- School of Electronic Engineering and Computer Science, Queen Mary University of London, London, United Kingdom
Experimentation using extended reality (XR) technology is predominantly conducted in-lab with a co-present researcher. Remote XR experiments, without co-present researchers, have been less common, despite the success of remote approaches for non-XR investigations. In order to understand why remote XR experiments are atypical, this article outlines the perceived limitations, as well as potential benefits, of conducting remote XR experiments, through a thematic analysis of responses to a 30-item survey of 46 XR researchers. These are synthesized into five core research questions for the XR community, and concern types of participant, recruitment processes, potential impacts of remote setup and settings, the data-capture affordances of XR hardware and how remote XR experiment development can be optimized to reduce demands on the researcher. It then explores these questions by running two experiments in a fully “encapsulated” remote XR case study, in which the recruitment and experiment processes is distributed and conducted unsupervised. It discusses the design, experiment, and results from this case study in the context of these core questions.
1. Introduction
Extended reality (XR) technology—such as virtual, augmented, and mixed reality—is increasingly being examined and utilized by researchers in the HCI and other research communities due to its potential for creative, social and psychological experiments (Blascovich et al., 2002). Many of these studies take place in laboratories with the co-presence of the researcher and the participant (Kourtesis et al., 2020). The XR research community has been slow to embrace recruiting remote participants to take part in studies running outside of laboratories—a technique which has proven useful for non-XR HCI, social and psychological research (Paolacci et al., 2010; Preece, 2016). However, the current COVID-19 pandemic has highlighted the importance and perhaps necessity of understanding and deploying remote recruitment methods within XR research.
There is also limited literature about remote XR research, although what reports exist suggest that the approach shows promise: data-collection is viable (Steed et al., 2016), results are similar to those found in-lab (Mottelson and Hornbæk, 2017) even when the participants are unsupervised (Huber and Gajos, 2020), and recruiting is possible (Ma et al., 2018). Researchers have also suggested using the existing, engaged communities that have emerged around these technologies, such as social VR experiences, as combined platforms for recruitment and experimentation (Saffo et al., 2020). With the increasingly availability of consumer XR devices (estimates show five million high-end XR HMDs sold in 2020, raising to 43.5 million by 2025; Alsop, 2022), and health and safety concerns around in-lab experimentation, particularly for research involving head-mounted displays (HMDs), it is an important time to understand and investigate the conceptions around remote research from researchers who use XR technologies.
This paper outlines the methodology and results from a survey of XR researchers regarding remote XR research. The results were derived from 46 respondents answering 30 questions concerning existing research practice and beliefs around remote XR research. It then synthesizes these findings into five core questions for the XR research community: (1) who are the potential remote XR participants, and are they representative; (2) how can we access a large pool of remote XR participants; (3) to what extent do remote XR studies affect results compared with in-lab; (4) what are the built-in XR data collection affordances of XR hardware, and what can they help us study; (5) how can we lower the barriers to creating less researcher-dependent remote experiment software, to maximize the potential of remote XR research.
Additionally, it explores these questions through a case study of two experiment, in which the recruitment and experiment process is conducted remotely and unsupervised. The design of the experiments and results related to participants, participant recruitment and the experiment process are then examined in the context of the above core questions.
The paper offers five core contributions: (1) we summarize existing research on conducting remote XR experiments; (2) we provide an overview of the status quo, showing that many of the concerns regarding remote XR are those also applicable to other remote studies; and that the unique aspects of remote XR research could offer more benefits than drawbacks; (3) we set out recommendations for advancing remote XR research, and outline important questions that should be answered to create an evidence-backed experimentation process; (4) we present a detailed design process for creating an encapsulated study; and (5) we analyse the experience and results of the encapsulated approach in relation to the defined core questions.
2. Literature
We present a literature review of relevant publications on XR research, remote research and remote XR research. We use “XR” as the umbrella term for virtual reality (VR), augmented reality (AR) and mixed reality (MR) (Ludlow, 2015). This space is also sometimes referred to as spatial or immersive computing.
The chapter is organized in three parts. First, we explore conventional XR experiments under “normal” conditions (e.g., in laboratory andor directly supervised by the researcher). We then summarize existing literature on remote experiments in XR research. Finally, we report the main findings in previous publications on remote data collection and experimentation.
2.1. Conventional XR experiments
2.1.1. Experiment types and fields of interest
According to Suh and Prophet (2018)'s systematic literature review, XR experiments involving human participants can broadly be categorized into two groups: (1) studies about XR, and (2) studies about using XR. The first group focuses on the effects of XR system features on the user experience (e.g., monitoring presence outcomes based on the amount of physical interaction), whereas the second category examines how the use of an XR technology modifies a measurable user attribute (e.g., if leveraging physical interaction in XR affects learning outcomes).
Across these categories there have been a variety of explorations on different subjects and from different academic fields. These include social psychological (Blascovich et al., 2002), including social facilitation-inhibition (Hoyt et al., 2003), conformity and social comparison (Blascovich, 2002), social identity (Kilteni et al., 2013); neuroscience and neuropsychology (Kourtesis et al., 2020), visual perception (Wilson and Soranzo, 2015), multisensory integration (Choi et al., 2016), proxemics (Sanz et al., 2015), spatial cognition (Waller et al., 2007), education and training (Radianti et al., 2020), therapeutic applications (Freeman et al., 2018), pain remediation (Gromala et al., 2015), motor control (Connelly et al., 2010), terror management (Josman et al., 2006), and media effects such as presence (Bailey et al., 2012).
The theoretical approaches behind these studies are also disparate, including theories such as conceptual blending, cognitive load, constructive learning, experiential learning, flow, media richness, motivation, presence, situated cognition, the stimuli-organism-response framework, and the technology acceptance model (Suh and Prophet, 2018).
2.1.2. Data collection, approaches, and techniques
According to Suh and Prophet (2018)'s meta-analysis, the majority of XR research explorations have been experiments (69%). Other types of explorations include surveys (24%), interviews (15%), and case studies (9%). These approaches have been used both alone and in combination with each other. Data collection methods are predominantly quantitative (78%), although qualitative and mixed approaches are also used. Another systematic review of XR research (focused on higher education) (Radianti et al., 2020) adds focus group discussion and observation as research methods, and presents two potential subcategories for experiments: mobile sensing and “interaction log in VR app”, in which the XR application logs the user's activities and the researcher uses the resulting log for analysis.
The types of data logging found in XR experiments are much the same as those listed in Weibel's exploration of physiological measures in non-immersive virtual reality (Weibel et al., 2018), with studies using skin conductance (Yuan and Steed, 2010), heart rate (Egan et al., 2016), blood pressure (Hoffman et al., 2003), as well as electroencephalogram (EEG) (Amores et al., 2018). Built-in inertial sensors that are integral to providing an XR experience, such as head and hand position for VR HMDs, have also been widely used for investigations, including posture assessment (Brookes et al., 2020), head interaction tracking (Zhang and Healey, 2018), gaze and loci of attention (Piumsomboon et al., 2017) and gesture recognition (Kehl and Van Gool, 2004), while velocity change (Warriar et al., 2019) has also been used in both VR and AR interventions.
2.1.3. Benefits of XR experiments
There are many suggested benefits to using XR technology as a research tool: it allows researchers to control the mundane-realism trade-off (Aronson, 1969) and thus increase the extent to which an experiment is similar to situations encountered in everyday life without sacrificing experimental control (Blascovich et al., 2002); to create powerful sensory illusions within a controlled environment (particularly in VR), such as illusions of self-motion and influence the proprioceptive sense (Soave et al., 2020); improve replication (Blascovich et al., 2002) by making it easier to recreate entire experimental environments; and allow representative samples (Blascovich et al., 2002) to experience otherwise inaccessible environments, when paired with useful distribution and recruitment networks.
2.1.4. Challenges of XR experiments
Pan and Hamilton (2018) explored some of the challenges facing experiments in virtual worlds, which continue to be relevant in immersive XR explorations. These include the challenge of ensuring the experimental design is relevant for each technology and subject area; ensuring a consistent feeling of self-embodiment to ensure engaged performance (Kilteni et al., 2012); avoid uncanny valley, in which characters which look nearly-but-not-quite human are judged as uncanny and are aversive for participants (Mori et al., 2012); simulation sickness and nausea during VR experiences (Moss and Muth, 2011); cognitive load (Sweller, 2010) which may harm participation results through over-stimulation, particularly in VR (Steed et al., 2016; Makransky et al., 2019); novelty effects of new technology interfering with results (Clark and Craig, 1992; Ely and Minor, 1994); and ethics, especially where experiences in VR could lead to changes in participants' behavior and attitude in their real life (Banakou et al., 2016) and create false memories (Segovia and Bailenson, 2009).
2.2. Remote XR experiments
There has been little research into remote XR experimentation, particularly for VR and AR HMDs. By remote, we mean any experiment that takes place outside of a researcher-controlled setting. This is distinct from field or in-the-wild research, which is research “that seeks to understand new technology interventions in everyday living” (Rogers and Marshall, 2017), and so is dependent on user context. These definitions are somewhat challenged in the context of remote VR research, as for VR, remote and field/in-the-wild are often the same setting, as the location where VR is most used outside the lab is also where it is typically experienced (e.g., home users, playing at home; Ma et al., 2018). For AR, there is a greater distinction between remote, which refer to any AR outside of the controlled setting of the lab; and field/in-the-wild, which require a contextual deployment.
In terms of remote XR research outcomes, Mottelson and Hornbæk (2017) directly compared in-lab and remote VR experiment results. They found that while the differences in performance between the in-lab and remote study were substantial, there were no significant differences between effects of experimental conditions. Similarly, Huber and Gajos (2020) explored uncompensated and unsupervised remote VR samples and were able to replicate key results from the original in-lab studies, although with smaller effect sizes. Finally, Steed et al. (2016) showed that collecting data in the wild is feasible for virtual reality systems.
Ma et al. (2018) is perhaps the first published research on recruiting remote participants for VR research. The study, published in 2018, used the Amazon Mechanical Turk (AMT) crowdsourcing platform, and received 439 submissions over a 13-day period, of which 242 were eligible. The participant demographics did not differ significantly from previously reported demographics of AMT populations in terms of age, gender, and household income. The notable difference was that the VR research had a higher percentage of U.S.-based workers compared to others. The study also provides insight into how remote XR studies take place: 98% of participants took part at home, in living rooms (24%), bedrooms (18%), and home offices (18%). Participants were typically alone (84%) or in the presence of one (14%) or two other people (2%). Participants reported having “enough space to walk around” (81%) or “run around (10%)”. Only 6% reported that their physical space would limit their movement.
While Ma et al.'s work is promising in terms of reaching a representative sample and the environment in which participants take part in experiments, it suggests a difficulty in recruiting participants with high-end VR systems, which allow six-degrees of freedom (the ability to track user movement in real space) and leverage embodied controllers (e.g., Oculus Rift, HTC Vice). Only 18 (7%) of eligible responses had a high-end VR system. A similar paucity of high-end VR equipment was found by Mottelson and Hornbæk, in which 1.4% of crowdworkers had access to these devices (compared to 4.5% for low-end devices, and 83.4% for Android smartphones). This problem is compounded if we consider Steed et al.'s finding that only 15% of participants provide completed sets of data.
An alternative approach to recruiting participants is to create experiments inside existing communities of XR users, such as inside the widely-used VR Chat software (Saffo et al., 2020). This allows researchers to enter into existing communities of active users, rather than attempt to establish their own. However, there are significant limitations for building experiments on platforms not designed for experimentation, such as programming limitations, the ability to communicate with outside services for data storage, and the absence of bespoke hardware interfaces.
