- School of Marketing and Communication, University of Vaasa, Vaasa, Finland
There is much discussion about super artificial intelligence (AI) and autonomous machine learning (ML) systems, or learning machines (LM). Yet, the reality of thinking robotics still seems far on the horizon. It is one thing to define AI in light of human intelligence, citing the remoteness between ML and human intelligence, but another to understand issues of ethics, responsibility, and accountability in relation to the behavior of autonomous robotic systems within a human society. Due to the apparent gap between a society in which autonomous robots are a reality and present-day reality, many of the efforts placed on establishing robotic governance, and indeed, robot law fall outside the fields of valid scientific research. Work within this area has concentrated on manifestos, special interest groups and popular culture. This article takes a cognitive scientific perspective toward characterizing the nature of what true LMs would entail—i.e., intentionality and consciousness. It then proposes the Ethical Responsibility Model for Robot Governance (ER-RoboGov) as an initial platform or first iteration of a model for robot governance that takes the standpoint of LMs being conscious entities. The article utilizes past AI governance model research to map out the key factors of governance from the perspective of autonomous machine learning systems.
Introduction
For decades now, the performance reality of artificial intelligence (AI) development in relation to our ideas of AI in public and individual imagination have not failed to disappoint (Wilner, 2018; Smith, 2019). Many are still waiting for Number 5 to come alive as that adorable yet clumsy looking robot from the 1980s movie Short Circuit. Others are ready to jump on the opportunity to own their own Rosey, from the children's animated series The Jetsons, to come and take care of the housework. No doubt, thanks to the prominence of robot representation in popular culture, people are also worried about the flip side of autonomous robotics and singularity as seen notoriously in movies such as The Terminator series, I, Robot, and even the legendary HAL from 2001: A Space Odyssey. While interest in issues of robot governance and the potential reality of a human society inhabited by autonomous robot systems has existed for decades, if not centuries (Murphy, 2021), the distance between a here and now to that futuristic world has rendered scholarly efforts as speculative, ambiguous and even on the verge of anti-progressive. These efforts may even be understood as deconstructive or even destructive to the advancements of science and technology.
Yet, already in today's world, citizens are gradually becoming accustomed to the idea of robots everywhere—cleaning interior spaces (Roomba for instance) and mowing the lawns. Self-driving cars and their more sophisticated systems are also rising in societal consciousness for the imminent and immediate future. Through the development and implementation of these increasingly “aware” systems, concerns are emerging for safety, security, fairness, and human integrity (Jobin et al., 2019). Before even reaching the topic of learning machines in robot form, we may observe the myriad of controversies already existing in our so-called everyday intelligent systems. Targeted news and advertising for instance, are causing controversy regarding privacy (Zarouali et al., 2018; West, 2019), reliability (see e.g., Seo and Faris, 2021, mis- and disinformation), and information bubbles (Koivula et al., 2019). To some extent these dissolve into the “shrug affect” or a “dejected acceptance and compliance” (Shklovski et al., 2014) of systems that operate beyond the general tolerance or comfort zone of human psycho-physiological acceptability.
To understand the complexity that learning artifacts and systems introduce to an already baffling technological landscape, one only needs to step back to observe recent controversies that have unfolded in and through popular digital business—Facebook, Uber, Airbnb to name some. Problems that can be witnessed in online services such as offered by these from the recent past already show the urgent need to devise and implement governance models that incorporate an understanding of responsibility relations and accountability standards (Smyth, 2019). Facebook for instance, has had plentiful scandals, many regarding moderation issues, e.g., who is responsible for the dissemination of fake news and the damage that it causes? Other problems have included general data, privacy, copy-write issues and psychological tests on unwilling, non-consenting users (see e.g., Meisenzahl and Canales, 2021). One Uber incident involved driver, Jason Dalton, who had murdered six people in Kalamazoo during his Uber shift (Heath, 2016). While, Airbnb spends considerable resources per year, smoothing out “nightmares” (Carville, 2021) of sexual assault and other types of violence and theft.
Economist Rachel Botsman (2012) talks of trust as being the new currency. Yet, in order to have trust, individuals need to perceive the right conditions for safety and security (Saariluoma and Rousi, 2020). The notion of accountability refers to the act of being held responsible for, and causally linked to phenomena, people and acts through readiness to either compensate or correct matters when challenges arise (Merriam-Webster Dictionary, 2021a). Accountability in other words, can be understood as a “safety net” and oversight mechanism in which parties are ready to respond and assist (Hochman, 2020). One great concern for the emerging era of AI societies stems from what is already observed in relation to data intensive technology and business; confusion over and lack of accountability and responsibility for the consequences of the technology's behavior, and what the technology affords humans to do to other humans and life (animals, nature etc.) in general (Smyth, 2019).
Thus, the main research question at the heart of this article is: Who will be responsible and accountable for autonomous, self-learning robots in society? Theoretical considerations linked to this question and the proposed model of this article are AI governance model development to date, in addition to understanding the nature of self-learning machinery. From cognitive science and constructive psychological perspectives, learning is intentional. That is, in order for an organism or system to learn, it needs to possess intentionality, or in other words, consciousness (Félix et al., 2019; O'Madagain and Tomasello, 2022). Therefore, the next question of the article pertains to: Will robots be able to be held responsible for their own actions? And, how will these entities and systems be governed?
Autonomous Learning Systems in Our Communities
The motivation behind this article is both practical and philosophical, which in turn, shifts back toward the practical. For, we are already beginning to witness challenges of responsibility and accountability with the few learning machines (LMs) that are steadily entering our societies, i.e., self-driving vehicles. It is no wonder that difficulties are faced when introducing and deploying such complex systems, if the prominent existing systems (mentioned above) are already posing immense challenges. Incidentally, returning to Uber, in her paper “Moral Crumple Zones: Cautionary Tales in Human-Robot Interaction,” Elish (2019) describes a 2018 case in which a self-driving Uber car ran over a pedestrian on an Arizonan road in the United States. The matter that drew Elish's attention to the case was the emphasis that was placed on the responsibility of the human “safety driver”—a person who sits in the car overseeing that the car will not head into collision course and/or takes action to prevent the incident from occurring. As a result of the accident, authorities rendered the safety driver accountable for the occurrence, meaning that she would face charges of vehicle manslaughter (Somerville and Shepardson, 2018). Elish's argument is that similarly to the crumple zones of cars that were introduced to car bodies in the 1970s and 80s, withholding a great amount of the impact in the event of collisions, humans are the moral crumple zones of technology. More directly to the point, Elish highlights that in prominent media and other public discourse, oftentimes when incidents occur in which complex technological systems are involved the end-user is held accountable. This can be seen also in cases such as the 1970s Three Mile Island Nuclear Generating Station that underwent a partial core meltdown 3 months after the second nuclear unit had been launched into operation. It was then claimed that the power plant employees were to blame for the partial meltdown, not the technology, its usability, logic or safety systems.
In particular, Elish cites the terminology used by an aviation expert who articulated in the 1980s that the fly-by-wire commercial jets (i.e., Airbus320 and subsequently A330), could be programmed to safeguard incidents when even if the human pilots would make mistakes, the aircraft could continue to travel safely (Oslund, 1986). While, at the same time as the aircraft was more or less being described as ‘fool-proof' and safe, for instance the Federal Aviation Authority had specified that, “[t]he pilot in command of an aircraft is directly responsible for, and is the final authority of the operation of that aircraft” (14 CFR 91.3.).1 Thus, the developed and implemented technology is faultless and safe, while the humans implicated in their usage are responsible if anything goes wrong. Fool-proof does not mean user-proof, and the technology is safe for as long as something does not go wrong. This breaks with any kind of logical understanding in at least two ways: (1) if the technology is faultless, then (as described by the 1980s aviation expert) even if a human user does not fully understand the technology and fails to operate appropriately, no negative incident should occur; and (2) there seems to be a complete invisibility of the humans at the other end of the technological development process—the developers, designers, development commissioners, owners, legislation overseers etc.
