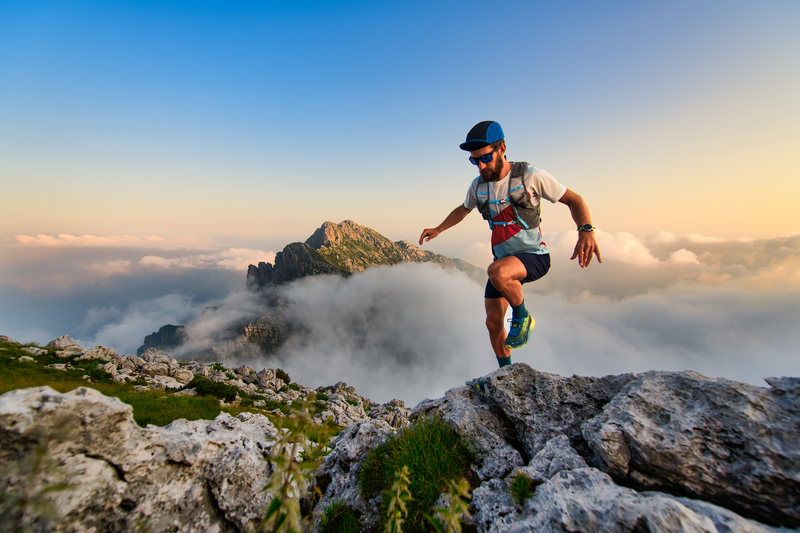
95% of researchers rate our articles as excellent or good
Learn more about the work of our research integrity team to safeguard the quality of each article we publish.
Find out more
PERSPECTIVE article
Front. Comput. Sci. , 26 September 2022
Sec. Human-Media Interaction
Volume 4 - 2022 | https://doi.org/10.3389/fcomp.2022.885657
This article is part of the Research Topic Effective and Attractive Communication Signals in Social, Cultural, and Business Contexts View all 36 articles
In this perspective paper we explore the question how audible smiling can be integrated in speech synthesis applications. In human-human communication, smiling can serve various functions, such as signaling politeness or as a marker of trustworthiness and other aspects that raise and maintain the social likeability of a speaker. However, in human-machine communication, audible smiling is nearly unexplored, but could be an advantage in different applications such as dialog systems. The rather limited knowledge of the details of audible smiling and their exploitation for speech synthesis applications is a great challenge. This is also true for modeling smiling in spoken dialogs and testing it with users. Thus, this paper argues to fill the research gaps in identifying factors that constitute and affect audible smiling in order to incorporate it in speech synthesis applications. The major claim is to focus on the dynamics of audible smiling on various levels.
Would users appreciate audible smiling in speech produced by machines, be it text-to-speech (TTS) systems on computers, virtual agents or social robots? If yes, how should the synthesis of audible smiling be approached? There is evidence that smiling in human-human interaction is not only visible but also audible (Tartter, 1980; Tartter and Braun, 1994). Visual signals are often seen as primary in face-to-face situations and acoustic signals as secondary. However, in situations restricted to the auditory-acoustic channel, for instance on the telephone, smiling can only be perceived by vocal and consequently acoustic features.
There is a multitude of functions smiling can have in different everyday social settings. Smiling can be interpreted as a marker of friendliness and politeness, it can be used to express amusement and exhilaration, and it is often applied to build trust between speakers because it strongly increases their social likeability. Obviously, there is a great potential for this effective and attractive social signal to be exploited in human-computer interaction (HCI) and we see a need to clarify the complexity of this topic before we start with the development of technical solutions and the testing of their usability. Thus, the aim of this perspective paper is to provide an ordered collection of thoughts on audible smiling in synthetic speech. We identify findings, but also problems, on various levels and suggest approaches for solutions. These critical thoughts are neither meant as “wishful thinking” nor as a feasibility study, but they are supposed to shed light on a potential asset to technical systems in terms of an increased naturalness in the interaction with a human user. Our thoughts are always directed to the auditory channel (without ignoring the dominant visual channel).