2.3. Remote data collection and experimentation
2.3.1. Validity, benefits, drawbacks, and differences
Using networks for remote data collection from human participants has been proven valid in some case studies (Krantz and Dalal, 2000; Gosling et al., 2004). In Gosling et al.'s comprehensive and well-cited study, internet-submitted samples were found to be diverse, generalize across presentation formats, were not adversely affected by non-serious or repeat respondents, and present results consistent with findings from in-lab methods. There is similar evidence for usability experiments, in which both the lab and remote tests captured similar information about the usability of websites (Tullis et al., 2002).
That said, differences in results for lab and remote experiments are common (Stern and Faber, 1997; Senior et al., 1999; Buchanan, 2000). The above website usability study also found that in-lab and remote experiments offered their own advantages and disadvantages in terms of the usability issues uncovered (Tullis et al., 2002). The factors that influence differences between in-lab and remote research are still being understood, but even beyond experiment design, there is evidence that even aspects such as the participant-perceived geographical distance between the participant and the data collection system influences outcomes (Moon, 1998).
Reips (2000)'s well-cited study outlined 18 advantages of remote experiments, including (l) easy access to a demographically and culturally diverse participant population, including participants from unique and previously inaccessible target populations; (2) bringing the experiment to the participant instead of the opposite; (3) high statistical power by enabling access to large samples; (4) the direct assessment of motivational confounding; and (5) cost savings of lab space, person-hours, equipment, and administration. He found seven disadvantages: (l) potential for multiple submissions, (2) lack of experimental control, (3) participant self-selection, (4) dropout, (5) technical variances, (6) limited interaction with participants and (7) technical limitations.
2.3.2. Supervised vs. unsupervised
With the increasing availability of teleconferencing, it has become possible for researchers to be co-“tele” present and supervise remote experiments through scheduling webcam experiment sessions. This presents a distinction from the unsupervised internet studies discussed above, and brings its own opportunities and limitations.
Literature broadly suggests that unsupervised experiments provide suitable quality data collection (Ryan et al., 2013; Hertzum et al., 2015; Kettunen and Oksanen, 2018). A direct comparison between a supervised in-lab experiment and a large, unsupervised web-based experiment found that the benefits outweighed its potential costs (Ryan et al., 2013); while another found that a higher percentage of high-relevance responses came from unsupervised participants than supervised ones in a qualitative feedback setting (Hertzum et al., 2015). There is also evidence that unsupervised participants react faster to tasks over the internet than those observed in the laboratory (Kettunen and Oksanen, 2018).
For longitudinal studies, research in healthcare has found no significant difference between task adherence rates between unsupervised and supervised groups (Creasy et al., 2017). However, one study noted that supervised studies had more effective outcomes (Lacroix et al., 2015).
2.3.3. Crowdworkers: Viable?
Remote data collection was theorized to bring easy access to participants, including diverse participants and large samples (Reips, 2000). Researchers have found that recruiting crowdworkers, people who work on tasks distributed to them over the internet, allowed them access to a large participant pool (Paolacci et al., 2010), with enough diversity to facilitate cross-cultural and international research (Buhrmester et al., 2016). Research has found that crowdworkers were significantly more diverse than typical American college samples and more diverse than other internet recruitment methods (Buhrmester et al., 2016), at an affordable rate (Paolacci et al., 2010; Buhrmester et al., 2016). This has allowed researchers a faster theory-to-experiment cycle (Mason and Suri, 2012).
Results from crowdworker-informed studies have been shown to reproduce existing results from historical in-lab studies (Paolacci et al., 2010; Sprouse, 2011; Buhrmester et al., 2016), while a direct comparison between experiment groups of crowdworkers, social media-recruited participants and on-campus recruitment, found almost indistinguishable results (Casler et al., 2013).
Some distinctions between crowdworkers and in-lab have been discovered, however. Comparative experiments between crowdworkers and in-person studies have suggested slightly higher participant rejection rates (Sprouse, 2011), while participants have been shown to report shorter narratives than other groups of college students (both online and in-person) and use proportionally more negative emotion terms than college students reporting verbally to an experimenter (Grysman, 2015).
Distinctions also exist within crowdworker recruitment sources. A study of AMT, CrowdFlower (CF) and Prolific Academic (ProA) found differences in response rate, attention-check question results, data quality, honesty, diversity and how successfully effects were reproduced (Peer et al., 2017).
Data quality is a common concern regarding crowdworkers (Goodman et al., 2013). However, attention-check questions used to screen out inattentive respondents or to increase the attention of respondents have been shown to be effective in increasing the quality of data collected (Aust et al., 2013), as have participant reputation scores (Peer et al., 2014).
A growing concern regarding crowdworkers is non-naivete, in which participants having some previous knowledge of the study or similar studies that might bias them in the experiment. Many workers report having taken part in common research paradigms (Paolacci and Chandler, 2014), and there are concerns that if researchers continue to depend on this resource, the problem may expand. As such, further efforts are needed by researchers to identify and prevent non-naive participants from participating in their studies (Buhrmester et al., 2018).
2.4. Summary
It is clear that remote methods have been usefully deployed for non-XR research, and seemingly bring benefits such as easier participant recruitment, reduced recruitment cost and broadened diversity, without introducing major biases. However, there is still a paucity of research regarding the extent to which remote XR research can and has been used to leverage the unique benefits of both XR (environmental control, sensory illusions, data collection, replication) and remote (participation, practicality, cost-savings) methods, as well as the potential impact of their combined limitations. Therefore a survey of XR researcher experiences and beliefs regarding remote XR research could help us understand how these apply practically at the current time, and understand the key areas for future developments in this field.
3. Methodology
3.1. Survey
We surveyed current practice to outline the researcher-perceived benefits and drawbacks of lab-based and remote XR research. We used a 30-item qualitative questionnaire that enquired about participants' existing lab-based and remote research practices; thoughts on future lab-based and remote research; and potential benefits and drawbacks for each area. The survey was circulated through relevant mailing lists (visionlist@visionscience.com, BCS-HCI@jiscmail.ac.uk, chi-announcements@listserv.acm.org), to members of groups thinking of or currently running remote studies, and to members of universities' virtual and augmented reality groups found via search engines.
Responses were thematically analyzed using an inductive approach based upon Braun and Clarke's six phases of analysis (Braun and Clarke, 2006). The coding and theme generation process was conducted twice by independent researchers; themes were then reviewed collaboratively to create the final categorizations.
3.2. Participants
We received 46 responses to our survey from 36 different (predominantly academic) institutions. Most responses came from researchers based in Europe and North America, but responses also came from Asia. The majority of participants were either PhD students (18) or lecturers, readers or professors (11) at universities. Other roles were academic/scientific researcher (5), masters student (5), corporate researcher (4) and undergraduate student (2). A diverse set of ages responded to the survey: 18–24 (5), 25–34 (22), 35–44 (11), 45+ (6), and gender skewed male (29) over female (16), or other (1).
4. Participant XR setup results
Participants were more likely to have previously ran in-lab studies (37) than remote studies (14). Twenty-seven participants noted that, because of the COVID-19 pandemic, they have considered conducting remote XR experiments. In the next 6 months, more researchers were planning to run remote studies (24) than lab-based (22).
Participants predominantly categorized their research as VR-only (28) over AR-only (5). Ten participants considered their research as both VR and AR (and three did not provide an answer). This result is illustrated in Figure 1. In terms of research hardware, the majority of VR research leveraged virtual reality HMD-based systems with six degrees of freedom (32), that tracks participants' movements inside the room, over three degrees of freedom (15) or CAVE systems (1). Nineteen researchers made use of embodied or gesture controllers, where the position of handheld controllers are tracked in the real world and their position virtualized. For AR, HMDs were the predominant medium (13) over smartphones (9), with some researchers (5) using both.
An array of supplementary technologies and sensors were also reported by 13 respondents, including gaming joysticks, haptic actuators, a custom haptic glove, motion capture systems, e-textiles, eye-trackers, microphones, computer screens, Vive body trackers, brain-computer interfaces, EEG and electrocardiogram (ECG) devices, galvanic skin response sensors, and hand-tracking cameras, as well as other spatial audio and hardware rigs.
The use of a variety of different off-the-shelf systems was also reported: Vive, Vive Pro, Vive Eye, Vive Index, Vive Pucks, Quest, Go, Rift, Rift S, DK2, Cardboard, Magic Leap One, Valve Knuckles, Hololens. Predominantly used devices are part of HTC Vive (25) and Oculus (23) family.
Respondents outlined numerous features of immersive hardware that they used in their research, visible in Figure 2. The most prominent were embodiment aspects, including embodiment interactivity, in which a user's hand or body movements are reflected by a digital avatar (37) and embodiment movement (35), where participants can move in real space and that is recognized by the environment. Abstract movement (13), where a user controls an avatar via an abstracted interface (like a joystick) and abstract interactivity (8) were less popular. Spoken input was also used (10), as well as 3D sound (13) and non-3D sound (6). Scent was also noted (1) along with other unique features.
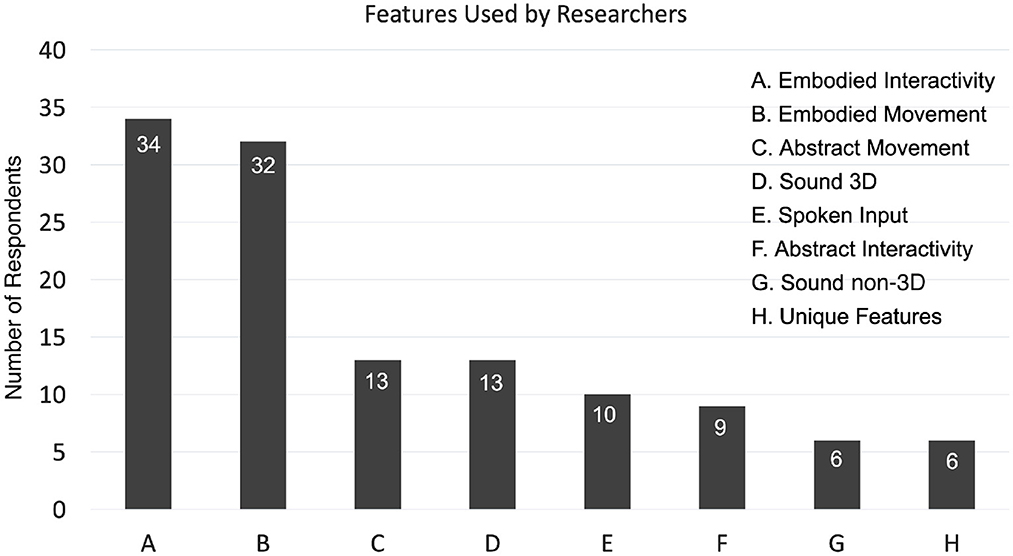
Figure 2. Features used by respondents in their user studies. (A) Embodied Interactivity: using embodied controller/camera-based movement. (B) Embodied movement: Using your body to move/“roomscale”. (C) Abstract movement: Using a gamepad or keyboard and mouse to move. (D) Sound 3D: Binaural acoustics. (E) Spoken input. (F) Abstract interactivity: using a gamepad or keyboard and mouse to interact. (G) Sound non-3D: Mono/stereo audio. (H) Unique features: e.g., haptics, hand tracking, scent.
5. Thematic analysis results
In this section, we present and discuss the themes found in our survey study. The key points of each theme are summarized in a table at the start each subsection. Some of these points were found across multiple themes as they touch various aspects of user-based XR research.
5.1. Theme: Study sub-types
Our analysis suggests that in-lab and remote studies can be additionally distinguished by whether the setting type is vital or preferred (summarized in Table 1). Broadly, in-lab (vital) studies require experimental aspects only feasible in-lab, such as bespoke hardware or unique data collection processes; in-lab (preferred) studies could take place outside of labs, but prefer the lab-setting based upon heightened concerns regarding the integrity of data collected and place a high value on a controlled setting. Remote (vital) studies are required when a user's natural environment is prioritized, such as explorations into behavior in Social VR software; and remote (preferred) studies are used when cross-cultural feedback or a large number of participants are needed, or if the benefits offered by an in-lab setting are not required.