What is more, unlike in the cases of previous complex technology, the introduction of learning machinery, or autonomous robots (and their systems) into society, will mean not only that humans will deal with greater levels of technological complexity, but that these machines are indeed learning entities. For this reason two important aspects should be highlighted regarding attempts to create a governance model that focuses on ethical responsibility of robots in society: (1) is the role of development and programming, and at what stage and in relation to what should calculated decisions be made to ascertain and maintain human oversight and control over the systems; and (2) if the systems would actually be fully self-learning entities, then that they and/or their makers, commissioners and owners would be made responsible for the robots' actions. Yet, similarly to the conflict in understanding the above-mentioned example of “perfect technology” still equaling human user responsibility, this article also argues that the idea of developing and releasing self-learning machinery in any society is absurd. On the basis of basic learning and constructive psychology, and drawing on knowledge from cognitive science, any organism or entity that is capable of learning possesses the predisposition of intentionality and consciousness (Félix et al., 2019; O'Madagain and Tomasello, 2022). That is, if a technological artifact or system that possessed the function of learning were to be released into society, indeed the entity would and should be considered at the same level as other learning individuals (human citizens). With this, firm roles and regulations should be developed that included the robotic entities as subjects.
Accountability and Responsibility as a Point of Governance
Before describing the proposed governance model of this paper, it is important to establish a basis for understanding the concepts of accountability and responsibility. In recent decades both concepts have been raised to buzz status in public, media, technological, and business discourse. Likewise, these concepts have been heavily studied and contested, with no clear outcome of unified definitions (see e.g., Bovens et al., 2014). Responsibility however, is noted by Giddens (1999) as only emerging in the English language during the eighteenth-century. While the word “responsible” is much older, “responsibility” as a term has emerged hand-in-hand with the emergence of modernity. In its form today, responsibility is a multi-layered and ambiguous concept. The first account of responsibility is closely connected to the notion of responsible. That is, an actor (person) is responsible, or the author, of an event. In this first instance, the actor possesses agency—they cause or have caused an event or chains of events to happen in an active way. Another account of responsibility holds a much more ethical and accountable weighting. In this instance, responsibility is an obligation or liability. This second notion of responsibility is fascinating from the perspective of risk.
Risk, also a complex construct to define, has been described as pertaining two distinct characteristics: (1) uncertainty, and (2) exposure (Holton, 2004). Holton (2004) argues that in the case of risk, uncertainty and exposure only exist on the level of perception, or interpretation. This is due to the fact that as with trust—we trust that a relationship, interaction or an event will have a positive outcome, but can never be sure (or it is not trust, see e.g., Saariluoma et al., 2018)—that there is no such thing as absolute risk. If the outcome of a chain of actions or events is known, then there is no risk, simply certainty of causality. Thus, as Giddens (1999) argues, risk is a concept, “bound up with the aspiration to control and particularly with the idea of controlling the future” (p. 3). He goes on to mention that at all times risk is associated with a negative connotation. Risk alludes to the potentiality that an unwanted outcome may in fact be avoided. Thus, from this perspective, responsibility is interesting to compare with risk as there is an observable relationship. There is only a possibility for risks to exist if and when decisions need to be made. Responsibility emerges from the fact that when making decisions, people are engaging in a situation with discernable consequences.
Regarding the present paper, Giddens' observations of external and manufactured risk are particularly interesting. External risk, is the type of risk in which events or negative occurrences may happen to people unexpectedly. In other words, external risks happen from the outside. Manufactured risks are generated through the advancement of human development. This manufactured risk is synonymous with science and technology. This type of risk entails new risks that emerge through the progress of human technology. As seen in the instance of COVID-19 for instance, and human biology's inability to recognize the newly introduced virus, manufactured risks arise from circumstances in which humans have had little experience in the past. At this point, one may ponder: Are the risks that are arising from the introduction of learning machinery in society in fact new? Ideas of robots existing among humans are certainly not new. Yet perhaps, as also observed in the above-mentioned scandals arising from the data economy, the introduction of self-learning and autonomous robots within human communities may actually bring with them a myriad of threats, challenges and indeed risks, that have not been considered before their concrete implementation. In other words, why did the self-driving Uber not avoid the pedestrian in 2018? Particularly if it was, indeed perfect?
Bouncing Elish's (2019) insight on the role of the safety driver and their position of being made accountable for the incident, as with the dilemma of considering networks of responsibility and accountability in extremely complex, self-learning systems, the crisis of responsibility becomes ever more intense. As Giddens continues, in relation to manufactured responsibility, it “can neither easily be attributed nor assumed” (p. 8). Responsibility can either mean minimizing risk, e.g., forging backup plans, safety nets, emergency brakes or kill switches, or operates as an “energizing” principle, i.e., corporate social responsibility etc.
Accountability is a progressive concept that is constantly in flux (Mulgan, 1997; Flinders, 2017). It can be defined as, “a social relationship in which an actor feels an obligation to explain and to justify his or her conduct to some significant other” (Day and Klein, 1987, p. 5; Romzek and Dubnick, 1998, p. 6; Lerner and Tetlock, 1999, p. 255; McCandless, 2002, p. 22; Bovens, 2005, p. 184). The notion of accountability possesses ancient origins (Bovens et al., 2014). Accountability as a construct is close to accounting (Dubnick, 2002 pp. 7–9). In 1085 King William I of England demanded that all property holders count their possessions. The property of the holders was listed and valuated by agents of the king in what was known as the Domesday books (Bovens, 2005, p.182). This practice was intended to establish royal governance over everything within the kingdom. The landowners were made to swear allegiance to the king and this centralized administrative practice was carried out twice yearly. Interestingly, accountability has evolved from this sovereign act of authority in which subjects are accountable to the king, to a dynamic system in which public authorities are expected to possess and demonstrate accountability to citizens.
In the technologically-connected incidents of late, these relations of responsibility and obligation have become confused and perhaps even, conveniently blurred. When looking at the development of the idea of accountability, it may be surmised that perhaps the ultimate obligation and responsibility for actions and situations that unfold in relation to technology, business and people would be in the hands of public authorities. For, these are the organizations and agents that enable such systems and artifacts to exist and operate within specific communities and societies (Ulnicane et al., 2021). Part of the wicked problem in which these various social-technological discourses are unfolding is the interwoven nature in which the development is unfolding. For instance, sustainability and environmental issues are currently utilized as a trajectory for the infiltration of electric cars (Lazzeroni et al., 2021). Likewise, these electric cars are also a part of the same brand and company (i.e., Tesla) that are set to become a major player in the self-driving car scene. The argument for self-driving cars is safety (Nees, 2019), while also linking to electric car discourse and sustainable development ideology.
Learning Machinery, Intentionality, Emotionality and Potential Responsibility
From the outset, current electric cars on the market may not always be directly associated with self-driving vehicles. They may be considered “smart cars,” yet the intelligence stops at the seeming lack of direct fossil fuel usage and maybe even energy efficiency. However, a major dream or fantasy that is currently being physically and informationally manifested is that of learning machinery. For centuries humans have aspired to create machines and objects that think, and now it is finally happening (Murphy, 2021). Even back in Rene Descartes' time, the great mathematician and philosopher speculated over the possibilities for developing a thinking machine (Wheeler, 2008). It was through these philosophical workings that Descartes determined that the human mind could not be reduced to a mathematical equation (Hatfield, 2018). Likewise, centuries later, another mathematician Charles Sanders Peirce arrived upon the same observation—it is difficult if not impossible to harness the mind and human logic in its entirety as it is tainted and influenced by shades of moods and the effect of emotions (Beeson, 2008).
