- 1Human Machine Teaming, TNO, Soesterberg, Netherlands
- 2Human-Media Interaction, University of Twente, Enschede, Netherlands
Implicit physiological measures such as heart rate and skin conductance convey information about someone's cognitive or affective state. Currently, gastrointestinal (GI) tract-based markers are not yet considered while both the organs involved as well as the microbiota populating the GI tract are bidirectionally connected to the brain and have a relation to emotion, cognition and behavior. This makes GI tract-based measures relevant and interesting, especially because the relation may be causal, and because they have a different timescale than current physiological measures. This perspective paper (1) presents the (mechanistic) involvement of the GI tract and its microbiota in emotion, cognition and behavior; (2) explores the added value of microbiome-based implicit measures as complementary to existing measures; and (3) sets the priorities to move forward. Five potential measures are proposed and discussed in more detail: bowel movement, short-chain fatty acids, tyrosine and tryptophan, GI tract flora composition, and cytokine levels. We conclude (1) that the involvement of the GI tract in emotion, cognition and behavior is undisputed, (2) that GI tract-based implicit measures are still in a conceptual phase of development but show potential and (3) that the first step to bring this field forward is to start validation studies in healthy humans and that are designed in the context of implicit measurements.
Introduction
Implicit measures can provide information about an individual's cognitive or affective state and are used in fields like affective computing, brain-computer interfaces, and neuro-ergonomics (Van Erp et al., 2012; Toet et al., 2022). Implicit physiological measures are based on for instance brain activity, heart rate, and skin conductance (Brouwer et al., 2015) and application areas include learning (Gerjets et al., 2014; Stikic et al., 2014), adaptive interfaces (Dijksterhuis et al., 2013; Touryan et al., 2014; Wang et al., 2014), and assessing food experience and food choices (Kaneko et al., 2018).
So far, there are no gastrointestinal tract (GI tract) related implicit measures used in these and related domains. There are, however, reasons to explore them because the significance of the GI tract organs and their microbiota goes beyond digesting food. For instance, Komanduri et al. (2022) showed a relationship between specific families / genera of bacteria and different cognitive functions in healthy elderly, for instance between Carnobacteriaceae and episodic memory, Alcaligenaceae and Clostridiaceae and working memory, and Bacteroidaceae, Barnesiellaceae, Rikenellaceae and Gemellaceae and concentration. Ren et al. (2020) did so in elderly with Parkinson's disease and found a lower presence of Blautia and Ruminococcus for individuals with mild cognitive impairment compared to those with normal cognition. Research indicates that the microbiome (here used to refer to the collection of genomes and metabolites of the microbiota) may even have a causal relation to emotional, cognitive and behavioral processes (Dinan et al., 2015). Hence the recent interest in psychobiotics: live microorganisms intended to provide mental health benefits when consumed (Sharma et al., 2021).
This perspective therefore explores the potential of GI tract-based implicit measures with the following goals: (a) to give an overview of the (mechanistic) involvement of the GI tract and its microbiota in emotion, cognition and behavior; (b) to explore the potential added value of GI tract-based implicit measures as complementary to existing measures; (c) to identify the way forward to develop GI tract-based implicit measures.
Microbiota-gut-brain axis
Humans are colonized by many microorganisms. Estimates go up to 100 trillion bacterial cells, which is at least as much as human cells (Sender et al., 2016). Microorganisms are present in many external and internal parts of the human body, but both the absolute number and the number of species of microbiota in the GI tract is larger than at other body loci (Dinan and Cryan, 2015). Healthy GI tract microbiota are important to maintain homeostasis and aid metabolism, inhibit pathogens, support the developing immune system, and influence emotion, cognition and behavior through the so-called microbiota-gut-brain axis. This axis includes the GI tract flora and their metabolites, the central nervous system, neuro-endocrine and neuro-immune systems including enteric endocrine cells and the hypothalamic–pituitary–adrenal axis (HPA axis), sympathetic and parasympathetic arms of the autonomic nervous system including the enteric nervous system, and the vagus nerve.
The word axis in the microbiota-gut-brain axis refers to the fact that several organs signal each other in a coherent manner. Four pathways between the GI tract and the brain are relevant for this perspective, see Figure 1: (1) efferent and afferent nerves; (2) metabolites that have a neuro-active effect; (3) neuro-endocrine signaling, and (4) neuro-immune and inflammatory reactions.