For the domain of visual smiles, it is understood that dynamic characteristics of facial and head movements inherently contribute to the production and perception of the social signal categorization that is manifested as visible smile (Frank et al., 2003). Quite relevant for audible smiles, this includes duration, as, e.g., videos of smiling faces rated as amused were longer than those rated as polite (Ambadar et al., 2009). Examining the morphological and dynamic characteristics of smiles is required in order to discriminate various functions of smiles (Rychlowska et al., 2017). For the acoustic channel, however, this change of perspective has not yet taken place. So, our claim is to concentrate on the dynamics of smiled speech. This change needs to happen on various levels, as the challenges are
• Temporal dynamics: Since it is highly unlikely that entire utterances are articulated smiling, the factors affecting choice of sections for expressing a social signal with smiling needs to be identified. In a conversation, this comprises when spoken feedback (“u-hu”, “m-hm”, “yeah”) is audibly smiled.
• Intensity dynamics: Are there, like with other affective display in speech, degrees and nuances of audible smiling, and what are their regulatory factors? From a perceptual point of view, graded intensity in smiled speech synthesis can be perceived by humans (El Haddad et al., 2015).
• Social signaling: Smiling can be analyzed as a referring expression. But it is still unclear, if referents can be distinguished in speech, what they are and which social and affective function is linked with this smile.
To meet these challenges, we propose to deepen the research on the acoustic properties, perception, and interpretation of smiled speech and to identify factors affecting its dynamics, e.g., content, discourse markers, and social function/meaning.
Prototypical associations of smiling are positive affective states such as happiness and joy, a good mood or contentment. Smiling can also be used for seduction or as an expression of amusement (Schröder et al., 1998), but also to mark irony. On the recipient's side, smiling belongs to those social signals that can generate the impression of interest, friendliness, and intimacy (Floyd and Erbert, 2003; Krumhuber et al., 2007; Burgoon et al., 2018).
These positively associated types of smiling bear an authentic character. In addition there are non-authentic or not genuinely felt types of smiling. Happiness is probably often expressed with a smile, but a smile is not necessarily linked to positive emotions. Examples include situations where negative emotions are masked with an expression of joy or situations in which individuals have feelings of uncertainty, nervousness or embarrassment (Keltner, 1995). This wide range of meanings of smiling have even been replicated in artificial faces (Ochs et al., 2017). Further examples include the expression of dominance toward others. The difference between “authentic/felt” and “non-authentic/non-felt” smiling in the visual channel is mainly reflected by the contraction or non-contraction of the eye-ring muscle (m. orbicularis oculi), the so-called Duchenne-smile (Ekman and Friesen, 1982). This difference in muscle contraction is also the base for separating trustworthy from deceptive behavior (Ekman and Friesen, 1982). For the visual channel, this fundamental distinction seems to be established, however not for the acoustic channel.
The general impression that a smiling face is regarded as more attractive than a non-smiling face is evidenced by numerous studies. For instance, in a Brazilian study smiling faces were considered as happier and even as more attractive than a neutral expression (Otta et al., 1982). Regarding the concept of visual attractiveness, a smile enforces the positive assessment of faces, particularly of females (Lau, 1982). Moreover, females typically smile more when flirting (Moore, 1985). For male faces this effect is not that clear (Mehu et al., 2008; Okubo et al., 2015). The transfer of these findings to synthetic voices is difficult because non-verbal material (photographs and videos), particularly in field research, has no reported relation to co-verbal smiling.
There are several forms of social smiling. They are core features for the display of politeness and friendliness, but they are not necessarily expressed with a Duchenne marker. A smile can also be used to show empathy and agreement with somebody else. Studies demonstrate that smiles that were rated as more genuine strongly predict judgments about the trustworthiness (Centorrino et al., 2015). In the majority of cases, genuine smiles trigger a reciprocal social action, even in HCI (Krämer et al., 2013).
It would generally be helpful if synthesized speech applications could express social functions associated with a smiling voice when appropriate. However, while state-of-the-art findings (Section 3) and data-driven models (Section 5.1) have reached a level to produce audible smiling, a solid basis to confirm or reject differences in audible smiles that refer to different social functions, is not known to us. In addition, other factors regulating the dynamics and location of audible smiling are yet to be modeled. As an example can serve the reciprocity in terms of initiating smiles and smiling back (Arias et al., 2018). While the relevance of reciprocate smiling is evident, is still unknown which conversational sections have to be synthesized and how the exact timing of this reciprocal mechanism works. On a broader lever, however, i.e., by treating this mechanism as synchrony, it could be shown that it is observable throughout whole conversations (Rauzy et al., 2022).
