rossano, 2013). In our corpus, the gaze behavior of the discriminator and the persuader seems quite similar.
4.2.2. The impact of attitude on the behavior
For each confrontation scene, the discriminator was instructed to simulate a specific social attitude: a denial attitude, a conciliatory attitude, or an aggressive attitude. In the confrontation corpus, we have six scenes with a discriminator having the instruction to adopt an aggressive behavior, six scenes with a discriminator adopting a conciliatory behavior, and three scenes with a discriminator acting with a denial behavior. We report Table 4, the descriptive statistics to compare the behaviors of the two actors (the discriminator and the persuader) according to the attitudes acted by the discriminator.
Several research works have demonstrated the influence of social attitudes on behavior (e.g., Mehrabian, 1969; Carney et al., 2005; Argyle, 2013). In line with this research, in our corpus, the descriptive statistics show an effect of the social attitudes on the behavior. For instance, in a conciliatory situation, the actors spoke more (more frequent IPUs) than in an aggressive or denial situation. The pauses are also more frequent and longer in the conciliatory and the denial situations, reflecting a more calm conversation. Less tokens and more pauses could be interpreted by a slower speech rate, which is coherent with a more calm conversation.
The quantity of nods, more important in the aggressive situation than in the conciliatory or the denial situation, may be explained by the high level of arousal in the aggressive situation leading to a higher activity in terms of head movements. In the same way, we can observe more frequent changes in gaze direction (higher quantity of gazes toward the speaker and elsewhere) in the aggressive situation compared to the other situations.
Further analyses should be performed to explore the actors' behaviors. In particular, we plan to explore the impact of the attitudes and roles on gestures, prosody, alignment of the signals between the two actors, overlaps, and feedback.
5. Toward a virtual reality forum theater
In the virtual reality forum theater for discrimination awareness, we aim at developing, in a first step, the user sees a discrimination scene simulated by two virtual actors through a VR headset. For this purpose, we use the collected discrimination scenes played by the real actors (Section 2.1). The scenes of discrimination have been used to simulate the exact same scenes but played by virtual actors (Figure 9).
From the raw animations, corrections are made via Qualisys' QTM software to interpolate missing marker positions or to remove ghost markers. The animations are exported in FBX format to the Maya software (Autodesk) in order to make their positions and orientations identical. The animations are then exported to Reallusion's suite of 3DXChange, Character Creator, and Iclone software. 3DXChange allows the import of models and animations in FBX format into its proprietary formats. Character Creator creates avatars from libraries of humans, clothes, and accessories. Iclone allows editing the created avatars and animations. Iclone plugins allow interfacing with facial capture software such as Faceware. The latter relies on the video stream of a simple webcam to animate a facial model of an avatar. Finally, the animated avatars are exported in FBX format to graphics engines such as Unity3D or Unreal.
Through this procedure, we are creating the simulation of discrimination scenes with virtual actors similar to the discrimination scenes collected with the real actors. The motion capture system, as well as the recording of the actors' faces, has enabled us to simulate exactly the same non-verbal behaviors of the real actors on the virtual ones.
In the second step, the user is instructed to converse with the virtual actor displaying discriminating behavior to make the virtual actor aware of its behavior. Different interactive situations are simulated, involving different types of social discrimination (ordinary sexism and ordinary racism) in different social contexts (e.g., hierarchical relations and opposite genders) and through various socio-emotional behaviors of the virtual actors (conciliatory, aggressive, or denial attitude). For this second step, we use the collected confrontation scenes played by real actors.
Creating a virtual agent that can interact in natural language with a user and simulate different social attitudes is a complex task. The complexity of the task is reduced by the specific use case and the limited three social attitudes considered. The autonomous virtual agent will play the role of the author of the discriminatory behavior, and the user will have the task of making the virtual actor aware of their behavior. The behavioral model of the virtual actor with different social attitudes will be based on the collected corpus of confrontation scenes. An in-depth study of the corpus combining conversational analysis and data mining methods will be performed to construct the computational behavioral model. In particular, we aim to explore methods for the automatic generation of behavior from corpus as proposed in Cherni et al. (2022) and Delbosc et al. (2022).