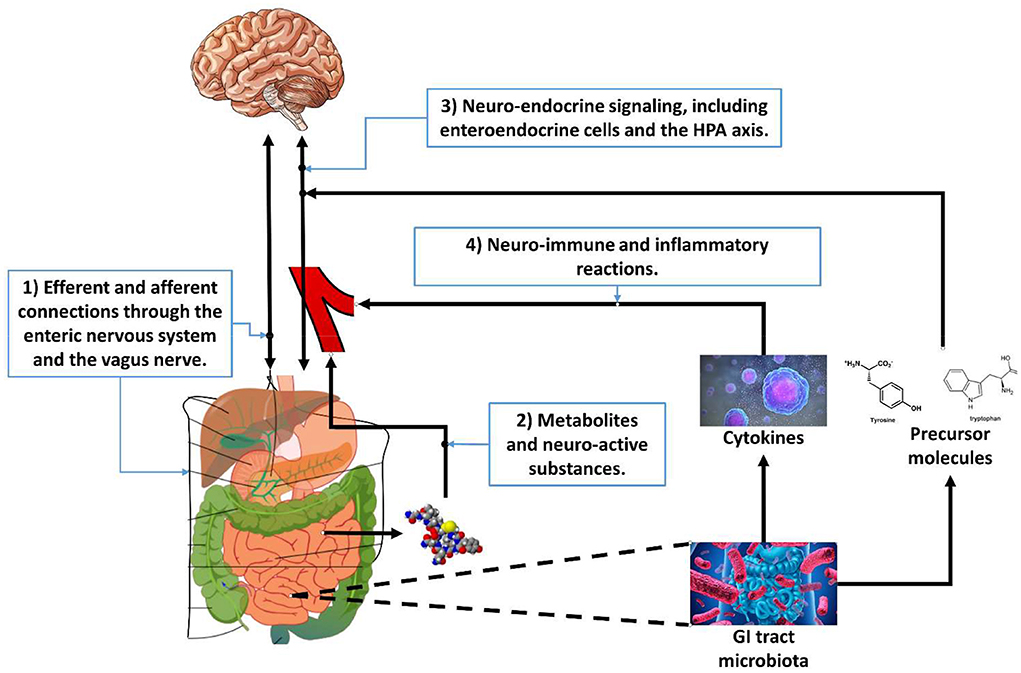
Figure 1. The four pathways of the microbiota-gut-brain axis relevant for gastrointestinal tract-based implicit measures.
Efferent and afferent connections
The first pathway of the microbiota-gut-brain axis is through the efferent and afferent connections of the enteric nervous system. The enteric nervous system is part of the autonomic nervous system and contains about 500 million neurons embedded in the GI tract (see e.g., Burns and Thapar, 2006 for a review). Its primary role is to govern the function of the GI tract but is sometimes nicknamed “the second brain” (Gershon, 1999). It can operate autonomously but is connected to the central nervous system through the vagus nerve (parasympathetic) and the prevertebral ganglia (sympathetic). It includes efferent neurons, afferent neurons, and interneurons, which makes it capable to integrate signals.
GI tract neuro-active substances
The second pathway of the microbiota-gut-brain axis is through neuro-active metabolites (Yarandi et al., 2016). Metabolites are intermediate products of metabolism. Metabolites have various functions, including signaling. The GI tract flora can produce a range of neuro-active molecules, and many of the neurotransmitters in the enteric system are identical to those in the central nervous system such as acetylcholine, catecholamines, γ-aminobutyric acid, dopamine, and serotonin. The GI tract contains more than 90% of the body's serotonin and about 50% of the body's dopamine (Berger et al., 2009). The GI tract flora also release molecules that can directly activate the vagus nerve (Torres-Fuentes et al., 2017).
Neuro-endocrine signaling
The third pathway of the microbiota-gut-brain axis is through neuro-endocrine signaling. The endocrine system is comprised of feedback loops of hormones released by endocrine glands (Hiller-Sturmhöfel and Bartke, 1998). Hormones also regulate physiological processes and behavioral activities, such as digestion, metabolism, respiration, sensory perception, sleep, excretion, lactation, stress, growth and development, movement, reproduction, and mood.