In order to really re-create a form of human intelligence or artificial general intelligence in machines there must be a cognitive-affective system (Pérez et al., 2016) that aggregates and processes information in meaningful and purposeful ways. These ways would be meaningful for the survival and concerns of the being (machine) that is processing and interpreting the information (Shah and Higgins, 2001). Thus, in order to understand learning to the full extent of its concept, “the acquisition of knowledge or skills through study, experience, or being taught” (Merriam-Webster Dictionary, 2021b), it also must be understood that learning is intentional and/or is intertwined with intentionality (Bereiter and Scardamalia, 2018) and indeed consciousness (Beyer, 2018). Whether or not an individual enters a situation with the plan of learning, experience provides new knowledge that acquired and assimilated to previously existing knowledge in a way that is shaped by already held “frames”—beliefs, experiences (narratives, events, scenarios), associated emotions (valence—negative-positive; arousal—activated-deactivated) that are guided by concerns for the preservation and wellbeing of the individual (Russell, 2009).
That is, driving these cognitive-affective processes of either appraisal (Roseman and Smith, 2001; Scherer, 2001) or core affect (Russell, 2009) is a form of self-awareness (Beyer, 2018), or consciousness that is motivated by the will to survive (Winkielman et al., 2005). Yet, cognitive-affective processing is no menial matter, in fact as may be determined by the article so far, imagining fully autonomous learning technology existing among humans involves incredible levels of complexity. Learning could be argued as being enabled via dynamic intentional structures that induce both primitive cognitive-emotional responses as well as higher order associative processes, which operate in interaction with one another at certain points in time (Husserl, 1999). The puzzle of exactly how this operates, and the challenges developers face when attempting to build learning, thinking machines (robots), is aptly captured by cognitive scientist Dennett (1984) in reference to the frame problem of AI. Although published in 1984, Dennett's version of the frame problem in AI still highlights the difficulty in not only predicting and explaining the full facets of human logic, but also the complex levels and multitude of options regarding interpretation and the possibilities of action. While emphasizing the complexity and trepidations of human logic and reasoning Dennett also demonstrates the epistemological dimension in which beliefs impede human's ability to act effectively. This is shown in the hypothetical situation of the robots R1D1, R1D2, R2D2 etc. who have the challenge of rescuing their own power supply (a battery) from a room in which a grenade is located (incidentally on the same wagon upon which the battery resides).
Likewise, another philosopher Jerry Fodor also uses robots as an example for the same problem by posing the question of, “How … does the machine's program determine which beliefs the robot ought to re-evaluate given that it has embarked upon some or other course of action?” (Fodor, 1983, p. 114). Interestingly, Dennett is famous for his critique of Fodor and scholarship on the qualia of consciousness in general, as he argues that literature supporting qualia's existence cannot be “neutrally” or objectively proven (Johnsen, 1997). In fact, Dennett advocated a method for studying experience, heterophenomenology, as a means to build a theory concerning mental events via the utilization of data from a third-person perspective. More to the point, qualia or the qualities of experience, are seen by Dennett as beliefs of what people feel they are mentally experiencing—mental images, pain, perception etc.—yet he argues that there can be no proof, thus no scientific validation that indeed these sensations, (a) exist and (b) are subjective.
Returning to the focus of the article, when talking about the development and existence of learning machinery in human society, and in particular of robots—any type of robot that has the ability to think and learn for itself—it must be understood that intentionality is a necessary component (Rousi, forth comming). Otherwise, the object or system, is not necessarily learning. Rather, the system is acquiring data through sensors that are then processed via programming logic (the input of programmers and how they arrange information through algorithms), which is then interpreted in ways that once again have been programmed. The interpretation does not take place by the robot itself, rather by the programmer and other developers who have decided on the operational logic (Johnson and Verdicchio, 2017). The reality of machines that acquire data, process it according to rules, which then guide the course of action and perhaps adaption, still rest in the hands and minds of the humans who create them (Müller, 2021). Unless, of course, technologists crack the code of consciousness—which on some levels, according to Dennett, they possibly can (however, with major difficulties and challenges), as consciousness from the qualia (quality of thought) perspective cannot be scientifically proven.
Let us suppose that truly intelligent, self-learning, conscious machines with intentionality actually would exist in human society. An extremely complicated area of governance in relation to complex systems becomes even more entangled2 when considering the fact that a machine is not purely a machine. Instead, when reflecting on the nature of human thought and logic, a machine possessing intentionality would also possess emotions. Consciousness, intentionality and live cognitive-affective processes would mean that while designers, developers and other technologists (including cognitive scientists) would have built the systems, these systems would also be truly autonomous. Or, as autonomous as we can expect an individual human being would be considering their socially and culturally conditioned (programmed) nature.3 Current hypes and initiatives including Saudi Arabia's issuing of citizenship to Sofia the Hansen Laboratories robot (Stone, 2017), may seem entertaining enough. Yet, if an artifact or system that is superior to human beings in terms of computation and physical performance, for instance, also was developed with the capability to emotionally experience, then it might be anticipated that incidents (accidents, crime and violence) involving robots and humans would certainly escalate (Rousi, 2018). From simple situations in which a robot experiences road rage or impatience, to moments in which the thinking and learning machine wanted to take revenge on the neighbor who chopped down its tree. There may even be a jealous robot, afraid that its partner is cheating with a human colleague. Questions of responsibility and accountability would become ever more prominent. Who will be responsible for the avenging android—the robot itself or its maker? For surely, in instances of learning machinery, there would not be an owner, would there?
Proposed Governance Model for LMS (AKA Robots) in Society
Research and development toward a governance model of AI is currently at its peak. Numerous groups and organizations are devoting resources toward understanding how and what elements must be present in the establishment of effective AI governance. Some recent governance models are seemingly straight-forward. Those such as AIbotics' Jean-Francois Gagné's (2021) “Framework for AI Governance” contain a scale of autonomy and human-dependency, from human-driven (watching to coaching) to AI-driven (collaborating to autonomous) technology in relation to stable dimensions of governance: (1) performance—accuracy, bias, completeness; (2) security—adaptability and adversarial robustness; (3) privacy—IP capture and impacted users; and (4) transparency—explainability and intent. These stable factors resonate with contemporary ethical AI principles, guidelines and methods (see e.g., Vakkuri et al., 2020; Agbese et al., 2021; Halme et al., 2021; IEEE's “Ethics in Action”).
There is also a “Layered Model for AI Governance (Gasser and Almeida, 2017) that presents a sandwich framework in which AI systems and society are posed as the two outer pieces of bread, while the inner layers comprise: (1) the technical layer of algorithms and data [bottom layer / near-term timing]—data governance, algorithm accountability and standards; (2) the ethical layer [middle layer / mid-term timing]; and (3) the social and legal layer [top layer / long-term timing]—norms, regulation and legislation. Another popular science style model by Senior Data Scientist, Laferrière (2020) posts “Trust” at the top of the framework. This is governed by the pillars of, “effective,” “compliant,” and “principled,” while being based on: data quality, data literacy, model performance, privacy, security, transparency & explainability, fairness, and human-in-control (human oversight).