This goal is in line with the aims of social signal processing, e.g., formulated by Vinciarelli et al. (2012): “a human-centered vision of computing where intelligent machines seamlessly integrate and support human-human interactions, embody natural modes of human communication for interacting with their users […] At its heart, social intelligence aims at correct perception, accurate interpretation, and appropriate display of social signals”. In our view, this demand is still completely blank with respect to appropriate and perceivable synthesis of smiled speech.
It is tempting to assume that positive smiles are always realized as an “authentic” smile with a Duchenne marker. Likewise, it could be assumed that a smile of an unacquainted person is generally perceived as attractive, friendly and definitively positive (e.g., pictures in application letters or on personal homepages). However, there is evidence that in some non-Western cultures an authentic smile is not bound to a Duchenne marker (Thibault et al., 2012). Moreover, in a cross-cultural comparative study investigating face perception with subjects from more than 40 cultures, it could be shown that in some cultures smiling faces of unacquainted persons leave a negative impression on observers (Krys et al., 2016). Thus, smiling per se does not necessarily lead to a more positive impression of the perceiver. This could also be the case for audibly transmitted smiling, particularly when coming from a synthesized voice. Another example of cultural diversity regarding the usage of smiling is provided by a study where Chinese and Dutch kindergarten children were asked to play a game—either alone or together with peers (Mui et al., 2017). In contrast to the Dutch children, who did not change their smiling behavior between both conditions, the Chinese children smiled more when playing with other children.
A clear distinction should be made between smiling and laughter. Both concepts can have similar functions and sometimes they are used as synonyms (e.g., the expression “s/he laughed with me” when actually the smile of a person was directed to another person). Laughter can occur with much variability and complexity (Truong et al., 2019). Most forms of laughter do not overlap with speech, in contrast to “speech-laughs” (Nwokah et al., 1999; Trouvain, 2001) where laughter occurs while articulating. This “laughed speech” is mainly characterized by a high degree of breathiness together with a vibrato-like voice quality (often only for two syllables) and thus differs from “smiled speech” (Trouvain, 2001; Erickson et al., 2009).
Various studies were able to show that smiling is also perceivable from speech and without visual information (Tartter, 1980; Tartter and Braun, 1994). Utterances produced with a non-emotional mechanical lip spreading are perceived as being more “smiled” than utterances without lip spreading (Robson and Beck, 1999). Perceivable smiled speech can be explained with changes of various acoustic parameters: Compared to non-smiled speech the fundamental frequency (F0) is higher due to a higher overall muscular tension, the second formant (F2) is higher due to a shortened vocal tract from lip spreading and a raised larynx. By articulatory synthesis, it could be verified that all three factors have a perceptual effect, but combined, the audible smiling is stronger (Stone et al., 2022). These effects can also be observed for the high unrounded front vowel [i], in contrast to vowels that are (more) rounded and/or lower and/or further back, e.g., [o]. This reflects Ohala's “i-face” for smiling and “o-face” for threatening (Ohala, 1980, 1984). The described tendencies have been confirmed by later studies (Schröder et al., 1998; Drahota et al., 2008).
The perception of smiling from voice also depends on the perceived intensity. A cross-lingual study (Emond et al., 2016) showed that listeners need more time to recognize a mild smile compared to more intense smiles. The same study also revealed a linguistic advantage for the recognition of audible smiles. Listeners were slower in smiling detection and recognized fewer smiles when they did not share the same accent or the same language as the speakers.
Further studies are needed to achieve a more differentiated overview of phonetic parameters such as intensity, duration and voice quality in smiled speech. A particular focus should be on perception, especially the timing of smiling in dialogues, the perceived intensity of audible smiling, and the cross-modal aspects of smiling perception.
Often the motivation of researchers and developers is to make machines more human-like. An example for this positive transfer is a study with a human-like virtual agent where adult subjects smiled longer at the robots when the robots showed some (visual) smile as well. This means that the reciprocal smile was increased on the side of the users (Krämer et al., 2013). However, it is not clear whether human users really benefit from a smiling interaction with a machine. For instance, a study where children (9 years) used social robots as learning tutors showed that the children achieved better results when the robots did not act in a friendly way (Kennedy et al., 2017). This illustrates the need for shifting from mere synthesis of smiling toward proper manifestation of audible smiles as expression of a specific social meaning, for which a solid basis on constituting factors is required.