6. Conclusion
In this article, we have presented a collected annotated corpus of data containing scenes of social discrimination performed by professional actors specialized in training through simulation and with strong experience in the field of preventing discrimination. Different situations of ordinary racism and sexism have been simulated with different social attitudes within an interactional context. A specific experimental design with motion capture systems and audio–video recording has been set up to allow a semi-automatic annotation and a fine-grained analysis of the multimodal socio-emotional cues related to this type of social interactions. The corpus TRUENESS is disseminated through the Ortolang platform.4
Specifically designed for the development of a virtual reality forum theater, our next step aims at analyzing the verbal and non-verbal behaviors of actors in confrontations scenes to create a multimodal computational model of social attitudes using an approach combining data-mining techniques and conversational analysis.
Data availability statement
The raw data supporting the conclusions of this article will be made available by the authors, without undue reservation.
Ethics statement
Written informed consent was obtained from the individual(s) for the publication of any potentially identifiable images or data included in this article.
Author contributions
All authors listed have made a substantial, direct, and intellectual contribution to the work and approved it for publication.
Acknowledgments
The collection of the presented corpus is funded by the CNRS MITI, the Cognition Institute and the ANR (Project COPAINS—ANR-18-CE33-0012).
Conflict of interest
The authors declare that the research was conducted in the absence of any commercial or financial relationships that could be construed as a potential conflict of interest.
Publisher's note
All claims expressed in this article are solely those of the authors and do not necessarily represent those of their affiliated organizations, or those of the publisher, the editors and the reviewers. Any product that may be evaluated in this article, or claim that may be made by its manufacturer, is not guaranteed or endorsed by the publisher.
Footnotes
1. ^Glassdoor, Diversité et Inclusion 2019, Rapport d'enquête.
2. ^https://clarin.phonetik.uni-muenchen.de/BASWebServices/interface
3. ^Block of speech bounded by silent pauses and often assimilated to turns.
References
Anderson, K., André, E., Baur, T., Bernardini, S., Chollet, M., Chryssafidou, E., et al. (2013). “The tardis framework: intelligent virtual agents for social coaching in job interviews,” in International Conference on Advances in Computer Entertainment Technology (Boekelo: Springer), 476–491.
Assencio, C. (2016). Bilan du thtre forum interactif sur la sensibilisation auxstrotypes de genre. Technical report, Communiqu de presse ISAT.
Bigi, B. (2015). Sppas-multi-lingual approaches to the automatic annotation of speech. the Phonetician. J. Int. Soc. Phonetic Sci. 111, 54–69.
Bigi, B., and Meunier, C. (2018). Automatic segmentation of spontaneous speech. Revista de Estudos da Linguagem 26, 1530. doi: 10.17851/2237-2083.26.4.1489-1530
Blache, P., Bertrand, R., Ferré, G., Pallaud, B., Prévot, L., and Rauzy, S. (2017). “The corpus of interactional data: a large multimodal annotated resource,” in Handbook of Linguistic Annotation (Springer), 1323–1356.
Boudin, A., Bertrand, R., Rauzy, S., Ochs, M., and Blache, P. (2021). “A multimodal model for predicting conversational feedbacks,” in International Conference on Text, Speech, and Dialogue (TSD) (Olomouc).
Bruijnes, M., Linssen, J., and Heylen, D. (2019). Special issue editorial: virtual agents for social skills training. J. Multimodal User Interfaces 13, 1–2. doi: 10.1007/s12193-018-00291-7
Carney, D. R., Hall, J. A., and LeBeau, L. S. (2005). Beliefs about the nonverbal expression of social power. J. Nonverbal Behav. 29, 105–123. doi: 10.1007/s10919-005-2743-z
Cherni, A., Bertrand, R., and Ochs, M. (2022). “From neutral human face to persuasive virtual face, a new automatic tool to generate a persuasive attitude,” in Advances in Signal Processing and Artificial Intelligence (ASPAI 2022) (Corfu).