Robotic Governance
This line of thought is carried forth when entering the domain of robotic learning technology, and particularly robot (robotic) AI governance. “Robotic governance” is a concept that describes an attempt to establish a regulatory framework enabling the handling of issues related to intelligent and autonomous machines (Asaro et al., 2015). Robotic governance is intended to guide and govern processes and activities that range from research and development to the ways in which humans treat the LMs (Boesl and Bode, 2016; Boesl and Liepert, 2016). Related concepts include corporate governance, AI governance, IT-governance and technology governance. Incorporated in robotic governance is a holistic view on robotics, AI and automation and their impact on global societies. It includes considerations for implications of the technology and its dynamics in relation to human beings, and is linked to what is known as the “Robot Manifesto” (Boesl et al., 2018). This manifesto is still in development and is facilitated by the Robotic & AI Governance Foundation.4
The governance framework presented in this current article has derived from research in discourse ethics. Discourse ethics is invested in communicative rationality, examining moral insight and expressions of normative validity (Habermas, 1990, 1991). Thus, communication is seen as key to building and sustaining ethical practice, it can also be witnessed in contemporary approaches to ethical design and ethical AI development (e.g., Baldini et al., 2018; Vakkuri et al., 2020; Agbese et al., 2021). In particular, this communicational factor is pronounced in some key facets of AI ethics—explainability, transparency and understandability. In other words, importance is placed on the development of AI systems that do not propone the black box of high-level complexity, that cannot be explained to laypeople through even careful (Confalonieri et al., 2021; Rousi, forth comming). Thus, human connection to and understanding of the workings of the AI-based technology is extremely important in terms of establishing an ethical basis of co-existence. Perceived understanding of phenomena, people and systems, increases the likelihood of trust (Saariluoma et al., 2018; Jakku et al., 2019). Trust is integral for any relationship and interaction, from human-to-human, to human-to-object, organization or system, and vice versa (Saariluoma et al., 2018).
While still in its infancy and quite obviously lacking in empirical evidence in ecologically valid scenarios, the Robot Manifesto (Boesl et al., 2018) is an early attempt to establish voluntary guidelines for self-regulation in fields ranging from research and development to sale, implementation and application. These guidelines cover the scope of opportunities and opportunity costs from a range of perspectives that include both technology-oriented matters to human-oriented and environmental issues. At this stage, awareness raising is a key goal of these types of initiatives as the “greater the public awareness and pressure with become concerning this topic, the harder it will get for companies to conceal or justify violations” (Boesl et al., 2018, n.p.). Thus, communication that enables transparency, increased public consciousness and understanding is seen as the key to ethical agency regarding special interest and activist groups and the general publics.
The Proposed Framework
Building a governance framework for a yet incompletely realized future on the basis of scholarship into governance of another type of emerging technology (AI) is no exercise based on accuracy and indeed full accountability. There will always be something missing and perhaps inaccurate when and if these systems and objects become a part of societal daily reality. Moreover, given constantly changing socio-technological conditions, no governance model will ever be complete in and of itself. They must always be treated as iterative “works-in-progress.” The current version of model in this present paper (see Figure 1) has been developed on the basis of models in AI governance. One framework in particular, the “Integrated AI Governance Framework” (Wirtz et al., 2020) intended to account for and anticipate the dark sides of AI, was chosen for adaption in the learning robotic context. In Anne Stenros' (2022) words, dark sides of intelligent robotics in respect to this article can be described as both the known unknowns (e.g., the ways in which LMs may be used for warfare, see for instance, Wagner, 2018 or Dujmovic, 2021 and their research on sexbotics) and the unknown unknowns, the unpredictable outcomes of self-renewing algorithms and their threats to security and safety (see e.g., Geer, 2019).
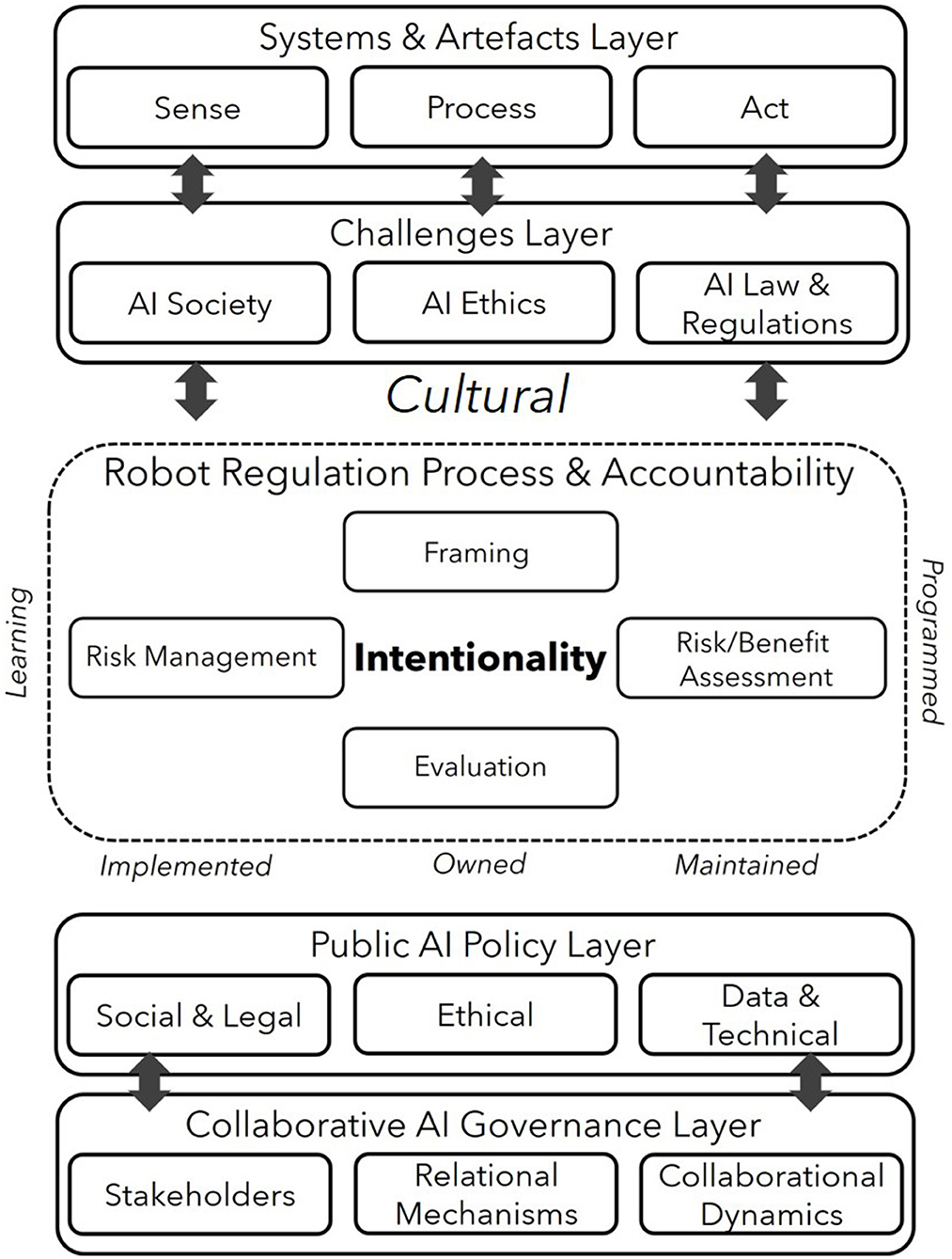
Figure 1. Ethical responsibility model for robot governance [ER-RoboGov; adapted from Wirtz et al. (2020), p. 818–829].
The Ethical Responsibility Model for Robot Governance (ER-RoboGov) has been built on the basic components of Wirtz et al. (2020) Integrated AI Governance Framework. The foundational elements of this framework comprise five main layers: (1) Systems and artifacts; (2) Challenges; (3) Public AI Policy; (4) Collaborative AI Governance; and at the center of the framework rests (5) AI regulation process and accountability. This central layer exists at the core of this article's argument of how can we regulate AI (LMs, intelligent robotics) and who is accountable for incidents that occur in which this technology rests at the cause? The following is an explanation of the components of the governance model.