Audiobooks are a wide field of applications for synthetic speech. In audiobook productions using human voices, direct speech of various characters in fictional literature can either be displayed by different professional speakers or by the same speaker who uses different voice qualities for the characters. In synthesized audiobooks, a given character or situation could be displayed by a “smiled voice”. A requirement for an appropriate application of smiled speech synthesis would be a text analysis tool that finds those portions of direct speech where smiling fits. This could either be done by finding words of the semantic field of smiling (e.g., grin, mischievous, friendly) or by a sentiment analysis directed to friendliness, politeness and further functions of smiling.
In contrast to virtual (embodied conversational) agents where a high-quality animation of the facial expression is possible (Ochs et al., 2010), many social robots without a display head like Pepper or Nao, do not have the possibility to generate a visible smile. An audible smile could be helpful as a social signal to avoid an uncanny valley effect. Virtual agents with visual smiling were regarded as friendlier and more attractive than those without, and smiling also enforces the impression of extroversion (Cafaro et al., 2012).
A special dimension is opened up when social robots have children as users, for instance care takers in nursing homes or training dolls for autistic children. In the interaction between children and social robots an increased degree of familiarity and trust seems to be substantial. Important components to achieve this are non-verbal behavior, feedback control and other forms of interaction management (Belpaeme et al., 2018). Smiling, including audible smiling, can also play a relevant role in this context.
The coordinated interaction of conversations depends on proper timing of production or even missing production of spoken signals to convey meaning and to ensure the conversational flow. This kind of coordination comprises back-channel and turn-taking signals (Enfield, 2017) as well as (automatic) convergence (Branigan et al., 2010). Both kinds are potentially subject to audible smiles, but only for the latter, empirical evidence is known to the best of our knowledge.
This kind of convergence could also be observed by Krämer et al. (2013) where adult subjects smiled for a longer time with artificial agents when also the agent showed a (visual) smile. This is in line with Torre et al. (2020) who directly tested audible smiling in a gaming scenario and found increased trustworthiness even in contradicting behavioral evidence.
The challenge for integrating audible smiling in speech synthesis can be regarded at different levels. For different methods of signal generation the limited knowledge about audible smiling in humans should be exploited. Modeling audible smiling in dialogs requires the control of temporal, discourse-relevant and cultural aspects, in addition to the signal generation. Last but not least the evaluation of appropriateness in given applications represents the third component.
Articulatory synthesis would be an obvious choice for verifying the perceptual validity of findings concerning the properties of smiled speech. Testing and verifying such analytic results for perception, in our domain social signals manifested by smiling and its interplay with phonetic dynamics, testing and verifying such analytic results for perception could greatly benefit from articulatory synthesis to produce intensity and dynamic nuances in a controlled way. However, given the current increase in (and maybe demand for) high signal quality, a data-driven approach seems also advisable. An early attempt of smiled speech synthesis for HMM synthesis, utilizing parallel corpora, confirms the perceptual effect of smiling intensity, but also reveals issues with naturalness (El Haddad et al., 2015). These, however, seem to be overcome in more recent work (Kirkland et al., 2021). Still, the typical limitation of data-driven synthesis, i.e., difficulty to draw conceptual conclusions like identifying relevant factors, is not overcome.
Using smiled synthetic speech in real-world applications requires a contextually appropriate control of the synthesis that considers content and culture when selecting sections to be produced as smiled. An automatic symbolic annotation of those sections requires a language- and culture-specific model and a sentiment analysis of the text to be synthesized.
Interactive applications could be enhanced by the generation of discourse-dependent social signals, for instance reciprocal smiling. This in return requires a clarification how smiling in human-human interaction is distinct in audio-visual from audio-only situations. In general, we have to model the audible smiling with regard to its timing (duration, start and end relative to speech) and acoustic quality (distinctiveness to non-smiled speech).
Since smiling has so many functions, an evaluation must monitor the matching of the intention of the generated smile and the interpretation of the perceived smile in given situations. A general assessment with mean opinion scores seems not to be the right way for evaluation but contextual appropriateness as demanded by Wagner et al. (2019). Thus, the needs and preferences of the users of synthetic speech must be tested, preferably in a behavioral paradigm, i.e., not (solely) by explicit ratings, but observed behavioral (gamified) choices instead. Smiled voice should not be regarded as one style of expressiveness, but as a carrier mechanism to transport many different expressions—with each expressive function evaluated separately as illustrated in Figure 1.
Figure 1. Different functions of smiling as evaluation parameters to test the appropriateness of signal generation, application, acoustic quality, and timing.