Beyond these, another sub-type emerged as an important consideration for user studies: supervised or unsupervised. While less of an important distinction for in-lab studies (which are almost entirely supervised), participant responses considered both unsupervised “encapsulated” studies, in which explanations, data collection and the study itself exist within the software or download process, and supervised studies, in which researchers schedule time with the remote participant to organize, run, and/or monitor the study. These distinctions will be discussed in more detail throughout the analysis below, as the sub-types have a distinct impact on many of the feasibility issues relating to remote studies.
5.2. Theme: Study participants
5.2.1. Recruitment scope
Twenty-nine respondents stated the well-known challenge of recruiting a satisfactory number of participants for lab-based studies. Issues were reported both with the scale of available participants, and the problem of convenience sampling and WEIRD—Western, educated, industrialized, rich, and democratic societies—participants (Henrich et al., 2010).
Participant recruitment was mentioned by 27 respondents as the area in which remote user studies could prove advantageous over labs. Remote studies could potentially provide easier recruitment (in terms of user friction: accessing to lab, arriving at the correct time), as well as removing geographic restrictions to the participant pool.
Removing the geographic restrictions also simplifies researchers' access to cross-cultural investigations (R23, R43). While cross-cul-tural lab-based research would require well-developed local recruitment networks, or partnerships with labs in target locations, remote user studies, and more specifically, systems built deliberately for remote studies, introduce cross-cultural scope at no additional overhead.
There are, however, common concerns over the limitations to these benefits due to the relatively small market size of XR technologies. For AR, this is not a strong limitation for smartphones-based explorations, but the penetration of HMD AR and VR technology is currently limited, and it is possible that those who currently have access to these technologies will not be representative of the wider populations. Questions remain over who the AR/VR HMD owners are, if they exhibit notable differences from the general population, and if those differences are more impactful than those presented by existing convenience sampling.
Despite the belief that designing for remote participants will increase participant numbers, and therefore the power of studies, it seems unclear how researchers will reach HMD-owning audiences. Thirty respondents who have, or plan to, run remote XR studies have concerns about the infrastructure for recruiting participants remotely. Unlike other remote studies, the requirement for participants to own or have access to XR hardware greatly reduces the pool (around 5 million XR HMDs were sold in 2020; Alsop, 2022). A major outstanding question is how researchers can access these potential participants, although some platforms for recruiting XR participants have emerged in the past few months such as XRDRN.org.
Nine respondents noted that remote XR experiments may encourage participation from previously under-represented groups, including introverts and those who cannot or do not wish to travel into labs to take part (e.g., people who struggle to leave their homes due to physical or mental health issues).
However, respondents with research-specific requirements also raised concerns that recruitment of specific subsets of participants could be more difficult remotely. For example, when recruiting for a medical study of those with age-related mobility issues, it is unlikely that there will be a large cohort with their own XR hardware.
5.2.2. Theme: Efficiency
Twenty-five respondents noted the potential for remote studies to take up less time, particularly if remote studies are encapsulated and unsupervised. They stated that this removes scheduling concerns for both the researcher and the participant, and allows experiments to occur concurrently, reducing the total researcher time needed or increasing the scale of experiment. However, there are concerns this benefit could be offset by increased dropouts for longitudinal studies, due to a less “close” relationship between research and participant (R17, R25).
5.2.3. Participant precursor requirements
One respondent noted they needed to run physiological precursor tests (i.e., visual acuity and stereo vision) that have no remote equivalent. Transitioning to remote research has meant this criteria must now be self-reported. Similarly, experiments have general expectations of linguistic and cultural comprehension, and opening research to a global scale might introduce distinctions from typically explored population. One respondent cautioned that further steps should be taken to ensure participants are able to engage at the intended level, as in-lab these could be filtered out by researcher intuition. These themes are summarized in Table 2.
5.3. Data collection
The overwhelming drawback of remote XR research, as reported by the majority respondents, was that of data collection. Excluding changes to participant recruitment, as mentioned above, the issues can broadly be categorized as: (1) bespoke hardware challenges, (2) monitoring/sensing challenges, and (3) data transmission and storage.
The use of bespoke hardware in any type of remote user study is a well-known issue, predominantly regarding the difficulty of managing and shipping bespoke technology to participants and ensuring it works in their test environments. In the context of XR technologies, 13 respondents voiced concerns about the complicated and temperamental system issues that could arise, particularly surrounding the already strenuous demands of PC-based VR on consumer-level XR hardware, without additional overheads (e.g., recording multiple cameras).
Four respondents felt it was unreasonable to ask remote participants to prepare multiple data-collection methods that may be typical in lab-studies, such as video recording and motion tracking. There were also concerns regarding the loss of informal, ad-hoc data collection (e.g., facial expressions, body language, casual conversations).
Finally, concerns were also raised regarding the efforts required to encapsulate all data capture into the XR experience, the effects this might have on data collection (for example, a recent study highlighted a difference on the variability of presence when participants recorded it from inside the VR experience vs. outside; Schwind et al., 2019), the reliability of transferring large amounts of data from participants, and how sensitive information (especially in the context of medical XR interventions) can securely be transferred and stored. This areas perhaps presents the biggest area for innovation for remote XR research, as it is reasonable to assume the academic community could create efficient, easy-to-use toolkits for remote data collection in XR environments which integrate to ethics-compliant data archives.
Many data collection methods were deemed infeasible for remote experimentation: EEG, ECG, eye/hand tracking, GSR, as well as body language and facial expressions. Five researchers noted adaptions they had been working on to overcome these, including using HMD orientation to replace eye tracking, and using built-in HMD microphones to record breaths instead of ECG monitoring to determine exertion, or using the HMD controllers to perform hand tracking.
Respondents also noted some behavioral concerns and changes for remote, unsupervised participants. These included a lack of participation in qualitative feedback (6 respondents); for one researcher (R20), participants were “encouraged to provide feedback but few took the initiative.” Another researcher (R31) stated “Debriefing is such a good space to collect unstructured interview data. Users relax after the questionnaire/debriefing ... produc[ing] a ... meta-narrative where participants consider your questions and their experiences together”. The lack of supervision raised concerns regarding whether participants were being “truthful” in their responses, with one researcher (R41) stating that participants attempted to “game” their study in order to claim the participation compensation. However, others stated that unsupervised studies could reduce research bias arising from their perception of the participants' appearance and mannerisms. These themes are summarized in Table 3.
5.4. Theme: Experiment processes
5.4.1. Process and guidance
Many respondents were concerned that unsupervised participants may conduct the experiments incorrectly, or have incorrect assumptions, or misunderstand processes or target actions. Twenty-four respondents felt that guidance would be better provided (introduction, explanations, etc.) in a lab setting that also allows ad-hoc guidance and real-time corrections.
There were also concerns over the mental state of participants: remote participants “may not take it seriously” or not focus (lack of motivation and engagement) or approach the study with a specific mood unknown to the researcher (R19, R30). Contrasting opinions suggested that participants may feel that the in-lab experience is “overly formal and uncomfortable” (R32).
Some respondents stated that remote experiments risk losing the “rapport” between researcher and participant, which might negatively influence the way a participant performs a remote study. However, one respondent stated that the transition to remote experimentation allowed them different, deeper, on-going connection with their participants. Their research was for a VR machine learning tool, and they found that moving away from in-person experimentation and to a remote workshop process encouraged the up-take of longitudinal community-building tools. The chosen communication method between researcher and user—Discord servers—became a place for unsupervised interaction between participants, and led to an on-going engagement with the research (R33). However it should be considered that any “rapport” between participant and researcher might introduce bias.
5.4.2. Environment
Concern was raised around participants' environments, and their potential varying unsuitability for remote experimentation, compared with controlled laboratory settings. For example, one respondent (R20) stated: “one user reported walking into their whiteboard multiple times, causing low presence scores.” The concern is particularly strong for unsupervised remote experiments, as distractions could enter into the experiment and affect data without the researcher being aware.
This concern was not universal, however. Four respondents noted that their laboratories space was far from distraction free, and even suggested that a remote space could prove freer of interruptions than the space available to them in their research setting; while others stated that researchers should be mindful that the laboratory itself is an artificial space, far more so than where people will typically use their VR setups—in their homes. Five respondents highlighted how XR research could benefit from being deployed in “the participants' own environment”.
The immediate environment of the user was also raised as a concern for VR experiment design: the choice of being able to move freely in an open space in a laboratory against a more adaptive solution for the unknown variables of participants' home environments.
Respondents noted that supporting the different VR and AR setups to access a larger remote audience would also prove more labor-intensive, and would introduce more variables compared with the continuity of the tech stack available in-lab. With remote experiments, and more so for encapsulated unsupervised ones, 10 respondents believe there will be more time spent in developing the system.
5.4.3. Hardware and software
A concern regarding remote experiments, particularly unsupervised, is that calibration processes are harder to verify (R30). This could either cause participants to unknowingly have faulty experiences, and therefore report faulty data; or it will increase time taken to verify user experiences are correct. Unknown errors can effect data integrity or participant behavior. Respondents noted that this type of remote error are often much more difficult and labor-intensive to fix compared with in-lab. This issue is compounded by individual computer systems introducing other confounding factors (for both bug-fixing and data collection) such as frame-rates, graphic fidelity, tracking quality and even resolution can vary dramatically.
Five respondents reflected that overcoming these issues could lead to more robust research plans, as well as better development and end-product software to overcome problems listed. This encapsulation could also lead to easier opportunities for reproducability, as well as the ability for researchers to share working versions of the experiment with other researchers, instead of just the results. It could also help with the versioning of experiments, allowing researchers to build new research on-top of previous experiment software.
Four respondents were aware these advantages are coupled with longer development times. The increased remote development requirements could also be limiting for researchers who face constrained development resources, particularly those outside of computer science departments. This is compounded by the fact that the infrastructure for recruiting remote XR participants, data capture, data storage and bug fixing is not particularly developed. Once these are established, however, respondents felt these might make for a higher overall data quality compared with the current laboratory-based status quo, due to more time spent creating automated recording processes, and not relying on researcher judgement. There are also arguments that the additional development time is offset by the potential increase in participants and, if unsupervised, the reduction in experiment supervision requirements.
Six respondents that use specific hardware in their research, noted that it was currently difficult to measure physiological information in a reliable way, and included hand tracking in this. However, we are aware that some consumer VR hardware (Oculus Quest) allows hand-tracking, and so there is an additional question of whether researchers are being fully supported in knowing what technologies are available to them.
To alleviate issues with reaching participants, two respondents wrote about potentially sending equipment to participants. The limitations of this were noted as hardware having gone missing (which had happened, R35), and participants being unable to use equipment on their own (which had not happened yet).
5.4.4. Research questions
Five respondents noted that their research questions changed or could change depending on whether they were aiming for a laboratory or remote settings. For example, one respondent (R31) suggested that “instead of the relationship of the physical body to virtual space, I'd just assess the actions in virtual space”. Others explored the potentiality of having access to many different system setups, for example, now being able to easily ask questions like “are there any systematic differences in cybersickness incidence across different HMDs?” (R39).
Nine respondents speculated that remote research has potential for increasing longitudinal engagement, due to lower barriers to entry for researcher (room booking, time) and participant (no commute), and that rare or geographically based phenomena could be cheaply studied using remote research; as providing those communities access to VR may be cheaper than relocating a researcher to them.
5.4.5. Costs
Eight respondents noted the potential of remote experimentation for reducing some of the cost overheads for running experiments. Laboratories have important costs that are higher than remote studies: lab maintenance, hardware maintenance, staff maintenance. Without these, costs per participant are lower (and for unsupervised studies, almost nil). As experiment space availability was also noted as a concern for laboratory-based experiments, this seems a potentially under-explored area of benefit, provided remote participant recruitment is adequate. These themes are summarized in Table 4.