Chollet, M., Ghate, P., Neubauer, C., and Scherer, S. (2018). “Influence of individual differences when training public speaking with virtual audiences,” in Proceedings of the 18th International Conference on Intelligent Virtual Agents, Sydney, 1–7.
Dardenne, B., Dumont, M., and Bollier, T. (2007). Insidious dangers of benevolent sexism: consequences for women's performance. J. Pers. Soc. Psychol. 93, 764. doi: 10.1037/0022-3514.93.5.764
Delbosc, A., Ochs, M., and Ayache, S. (2022). “Automatic facial expressions, gaze direction and head movements generation of a virtual agent,” in Companion Publication of the 2022 International Conference on Multimodal Interaction (Bangalore), 79–88.
Fourati, N., Pelachaud, C., and Darmon, P. (2019). “Contribution of temporal and multi-level body cues to emotion classification,” in 2019 8th International Conference on Affective Computing and Intelligent Interaction (ACII) (Cambridge, UK: IEEE), 116–122.
Grésy, B. (2015). Le sexisme dans le monde du travail, entre dni et ralit. Technical report, Rapport du Conseil suprieur de lgalit professionnelle entre les femmes et les hommes solidaire.
Gret (2012). Le thtre-forum: une rponse aux obstacles lis au genre auburkina faso. Technical report, GRET  Professionnels du dveloppement solidaire.
Hall, L., Jones, S. J., Aylett, R., Andre, E., Paiva, A., Hofstede, G. J., et al. (2011). “Fostering empathic behaviour in children and young people: interaction with intelligent characters embodying culturally specific behaviour in virtual world simulations,” in INTED2011 Proceedings, Valencia, 2804–2814.
Heylen, D. (2005). “Challenges ahead: Head movements and other social acts in conversations,” in Virtual Social Agents (Hatfield), 45–52.
Mehrabian, A. (1969). Significance of posture and position in the communication of attitude and status relationships. Psychol. Bull. 71, 359. doi: 10.1037/h0027349
Ochs, M., Mestre, D., De Montcheuil, G., Pergandi, J.-M., Saubesty, J., Lombardo, E., et al. (2019). Training doctors  social skills to break bad news: evaluation of the impact of virtual environment displays on the sense of presence. J. Multimodal User Interfaces 13, 41–51. doi: 10.1007/s12193-018-0289-8
Rauzy, S., and Amoyal, M. (2020). “Smad: a tool for automatically annotating the smile intensity along a video record,” in HRC2020, 10th Humour Research Conference, Texas.
Rauzy, S., Montcheuil, G., and Blache, P. (2014). “Marsatag, a tagger for french written texts and speech transcriptions,” in Second Asian Pacific Corpus linguistics Conference, Takamatsu City, 220–220.
Yves, G. (1999). Le théâtre forum, pour une pédagogie de la citoyenneté. Paris: L'Harmattan. Available online at: http://digital.casalini.it/9782296378032
Keywords: multimodal corpus, discrimination, virtual agents, motion capture, social attitude
Citation: Ochs M, Pergandi J-M, Ghio A, André C, Sainton P, Ayad E, Boudin A and Bertrand R (2023) A forum theater corpus for discrimination awareness. Front. Comput. Sci. 5:1081586. doi: 10.3389/fcomp.2023.1081586
Received: 27 October 2022; Accepted: 03 January 2023;
Published: 16 February 2023.
Edited by:
Beatrice Biancardi, LINEACT CESI, FranceReviewed by:
Eleonora Ceccaldi, University of Genoa, ItalyAmine Benamara, Universitï Paris-Saclay, France
Copyright © 2023 Ochs, Pergandi, Ghio, André, Sainton, Ayad, Boudin and Bertrand. This is an open-access article distributed under the terms of the Creative Commons Attribution License (CC BY). The use, distribution or reproduction in other forums is permitted, provided the original author(s) and the copyright owner(s) are credited and that the original publication in this journal is cited, in accordance with accepted academic practice. No use, distribution or reproduction is permitted which does not comply with these terms.
*Correspondence: Magalie Ochs, magalie.ochs@lis-lab.fr