There is no doubt that smiling serves a multitude of important social functions. Currently, politeness and friendliness are not yet the core features of synthetic voices. Although the call for more and better expressiveness in speech synthesis has been around for a while (Schröder, 2009), there have been hardly any attempts to tackle this challenge for smiled voices.
For audio books, it can clearly be beneficial to use smiled voices. In dialogical applications, there may be an advantage when users are made aware of smiled voice. Irritations for users evoked by such a human-like unfamiliarity and unexpected peculiarity should be avoided.
Should speaking machines be able to smile? Anthropomorphizing of non-human objects is a possibility which can be applied to building trust. However, it could also lead to disappointed expectations regarding social competence or even to an “uncanny valley of mind” (Gray and Wegner, 2012).
What should be the next steps in the upcoming years (or decades) in order to achieve a more human-like smiling behavior in speech synthesis? There is a research gap for smiled voice in many respects. Research in voice attractiveness still lacks vocal aspects of smiling as an effective mean of sexy, likable and charismatic speakers (Weiss et al., 2020). The majority of research in human smiling is exclusively concentrated on the visual channel. In this research direction, the main objects of study are pictures of faces (often without glasses and beards, and face masks). It lacks the temporal dynamics, the changing intensity of the smile, and the situational and verbal context in which the smile occurred. These can be very important features when modeling smiling in speech (for synthesis or other applications).
In addition, the relation between the visual and the acoustic information is under-explored, particularly in talk-in-interaction (between humans and in HCI). Moreover, situations with machine-aided communication, such as human-robot interaction or as a training device for autistic children, require a thorough understanding of the effects of smiling in the audio-only and the audio-visual modalities as well as in “smile-in-interaction”.
Although our thoughts aim at the audio-only aspects of synthesized speech, it can of course also be useful when thinking about audio-visual aspects in speech synthesis, as e.g., in embodied conversational agents or in social robots.
Based on the presented, albeit limited, state description, we argue to fill the identified gaps. While in principle, audible smiling can already be synthesizes for a given duration of speech, the challenges are in quantitative models that incorporate the communicative factors of smiling function (Figure 1), and timing and dynamics of audible smiling in their interrelation to the linguistic and coordinating properties of speech, like smiling duration and intensity within phrases and turns. With such models, we expect a major advance in communicative meaningful synthesis of, e.g., audio books' or artificial agents' speech. In short, basic research is needed with respect to (i) when exactly, (ii) to which degree, and (iii) for which purpose humans smile in spoken interaction. The research gap also concerns the phonetic aspects of smiled speech. How do acoustics and perception interact? How is visual information processed in combination with acoustic information in speech? How can manipulations be evaluated? Research and development both require more (annotated) data which currently do not exist in the quality and amount needed. We did not regard other social factors, like gender (Hiersch et al., 2022) or status, which are known to affect overall amounts of smiling display, but which we expects not as impactful at this particular state of research. Taken together, we consider our thoughts on audible smiled speech as a contribution that helps to further develop social signal processing (Vinciarelli et al., 2012).
The original contributions presented in the study are included in the article/supplementary material, further inquiries can be directed to the corresponding author/s.
JT and BW: concept, literature research, and formulations. Both authors contributed to the article and approved the submitted version.
We acknowledge support by the Deutsche Forschungsgemeinschaft (DFG, German Research Foundation) and Saarland University within the ‘Open Access Publication Funding’ programme.
We thank commentators of a conference presentation given to a similar topic (Trouvain and Weiss, 2020). We are particularly grateful to Iona Gessinger and Mikey Elmers for comments on an earlier draft of this paper.
The authors declare that the research was conducted in the absence of any commercial or financial relationships that could be construed as a potential conflict of interest.
All claims expressed in this article are solely those of the authors and do not necessarily represent those of their affiliated organizations, or those of the publisher, the editors and the reviewers. Any product that may be evaluated in this article, or claim that may be made by its manufacturer, is not guaranteed or endorsed by the publisher.