Systems and Artifacts Layer
To elaborate on the framework and this current model adaptation, the first layer, the systems and artifacts layer, can be interpreted and dissected as the technical layer within the model. This is perhaps the most obvious and concrete element of the model. This particularly holds when considering empirical examples of the technology itself. These technological systems and artifacts can be evidenced and described through research and application in real life settings. Learning machinery, at its most basic level, can be characterized as being capable of sensing, processing and acting. Sensing and sensory perception from the human perspective, is also important within this layer, for it is the concrete technological product that most readily places an embodied understanding of what is in question, within the minds and discourse (communication) of humans. Semiotically the technology is both the object (that which is in question) and the signifying element (the symbolic nature of the technology itself, see e.g., Rousi, 2013). As mentioned earlier in the article, the ways in which human intelligence differs from AI systems and so-called learning machinery is the act of processing, rather than meaningful, assimilated and apperceived interpretation (Saariluoma, 2003; Rousi, 2013). Yet, these systems can take on a human-like resemblance in the response to sensing, which is acting. This is where it is difficult for humans to “compute” that machines do not possess a consciousness. For, when an object behaves somehow in relation to us, we generally endow it with human qualities, emotions and assumptions of consciousness5 (Nass and Yen, 2010).
Challenges Layer
The challenges layer is viewed in terms of AI in society and AI and society, AI ethics, AI law and regulations. AI in society is characterized by the challenges that have been described above in terms of the accountability and responsibilities concerns, as well as in terms of the true nature of AI—is it conscious and actually intentionally learning, or is it simply machinery that we know; sensing, processing, and acting? The nature of interactions and relationships with humans is additionally a major concern, both from the perspective of how a robot may eventually treat human beings6 (see Rousi, 2018) as well as how humans may in fact treat robots. It was Hiroshi Ishiguru who famously stated that what fascinates humans the most about robots, is what these technological entities teach us about what it means to be human (Barbican Center, 2019). It can therefore be assumed, and as hypothesized in popular cultural products such as the television series West World, that humans have the potential to become inhumane and immoral when given the chance to act out dark fantasies (see also Rousi, 2021). These dark behavioral patterns may not be seen as improving in terms of moral or ethical conduct toward other humans, rather, quite the opposite through de-sensitization7 and even gamification of harming others (Kim and Werbach, 2016). This leads to the question—as with any point of ethical, safety, security and privacy related discussions pertaining to human-technology relations—of whom humans should be more concerned about, technology, or other humans? Therefore, robot ethics is especially important as it incorporates insight of moral principles and codes of conduct from multiple angles.
Robot law and regulation taps into the preliminary ponderings for this article. Based on Isaac Asimov's (1950), “Three Laws of Robotics” followed by the fourth law, Zeroth Law “a robot may not harm humanity, or, by inaction, allow humanity to come to harm” (Asimov, 1985), careful consideration was given for the Second Law—“A robot must obey the orders given to it by human beings except where such orders would conflict with the First Law.” To interpret this, particularly through the lens of the short story I, Robot (Binder, 1939), the story that inspired Asimov that was about a sympathetic and emotional robot named Adam Link, robots should indeed be considered as slaves. This is said due to the details placed forth earlier in the article, that in order for a machine to actually be able to learn—really learn through integrating acquired information with previously acquired, interpreted and held knowledge—the machine must possess intentionality (the direction and goals of learning, what to learn and how). Intentionality is an integral part of consciousness (Searle and Willis, 1983; Chalmers, 1994), which therefore would be accompanied by emotions (Goldie, 2002; Fish, 2005). These elements lead to the main dilemma of applying for instance, Asimov's laws to the laws and regulations currently in development. For, if we understand that in order for the machines to be learning entities, we also must understand that they are conscious and emotions beings. Even if, the creation of human, this would mean that overall compliance, control and for that matter governance over these conscious artifacts (and systems) is a highly evolved for of slavery. In this day and age, and/or in the year 2030 or even 2040 for instance, would slavery actually be ethically acceptable?
With these dilemmas aside, the notion of robot law and regulation is heavily implicated in issues of responsibility and accountability. Who, at the end of the day, is responsible for the robot's actions? Is it the owner (master)? Or the creator—developer, designer, technologist's laboratory, corporate technology owner? Is it the law and policy makers? Or, is it the robot itself? And, if a human behaves in an inhumane way toward the robot, what rights does the robot have to justice? Will abuse and violence toward a robot be treated equally to that between human beings?
Public Robot Policy Layer
Skipping over the middle section of the framework for a moment, the public robot policy layer relates to the social and legal, as well as ethical, and data and technical layers of robot governance. This should, in a sense, be seen as more of a “straight forward” layer than the previous one of challenges. While featuring some of the same elements, the public robot policy layer, i.e., social and legal as well as ethical, this can be seen as the more formal component of the governance model. The social and legal elements relate to the citizen status of such machinery and their obligations to the community and society. If a robot possesses legal responsibilities and should be recognized as a social actor, then will they also possess rights and obligations as citizens, i.e., the right to vote? Or, the right to “healthcare” (maintenance services when components cease to function or become outdated) and insurance? Will they also earn salaries and pay taxes in the same way as humans do? This may seem counter-intuitive in a global economy that is motivated by the perceived “free labor” or “affordable labor” of automated services and machinery. But, if they are learning artifacts and systems—as stated before—they will have a consciousness, meaning that the acceptance of continuous “volunteer status” may not be a viable option. And, maybe, given the element of consciousness, humans could expect that robots with their supreme performance abilities, may even demand higher wages than imperfect human co-workers.
The ethical, in the case of this layer, are the guidelines set out in agreement with the status of robots in society. Ethical policy would cover humans and human rights, in relation to robots, as well as robots and robotic rights—both in relation to humans and other robots. The data and technical components pose quite a few challenges, and much revision of current privacy, data ownership (sovereignty, see e.g., Mühle et al., 2018) and security issues (Papernot et al., 2016) will need to be made. With these LMs roaming and operating autonomously in society, gathering data in a natural, or even supernatural (enhanced) way in comparison to humans, it is almost certainly that not every individual human they encounter and gather data from will and has been given the opportunity to give consent to data collection. Yet, unlike the minds of their human counterparts, it would also be assumed that there would be databases that humans can access in order to observe what the machines are collecting and how they are processing this information. For after all, humans will have developed the machinery and from this perspective, oversight would and should occur through some form of monitoring of their systems and internal logic. However, even the monitoring of data and technical operations pose ethical problems in relation to LMs. If they are autonomous learning entities in society then should not their privacy concerns be taken into consideration? It does seem unethical to expect that entities with greater performance requirements than humans would not be able to maintain privacy and the choice of disclosure to those they trust. This additionally leads to discussions on identity and the self-awareness of LMs, which will be explained in a later paper.
The collaborative robot governance layer adopts the stance that robots or LMs are governed and co-govern within societies. As LMs there would be the expectation that they possess the capacity, or maybe even superior capacity, to be able to participate in the collaborative governance of their actions and societal status. Numerous stakeholder groups will additionally be required for this collaborative effort including corporate and union representatives, law enforcement, health and other special interest groups. Collaborational dynamics are fostered and enhanced through a careful understanding of the relational mechanisms between the stakeholder groups, governance layers and even the pure technical logic of human-LM (human-robot) interaction.
How Will ER-RoboGov Work in Practice?
The ER-RoboGov model is intended as a scaffolding for understanding LMs (autonomous intelligent robots) in a societal systemic context. While it is intended to establish orientation within a public governance and policy setting, it can be applied within a range of other situations from organizational governance to design and manufacturing. ER-RoboGov illustrates the necessity for the design and development of LMs to be based on contractual societal agreement. Thus, before even the prototype level of development, companies and technologists should enter progressive discussions with stakeholders across a range of sectors. In particular, through the layer of Public Robot Policy, experts from fields across the board—ethics, sociology, law, health, psychology, design and engineering—should be engaged in deliberating codes of practice and requirements for the technology that locate it, its behavior and consequences in relation to those who should be held responsible and accountable. Experts from these disciplines should be gathered to provide input for each of the components represented in the model. This may be described as robot governance mapping in practice.