Enteric endocrine cells in the GI tract form the enteric endocrine system. This is a branch of the endocrine system, comparable to the enteric nervous system as branch of the nervous system. The enteric endocrine cells produce numerous hormones (Schonhoff et al., 2004) with an important systemic effect that can also activate the enteric nervous system, making it the largest endocrine organ in the body (Ahlman and Nilsson, 2001). However, the molecules produced by the enteric endocrine cells may act as a hormone, as well as as a neurotransmitter. This implies that their function goes beyond that of a local regulator of the digestive tract (see Rehfeld, 1998 for a review). For instance, cholecystokinin stimulates the digestion of fat and protein, but also plays important role in processes related to satiety and anxiety in the central nervous system. Glucagon-like peptide-1 and−2 are produced and secreted upon food consumption, but are also linked to neurotrophic effects, neuroprotective effects, and (through the brainstem and hypothalamus) induction of satiety and thereby reduction of food and water intake. Finally, bile acids allow digestion of dietary fats and oils, but also have hormonal actions throughout the body.
In addition to (human) enteric endocrine cells in the intestine, GI tract microorganisms are involved in neuro-endocrine signaling because they ferment dietary fibers into short-chain fatty acids (SCFAs) and other compounds like (precursors of) hormones. Free short-chain fatty acids can cross the blood-brain barrier via monocarboxylate transporters. As shown by Byrne et al. (2015), GI tract flora influence for instance appetite regulation, energy homeostasis, and insulin sensitivity (Canfora et al., 2015). Because of these and other effects, some consider the gut flora as an endocrine organ itself that is part of the endocrine system and its feedback loops. For instance, chronic or acute stressors activate the HPA axis, causing changes in the GI tract flora, resulting in systemic effects (Petra et al., 2015).
In addition to short-chain fatty acids, GI tract flora produce precursors of hormones of which tyrosine (the precursor of L-DOPA, dopamine, norepinephrine, and finally epinephrine), and tryptophan (the precursor of serotonin) are the most relevant for this perspective. Dopamine is a hormone and a neurotransmitter and the brain has several dopaminergic pathways, amongst others involved in reward and reward-motivated behavior (Puglisi-Allegra and Ventura, 2012) and in motor control. Norepinephrine (or noradrenaline) also functions both as a hormone and as a neurotransmitter and prepares the body for action in situations of stress or danger. Increased levels of norepinephrine result in increased heart rate, blood pressure, and blood flow to the muscles, and in the release of glucose. Its effect in the brain is to increase arousal and alertness, promote vigilance, enhance memory, and focus attention. Finally, epinephrine also plays an important role in the fight-or-flight response and the release of adrenaline in response to stress.
Tryptophan is another relevant precursor and the human body cannot synthesize it itself and thus depends on GI tract flora to produce it (Yano et al., 2015). Tryptophan is a precursor of serotonin, and approximately 90% of the human body's total serotonin is located in the cells in the GI tract. Serotonin is involved in many physiological processes (Berger et al., 2009) and modulates amongst others cognition, reward, learning and memory (Jenkins et al., 2016).
Neuro-immune and inflammatory reactions
The forth pathway of the microbiota-gut-brain axis is through neuro-immune and inflammatory reactions. Research (Quigley, 2013; Shen and Wong, 2016) has shown that the composition of the gut microbiota relates to inflammatory and autoimmune conditions in for instance asthma, obesity and diabetes. Changes in the composition of the GI tract flora due to diet, drugs, antibiotics, or disease correlate with changes in levels of circulating cytokines, like interleukin 6 (IL-6) and tumor necrosis factor alpha (TNF-alpha), some of which can affect brain function (see Marsland et al., 2017 for a meta-analysis of the relation between acute stress and inflammatory markers). IL-6 plays an important role in promoting inflammatory responses in the gut and can affect the intestinal epithelial barrier and result in an increased permeability (Al-Sadi et al., 2014), informally known as “leaky gut.” In a meta-analysis, Dowlati et al. (2010) show that significantly higher concentrations of IL-6 and TNF-alpha are present in depressed participants compared with controls. In turn, IL-6 and TNF-alpha can affect the hypothalamus that is involved in learning and memory and the HPA axis that is involved in amongst others stress responses.
Classes of potential measures
The microbiota-gut-brain axis can influence emotion, cognition, and behavior. Based on the four pathways described in the previous sections we can derive a preliminary selection of classes of implicit measures.