Ambadar, Z., Cohn, J. F., and Reed, L. I. (2009). All smiles are not created equal: Morphology and timing of smiles perceived as amused, polite, and embarrassed/nervous. J. Nonverbal Behav. 1, 17–34. doi: 10.1007/s10919-008-0059-5
Arias, P., Belin, P., and Aucouturier, J.-J. (2018). Auditory smiles trigger unconscious facial imitation. Curr. Biol. 28, R782. doi: 10.1016/j.cub.2018.05.084
Belpaeme, T., Vogt, P., van den Berghe, R., Bergmann, K., Goksun, T., Haas, M, et al. (2018). Guidelines for designing social robots as second language tutors. Int. J. Soc. Robot. 10, 325–341. doi: 10.1007/s12369-018-0467-6
Branigan, H., Pickering, M., Pearson, J., and Mclean, J. (2010). Linguistic alignment between people and computers. J. Pragmat. 42, 2355–2368. doi: 10.1016/j.pragma.2009.12.012
Burgoon, J., Buller, D., Hale, J., and Turck, M. (2018). Relational messages associated with nonverbal behaviors. Hum. Commun. Res. 10, 351–378. doi: 10.1111/j.1468-2958.1984.tb00023.x
Cafaro, A., Vilhjalmsson, H., Bickmore, T., Heylen, D., Johannsdottir, K., and Valgarosson, G. (2012). “First impressions: users' judgments of virtual agents' personality and interpersonal attitude in first encounters,” in Proc. 12th Int'l Conf. Intell. Virtual Agents (Santa Cruz, CA), 1–14. doi: 10.1007/978-3-642-33197-8_7
Centorrino, S., Djemai, E., Hopfensitz, A., Milinski, M., and Seabright, P. (2015). Honest signaling in trust interactions: smiles rated as genuine induce trust and signal higher earning opportunities. Evol. Hum. Behav. 36, 8–16. doi: 10.1016/j.evolhumbehav.2014.08.001
Drahota, A., Costall, A., and Reddy, V. (2008). The vocal communication of different kinds of smile. Speech Commun. 50, 278–287. doi: 10.1016/j.specom.2007.10.001
Ekman, P., and Friesen, W. (1982). Felt, false, and miserable smiles. J. Nonverbal Behav. 6, 238–258. doi: 10.1007/BF00987191
El Haddad, K., Cakmak, H., Moinet, A., Dupont, S., and Dutoit, T. (2015). “An HMM-approach for synthesizing amused speech with a controllable intensity of smile,” in IEEE International Symposium on Signal Processing and Information Technology (Abu Dhabi), 7–11. doi: 10.1109/ISSPIT.2015.7394422
Emond, C., Rilliard, A., and Trouvain, J. (2016). “Perception of smiling in speech in different modalities by native vs. non-native speakers,” in Proc. Speech Prosody, (Boston, MA), 639–643. doi: 10.21437/SpeechProsody.2016-131
Erickson, D., Menezes, C., and Sakakibara, K. (2009). “Are you laughing, smiling or crying?” in Proc. APSIPA Summit and Conference (Sapporo), 529–537.
Floyd, K., and Erbert, L. (2003). Relational message interpretations of nonverbal matching behavior: an application of the social meaning model. J. Soc. Psychol. 143, 581–597. doi: 10.1080/00224540309598465
Frank, M., Ekman, P., and Friesen, W. (2003). Behavioral markers and recognizability of the smile of enjoyment. J. Pers. Soc. Psychol. 64:83–93. doi: 10.1037/0022-3514.64.1.83
Gray, K., and Wegner, D. (2012). Feeling robots and human zombies: mind perception and the uncanny valley. Cognition 125, 125–130. doi: 10.1016/j.cognition.2012.06.007
Hiersch, P., McKeown, G., Latu, I., and Rychlowska, M. (2022). “Gender differences, smiling, and economic negotiation outcomes,” in Proceedings of the Workshop on Smiling and Laughter across Contexts and the Life-Span within the 13th Language Resources and Evaluation Conference (Marseille: European Language Resources Association), 11–15.