By undertaking deliberation sessions that place the characteristic of “intentionality” (learning through consciousness) at the center, and then mapping out the parameters of the technology (framing), risks vs. benefits, risk management and evaluation, strategies may be devised for not only the governance of the technology “in-the-wild” (in society), but for its very manufacturing. If one of the facets within the model either poses too many risks, or exhibits a significant level of unknown unknowns (in terms of any element of the model from stakeholders to collaborational dynamics), then from a responsible decision-making perspective it would not be wise to develop the technology in the first place. Or at least, the development should not move forward until the unknown unknowns have emerged as knowns, after a period of time, research and observation, and the information attached to each component of the governance model may be completed. This model takes accountability and responsibility into its sights already at the ideation phase, which calls for public governance activity that is continuously involved in interaction, and communication (discourse ethics) with the producers of the technology that shapes our societies, the companies driving this production and scientific communities. In terms of fully autonomous LMs, already at a glance and through light application of the governance model, these entities and systems pose too many known unknowns, and known unknown unknowns to be adequately governed within human societies.
The completed robot governance map that has been created for each robotic development project together with its strategy (in the form of a signed report) should be held as a contract between stakeholders on the governance of the developed technology. This will undoubtedly need updating as technology advances. Yet, clarity is needed already at the outset in terms of predicting potential actions, consequences and accountability relational chains and dynamics. The ER-RoboGov model is intended to discourage the usage of end-users as moral crumple zones, and instead, revert responsibility and accountability to those who have power in decision-making and guiding system logic.
Conclusion
This article has attempted to account for the complexity of ethical AI governance in the context or autonomous, learning robotics. The paper began by articulating the nature of learning, its intentionality, conscious and constructive nature, and how learning should be understood in a future during which learning machines, or LMs, will exist. LM differs to current understandings of ML, as the learning that occurs within the entity is meaningful from the perspective of the LM, or robot. This means that perceived information is assimilated into previously stored knowledge, being influenced by and further influencing the knowledge or mental models that the entity already possesses (Saariluoma, 2003; Helfenstein and Saariluoma, 2007). While this seems similar to contemporary models, the meaningful nature comes from the presence of consciousness and intentionality that drive and are driven by emotions (Chalmers, 1996; Chella et al., 2019). Hansen Robotics' Sofia may possess Saudi Arabian citizenship, but does it actually mean anything to her/it, particularly is she/it does not possess self-awareness or sense of conscious identity (identity of self in relation to others, see e.g., Ezzy, 1998)?
The world already faces extremely complicated challenges in terms of governing pervasive connected computing, especially in the domain of the Internet and social media. The prospect of developing governance models for autonomous learning machinery within the physical, geographical and social worlds of humans seems futile. Simply from the levels of design and development, programming and algorithms, ownership and even cross-platform operation of non-conscious ML there are striking problems of accountability and responsibility. When or if machinery that may really intentionally learn is developed and implemented in society a range of other mechanisms and models, particularly those for collaborative governance must be in check. Questions such as “will the robot be responsible for its own actions?” and even, “should we be developing fully autonomous technology in the first place?” should be thought of at this stage. In fact, during these moments of the eve of intelligent digital transformation, humans should recognize that this is the last opportunity to decide on the whether the existence of learning machinery is a good idea or not.
There are obvious limitations to the present article. As these issues are still not quite the reality, no empirical study was undertaken. Instead, current writings on robot and AI ethics and governance were referred to. Even current progress on robotic existence, the Robot Manifesto (Boesl et al., 2018) was drawn on to ascertain the current societal status on consideration for autonomous robotics. The proposed model, ER-RoboGov (Ethical Responsibility Model for Robot Governance), was developed on the basis of Wirtz et al.'s (2020) “Integrated AI Governance Framework.” The framework was chosen among others due to its relational detail through the layers of governance. It highlights the overlaps, particularly relating to ethics, and accentuates how these overlaps possess alternative meanings and associations depending on the perspective from which they are viewed. The model as it stands now is still not complete—neither Wirtz et al.'s model, nor ER-RoboGov (version 1.0), possess an adequate description of how the collaborative governance layer can by fully structured and implemented. Moreover, the data and technical layer, particularly of LMs remains problematic, not simply in terms of human oversight, control and responsibility regarding data collection, data handling and resulting actions, but also from the perspective of robot responsibility, robot ethics and privacy.
The model serves as a concrete starting point for considering the entities and their social-technical components in relation to ethical responsibility and accountability. There is no way of foretelling all the fundamental facets that need to be included within such a model until this technological science becomes a reality. Yet, it is critical already at this point to establish an understanding of the governance relationships and ultimate “stop points” for where accountability should and must be placed. When significant problems begin to arise from complex autonomous LMs in society, as seen contemporary narrow AI examples are already showing us8, who will claim the responsibility for this technology? Amazon's recruitment tools algorithms learned on the basis of data that was not simply collected and contained in one source, but from plentiful data bases that reflected biases on a collective level (Dastin, 2018). Will the responsibility for the robots' actions be in the hands of the development teams, their commissioners, their owners, the owners of organizations in which they are deployed, or will it be in the hands of global society as a whole? Or perhaps, as Hick (2013) has famously stated, “instead of blaming [ourselves] for something [we] cannot undo” we may let it define us. Even if we enter this ethical, governance and societal dilemma with clear intent.
Data Availability Statement
The original contributions presented in the study are included in the article/supplementary material, further inquiries can be directed to the corresponding authors.
Author Contributions
The author confirms being the sole contributor of this work and has approved it for publication.
Funding
This article has been partially funded by the Strategic Research Council (SRC), Academy of Finland through the ETAIROS project (Decision No. 327354), as well as the Sea4Value Fairway (S4VF) project funded by Business Finland (funding code 110/31/2020) and Stroke-Data project, and also funded by the Digital Economy Platform, University of Vaasa.
Conflict of Interest
The author declares that the research was conducted in the absence of any commercial or financial relationships that could be construed as a potential conflict of interest.
Publisher's Note
All claims expressed in this article are solely those of the authors and do not necessarily represent those of their affiliated organizations, or those of the publisher, the editors and the reviewers. Any product that may be evaluated in this article, or claim that may be made by its manufacturer, is not guaranteed or endorsed by the publisher.
Acknowledgments
The author of this article would like to thank the Digital Economy Platform as well as Communication Studies (School of Marketing and Communication) at the University of Vaasa, Finland, for their support. Likewise, gratitude goes to the Academy of Finland and the AI Ethics Research Group at the University of Jyväskylä StartUp Lab, led by Prof. Pekka Abrahamsson.
Footnotes
1. ^Code of Federal Regulations. 14 CFR 91.3.
2. ^‘Entanglement' is a concept that has been applied in sociology and subsequently design research, HCI and educational science to describe human's entangled nature, dependence and inseparability from systems of objects and systems of systems (see e.g., Sobe and Kowalczyk, 2017; Antczak and Beaudry, 2019; Frauenberger, 2019).
3. ^See Rousi and Alanen (2021) for more on the intertwined nature of the social and emotional in relation to human experience.
4. ^Robot and AI Governance Foundation. Available online at: https://www.roboticgovernance.com/ (accessed December 7, 2021).
5. ^For more on anthropomorphism or the human tendency to endow objects with feelings and emotions, please see Nass et al. (1993) and Kim and Sundar (2012).