Efferent and afferent connections
In this class of measures, one can think of measures that reflect enteric nervous system activity like gastric myoelectrical activity that paces the contraction of the stomach. Vianna and Tranel (2006) studied gastric myoelectrical activity and found that peak amplitudes in the Electrogastrogram were different when participants watched emotional film clips compared to neutral clips, and Muth et al. (1999) report the relation between emotional responses and gastric myoelectrical activity in stressful tasks. Measuring bowel movement does not involve the analysis of specific substances or molecules. The use of sensors attached to the skin of the abdomen (Mladenović, 2018) or integrated into textile may already provide the required data that may also be processed in real time by for instance a smart watch or another wearable computing device.
In addition, because the connection to the brain is via the vagus nerve, measures based on vagal nerve activity could be relevant. However, vagus nerve activity is also linked to for instance the lungs and heart and thus to existing measures such as respiration rate, skin conductance, heart rate and heart rate variability (Brouwer et al., 2015). Therefore, there is probably little –if any– added value of exploring additional GI tract-based measures.
GI tract flora, neuro-active substances and neuro-endocrine signaling
One can think of several classes of implicit measures considering neuro-active substances and neuro-endocrine signaling: measures reflecting the composition of the GI tract flora, measures reflecting neuro-active metabolites produced by the GI tract microbiota like short-chain fatty acids and precursors of hormones like tyrosine and tryptophan, and measures reflecting the (human) enteric endocrine system.
GI tract flora can potentially be measured in a feasible and non-invasive way using fecal samples. However, fecal samples are only a proxy (Tang et al., 2020) and there are no universally accepted protocols for collection and microbial DNA extraction (Thomas et al., 2015). Nevertheless, new approaches are being developed that may allow quick and cheap analyses in the near future, e.g., based on images of fecal samples (Furusawa et al., 2021).
Regarding metabolites, short-chain fatty acids may be interesting since they can cross the blood-brain barrier and have neuro-active properties. In addition, tyrosine and tryptophan may be relevant as they are precursors of important substances that modulate emotion, cognition and behavior. Tryptophan is a so-called essential amino acid, which means that the human body cannot synthesize it and it is thus exclusively linked to GI tract flora activity. Tyrosine and tryptophan can be measured in blood, in tissue, en (non-invasively) in gastric content and urine (Wiggins et al., 2015).
Regarding measures that reflect the enteric endocrine system, we should keep in mind that the enteric endocrine system is part of the complete endocrine system. There are currently several hormones that are already used as proven implicit measures like cortisol. This raises the question if there are additional, unique hormones that can be exclusively linked to the GI tract and that have additional value over the current hormone-based implicit measures.
Neuro-immune and inflammatory processes
Measures based on the levels of specific cytokines in blood are potentially relevant. However, the invasive way needed to obtain blood samples makes the application for healthy users more complex or even undesirable. An alternative is to measure salivary cytokines (e.g., Riis et al., 2014; La Fratta et al., 2018) although they may be less stable and include locally produced (oral) cytokines.
Discussion
The GI tract and its microbiota are bidirectionally connected to the brain and peripheral physiological processes through multiple pathways. The brain influences the microbiota and the microbiota and the substances they produce have an effect on emotion, cognition and behavior. Despite the relation between the GI tract and amongst others anxiety, social interaction, learning and memory, appetite and satiety, emotional behavior, psychological stress, and mood, GI tract-based implicit measures are not yet considered in affective computing, neuro-ergonomics, and other computer science and human factors domains. Additional reasons to explore them are the fact that the relation of GI tract flora to emotion, cognition and behavior can be causal. Also, GI tract-based measures can be complementary to existing physiological measures, for instance because they have a different timescale (hours or days) than physiological measures like heart rate and skin conductance (seconds or minutes).
Based on the different pathways between the GI tract and the brain, the previous sections presented five potential measures: bowel movement, short-chain fatty acids, tyrosine and tryptophan, GI tract flora composition, and cytokine levels. Although there is research available, none of it is done in the context of implicit measures. In this phase, we can only discuss the feasibility of the different measures in general terms and using common sense. To move forward, the following topics are relevant.