Keltner, D. (1995). Signs of appeasement: evidence for the distinct displays of embarrassment, amusement, and shame. J. Pers. Soc. Psychol. 68, 441–454. doi: 10.1037/0022-3514.68.3.441
Kennedy, J., Baxter, P., and Belpaeme, T. (2017). The impact of robot tutor nonverbal social behavior on child learning. Front. ICT Hum. Media Interact. 4, 6. doi: 10.3389/fict.2017.00006
Kirkland, A., Wå,odarczak, M., Gustafson, J., and Szekely, E. (2021). “Perception of smiling voice in spontaneous speech synthesis,” in Proc. 11th ISCA Speech Synthesis Workshop (SSW 11), 108–112. doi: 10.21437/SSW.2021-19
Krämer, N., Kopp, S., Becker-Asano, C., and Sommer, N. (2013). Smile and the world will smile with you – the effects of a virtual agent's smile on users' evaluation and behavior. Int. J. Hum. Comput. Stud. 71, 335–349. doi: 10.1016/j.ijhcs.2012.09.006
Krumhuber, E., Manstead, A., Cosker, D., Marshall, D., Rosin, P., and Kappas, A. (2007). Facial dynamics as indicators of trustworthiness and cooperative behavior. Emotion 7, 730–735. doi: 10.1037/1528-3542.7.4.730
Krys, K., Vauclair, C.-M., Capaldi, C. A., Miu-Chi Lun, V., Bond, M. H., Domínguez-Espinosa, A., et al. (2016). Be careful where you smile: culture shapes judgments of intelligence and honesty of smiling individuals. J. Nonverbal Behav. 40, 101–116. doi: 10.1007/s10919-015-0226-4
Lau, S. (1982). The effect of smiling on person perception. J. Soc. Psychol. 117, 63–67. doi: 10.1080/00224545.1982.9713408
Mehu, M., Little, A., and Dunbar, R. (2008). Sex differences in the effect of smiling on social judgments: an evolutionary approach. J. Soc. Evol. Cult. Psychol. 2, 103–121. doi: 10.1037/h0099351
Moore, M. (1985). Non-verbal courtship patterns in women: context and consequences. Ethol. Sociobiol. 6, 237–247. doi: 10.1016/0162-3095(85)90016-0
Mui, P., Goudbeek, M., Swerts, M., and Hovasapian, A. (2017). Children's non-verbal displays of winning and losing: effects of social and cultural contexts on smiles. J. Nonverbal Behav. 41, 67–82. doi: 10.1007/s10919-016-0241-0
Nwokah, E., Hsu, H.-C., Davies, P., and Fogel, A. (1999). The integration of laughter and speech in vocal communication: a dynamic systems perspective. J. Speech Lang. Hear. Res. 42, 880–894. doi: 10.1044/jslhr.4204.880
Ochs, M., Niewiadomski, R., and Pelachaud, C. (2010). “How a virtual agent should smile? Morphological and dynamic characteristics of virtual agent's smiles,” in Proc. Int'l Conf. on Intelligent Virtual Agents, 427–440. doi: 10.1007/978-3-642-15892-6_47
Ochs, M., Pelachaud, C., and McKeown, G. (2017). A user-perception based approach to create smiling embodied conversational agents. ACM Trans. Interact. Intell. Syst. 7, 1–33. doi: 10.1145/2925993
Ohala, J. (1980). The acoustic origin of the smile. J. Acoust. Soc. Am. 68, S33. doi: 10.1121/1.2004679
Ohala, J. (1984). An ethological perspective on common cross-language utilization of f0 of voice. Phonetica 41, 1–16. doi: 10.1159/000261706
Okubo, M., Ishikawa, K., Kobayashi, A., Laeng, B., and Tommasi, L. (2015). Cool guys and warm husbands: the effect of smiling on male facial attractiveness for short- and long-term relationships. Evol. Psychol. 13, 1–8. doi: 10.1177/1474704915600567
Otta, E., Abrosio, F. F. E., and Hoshino, R. L. (1982). Reading a smiling face: messages conveyed by various forms of smiling. Percept. Motor Skills 82, 1111–1121. doi: 10.2466/pms.1996.82.3c.1111
Rauzy, S., Amoyal, M., and Priego-Valverde, B. (2022). “A measure of the smiling synchrony in the conversational face-to-face interaction corpus PACO-CHEESE,” in Proceedings of the Workshop on Smiling and Laughter across Contexts and the Life-Span within the 13th Language Resources and Evaluation Conference (Marseille: European Language Resources Association), 16–20.
Robson, J., and Beck, J. M. (1999). “Hearing smiles–perceptual, acoustic and production aspects of labial spreading,” in Proc. 14th Int'l Congress of Phonetic Sciences (ICPhS) (San Francisco, CA), 219–222.