5.5. Theme: Health and safety
The leading benefit given for remote user studies was that of health and safety, citing shared HMDs and controllers as a potential vector for COVID-19 transmission, as well as more general issues such as air quality in enclosed lab spaces. Concerns were raised for both viral transmission between participants, and between participant and the researcher. This concern has also increased administration overheads, with 6 respondents stating it could be more time consuming to prepare the lab and organize the studies or using new contract-tracing methods for lab users.
However, respondents also raised concerns about additional safety implications for remote participants. The controlled lab environment is setup to run the study, whereas remote participants are using a general-purpose space. One AR researcher who conducts research that requires participants to move quickly outside in fields noted his study could be considered “incredibly unsafe” if unsupervised or run in an inappropriate location. Additionally, for health and mental health studies, in-lab allows for researcher to provide support, especially with distressing materials. Finally, VR environment design has a direct impact on the level of simulator sickness invoked in participants. There were questions about the responsibility of researchers to be present to aid participants who could be made to feel unwell from a system they build. These themes are summarized in Table 5.
5.6. Theme: Ethics
Three ethics concerns were reported by respondents: encouraging risky behaviors, responsibility for actions in XR and data privacy. An example of this might be the ethical implications of paying participants, and therefore incentivising them, to take part in what could be considered a high-risk behavior: entering an enclosed space with a stranger and wearing a VR HMD.
Respondent (R30) raised the question of liability for participants who are injured in their homes while taking part in an XR research project. The embodied nature of XR interventions—and most respondents used this embodiment in their studies—could put participants at a greater risk of harming themselves than with other mediums.
Finally, while cross-cultural recruitment was seen as a potential boom for remote research, questions were raised about ethics and data storage and protection rules when participants are distributed across different countries, each with different data storage laws and guidelines. Although not limited to XR, due to the limited number of VR users, and the disproportionate distribution of their sales, it seems the majority of remote VR participants will originate from North America, and ethics clarification from non-US-based universities are needed. These themes are summarized in Table 6.
6. Future pandemic and COVID-19 implications
COVID-19 impacted many user studies around the world, but XR research's dependence on shared hardware, especially HMDs, has potentially unique or more impactful implications than many other types. The concerns reported by respondents were particularly related to COVID-19, and therefore should be reduced as the pandemic is resolved. However, as it is currently unclear when the pandemic will end, or if future pandemics will arise, we felt it was useful to discuss them in a dedicated section.
Most respondents noted that COVID-19 caused a suspension of studies and that they were unclear how long the suspension would last for, resulting in an overall drop in the number of studies being conducted, with 30 respondents stating it will change the research they conduct (e.g., moving to online surveys). The continuation of lab studies was eventually expected (and anecdotally, has mostly resumed) but with added sanitizing steps. However for many, it was unclear what steps they should take in order to make XR equipment sharing safe. These concerns extended beyond the XR hardware to general facility suitability, including room airflow and official protocols which may vary for each country and/or institution.
Five respondents also had concerns about participant recruitment and responsibilities regarding them. There were worries that lab-based recruitment would be slow to recover, as participants may be put off taking part in experiments because of the potential virtual transfer vectors. Similarly, respondents were concerned about being responsible for participants, and putting them in a position in which their is a chance they could be exposed to the virus.
There was also concerns around COVID-19 and exclusion, as researchers who are at high risk of COVID-19 or those who are in close contact with high risk populations, would now have to self-exclude from lab-based studies. This might introduce a participant selection bias toward those willing to attend a small room and sharing equipment.
It should be noted that not all labs are facing the same problems—some respondents had continued lab-based experimentation during this period, with COVID-19 measures ensuring that participants wore face masks during studies. This was considered a drawback as combined with an HMD, it covered the participant's entire face and was cumbersome. These measures are also known not to be 100% protective.
7. Discussion
In the previous section, we presented the results as themes we found in our analysis. Some of these presented common characteristics and some issues were reported in multiple themes. We now summarize the results, highlight the key points and suggest important questions for future research.
7.1. Recruitment and participants
As with non-XR experiments, researchers are interested in the potential benefits of remote research for increasing the amount, diversity and segmentation of participants compared with in-lab studies. However, with many respondents reporting that it has been difficult to recruit XR participants, it seems there is a gap between potential and practice. The unanswered question is how to build a pool of participants that is large and diverse enough to accommodate various XR research questions and topics, given that there are few high-end HMDs circulating in the crowdworker community (Mottelson and Hornbæk, 2017; Ma et al., 2018). So far, we have found three potential solutions for participant recruitment, although each requires further study:
(1) Establish a dedicated XR crowdworker community. However, concerns of non-naivety (Paolacci and Chandler, 2014), which are already levied at the much larger non-XR crowdworker participant pools, would surely be increased. We would also have to understand if the early version of this community would be WEIRD (Henrich et al., 2010) and non-representative, especially given the cost barrier to entry for HMDs.
(2) Leverage existing consumer XR communities on the internet, such as the large discussion forums on Reddit. These should increase in size further as they shift from early-adopter to general consumer communities. However, these communities may also have issues with representation.
(3) Establish hardware-lending schemes to enable access to a broader base of participants (Steed et al., 2020). However, the cost of entry and risk of these schemes may make them untenable for smaller XR research communities.
It is also not clear, beyond HMD penetration, what the additional obstacles are that XR poses for online recruitment. Technical challenges (e.g., XR applications needing to run on various devices, on different computers, requiring additional setup beyond simple software installation) and unintuitive experiment procedures (e.g., download X, do an online survey at Y, run X, record Z) for participants are notable distinct issues for remote XR research. It is also unclear if the use of XR technology has an impact on what motivates participants to take part in remote studies, an area of study that has many theoretical approaches even in the non-XR area (Keusch, 2015).
7.2. Data collection
Respondents feel that many types of physiological data collection are not feasible with either XR or non-XR remote research. For remote XR research, there are unique concerns over video and qualitative data collection as using XR technologies can make it (technically) difficult to reliably video or record the activity, as well as moving participants' loci of attention away from the camera or obscuring it behind an HMD. However, the hardware involved in creating XR experiences provides a variety of methods to gather data, such as body position, head nodding, breath-monitoring, hand tracking, HMD angle instead of eye tracking. These can be used to explore research topics that are often monitored via other types of physiological, video or qualitative data, such as attention, motivation, engagement, enjoyment, exertion or focus of attention. It would be useful for XR researchers to build an understanding of what the technologies that are built into XR hardware can tell us about participant experiences, so as to allow us to know the data collection affordances and opportunities of XR hardware.
That said, the infrastructure for collecting and storing this (mass) of XR data remotely is currently not fully implemented, and we are not aware of any end-to-end standardized framework. However, work is being done to simplify the data collection step for XR experiments build in Unity (Brookes et al., 2020). There are also opportunities to further develop web-based XR technologies that could send and store data on remote servers easily. There are also ethical concerns, as respondents were unclear on guidance regarding data collection from participants located in other nations, particularly when they should be paid. This includes how the data is collected, where it should be stored, and how can be manipulated.
7.3. Health, safety, and COVID-19
During the COVID-19 pandemic, there was a period in which many laboratories were considered unsafe for running user studies. Although some respondents reported being able to work in-lab, the limitations mean it was not feasible to run user studies under normal conditions. The main concern was, both during a pandemic and generally for maximizing the health of participants, a lack of standardized protocols to ensure safety of researchers and participants while running user studies and lack of clarity regarding ethics protocols of research institution. For XR research, it was unclear how to adequately sanitize equipment and tools, as well as how to maintain physical distancing. There were also concerns about the comfort of participants if they are required to wear face masks alongside HMDs. Finally, respondents reported concerns about a potential long-term fall in user motivation to take part in such experiments, when HMDs are a notable infection vector. There are distinctly different safety and ethics concerns around remote XR experiments, including the research responsibility for not harming participants (e.g., ensuring environments are safe for the movements, and not inducing simulator sickness), which, while also true of in-lab experiments, are considered a greater challenge when a participant is not co-located with the researcher.
7.4. Mediated impact
Respondents reported framing their research questions and experiments differently depending on the target experiment setting. The strongest transition was that of an in-lab study of participants using an AR HMD (Hololens), which changed to a remote study that had participants watch a pre-recorded video of someone using the AR HMD. It seems these kinds of transitions will continue to be necessary depending on how esoteric the hardware is, with fewer concerns for AR smartphone investigations.
A concern for respondents was that remote settings introduce additional uncontrolled variables that need to be considered by researchers, such as potential unknown distractions, trust in participants and their motivation, and issues with remote environmental spaces. However, previous research shows that most HMD-wearing remote participants engage in space well-known to them (the home) and predominantly when they are alone (Ma et al., 2018), which could alleviate some of the environmental space and distraction concerns. Further research into how a home environment could impact XR studies is needed, and the creation of well-defined protocols to alleviate uncontrolled influences remote XR results. Beyond this, we also need to understand any impact that remote experiments may have on results compared with in-lab experiences, especially if we are to be able to reliably contrast lab and remote research. Previous research for non-XR experiments suggest that distinctions between lab and remote settings exist (Stern and Faber, 1997; Senior et al., 1999; Buchanan, 2000), but it has been theorized that the impact might be less for XR experiments, as you “take the experimental environment with you” (Blascovich et al., 2002).
7.5. Maximizing remote benefits with “encapsulated” experiments
Perhaps the most notable suggested benefits of conducting XR experiments remotely were: (1) the ability to increase participation and reach of recruitment by reducing the dependency on a researcher's schedule, geographic location, organization or resources; (2) to reduce the demand on the researcher's time or in-lab resources (both hardware and location) when conducting and supervising experiments; and (3) to encourage experiments that are archiveable, re-deployable, and replicable, by reducing the dependency on the researcher's location or bespoke hardware setup.
A remote experiment design approach that enables these advantages would allow the experiment to be run unsupervised, use off-the-shelf XR hardware, allow participants to self-opt-in, for the experiments to be self-guided and feature their own tutorial or on-boarding process, for the experiment software to be easy to be installed by participants and for the data to be automatically collected and returned to the researcher. We will refer to this type of experiments as “encapsulated”, as all major aspects of the experiment process are encapsulated into the distributable experiment software.
Encapsulated experiments, deployed as all-in-one experiment-and-data-collection bundles, could run unsupervised, offering notable time-saving implications for researchers (and participants). This type of “encapsulated experiment” can also improve replication and transparency, as theorized by Blascovich et al. (2002), and allow for versioning of experiments, in which researchers can build on perfect replicas of other's experimental environments and processes. Finally, due to the similar nature of XR hardware, data logging techniques could easily be shared between system designers or standardized; something we have seen with the creation of the Unity Experiment Framework (Brookes et al., 2020).
However, there are some limitations to this approach. It is likely it will require additional development time from the researchers, especially as a comprehensive experiment framework is established. In addition, there are data collection limitations for remote XR studies, as discussed in previous sections. It is also interesting to consider how encapsulation might work for AR investigations, as the environment will only partially be controlled by the designer.
8. Core questions
It is clear from our survey that respondents believe that remote XR research has the potential to be a useful research approach. However, it currently suffers from numerous limitations regarding data collection, system development and a lack of clarity around participant recruitment. Analysis of our survey results and literature around remote and remote XR research suggest that, to better understand the boundaries of remote XR experimentation, researchers need answers to the following questions:
(1) Who are the potential remote XR participants, and are they representative?
(2) How can we access a large pool of remote XR participants?
(3) To what extent do remote XR studies affect results compared with in-lab?
(4) What are the built-in XR data collection affordances of XR hardware, and what can they help us study?
(5) How can we lower the barriers to creating less researcher-dependent remote experiment software, to maximize the potential of remote XR research?
9. Exploring core questions through the design and application of encapsulated experiments: A case study
The previous section outlined five core questions paramount to better understanding the process of remote XR experimentation: who are the remote XR participants; how can they be accessed; how do remote XR experimentation impact results compared with in-lab; are there certain built-in XR data collection affordances native to VR hardware; and how can we lower the barriers to creating remote experimental software experiences.