Complications of GI tract-based implicit measures
Like physiological implicit measures, analyzing GI tract-based data will face several complications. Three factors that can be expected to have a major effect are individual differences, non-stationarities due to for instance circadian rhythm and seasons effects [e.g., see Haus (2007) for a review], and changes in the composition of GI tract microbiota caused by factors that may not be relevant for implicit measurements, like diet changes, drugs, and antibiotics. This makes the data more difficult to model because the estimate of the mean will be changing. The good thing is that data-analysis techniques developed for physiological measures (e.g., AlZoubi et al., 2020) may also be applicable for GI tract-based measures although this will require specific adjustments.
Validation and lack of human studies
Most of the work on the role of GI tract flora in the microbiota-gut–brain axis is done in animals. Studies with humans are often with small sample sizes and in relation to disease, and have limited generalizability to healthy individuals. Extended validation of GI tract-based measures and their relation to the constructs of interest in healthy humans is of key importance, as is assessing their sensitivity and specificity. As with other implicit measures, one should carefully define the state of interest, the biological processes that are expected to be involved in that state, and the added value of GI tract-based measures compared with other measures and in the context of an application (see Brouwer et al., 2015 for a full list of recommendations).
Combining implicit measures and prospective applications
GI tract-based implicit measures are still conceptual and have not gone through numerous breakthroughs and replications let alone empiricism, the phase in which the first benefits of this new measures may become available. Compared to other (physiological) implicit measures, GI tract-based measures will have a low sampling rate and will reflect slower processes. This makes them less suited for applications like adaptive interfaces. However, this also means that they are complementary to existing measures. An example would be the physiological responses to emotional stimuli. Current implicit measures like heart rate operate in the range of seconds and are vulnerable to physical activity caused by metabolic need (Brouwer et al., 2018). With a main frequency of about 3 Hz, gastric myoelectrical activity operates in the range of minutes and will be less affected by the muscles' energy demand caused by physical activity. Combining heart rate and gastric myoelectrical activity, may reflect both the immediate and the sustained response to emotional stimuli.
According to Kaneko et al. (2018), instruments that tap into the lower levels of emotional and cognitive processes are a valuable addition to the current set of instruments, at least in the food domain. Other prospective applications include monitoring of alertness, mental state and fitness, emotion regulation over time, and recovery after adverse events. The added value would increase further for measures based on processes that modulate the state of interest in a causal way. An example would be the relation between lifestyle, gut permeability, pro-inflammatory cytokines, and cognitive performance.
Conclusion
GI tract-based implicit measures are still in a conceptual phase of development but have potential and the development of easier and cheaper non-invasive tools to for instance analyze bowel movement or metabolites and microbiota in saliva, urine, or fecal samples can push this field forward in the foreseeable future. The first aim was to explore the (mechanistic) involvement of the GI tract and its microbiota in emotion, cognition and behavior. We can conclude that this involvement is undisputed, and the knowledge base is extending. However, data in the context of implicit measures are still lacking and the role of many microorganisms and the substances they produce is still not well-understood, especially in healthy humans. The second aim was to explore the added value of GI-tract microbiota-based implicit measures as complementary to existing measures. We can conclude that this benefit may be in the potential causal relation, and in the different time scale: in the order of hours or days rather than seconds or minutes. Our third aim was to set the priorities to move forward. We consider validation studies with healthy participants and designed in the context of implicit measurements as the first priority.
In many domains, cognitive or emotional states are relevant for comfort, task performance, predicting future behavior, etc. For instance, pilots need to be fit for duty for safe operations and wanting and liking can be important predictors of future buying behavior of consumers. This perspective provides the scientific basis for the recommendation to develop GI tract-based implicit measures for cognition, emotion and behavior.
Author contributions
The author confirms being the sole contributor of this work and has approved it for publication.
Conflict of interest
The author declares that the research was conducted in the absence of any commercial or financial relationships that could be construed as a potential conflict of interest.
Publisher's note
All claims expressed in this article are solely those of the authors and do not necessarily represent those of their affiliated organizations, or those of the publisher, the editors and the reviewers. Any product that may be evaluated in this article, or claim that may be made by its manufacturer, is not guaranteed or endorsed by the publisher.