Rychlowska, M., Jack, R. E., Garrod, O. G. B., Schyns, P. G., Martin, J. D., and Niedenthal, P. M. (2017). Functional smiles: tools for love, sympathy, and war. Psychol. Sci. 28, 1259–1270. doi: 10.1177/0956797617706082
Schröder, M. (2009). “Expressive speech synthesis: past, present, and possible futures,” in Affective Information Processing, eds J. Tao and T. Tan (London: Springer), 111–126. doi: 10.1007/978-1-84800-306-4_7
Schröder, M., Auberge, V., and Cathiard, M.-A. (1998). “Can we hear smiles?” in Proc. Conference on Spoken Language Processing (ICSLP) (Sydney, NSW), 559–562. doi: 10.21437/ICSLP.1998-106
Stone, S., Abdul-Hak, P., and Birkholz, P. (2022). “Perceptual cues for smiled voice - an articulatory synthesis study,” in Studientexte zur Sprachkommunikation: Elektronische Sprachsignalverarbeitung 2022, eds O. Niebuhr, M. S. Lundmark, and H. Weston (Dresden: TUD Press), 131–138.
Tartter, V., and Braun, D. (1994). Hearing smiles and frowns in normal and whisper registers. J. Acoust. Soc. Am. 96, 2101–2107. doi: 10.1121/1.410151
Tartter, V. C. (1980). Happy talk: perceptual and acoustic effects of smiling on speech. Percept. Psychophys. 27, 24–27. doi: 10.3758/BF03199901
Thibault, P., Levesque, M., Gosselin, P., and Hess, U. (2012). The Duchenne marker is not a universal signal of smile authenticty–but it can be learned! Soc. Psychol. 43, 215–221. doi: 10.1027/1864-9335/a000122
Torre, I., Goslin, J., and White, L. (2020). If your device could smile: people trust happy-sounding artificial agents more. Comput. Hum. Behav. 105, 106216. doi: 10.1016/j.chb.2019.106215
Trouvain, J. (2001). “Phonetic aspects of ‘speech-laughs',” in Proc. Conference on Orality &Gestuality (ORAGE) (Aix-en-Provence), 634–639.
Trouvain, J., and Weiss, B. (2020). “Uberlegungen zu wahrnehmbarem Lacheln in synthetischen Stimmen,” in 31th Conference Elektronische Sprachsignalverarbeitung (Magdeburg), 26–33.
Truong, K., Trouvain, J., and Jansen, M.-P. (2019). “Towards an annotation scheme for complex laughter in speech corpora,” in Proc. Interspeech (Graz), 529–533. doi: 10.21437/Interspeech.2019-1557
Vinciarelli, A., Pantic, M., Heylen, D., Pelachaud, C., Poggi, I., D'Errico, F., et al. (2012). Bridging the gap between social animal and unsocial machine: a survey of social signal processing. IEEE Trans. Affect. Comput. 3, 69–87. doi: 10.1109/T-AFFC.2011.27
Wagner, P., Beskow, J., Betz, S., Edlund, J., Gustafson, J., Eje Henter, G., et al. (2019). “Speech synthesis evaluation–state-of-the-art assessment and suggestion for a novel research program,” in Proc. 10th ISCA Workshop on Speech Synthesis (SSW 10) (Vienna), 105–110. doi: 10.21437/SSW.2019-19
Keywords: speech synthesis, social signaling, computational paralinguistics, smiling, trustworthiness
Citation: Trouvain J and Weiss B (2022) Thoughts on the usage of audible smiling in speech synthesis applications. Front. Comput. Sci. 4:885657. doi: 10.3389/fcomp.2022.885657
Received: 28 February 2022; Accepted: 29 August 2022;
Published: 26 September 2022.
Edited by:
Oliver Niebuhr, University of Southern Denmark, DenmarkReviewed by:
Yonghong Yan, Institute of Acoustics (CAS), ChinaCopyright © 2022 Trouvain and Weiss. This is an open-access article distributed under the terms of the Creative Commons Attribution License (CC BY). The use, distribution or reproduction in other forums is permitted, provided the original author(s) and the copyright owner(s) are credited and that the original publication in this journal is cited, in accordance with accepted academic practice. No use, distribution or reproduction is permitted which does not comply with these terms.
*Correspondence: Jürgen Trouvain, dHJvdXZhaW5AbHN0LnVuaS1zYWFybGFuZC5kZQ==
Disclaimer: All claims expressed in this article are solely those of the authors and do not necessarily represent those of their affiliated organizations, or those of the publisher, the editors and the reviewers. Any product that may be evaluated in this article or claim that may be made by its manufacturer is not guaranteed or endorsed by the publisher.
Research integrity at Frontiers
Learn more about the work of our research integrity team to safeguard the quality of each article we publish.