6. ^Consider Isaac Asimov's (1950) “Three Laws of Robotics”: (1) A robot must not hurt a human or, through inaction, let a human be hurt; (2) a robot must obey orders delivered by humans unless these orders conflict with the First Law; and (3) a robot must protect its own existence to the extent that this protection does not conflict with the First and Second Laws.
7. ^This process in itself is complex as it involves a re-wiring, or extra ‘firing' of (mirror) neurons in the brain that while in the beginning, when first witnessing violence would be interpreted by the brain as ‘danger' or ‘inflicted pain', become ever more steadily automaticized (a common association with specific stimuli) that in the end results in a form of ignorance – the ability to not be affected by the violent acts unfolding in near proximity of the subject [see e.g., Kim and Werbach (2016)].
8. ^Jeffery Dastin (2018) discusses the example of Amazon's sexist recruiting tool and other AI governance problems have been discussed at the beginning of this present article.
References
Agbese, M., Alanen, H. K., Antikainen, J., Halme, E., Isomäki, H., Jantunen, M., et al. (2021). “Governance of ethical and trustworthy al systems: research gaps in the ECCOLA method,” in 2021 IEEE 29th International Requirements Engineering Conference Workshops (REW). IEEE, 224–229. doi: 10.1109/REW53955.2021.00042
Antczak, K. A., and Beaudry, M. C. (2019). Assemblages of practice. A conceptual framework for exploring human–thing relations in archaeology. Arch. Dia. 26, 87–110. doi: 10.1017/S1380203819000205
Asaro, P., Millar, J., and Thomasen, K. (2015). We Robot 2015 Conference – Robotic Governance Panel. University of Washington School of Law, Seattle, WA, 10. Available online at: https://robohub.org/werobot-2015-panel-3-robotics-governance-with-peter-asaro-jason-millar-kirsten-thomasen-and-david-post/ (accessed November 13, 2021).
Asimov, I. (1950). “Runaround”. I, Robot(The Isaac Asimov Collection ed). New York, NY: Doubleday. p. 40–54.
Baldini, G., Botterman, M., Neisse, R., and Tallacchini, M. (2018). Ethical design in the internet of things. Sci. Eng. Eth. 24, 905–925. doi: 10.1007/s11948-016-9754-5
Barbican Center (2019). Hiroshi Ishiguro: Are Robots a Reflection of Ourselves? 2019. Available online at: https://artsandculture.google.com/exhibit/hiroshi-ishiguro-are-robots-a-reflection-of-ourselves-barbican-centre/3AISlGQiWzL0Jw?hl=en (accessed November 3, 2021).
Beeson, R. (2008). Peirce on the Passions: The Role of Instinct, Emotion, and Sentiment in Inquiry and Action [PhD Thesis]. Tampa, FL: University of South Florida. Available online at” https://digitalcommons.usf.edu/cgi/viewcontent.cgi?article=1133andcontext=etd
Bereiter, C., and Scardamalia, M. (2018). “Intentional learning as a goal of instruction”, in Knowing, Learning, and Instruction, ed. L. B. Resnick (London: Routledge), 361–392. doi: 10.4324/9781315044408-12
Beyer, C. (2018). How to analyze (intentional) consciousness in terms of meta-Belief and temporal awareness. Front. Psych. 9:1628. doi: 10.3389/fpsyg.2018.01628
Boesl, D. B., and Bode, B. M. (2016). “Technology governance,” in 2016 IEEE International Conference on Emerging Technologies and Innovative Business Practices for the Transformation of Societies (EmergiTech), IEEE, 421–425. doi: 10.1109/EmergiTech.2016.7737378
Boesl, D. B., Bode, M., and Greisel, S. (2018). “Drafting a robot manifesto–new insights from the robotics community gathered at the European Robotics Forum 2018,” in 2018 27th IEEE International Symposium on Robot and Human Interactive Communication (RO-MAN), IEEE, 448–451. doi: 10.1109/ROMAN.2018.8525699
Boesl, D. B., and Liepert, B. (2016). “4 Robotic Revolutions-Proposing a holistic phase model describing future disruptions in the evolution of robotics and automation and the rise of a new Generation ‘R'of Robotic Natives,” in 2016 IEEE/RSJ International Conference on Intelligent Robots and Systems (IROS), IEEE, 1262–1267. doi: 10.1109/IROS.2016.7759209
Botsman, R. (2012). The Currency of the New Economy Is Trust. TED. Available online at: https://www.ted.com/talks/rachel_botsman_the_currency_of_the_new_economy_is_trust (accessed November 20, 2021).
Bovens, M., Goodin, R. E., and Schillemans, T., (eds.) (2014). The Oxford Handbook Public Accountability. Oxford: Oxford University Press. doi: 10.1093/oxfordhb/9780199641253.013.0012
Bovens, M. A. P. (2005). “From financial accounting to public accountability,” in Bestandsaufnahme und Perspektiven des Haushalts- und Finanzmanagements, ed H. Hill (Baden: Nomos Verlag), 183–193.
Carville, O. (2021). Airbnb Spends Millions Making Nightmares at Live Anywhere Rentals Go Away. Available online at: https://www.bloomberg.com/news/features/2021-06-15/airbnb-spends-millions-making-nightmares-at-live-anywhere-rentals-go-away (accessed November 20, 2021).
Chalmers, D. J. (1994). The Representational Character of Experience. Available online at: http://consc.net/papers/representation.pdf
Chalmers, D. J. (1996). The Conscious Mind: In Search of a Fundamental Theory. Oxford: Oxford Paperbacks.
Chella, A., Cangelosi, A., Metta, G., and Bringsjord, S. (2019). Consciousness in humanoid robots. Front. Rob. AI 6:17. doi: 10.3389/978-2-88945-866-0
Confalonieri, R., Weyde, T., Besold, T. R., and del Prado Martín, F. M. (2021). Using ontologies to enhance human understandability of global post-hoc explanations of black-box models. Artificial Intelligence 296:103471. doi: 10.1016/j.artint.2021.103471
Dastin, J. (2018). Amazon Scraps Secret AI Recruiting Tool that Showed Bias Against Women. Reuters. Available online at: https://www.reuters.com/article/us-amazon-com-jobs-automation-insight-idUSKCN1MK08G (accessed November 21 2021).
Day, P., and Klein, R. (1987). Accountabilities: Five Public Services (Vol. 357). Milton Park: Taylor and Francis.
Dennett, D. C. (1984). “Cognitive wheels: The frame problem of AI,” in Minds, Machines and Evolution ed. C. Hookway (Cambridge: Cambridge University Press), 129–151.
Dubnick, M. J. (2002). “Seeking salvation for accountability,” in Annual Meeting of the American Political Science Association, Vol. 29 (Boston, MA: American Political Science Association). Available online at: https://mjdubnick.dubnick.net/papersrw/2002/salv2002.pdf
Dujmovic, J. (2021). Opinion: Artificial Intelligence Has a Dark Side – Militaries Around the World Are Using It in Killing Machines. Available online at: https://www.marketwatch.com/story/artificial-intelligence-has-a-dark-side-militaries-around-the-world-are-using-it-in-killing-machines-11640107204 (accessed February 23, 2022).
Elish, M. C. (2019). Moral Crumple Zones: Cautionary Tales in Human-Robot Interaction:Engaging Science, Technology, and Society (Preprint). Available online at: https://papers.ssrn.com/sol3/papers.cfm?abstract_id=2757236
Ezzy, D. (1998). Theorizing narrative identity: symbolic interactionism and hermeneutics. Soc. Quart. 39, 239–252. doi: 10.1111/j.1533-8525.1998.tb00502.x
Félix, S. B., Pandeirada, J. N., and Nairne, J. S. (2019). Adaptive memory: Longevity and learning intentionality of the animacy effect. J. Cog. Psych., 31, 251–260. doi: 10.1080/20445911.2019.1586716
Fish, W. (2005). Emotions, moods, and intentionality. Intentionality 173, 25–35. doi: 10.1163/9789401202145_006
Flinders, M. (2017). The Politics of Accountability in the Modern State. London: Routledge. doi: 10.4324/9781315206950
Frauenberger, C. (2019). Entanglement HCI the next wave?. ACM Trans. Comp.-Hu. Interact. (TOCHI) 27, 1–27. doi: 10.1145/3364998
Gagné, J.-F. (2021). Framework for AI Governance. Available online at: https://jfgagne.ai/blog/framework-for-ai-governance/ (accessed December 7, 2021).