References
Ahlman, H., and Nilsson, O. (2001). The gut as the largest endocrine organ in the body. Ann. Oncol. 12 Suppl 2, S63–S68. doi: 10.1093/annonc/12.suppl_2.S63
Al-Sadi, R., Ye, D., Boivin, M., Guo, S., Hashimi, M., Ereifej, L., et al. (2014). Interleukin-6 modulation of intestinal epithelial tight junction permeability is mediated by JNK pathway activation of claudin-2 gene. PLoS One 9, e85345. doi: 10.1371/journal.pone.0085345
AlZoubi, O., AlMakhadmeh, B., Tawalbeh, S. K., Yassien, M. B., and Hmeidi, I. (2020). “A deep learning approach for classifying emotions from physiological data.,” in: 11th International Conference on Information and Communication Systems (ICICS) (New York, NY: IEEE), 214–219.
Berger, M., Gray, J. A., and Roth, B. L. (2009). The expanded biology of serotonin. Ann. Rev. Med. 60, 355–366. doi: 10.1146/annurev.med.60.042307.110802
Brouwer, A.-M., Van Dam, E., Van Erp, J. B. F., Spangler, D. P., and Brooks, J. R. (2018). Improving real-life estimates of emotion based on heart rate: a perspective on taking metabolic heart rate into account. Front. Human Neurosci. 12, 284. doi: 10.3389/fnhum.2018.00284
Brouwer, A.-M., Zander, T. O., Van Erp, J. B. F., Korteling, J. E., and Bronkhorst, A. W. (2015). Using neurophysiological signals that reflect cognitive or affective state: Six recommendations to avoid common pitfalls. Front. Neurosci. 9, 00136. doi: 10.3389/fnins.2015.00136
Burns, A. J., and Thapar, N. (2006). Advances in ontogeny of the enteric nervous system. Neurogastroenterol. Motil. 18, 876–887. doi: 10.1111/j.1365-2982.2006.00806.x
Byrne, C. S., Chambers, E. S., Morrison, D. J., and Frost, G. (2015). The role of short chain fatty acids in appetite regulation and energy homeostasis. Int. J. Obesity (2005) 39, 1331–1338. doi: 10.1038/ijo.2015.84
Canfora, E. E., Jocken, J. W., and Blaak, E. E. (2015). Short-chain fatty acids in control of body weight and insulin sensitivity. Nat. Rev. Endocrinol. 11, 577–591. doi: 10.1038/nrendo.2015.128
Dijksterhuis, C., De Waard, D., Brookhuis, K., Mulder, B., and De Jong, R. (2013). Classifying visuomotor workload in a driving simulator using subject specific spatial brain patterns. Front. Neurosci. 7, 149. doi: 10.3389/fnins.2013.00149
Dinan, T., and Cryan, G. (2015). The impact of gut microbiota on brain and behavior: implications for psychiatry. Curr. Opin. Clin. Nutr. Metab. Care. 18, 552–558. doi: 10.1097/MCO.0000000000000221
Dinan, T. G., Stilling, R. M., Stanton, C., and Cryan, J. F. (2015). Collective unconscious: how gut microbes shape human behavior. J. Psychiatric Res. 63, 1–9. doi: 10.1016/j.jpsychires.2015.02.021
Dowlati, Y., Herrmann, N., Swardfager, W., Liu, H., Sham, L., Reim, E. K., et al. (2010). A meta-analysis of cytokines in major depression. Biol. Psychiatry 67, 446–457. doi: 10.1016/j.biopsych.2009.09.033
Furusawa, C., Tanabe, K., Ishii, C., Kagata, N., Tomita, M., and Fukuda, S. (2021). Decoding gut microbiota by imaging analysis of fecal samples. iScience 24, 103481. doi: 10.1016/j.isci.2021.103481
Gerjets, P., Walter, C., Rosenstiel, W., Bogdan, M., and Zander, T. O. (2014). Cognitive state monitoring and the design of adaptive instruction in digital environments: lessons learned from cognitive workload assessment using a passive brain-computer interface approach. Front. Neurosci. 8, 385. doi: 10.3389/fnins.2014.00385
Gershon, M. D. (1999). The Second Brain: A Groundbreaking New Understanding of Nervous Disorders of the Stomach and Intestine, Harper Perennial. New York, NY: HarperCollins Publishers.