In order to explore these questions, we ran a case study of two remote XR experiments and reflected on how the design, experiment process and results respond to the questions above.
The following sections present the design of these experiments, experiment results related to recruitment and experiment processes, and a discussion of these findings in the context of the previous thematic analysis and the questions outlined above.
10. Case study design
We ran two experiments that tested an encapsulated approach to remote XR experimentation. An encapsulated approach was chosen as it offered the most potential benefits for researchers, including increasing participation and reach of recruitment; reducing demand on the researcher's resources; and encouraging experiments that are archiveable, re-deployable, and replicable.
In this section, we present our process for recruiting participants, and the design and implementation of the experiment process and data collection.
10.1. Recruitment and participants
Calls for participants for the studies were placed in three locations: on social media platforms Reddit and Twitter, and on XRDRN.org, a web directory for remote XR experiments. These were non-promotion or unfunded posts, which took the form of normal content typically shared on the social platforms, and did not make use of the funded advertisement options that the platforms offered.
The Reddit call was placed in the most popular IVR “subreddit” or discussion community, /r/Oculus. The Twitter post was promoted on the researcher's timeline and amplified via retweets from their network or through organic finding of the post, and labeled with the hashtag #vr.
The calls promoted four key aspects: VR, language learning, the compensation ($14) and the time commitment (40 min). The action was to click a hyperlink which took people to website that provided more information and a step-by-step guide to the experiment process.
Study participants were able to opt-in, download and take part in the study without approval from a researcher; this is a similar approach to how many form-based surveys are conducted.
10.2. Experiment process
The experiment website outlined (1) the goals of the study; (2) two major inclusion requirements (presented in bold, including a requirement of access to a VR headset); (3) the experiment sections and duration; (4) the compensation level; and (5) a link to a privacy policy, and contact information for the researcher and the university's research ethics office.
After the study had potentially recruited enough participants to use the experiment budget, a notice explaining that participants taking part from that point onwards may not be compensated was added. From this point, participants were only compensated if previous participants were ineligible for compensation by either (1) not completing all aspects of the study; (2) not taking part according to requirements; (3) providing low quality answers.
If participants clicked the “Continue” link, a page with four consent statements loaded. Choosing “Continue” here presented a final page showing step-by-step instructions for the experiment.
The webpage showed a randomly generated three-letter participant ID (e.g., MGY), and instructed participants to enter this inside the VR experience. We felt the use of a three letter ID was memorable for participants, who would have to entire the ID inside the VR experiment, and provided 17,576 unique IDs.
The webpage also presented the three stages of the experiment: (1) Download and prepare the VR software, a link and process to download and setup the VR software; (2) Do the VR experiment, an explanation of how to run the software and a short outline of what to expect in the experience; and (3) Complete a survey about your experience, which provided a link to a survey website.
Participants were asked to ensure they had an unbroken period of time to do the experiment, and to ensure that their area was free form distractions.
10.3. In-VR experiment process
Participants were asked to download the experiment in .zip format, and then extract it onto their computer (instructions were provided how to do this). They were then asked to run the named executable file, move to the center of their play area and continue in the headset.
The VR experience loaded into an on-boarding process. Participants were shown instructions written on a virtual wall explaining how to move and grab objects. Participants were asked to move and grab a cube to continue the experiment.
After grabbing the cube, participants were teleported to a tutorial area. At this point, participants were randomly assigned an experiment condition. They were also asked to enter their ID by moving letter blocks into set positions.
Participants were then introduced to the two main parts of the experiment, (1) testing and (2) learning. For both of these aspects, the participant stood in one location and different virtual objects and instructions were presented in their immediate surroundings. A voice-over described the process and provided instructions, as well as bullet points written on a virtual wall.
The experiment was design so that complete actions would be detected when completed, allowing the participant to continue. For example, in the testing section, participants had to press an audio player button to hear a second-language word, then press a button to select a translation for that word, and press a final button to confirm the selection. In the learning section, the correct actions were monitored, such as detecting when a jug had rotated over 90 degrees in order to determine if the participant had conducted a pouring action. This meant that the experiment was self-paced, progressing when participants successfully completed a target interaction. A skip button was also available in case a participant was unable to complete an interaction.
At the end of the experiment, participants were instructed to remove their headset and continue on the website from before.
10.4. Data collection
Data was collected from participants in two places: during the VR session and through an online form-based survey after the VR session.
During the VR session, telemetry regarding the participant's movement was recorded. This was head and hand locations, including positions in real-space (x, y, z) and rotation (pitch, yaw, roll) of each body part. Data was sampled every 100 ms, or 10 times per second.
During the testing processes, whether the audio button was pressed, an answer pressed, and the submit button pressed, was recorded for each question, as was the length of time to submit (recorded as time between pressing the audio button and pressing the submit button).
During the learning process, whether an action was completed or not was recorded.
The participant's ID was also stored, as well as a timestamp for when the VR experiment was started and the directory structure in which the executable file was stored. This information was stored in a CSV file and sent to a web-server in a single bundle near the end of the experiment. The file transfer was handled using a web request from within the software, and a PHP processor on the server.
For the web-based form survey, the participant's ID was auto-populated into the form. All other information from the survey was stored in a database using the open source LimeSurvey platform.
11. Results
This section presents results of the two encapsulated experiments relating to the previous areas: participants and recruitment, experiment process and data collection.
11.1. Study participants and recruitment
Both experiments were able to attract large sample in a short period of time. In under 24 h, the experiments had 56 and 74 people take part (these numbers include anyone who had started at least one part of the experiment). After removing people who did not fully complete the experiments or were ineligible for participation, 48 and 56 participants provided viable data.
Most of the removed participant (21) were due to participant data existing for the online survey but not for the VR software. Of these 21 removals, only one responded to communication from the researcher.
Two participants were removed for having high pre-existing knowledge of the experiment subject matter.
Two participants were removed for participating in the VR software but not the online survey.
Only one participant (that we are aware of) participated—and completed—an experiment despite not owning VR hardware. They reported their process as “a hacky way of having VR to the pc, via reverse tethering with Virtual Desktop and OculusGo, I also had to use a combination of keyboard and controller to perform the moves needed.” This unusual setup was detected from playing back the telemetry, due to the artificial movement of their head and hands. Their data was removed from the study.
The sample provided a notable range of ages, arguably wider than many on-campus based recruitment processes, which are known for a 18–21 year-old bias. Viable participants ages in the first study ranged from 18 to 66, with an average age of 27 (SD = 6.75); the second ranged from 18 to 51, with an average age of 25 (SD = 7.79).
However, gender was highly skewed, with women under-representing in both the first [male (38), female (8), other/did not say (2)] and second [male (50), female (1), non-binary (3), and or other/did not say (0)] experiments.
Although country data was not recorded, observation of participant payments suggested a similar number of payments in North American currencies (USD and CAD) and European currencies (EUR and GBP) for the first experiment; and a skew toward North American currencies the second. This suggests that their may have been something in the recruitment that encouraged participants from different geographies.
In follow-up communication with viable participants following the first case study, participants overwhelming listed the Reddit call for participants as their source on recruitment; all 38 of those who reported their referrer mentioned Reddit and the forum /r/Oculus.
11.2. Experiment process
In the second experiment, participants were specifically asked to record factors that may have negatively impacted their experiment experience. Thirty-seven (of 54) participants recorded issues. These broadly fall across three categories: environmental issues (21), hardware issues (6), and issues in the software (13).
The environmental issues reported can be categorized as follows: issues noise (14); with physical space of the “play area” (3); and with managing other responsibilities during the experiment (2).
Noise issues can further be broken down into general background noise (6), including “air conditioning buzz”, music playing, “sounds from other people in the house watching TV”, “someone was mowing their lawn”, “noises that occur on a regular Sunday” and general background sound; and attention-grabbing noises (7), such as smartphone notifications (4), an opening door (1), chat room notifications (1), and the sound of the graphics card fan turning up (1).
Physical space issues included a distraction from seeing the virtual reality space boundary warning (2), self-monitoring their position in the room (1), and walking into obstacles (“bumped into my tower fan”) (1).
Managing other responsibilities included “having a brief phone call” and “watching 4-mo[nth-old] baby, fussing sporadically”. It is unclear if the baby or the guardian were fussing.
The hardware issues reported were miscellaneous audio issues (2), a wireless connection dropping (1), too much light getting into headset (1), the headset being tethered to the PC with a wire (1), and controller tracking issues (1).
The software issues, where described, predominantly focused on issues with in-game physics collision. Most reports only mentioned a pen object that would fall through a table, rather than sit on top of it, or slowed the game down when it connected with a piece of paper. One user reported feeling too tall for the software (it is likely the virtual work-surface was positioned too low for him). Due to the lack of supervision and rapid recruitment cycle, software issues may have been resolved earlier in an in-lab study, instead of unresolved in the remote unsupervised one.
Despite this high number of potential distracting factors, some responses specifically stated that they did not believe the potentially distracting factor they listed had a negative impacted their performance or experience. Therefore, we should be careful not to consider this feedback as a quantifiable indication of how distracted these participants felt.
Notably, no users recorded procedural issues with the experiment process. However, there were two participants who completed the VR software experience but not the online survey afterwards. It is possible these participants dropped out due to unclear instructions regarding the full experiment; however it is also possible that they only wanted to experience the VR software and were not interested in completing the experiment.
11.3. Data collection
We believe the automated data collection processes were mostly successful. We only identified one participant whose VR experiment data did not automatically transfer to the web-server. Their data was able to be retrieved from the local data file stored on their computer, once instructions were sent by the researcher. The original failure of data collection was potentially due to local firewall settings on the machine, or remote web-server downtime.
There were multiple other participants—five in the first experiment and 18 in the second—whose VR experiment data did not transfer to the web-server. However, all but one of these participants did not respond to email communication, and on inspection of their online survey answers and completion time, it is likely that these participants did not actually take part in the experiment. Instead, it seems they completed the web-survey only, hoping to gain the compensation.
One of these provided a CSV file that demonstrated an exact match of the data from another participant, except with the ID information changed. On discussing with both participants, it emerged that the sender of the file had invited his contact to take part in the study, and then asked his friend to share his CSV file. We assume this participant then changed the ID information and forwarded his contact's information, passing it off as his own.
One participant, mentioned earlier, was excluded for having unusual movement telemetry due to not actually using a VR headset.
In the second study, there was one participant who entered an incorrect ID in VR that was different from the one auto-populated into the survey. This did not prove an issue, however, as the timestamps of the data made it easy to identify the matching submissions. The error was also mentioned in the participant's comment at the end of the survey.
12. Discussion and core questions
In this section, we discuss the core questions in light of the findings from the case study results and the process of designing and running the experiments.
12.1. Who are the potential remote XR participants, and are they representative?
The number of people with access to a home IVR set is ever-increasing, with one prediction suggesting that around 43.5 million high-end consumer headsets will have been sold by 2025; Alsop, 2022.
As this is such a large population, it is difficult to write in too much detail about who the potential remote participants are. However, this case study provides evidence regarding the representation available in recruiting through some of the most active subject-matter social media channels. In this case, that is Reddit's VR-specific communities, which could be considered a low effort, high reach recruitment method.
The results here show two different notable demographic factors: first, the groups are so far more representative of the age range of society than many in-lab, convenience sampled participants. The average ages of 27 and 25 is older than many studies, which can be heavily weighed toward 18–22 year-olds. Conversely, the gender demographics present a huge under-representation of women, with a total of 88 males, 9 females, 3 non-binary and 2 other/did not say. This is skewed more dramatically even than Reddit's typical gender disparity (63.8% male vs. 32% female). For comparison, a previous, in-lab study on a similar topic had 15 males, 7 females, and 1 other/did not say.