Haus, E. (2007). Chronobiology in the endocrine system. Adv. Drug Deliv. Rev. 59, 985–1014. doi: 10.1016/j.addr.2007.01.001
Hiller-Sturmhöfel, S., and Bartke, A. (1998). The endocrine system: an overview. Alcohol Health Res. World 22, 153–164.
Jenkins, T. A., Nguyen, J. C., Polglaze, K. E., and Bertrand, P. P. (2016). Influence of tryptophan and serotonin on mood and cognition with a possible role of the gut-brain axis. Nutrients 8, 56. doi: 10.3390/nu8010056
Kaneko, D., Toet, A., Brouwer, A.-M., Kallen, V., and Van Erp, J. B. F. (2018). Methods for evaluating emotions evoked by food experiences: a literature review. Front. Psychol. 9, 911. doi: 10.3389/fpsyg.2018.00911
Komanduri, M., Savage, K., Lea, A., McPhee, G., Nolidin, K., Deleuil, S., et al. (2022). The relationship between gut microbiome and cognition in older Australians. Nutrients 14, 64. doi: 10.3390/nu14010064
La Fratta, I., Tatangelo, R., Campagna, G., Rizzuto, A., Franceschelli, S., Ferrone, A., et al. (2018). The plasmatic and salivary levels of IL-1β, IL-18 and IL-6 are associated to emotional difference during stress in young male. Sci Rep 8, 3031. doi: 10.1038/s41598-018-21474-y
Marsland, A. L., Walsh, C., Lockwood, K., and John-Henderson, N. A. (2017). The effects of acute psychological stress on circulating and stimulated inflammatory markers: a systematic review and meta-analysis. Brain Behav. Immun. 64, 208–219. doi: 10.1016/j.bbi.2017.01.011
Mladenović, J. (2018). Considering Gut Biofeedback for Emotion Regulation Proceedings of the 2018 ACM International Joint Conference and 2018 International Symposium on Pervasive and Ubiquitous Computing and Wearable Computers, Singapore. New York, NY: Association for Computing Machinery.
Muth, E. R., Koch, K. L., Stern, R. M., and Thayer, J. F. (1999). Effect of autonomic nervous system manipulations on gastric myoelectrical activity and emotional responses in healthy human subjects. Psychosom. Med. 61, 297–303. doi: 10.1097/00006842-199905000-00008
Petra, A. I., Panagiotidou, S., Hatziagelaki, E., Stewart, J. M., Conti, P., and Theoharides, T. C. (2015). Gut-microbiota-brain axis and its effect on neuropsychiatric disorders with suspected immune dysregulation. Clin. Ther. 37, 984–995. doi: 10.1016/j.clinthera.2015.04.002
Puglisi-Allegra, S., and Ventura, R. (2012). Prefrontal/accumbal catecholamine system processes high motivational salience. Front. Behav. Neurosci. 6, 31. doi: 10.3389/fnbeh.2012.00031
Rehfeld, J. F. (1998). The new biology of gastrointestinal hormones. Physiol. Rev. 78, 1087–1108. doi: 10.1152/physrev.1998.78.4.1087
Ren, T., Gao, Y., Qiu, Y., Jiang, S., Zhang, Q., Zhang, J., et al. (2020). Gut microbiota altered in mild cognitive impairment compared with normal cognition in sporadic Parkinson's disease. Front. Neurol. 11, 137. doi: 10.3389/fneur.2020.00137
Riis, J. L., Out, D., Dorn, L. D., Beal, S. J., Denson, L. A., Pabst, S., et al. (2014). Salivary cytokines in healthy adolescent girls: intercorrelations, stability, and associations with serum cytokines, age, and pubertal stage. Dev. Psychobiol. 56, 797–811. doi: 10.1002/dev.21149
Schonhoff, S. E., Giel-Moloney, M., and Leiter, A. B. (2004). Minireview: development and differentiation of gut endocrine cells. Endocrinology 145, 2639–2644. doi: 10.1210/en.2004-0051
Sender, R., Fuchs, S., and Milo, R. (2016). Revised estimates for the number of human and bacteria cells in the body. PLOS Biol. 14, e1002533. doi: 10.1371/journal.pbio.1002533
Sharma, R., Gupta, D., Mehrotra, R., and Mago, P. (2021). Psychobiotics: the next-generation probiotics for the brain. Curr. Microbiol. 78, 449–463. doi: 10.1007/s00284-020-02289-5
Shen, S. J., and Wong, C. H. Y. (2016). Bugging inflammation: role of the gut microbiota. Clin. Transl. Immunol. 5, e72. doi: 10.1038/cti.2016.12
Stikic, M., Berka, C., Levendowski, D., Rubio, R. F., Tan, V., Korszen, S., et al. (2014). Modeling temporal sequences of cognitive state changes based on a combination of EEG-engagement, EEG-workload, and heart rate metrics. Front. Neurosci. 8, 342. doi: 10.3389/fnins.2014.00342
Tang, Q., Jin, G., Wang, G., Liu, T., Liu, X., Wang, B., et al. (2020). Current sampling methods for gut microbiota: a call for more precise devices. Front. Cell. Infect. Microbiol. 10, 151. doi: 10.3389/fcimb.2020.00151
Thomas, V., Clark, J., and Dor,é, J. (2015). Fecal microbiota analysis: an overview of sample collection methods and sequencing strategies. Future Microbiol. 10, 1485–1504. doi: 10.2217/fmb.15.87
Toet, A., Brouwer, A.-M., Barski, J. J., Spink, A., Riedel, G., and Roy, R. N. (2022). Developments in Implicit Measurements. Lausanne: Frontiers Media SA.