The disparity in participants payment regions between North American and Europe between the two experiments suggests something about the participant recruitment may have differed. One potential explanation is the time the advertisement was added to Reddit. For the more even spread, the advertisement was posted around midday GMT on a Saturday; whereas for the second experiment, with the North American bias, the experiment was posted around 8 p.m. GMT on a Saturday. Therefore the second experiment's timezone may have been unfavorable to potential participants based in Europe.
12.2. How can we access a large pool of remote XR participants?
This case study provides evidence that recruiting from existing online communities provides a large number of participants, quickly. Recruiting 56 and 74 participants in under 24 h, of which 48 and 56 provided viable data, appears relatively high compared with many VR HCI studies. For comparison, a pre-pandemic, in-lab, supervised VR experiment on a similar subject matter took 28 days to recruit 25 participants, 24 viable.
As the posts on Reddit were not paid for, it was also a cost-effective way of recruiting participants.
It seems reasonable to argue that part of the efficiency of the recruitment process is due to the remote and unsupervised approach. A large number of potential participants were able to be reached via the recruitment medium (Reddit); while the self-opt-in and ability to run the entire process without the blocking influence of researcher approval or processing led to high uptake and completion rates.
Earlier, we summarized three approaches to accessing a large pool of remote participants, of which two were attempted here: (1) leverage existing consumer XR communities (Reddit's /r/Oculus); and (2) using a dedicated XR crowd-worker community (XRDRN). As all participants who reported their origin mentioned /r/Oculus, it is clear that, at this stage, leveraging existing consumer XR communities is a more fruitful approach.
12.3. To what extent do remote XR studies affect results compared with in-lab?
Our case studies do not include a controlled in-lab experiment to compare with, which would allow us a quantified insight into whether the remote nature of the experiments impacted the results. However, comparing with a prior in-lab experiment covering a similar topic as the case study, we noticed that there were a larger number of potential participants with invalid data for the remote experiment then in-lab. The reason for excluding these remote participants were either they did not meet the required participation conditions, with too much prior knowledge (2) or that they did not have the correct hardware (1); or some of their data was missing (23). Thus, it seems likely that remote, encapsulated studies may be more likely to have a higher percentage of participants who must be removed than in-lab studies (however, we should note that the in-lab study mentioned was uncompensated, which likely has an impact on recruitment self-selection).
One of the notable concerns expressed by researchers regarding remote experiments was the potential for the home environment to be distracting. In our second case study, we specifically asked participants to report potentially distracting factors, which presented a large list. However, despite the majority of participants stating a potentially distracting factor, some participants specifically stated that, although their listed factors could be considered distracting, they did not feel it harmed their experience or engagement with the study.
A core question, then, is not what may distract, but how distracted were participants during a remote XR study, and how distracted is too much? It might be useful to frame questions regarding distractions more directly, and rely on the participant's judgement regarding how distracting something is: “did you experience any distractions that you feel notably impacted your ability to take part in the study?”. Interestingly, some of the participants listed factors that could be experienced in-lab conditions, such as a door opening or hearing an air conditioning unit. Therefore, it is also possible that lab-based settings featured distracting factors for some participants. Perhaps better processes in understanding remote experiment distractions may also lead to advancements in understanding distracting factors of in-lab studies.
It is also important to consider the time considerations of running the study. At a minimum, the total number of viable participants in these two studies (102) multiplied by the length of the experiment (40 min) would have resulted in 68 h of supervision should the studies had been run in-lab. Considering a typical 8-h lab day, that is over 8 days for the experiment process in addition to however long in-lab recruitment might take. The remote encapsulated process took under 24 h, as many experiments ran concurrently.
12.4. What built-in XR data collection were used, and what can we study?
Information regarding the participant's head and hand position, captured from the VR hardware, served as a good indicator regarding attention and participation. For example, long periods of stillness indicates a potential external distraction, while a headset lowered to the floor suggests that it has been removed and the participant is no longer inside the VR session. Unusual movement suggests that the participant may have a compromised or emulated VR system.
In this case study, the telemetry data from these was also useful in detecting unusual participant movements, which resulted in the detection of someone using inappropriate hardware that did not fit the inclusion criteria.
Going beyond this, it does not seem infeasible to create a algorithm that examines this information and flags where potential anomalies occur, and could serve as a useful in-VR alternative to attention check questions.
12.5. How can we lower the barriers to creating less researcher-dependent remote experiment software, to maximize the potential of remote XR research?
In order to understand how to lower the barriers to creating less researcher-dependent remote experiment software and maximize the potential of remote XR research, we first need to understand what the barriers of creating this software is.
For both studies, there was additional labor compared with creating an in-lab experiment, which included (1) more thorough software development to ensure the experiences were ready for remote, unsupervised deployment; and (2) process development to ensure participants were informed enough to successfully take part in the experiment.
In terms of software development, the major addition was code for collecting the telemetry data and the posting of it to a web-server. Two types of data were collected: general player movement (position and orientation of the participant's head and hands); and experiment performance data, including information about progression through the experiment, successful completion of actions, answers and time taken for pre- and post-test questions.
The solution used to collect these involved addition C# code in the Unity environment and PHP code on a web-server. These are two separate programming languages and, typically, separate programming skill-sets. However, existing code created specifically for recording VR telemetry data and for uploading Unity data to a web-server was readily available from code repositories of existing researchers. Our experience with this specific repository was quick and easy, and we believe it would be for most Unity-based VR projects than run on Windows systems. We recorded the estimated additional time of deploying and testing this as under 5 h, although the experiment creator was experienced in C# and PHP programming.
The more notable additional work was in preparing the instructions for participants, scripting the tutorial, and testing the experiment software to ensure that it was intuitive to participants, who would go through the experiment unsupervised. This additional overhead was not minimal, adding perhaps two extra weeks to the development process, and required additional programming for the tutorial, additional vocal recording, and the creation of an experiment process website with a function for auto-generating a user ID.
We considered these additional steps a worthwhile time investment, considering the rapid recruitment and fast experiment process timelines. Of course, this benefit is limited if a study is looking to recruit only a few participants, but increases in value if the experiment is targeting the recruitment of a large number of participants is high. Given that most researchers are looking for a large number of participants, it seems like time required to create an encapsulated study would generally be a sensible investment.
13. Potential design improvements
In designing and running the two case studies, a few potential design and process improvements were observed that may improve future encapsulated studies. These are discussed below.
13.1. Build all data collection into VR
An additional administrative task that required extra work and could have introduced data integrity issues was the separation of data between the data sent from the VR experiment software, and the data captured in the post-VR survey via a web browser form. Researchers had to manually match the participant IDs between the submissions, which sometimes did not match. This was typically because the web browser survey was submitted without participants taking part in the VR experience; likely to receive the offered participant compensation without taking part in the experiment.
These issues could be avoided by providing users with an in-VR survey, instead of asking them to leave the experience to submit it. Research into how surveying participants directly in VR is already been conducted (Schwind et al., 2019; Alexandrovsky et al., 2020; Putze et al., 2020) without adverse impacts on outcomes, and with the additional benefits of reducing a study's duration and reducing participant disorientation (especially if, after a survey, the participant must return to the XR experience.
13.2. Develop web-based XR experiment, rather than hardware-specific
It was suggested earlier that a web-based XR experiment might make it easier and more reliable to transfer experiment data to a web-server. However, this case study presents other reasons why a web-based XR experiment might be beneficial.
These case studies ran on Windows, which meant that some users had to have their headsets connected to their computer via cable (tethered) rather than operate without a wired connection. Two users mentioned they found this a distraction, indicating that they are predominantly users of a wire-free IVR experience.
By creating the experience as web browser-based IVR experience, participants could run the software from whatever device they prefer, removing this concern.
Another benefit would be that researchers would not be asking participants to run executable files on their computer systems. Multiple potential participants raised concern of the executable file as a potential infection vector for computer viruses during the recruitment of both studies. Finally, the web browser-based software would be less likely to have data transfers blocked by the operating system's firewall.
However, there are notable performance and functionality limitations with developing intensive XR applications inside web browsers, and so it is important that the researcher understands whether these limitations may harm the experiment experience.
13.3. Smarter condition assignment and compensation management
In both case studies, participants were assign randomly to a condition inside the VR software. This led to an uneven number of participants in each condition. It might be helpful to use a smarter allocation of experiment condition, based upon knowledge of the number of participants for each condition. As the VR software is already connected to a web-server to send data, it could query the remote database and assign alternating conditions after each submission, reducing the potential disparity in assignments for each conditions.
Similarly, in both case studies, access to the experiments had to be manually stopped when the experiment compensation budget limit was reached. This required consistent monitoring of the experiment, and led to at least one late-night finish for the researcher. If the experiment monitored its own number of submissions, like many unsupervised survey studies do, it could reduce this demand on the researcher, and the associated potential of exceeding the experiment's budget.
14. Limitations and further work
This paper identified numerous areas for further research into remote XR experimentation. The core questions identified earlier—who are the potential remote XR participants, and are they representative; how can we access a large pool of remote XR participants; to what extent do remote XR studies affect results compared with in-lab; what are the built-in XR data collection affordances of XR hardware, and what can they help us study; and how can we lower the barriers to creating less researcher-dependent remote experiment software, to maximize the potential of remote XR research—all require more in-depth study to add to the findings presented here.
This is true both for encapsulated approaches, which were explored in the case studies presented here, and for other approaches to remote XR experiment design, particularly supervised experiments and those using specialized hardware or novice XR participants.
For encapsulated studies, a useful next step would be to contrast an encapsulated study with a non-encapsulated study for the same experiment, with particular attention paid to determining if there was any difference in results, participant experience or participant recruitment and gender. Additionally, exploring a similar comparison between an encapsulated study deployed remotely and one deployed in-lab might also provide useful insight and better inform us of any distinctions between the experiences.
We should also consider further work into the requirements and development of toolkits to support remote XR data capture and transfer. Leveraging existing tools designed for IVR data capture, such as the Unity Experiment Framework (Brookes et al., 2020), could be a potential starting point for this exploration.
It would also, perhaps, be helpful to split investigations from the XR umbrella into two: IVR and AR. While overlap exists, there will almost certainly be nuanced, unique distinctions between an experience that seeks to remove you from your surrounds (IVR) and one that seeks to augment them (AR).
Whatever the further work in this area, it will be of paramount importance to identify what type of remote XR experiment approach is being used, as it has become clear that approaches to remote XR experimentation can be broad with many varying factors.
15. Conclusion
The analysis of a survey of 46 XR researchers suggested that conducting XR experiments with remote participants could be a valid and efficient data collection process. However, concerns around a lack of information regarding the remote XR experimentation process were summarized in five core questions for the XR research community: (1) who are the potential remote XR participants, and are they representative; (2) how can we access a large pool of remote XR participants; (3) to what extent do remote XR studies affect results compared with in-lab; (4) what are the built-in XR data collection affordances of XR hardware, and what can they help us study; and (5) how can we lower the barriers to creating less researcher-dependent remote experiment software, to maximize the potential of remote XR research.
The follow-up case study of two XR experiments attempted to examine a specific remote XR approach that could provide efficiency benefits for researchers, in which recruitment, the experiment process and data collection were automated (the encapsulated approach). Analysis of the case study of this approach provides the following responses to the above questions: (1) Potential remote XR participants, especially when recruitment is automated, can be highly unrepresentative. We found a large discrepancy in gender representation—although it may expand the typical age range found in university campus-based convenience sampling. (2) It is quick and easy to access a large pool of remote XR participants using existing popular XR communities. (3) A better understanding of what comparisons between remote and in-lab are of interested is needed. If our interest in attention and distraction, then establishing quantitative measurements and processes would be a useful next step. If the question is whether experiments can recruit, run and provide data remotely, then the answer is yes. And if the question is about how efficiently or quickly they can be run, then from the evidence here, a remote, encapsulated process is a superior approach. (4) Data collection and submission of head and hand position and rotation can be efficiently captured without issue, and this data can be successfully used to identify and remove ineligible participants. (5) Developing encapsulated experimentation software and processes took more time than an in-lab equivalent might have. However, the use of data collection and submission approaches and code written by other XR researchers and available in the public domain was easy to use and quick to implement. We also suggest three further design approaches that could aid this further. First, ensure all data collection is inside the VR software, rather than moving participants between different mediums (such as web-browser). Second, develop web-based XR software, which increases the flexibility for participants and reduces potential data collection issues. Finally, use smarter condition assignment and compensation management, to equalize the number of valid participants in each condition and reduce administrative overheads.
In summary, the primary concern of remote XR research may still be akin to in-lab research: finding representative participants. However, once found, the efficiency of processing them seems to be a major benefit of the remote encapsulated approach.
Data availability statement
The raw data supporting the conclusions of this article will be made available by the authors, without undue reservation.
Ethics statement
The studies involving human participants were reviewed and approved by QMUL Ethics Committee. The patients/participants provided their written informed consent to participate in this study.
Author contributions
JR and LT contributed to the conception and design of the survey and case studies and for the conception and design of the case study experiments and authored the Discussion section. JR created the literature review, recruited for the expert survey, organized, analyzed, reviewed, and summarized the results, recruited for the case study, designed, developed, and ran the experiments, organized the data, and performed the statistical analysis. Both authors contributed to manuscript revision, read, and approved the submitted version.
Funding
This work was supported by the EPSRC and AHRC Centre for Doctoral Training in Media and Arts Technology (EP/L01632X/1).
Acknowledgments
The authors of this paper would like to acknowledge Francesco Soave, Nick Bryan-Kinns, and Ildar Farkhatdinov, who contributed greatly to precursor for this work, which presented the surveying and analysis of the 46 XR researchers.
Conflict of interest
The authors declare that the research was conducted in the absence of any commercial or financial relationships that could be construed as a potential conflict of interest.
Publisher's note
All claims expressed in this article are solely those of the authors and do not necessarily represent those of their affiliated organizations, or those of the publisher, the editors and the reviewers. Any product that may be evaluated in this article, or claim that may be made by its manufacturer, is not guaranteed or endorsed by the publisher.
References
Alexandrovsky, D., Putze, S., Bonfert, M., Höffner, S., Michelmann, P., Wenig, D., et al. (2020). “Examining design choices of questionnaires in VR user studies,” in Proceedings of the 2020 CHI Conference on Human Factors in Computing Systems (Honolulu, HI), 1–21. doi: 10.1145/3313831.3376260
Alsop T., Augmented reality (AR), virtual reality (VR), and mixed reality (MR) market size worldwide in 2021 and 2028. Statista. (2022). Available online at: https://www.statista.com/statistics/591181/global-augmented-virtual-reality-market-size/
Amores, J., Richer, R., Zhao, N., Maes, P., and Eskofier, B. M. (2018). “Promoting relaxation using virtual reality, olfactory interfaces and wearable EEG,” in 2018 IEEE 15th International Conference on Wearable and Implantable Body Sensor Networks (BSN) (Las Vegas, NV: IEEE), 98–101. doi: 10.1109/BSN.2018.8329668
Aronson, E. (1969). The theory of cognitive dissonance: a current perspective. Adv. Exp. Soc. Psychol. 4, 34. doi: 10.1016/S0065-2601(08)60075-1
Aust, F., Diedenhofen, B., Ullrich, S., and Musch, J. (2013). Seriousness checks are useful to improve data validity in online research. Behav. Res. Methods 45, 527–535. doi: 10.3758/s13428-012-0265-2
Bailey, J., Bailenson, J. N., Won, A. S., Flora, J., and Armel, K. C. (2012). “Presence and memory: immersive virtual reality effects on cued recall,” in Proceedings of the International Society for Presence Research Annual Conference (Philadelphia, PA), 24–26.
Banakou, D., Hanumanthu, P. D., and Slater, M. (2016). Virtual embodiment of white people in a black virtual body leads to a sustained reduction in their implicit racial bias. Front. Hum. Neurosci. 10, 601. doi: 10.3389/fnhum.2016.00601
Blascovich, J. (2002). “Social influence within immersive virtual environments,” in The Social Life of Avatars, ed R. Schroeder (London: Springer), 127–145. doi: 10.1007/978-1-4471-0277-9_8
Blascovich, J., Loomis, J., Beall, A. C., Swinth, K. R., Hoyt, C. L., and Bailenson, J. N. (2002). Immersive virtual environment technology as a methodological tool for social psychology. Psychol. Inq. 13, 146–149. doi: 10.1207/S15327965PLI1302_03
Braun, V., and Clarke, V. (2006). Using thematic analysis in psychology. Qual. Res. Psychol. 3, 77–101. doi: 10.1191/1478088706qp063oa
Brookes, J., Warburton, M., Alghadier, M., Mon-Williams, M., and Mushtaq, F. (2020). Studying human behavior with virtual reality: the unity experiment framework. Behav. Res. Methods 52(2):455–463. doi: 10.3758/s13428-019-01242-0
Buchanan, T. (2000). “Potential of the internet for personality research,” in Psychological Experiments on the Internet, ed M. H. Birnbaum (Zurich: Elsevier), 121–140. doi: 10.1016/B978-012099980-4/50006-X
Buhrmester, M., Kwang, T., and Gosling, S. D. (2016). Amazon's mechanical turk: a new source of inexpensive, yet high-quality data? Perspect. Psychol. Sci. 1, 3–5. doi: 10.1177/1745691610393980
Buhrmester, M. D., Talaifar, S., and Gosling, S. D. (2018). An evaluation of Amazon's mechanical turk, its rapid rise, and its effective use. Perspect. Psychol. Sci. 13, 149–154. doi: 10.1177/1745691617706516
Casler, K., Bickel, L., and Hackett, E. (2013). Separate but equal? A comparison of participants and data gathered via Amazon's mturk, social media, and face-to-face behavioral testing. Comput. Hum. Behav. 29, 2156–2160. doi: 10.1016/j.chb.2013.05.009
Choi, W., Li, L., Satoh, S., and Hachimura, K. (2016). Multisensory integration in the virtual hand illusion with active movement. Biomed. Res. Int. 2016, 8163098. doi: 10.1155/2016/8163098
Clark, R. E., and Craig, T. G. (1992). “Research and theory on multi-media learning effects,” in Interactive Multimedia Learning Environments, ed M. Giardina (Heidelberg: Springer; University of Southern California), 19–30. doi: 10.1007/978-3-642-77705-9_2
Connelly, L., Jia, Y., Toro, M. L., Stoykov, M. E., Kenyon, R. V., and Kamper, D. G. (2010). A pneumatic glove and immersive virtual reality environment for hand rehabilitative training after stroke. IEEE Trans. Neural Syst. Rehabil. Eng. 18, 551–559. doi: 10.1109/TNSRE.2010.2047588
Creasy, S. A., Rogers, R. J., Davis, K. K., Gibbs, B. B., Kershaw, E. E., and Jakicic, J. M. (2017). Effects of supervised and unsupervised physical activity programmes for weight loss. Obesity Sci. Pract. 3, 143–152. doi: 10.1002/osp4.107
Egan, D., Brennan, S., Barrett, J., Qiao, Y., Timmerer, C., and Murray, N. (2016). “An evaluation of heart rate and electrodermal activity as an objective qoe evaluation method for immersive virtual reality environments,” in 2016 Eighth International Conference on Quality of Multimedia Experience (QoMEX) (Lisbon: IEEE), 1–6. doi: 10.1109/QoMEX.2016.7498964
Ely, D. P., and Minor, B. B. (1994). Educational Media and Technology Yearbook, 1994, Vol. 20, ed B. B. Minor (Englewood, CO).
Freeman, D., Haselton, P., Freeman, J., Spanlang, B., Kishore, S., Albery, E., et al. (2018). Automated psychological therapy using immersive virtual reality for treatment of fear of heights: a single-blind, parallel-group, randomised controlled trial. Lancet Psychiatry 5, 625–632. doi: 10.1016/S2215-0366(18)30226-8
Goodman, J. K., Cryder, C. E., and Cheema, A. (2013). Data collection in a flat world: the strengths and weaknesses of mechanical turk samples. J. Behav. Decis. Mak. 26, 213–224. doi: 10.1002/bdm.1753
Gosling, S. D., Vazire, S., Srivastava, S., and John, O. P. (2004). Should we trust web-based studies? A comparative analysis of six preconceptions about internet questionnaires. Am. Psychol. 59, 93. doi: 10.1037/0003-066X.59.2.93
Gromala, D., Tong, X., Choo, A., Karamnejad, M., and Shaw, C. D. (2015). “The virtual meditative walk: virtual reality therapy for chronic pain management,” in Proceedings of the 33rd Annual ACM Conference on Human Factors in Computing Systems (New York, NY: Association for Computing Machinery), 521–524. doi: 10.1145/2702123.2702344
Grysman, A. (2015). Collecting narrative data on amazon's mechanical turk. Appl. Cogn. Psychol. 29, 573–583. doi: 10.1002/acp.3140
Henrich, J., Heine, S. J., and Norenzayan, A. (2010). Most people are not weird. Nature 466, 29–29. doi: 10.1038/466029a
Hertzum, M., Borlund, P., and Kristoffersen, K. B. (2015). What do thinking-aloud participants say? A comparison of moderated and unmoderated usability sessions. Int. J. Hum. Comput. Interact. 31, 557–570. doi: 10.1080/10447318.2015.1065691
Hoffman, H. G., Garcia-Palacios, A., Kapa, V., Beecher, J., and Sharar, S. R. (2003). Immersive virtual reality for reducing experimental ischemic pain. Int. J. Hum. Comput. Interact. 15, 469–486. doi: 10.1207/S15327590IJHC1503_10
Hoyt, C. L., Blascovich, J., and Swinth, K. R. (2003). Social inhibition in immersive virtual environments. Presence Teleoperat. Virtual Environ. 12, 183–195. doi: 10.1162/105474603321640932
Huber, B., and Gajos, K. Z. (2020). Conducting online virtual environment experiments with uncompensated, unsupervised samples. PLoS ONE 15, e0227629. doi: 10.1371/journal.pone.0227629
Josman, N., Somer, E., Reisberg, A., Weiss, P. L., Garcia-Palacios, A., and Hoffman, H. (2006). Busworld: designing a virtual environment for post-traumatic stress disorder in Israel: a protocol. Cyberpsychol. Behav. 9, 241–244. doi: 10.1089/cpb.2006.9.241
Kehl, R., and Van Gool, L. (2004). “Real-time pointing gesture recognition for an immersive environment,” in Sixth IEEE International Conference on Automatic Face and Gesture Recognition, 2004 (Seoul: IEEE), 577–582. doi: 10.1109/AFGR.2004.1301595
Kettunen, P., and Oksanen, J. (2018). “Effects of unsupervised participation over the internet on a usability study about map animation,” in New Directions in Geovisual Analytics: Visualization, Computation, and Evaluation (Melbourne: Lipics), 7:1–7:7.