Torres-Fuentes, C., Schellekens, H, Divan, T. G., and Cryan, J. F. (2017). The microbiota—gut—brain axis in obesity. Lancet Gastroenterol. Hepatol. 2, 747–756. doi: 10.1016/S2468-1253(17)30147-4
Touryan, J., Apker, G., Lance, B. J., Kerick, S. E., Ries, A. J., and McDowell, K. (2014). Estimating endogenous changes in task performance from EEG. Front. Neurosci. 8, 155. doi: 10.3389/fnins.2014.00155
Van Erp, J. B. F., Lotte, F., and Tangermann, M. (2012). Brain-computer interfaces: beyond medical applications. IEEE Computer 45, 26–34. doi: 10.1109/MC.2012.107
Vianna, E. P. M., and Tranel, D. (2006). Gastric myoelectrical activity as an index of emotional arousal. Int. J. Psychophysiol. 61, 70–76. doi: 10.1016/j.ijpsycho.2005.10.019
Wang, Y.-T., Huang, K.-C., Wei, C.-S., Huang, T.-Y., Ko, L.-W., Lin, C.-T., et al. (2014). Developing an EEG based on-line closed-loop lapse detection and mitigation system. Front. Neurosci. 8, 321. doi: 10.3389/fnins.2014.00321
Wiggins, T., Kumar, S., Markar, S. R., Antonowicz, S., and Hanna, G. B. (2015). Tyrosine, phenylalanine, and tryptophan in gastroesophageal malignancy: a systematic review. Cancer Epidemiol. Biomarkers Prev. 24, 32–38. doi: 10.1158/1055-9965.EPI-14-0980
Yano, J. M., Yu, K., Donaldson, G. P., Shastri, G. G., Ann, P., Ma, L., et al. (2015). Indigenous bacteria from the gut microbiota regulate host serotonin biosynthesis. Cell 161, 264–276. doi: 10.1016/j.cell.2015.02.047
Keywords: neuro-ergonomics, implicit measures, gut-brain axis, microbiota, emotion, cognition, microbiome, behavior
Citation: van Erp JBF (2022) Gastrointestinal tract-based implicit measures for cognition, emotion and behavior. Front. Comput. Sci. 4:899507. doi: 10.3389/fcomp.2022.899507
Received: 18 March 2022; Accepted: 01 July 2022;
Published: 22 July 2022.
Edited by:
Stephen Fairclough, Liverpool John Moores University, United KingdomReviewed by:
Rasoul Mirzaei, Pasteur Institute of Iran (PII), IranJustin Estepp, Air Force Research Laboratory, United States
Copyright © 2022 van Erp. This is an open-access article distributed under the terms of the Creative Commons Attribution License (CC BY). The use, distribution or reproduction in other forums is permitted, provided the original author(s) and the copyright owner(s) are credited and that the original publication in this journal is cited, in accordance with accepted academic practice. No use, distribution or reproduction is permitted which does not comply with these terms.
*Correspondence: Jan B. F. van Erp, amFuLnZhbmVycCYjeDAwMDQwO3Ruby5ubA==