Keusch, F. (2015). Why do people participate in web surveys? Applying survey participation theory to internet survey data collection. Manage. Rev. Q. 65, 183–216. doi: 10.1007/s11301-014-0111-y
Kilteni, K., Bergstrom, I., and Slater, M. (2013). Drumming in immersive virtual reality: the body shapes the way we play. IEEE Trans. Visual. Comput. Graph. 19, 597–605. doi: 10.1109/TVCG.2013.29
Kilteni, K., Groten, R., and Slater, M. (2012). The sense of embodiment in virtual reality. Presence Teleoperat. Virtual Environ. 21, 373–387. doi: 10.1162/PRES_a_00124
Kourtesis, P., Korre, D., Collina, S., Doumas, L. A., and MacPherson, S. E. (2020). Guidelines for the development of immersive virtual reality software for cognitive neuroscience and neuropsychology: the development of virtual reality everyday assessment lab (VR-EAL), a neuropsychological test battery in immersive virtual reality. Front. Comput. Sci. 1, 12. doi: 10.3389/fcomp.2019.00012
Krantz, J. H., and Dalal, R. (2000). “Validity of web-based psychological research,” in Psychological Experiments on the Internet, ed M. H. Birnbaum (Zurich: Elsevier), 35–60. doi: 10.1016/B978-012099980-4/50003-4
Lacroix, A., Kressig, R., Muehlbauer, T., Gschwind, Y., Pfenninger, B., Bruegger, O., et al. (2015). Effects of a supervised versus an unsupervised combined balance and strength training program on balance and muscle power in healthy older adults: a randomized controlled trial. Gerontology 62, 275–288. doi: 10.1159/000442087
Ludlow, B. L. (2015). Virtual reality: Emerging applications and future directions. Rural Spcl. Educ. Q. 34, 3–10. doi: 10.1177/875687051503400302
Ma, X., Cackett, M., Park, L., Chien, E., and Naaman, M. (2018). “Web-based VR experiments powered by the crowd,” in Proceedings of the 2018 World Wide Web Conference (Geneva), 33–43. doi: 10.1145/3178876.3186034
Makransky, G., Terkildsen, T. S., and Mayer, R. E. (2019). Adding immersive virtual reality to a science lab simulation causes more presence but less learning. Learn. Instruct. 60, 225–236. doi: 10.1016/j.learninstruc.2017.12.007
Mason, W., and Suri, S. (2012). Conducting behavioral research on Amazon's mechanical turk. Behav. Res. Methods 44, 1–23. doi: 10.3758/s13428-011-0124-6
Moon, Y. (1998). “The effects of distance in local versus remote human-computer interaction,” in Proceedings of the SIGCHI Conference on Human factors in Computing Systems (Los Angeles, CA: ACM Press/Addison-Wesley Publishing Co), 103–108. doi: 10.1145/274644.274660
Mori, M., MacDorman, K. F., and Kageki, N. (2012). The uncanny valley [from the field]. IEEE Robot. Automat. Mag. 19, 98–100. doi: 10.1109/MRA.2012.2192811
Moss, J. D., and Muth, E. R. (2011). Characteristics of head-mounted displays and their effects on simulator sickness. Hum. Factors 53(3):308–319. doi: 10.1177/0018720811405196
Mottelson, A., and Hornbæk, K. (2017). “Virtual reality studies outside the laboratory,” in Proceedings of the 23rd ACM Symposium on Virtual Reality Software and Technology (New York, NY: Association for Computing Machinery), 1–10. doi: 10.1145/3139131.3139141
Pan, X., and Hamilton, A. F. C. (2018). Why and how to use virtual reality to study human social interaction: the challenges of exploring a new research landscape. Brit. J. Psychol. 109, 395–417. doi: 10.1111/bjop.12290
Paolacci, G., and Chandler, J. (2014). Inside the turk: understanding mechanical turk as a participant pool. Curr. Direct. Psychol. Sci. 23, 184–188. doi: 10.1177/0963721414531598
Paolacci, G., Chandler, J., and Ipeirotis, P. G. (2010). Running experiments on Amazon Mechanical Turk. Judgment and Decision Making, Vol. 5, No. 5, 411–419, Available online at: https://ssrn.com/abstract=1626226
Peer, E., Brandimarte, L., Samat, S., and Acquisti, A. (2017). Beyond the turk: alternative platforms for crowdsourcing behavioral research. J. Exp. Soc. Psychol. 70, 153–163. doi: 10.1016/j.jesp.2017.01.006
Peer, E., Vosgerau, J., and Acquisti, A. (2014). Reputation as a sufficient condition for data quality on amazon mechanical turk. Behav. Res. Methods 46, 1023–1031. doi: 10.3758/s13428-013-0434-y
Piumsomboon, T., Lee, G., Lindeman, R. W., and Billinghurst, M. (2017). “Exploring natural eye-gaze-based interaction for immersive virtual reality,” in 2017 IEEE Symposium on 3D User Interfaces (3DUI) (Los Angeles, CA: IEEE), 36–39. doi: 10.1109/3DUI.2017.7893315
Preece, J. (2016). Citizen science: new research challenges for human-computer interaction. Int. J. Hum. Comput. Interact. 32, 585–612. doi: 10.1080/10447318.2016.1194153
Putze, S., Alexandrovsky, D., Putze, F., Höffner, S., Smeddinck, J. D., and Malaka, R. (2020). “Breaking the experience: effects of questionnaires in VR user studies,” in Proceedings of the 2020 CHI Conference on Human Factors in Computing Systems (Honolulu, HI), 1–15. doi: 10.1145/3313831.3376144
Radianti, J., Majchrzak, T. A., Fromm, J., and Wohlgenannt, I. (2020). A systematic review of immersive virtual reality applications for higher education: design elements, lessons learned, and research agenda. Comput. Educ. 147, 103778. doi: 10.1016/j.compedu.2019.103778
Reips, U.-D. (2000). “The web experiment method: advantages, disadvantages, and solutions,” in Psychological Experiments on the Internet. ed M. H. Birnbaum (Zurich: Elsevier), 89–117. doi: 10.1016/B978-012099980-4/50005-8
Rogers, Y., and Marshall, P. (2017). “Research in the wild,” in Synthesis Lectures on Human-Centered Informatics, ed J. M. Carroll (Cham: Springer), i-97. doi: 10.1007/978-3-031-02220-3
Ryan, R. S., Wilde, M., and Crist, S. (2013). Compared to a small, supervised lab experiment, a large, unsupervised web-based experiment on a previously unknown effect has benefits that outweigh its potential costs. Comput. Hum. Behav. 29, 1295–1301. doi: 10.1016/j.chb.2013.01.024
Saffo, D., Yildirim, C., Di Bartolomeo, S., and Dunne, C. (2020). “Crowdsourcing virtual reality experiments using VRchat,” in Extended Abstracts of the 2020 CHI Conference on Human Factors in Computing Systems (New York, NY: Association for Computing Machinery), 1–8. doi: 10.1145/3334480.3382829
Sanz, F. A., Olivier, A.-H., Bruder, G., Pettré, J., and Lécuyer, A. (2015). “Virtual proxemics: locomotion in the presence of obstacles in large immersive projection environments,” in 2015 IEEE Virtual Reality (VR) (Arles: IEEE), 75–80. doi: 10.1109/VR.2015.7223327
Schwind, V., Knierim, P., Haas, N., and Henze, N. (2019). “Using presence questionnaires in virtual reality,” in Proceedings of the 2019 CHI Conference on Human Factors in Computing Systems (New York, NY: Association for Computing Machinery), 1–12. doi: 10.1145/3290605.3300590
Segovia, K. Y., and Bailenson, J. N. (2009). Virtually true: children's acquisition of false memories in virtual reality. Media Psychol. 12, 371–393. doi: 10.1080/15213260903287267
Senior, C., Phillips, M. L., Barnes, J., and David, A. (1999). An investigation into the perception of dominance from schematic faces: a study using the world-wide web. Behav. Res. Methods Instrum. Comput. 31, 341–346. doi: 10.3758/BF03207730
Soave, F., Bryan-Kinns, N., and Farkhatdinov, I. (2020). “A preliminary study on full-body haptic stimulation on modulating self-motion perception in virtual reality,” in Augmented Reality, Virtual Reality, and Computer Graphics, eds L. T. De Paolis and P. Bourdot (Cham: Springer International Publishing), 461–469. doi: 10.1007/978-3-030-58465-8_34
Sprouse, J. (2011). A validation of amazon mechanical turk for the collection of acceptability judgments in linguistic theory. Behav. Res. Methods 43, 155–167. doi: 10.3758/s13428-010-0039-7
Steed, A., Frlston, S., Lopez, M. M., Drummond, J., Pan, Y., and Swapp, D. (2016). An “in the wild” experiment on presence and embodiment using consumer virtual reality equipment. IEEE Trans. Visual. Comput. Graph. 22, 1406–1414. doi: 10.1109/TVCG.2016.2518135
Steed, A., Ortega, F., Williams, A., Kruijff, E., Stuerzlinger, W., Batmaz, A. U., et al. (2020). Evaluating immersive experiences during Covid-19 and beyond. Interactions. 27, 4. doi: 10.1145/3406098
Steed, A., Pan, Y., Zisch, F., and Steptoe, W. (2016). “The impact of a self-avatar on cognitive load in immersive virtual reality,” in 2016 IEEE Virtual Reality (VR) (Greenville, SC: IEEE), 67–76. doi: 10.1109/VR.2016.7504689
Stern, S. E., and Faber, J. E. (1997). The lost e-mail method: milgram's lost-letter technique in the age of the internet. Behav. Res. Methods Instrum. Comput. 29, 260–263. doi: 10.3758/BF03204823
Suh, A., and Prophet, J. (2018). The state of immersive technology research: a literature analysis. Comput. Hum. Behav. 86, 77–90. doi: 10.1016/j.chb.2018.04.019
Sweller, J. (2010). Cognitive load theory: recent theoretical advances. Cogn. Load Theory Recent Theoret. Adv. 1, 29–47. doi: 10.1017/CBO9780511844744.004
Tullis, T., Fleischman, S., McNulty, M., Cianchette, C., and Bergel, M. (2002). “An empirical comparison of lab and remote usability testing of web sites,” in Usability Professionals Association Conference (Orlando, FL: Usability Professionals Association Conference).
Waller, D., Bachmann, E., Hodgson, E., and Beall, A. C. (2007). The hive: a huge immersive virtual environment for research in spatial cognition. Behav. Res. Methods 39, 835–843. doi: 10.3758/BF03192976
Warriar, V. R., Woodward, J. R., and Tokarchuk, L. (2019). “Modelling player preferences in AR mobile games,” in 2019 IEEE Conference on Games (CoG) (London: IEEE), 1–8. doi: 10.1109/CIG.2019.8848082
Weibel, R. P., Grübel, J., Zhao, H., Thrash, T., Meloni, D., Hölscher, C., et al. (2018). Virtual reality experiments with physiological measures. J. Visual. Exp. 1, e58318. doi: 10.3791/58318
Wilson, C. J., and Soranzo, A. (2015). The use of virtual reality in psychology: a case study in visual perception. Comput. Math. Methods Med. 2015, 151702. doi: 10.1155/2015/151702
Yuan, Y., and Steed, A. (2010). “Is the rubber hand illusion induced by immersive virtual reality?” in 2010 IEEE Virtual Reality Conference (VR) (Waltham, MA: IEEE), 95–102. doi: 10.1109/VR.2010.5444807
Keywords: extended reality, virtual reality, augmented reality, remote experiments, encapsulated studies, literature review, expert interviews, case study
Citation: Ratcliffe J and Tokarchuk L (2022) The potential of remote XR experimentation: Defining benefits and limitations through expert survey and case study. Front. Comput. Sci. 4:952996. doi: 10.3389/fcomp.2022.952996
Received: 25 May 2022; Accepted: 17 October 2022;
Published: 02 December 2022.
Edited by:
Anton Nijholt, University of Twente, NetherlandsReviewed by:
Farhan Mohamed, University of Technology Malaysia, MalaysiaKaļinka Māris, Riga Technical University, Latvia
Copyright © 2022 Ratcliffe and Tokarchuk. This is an open-access article distributed under the terms of the Creative Commons Attribution License (CC BY). The use, distribution or reproduction in other forums is permitted, provided the original author(s) and the copyright owner(s) are credited and that the original publication in this journal is cited, in accordance with accepted academic practice. No use, distribution or reproduction is permitted which does not comply with these terms.
*Correspondence: Jack Ratcliffe, j.ratcliffe@qmul.ac.uk