Gasser, U., and Almeida, V. A. (2017). A layered model for AI governance. IEEE Internet Comp. 21, 58–62. doi: 10.1109/MIC.2017.4180835
Geer, D. E. (2019). Unknowable unknowns. IEEE Security Privacy 17, 80–79. doi: 10.1109/MSEC.2019.2898636
Goldie, P. (2002). Emotions, feelings and intentionality. Phenom. Cog. Sci. 1, 235–254. doi: 10.1023/A:1021306500055
Habermas, J. (1990). Discourse ethics: Notes on a program of philosophical justification in Moral consciousness and communicative action, trans. C. Lenhardt and S. Weber Nicholsen (Cambridge, MA: MIT Press) 43–115.
Halme, E., Vakkuri, V., Kultanen, J., Jantunen, M., Kemell, K. K., Rousi, R., et al. (2021). How to Write Ethical User Stories? Impacts of the ECCOLA Method. In International Conference on Agile Software Development (Cham: Springer), 36–52. doi: 10.1007/978-3-030-78098-2_3
Hatfield, G. (2018). “René Descartes,” in The Stanford Encyclopedia of Philosophy, ed. Edward N. Zalta. Available online at: https://plato.stanford.edu/archives/sum2018/entries/descartes/ (accessed December 21 2021).
Heath, C. (2016). The Uber killer: The Real Story of One Night of Terror. GQ. Available online at: https://www.gq.com/story/the-uber-killer (accessed November 20, 2021).
Helfenstein, S., and Saariluoma, P. (2007). Apperception in primed problem solving. Cog. Proc. 8, 211–232. doi: 10.1007/s10339-007-0189-4
Hochman, M. (2020). The healthcare safety-net: time for greater transparency and accountability? J. Gen. Int. Med. 35, 983–984. doi: 10.1007/s11606-020-05667-8
Husserl, E. (1999). The Essential Husserl: Basic Writings in Transcendental Phenomenology. Bloomington, IN: Indiana University Press.
Jakku, E., Taylor, B., Fleming, A., Mason, C., Fielke, S., Sounness, C., et al. (2019). “If they don't tell us what they do with it, why would we trust them?” Trust, transparency and benefit-sharing in Smart Farming. NJAS-Wageningen J. Life Sci. 90:100285. doi: 10.1016/j.njas.2018.11.002
Jobin, A., Ienca, M., and Vayena, E. (2019). The global landscape of AI ethics guidelines. Nat. Mach. Intell. 1, 389–399. doi: 10.1038/s42256-019-0088-2
Johnsen, B. (1997). Dennett on Qualia and consciousness: a critique1. Can. J. Phil. 27, 47–81. doi: 10.1080/00455091.1997.10717473
Johnson, D. G., and Verdicchio, M. (2017). Reframing AI discourse. Min. Mach. 27, 575–590. doi: 10.1007/s11023-017-9417-6
Kim, T. W., and Werbach, K. (2016). More than just a game: ethical issues in gamification. Eth. IT. 18, 157–173. doi: 10.1007/s10676-016-9401-5
Kim, Y., and Sundar, S. S. (2012). Anthropomorphism of computers: is it mindful or mindless? Comp. Hum. Beh. 28, 241–250. doi: 10.1016/j.chb.2011.09.006
Koivula, A., Kaakinen, M., Oksanen, A., and Räsänen, P. (2019). The role of political activity in the formation of online identity bubbles. Pol. Internet 11, 396–417. doi: 10.1002/poi3.211
Laferrière, H. (2020). Artificial Intelligence Governance: An Operational Challenge 2020. Available online at: http://governmentanalytics.institute/magazine/december-2020/artificial-intelligence-governance-an-operational-challenge/ (accessed December 7, 2021).
Lazzeroni, P., Cirimele, V., and Canova, A. (2021). Economic and environmental sustainability of dynamic wireless power transfer for electric vehicles supporting reduction of local air pollutant emissions. Renew. Sustain. Energy Rev. 138:110537. doi: 10.1016/j.rser.2020.110537
Lerner, J. S., and Tetlock, P. E. (1999). Accounting for the effects of accountability. Psych. Bull. 125:255. doi: 10.1037/0033-2909.125.2.255
McCandless, H. E. (2002). A Citizen's Guide to Public Accountability: Changing the Relationship Between Citizens and Authorities. Bloomington, IN: Trafford Publishing.
Meisenzahl, M., and Canales, K. (2021). The 16 Biggest Scandals Mark Zuckerberg Faced Over the Last Decade as he Became One of the World's Most Powerful People. Business Insider. Available online at: https://www.businessinsider.com/mark-zuckerberg-scandals-last-decade-while-running-facebook-2019-12?r=USandIR=T#1-in-2013-a-whitehat-hacker-tried-to-report-a-security-bug-to-facebook-when-no-one-responded-he-hacked-mark-zuckerbergs-account-and-posted-the-bug-on-his-wall-1 (accessed December 10).
Merriam-Webster Dictionary (2021a). Accountability. Available online at: https://www.merriam-webster.com/dictionary/accountability (accessed November 21, 2021).
Merriam-Webster Dictionary (2021b). Learning. Available online at: https://www.merriam-webster.com/dictionary/learning (accessed November 17, 2021).
Mühle, A., Grüner, A., Gayvoronskaya, T., and Meinel, C. (2018). A survey on essential components of a self-sovereign identity. Comp. Sci. Rev. 30, 80–86. doi: 10.1016/j.cosrev.2018.10.002
Mulgan, R. (1997). The processes of public accountability. Aust. J. Pub. Admin., 56: 25–36. doi: 10.1111/j.1467-8500.1997.tb01238.x
Müller, V. C. (2021). Ethics of Artificial Intelligence and Robotics in The Stanford Encyclopedia of Philosophy. ed. E. N. Zalta. Available online at: https://plato.stanford.edu/archives/sum2021/entries/ethics-ai/ (accessed December 21 2021).
Murphy, R. R. (2021). Robots have grasped and manipulated the imagination since 1839. Sci. Rob. 6:eabi9227. doi: 10.1126/scirobotics.abi9227
Nass, C., Steuer, J., Tauber, E., and Reeder, H. (1993). “Anthropomorphism, agency, and ethopoeia: computers as social actors,” in INTERACT'93 and CHI'93 Conference Companion on Human Factors in Computing Systems, 111–112. doi: 10.1145/259964.260137
Nass, C., and Yen, C. (2010). The Man Who Lied to His Laptop: What We Can Learn About Ourselves From Our Machines. London: Penguin.
Nees, M. A. (2019). Safer than the average human driver (who is less safe than me)? Examining a popular safety benchmark for self-driving cars. J. Saf. Res. 69, 61–68. doi: 10.1016/j.jsr.2019.02.002
O'Madagain, C., and Tomasello, M. (2022). Shared intentionality, reason-giving and the evolution of human culture. Phil. Trans. R. Soc. B 377:20200320. doi: 10.1098/rstb.2020.0320
Papernot, N., McDaniel, P., Sinha, A., and Wellman, M. (2016). Towards the science of security and privacy in machine learning. arXiv preprint arXiv:1611.03814.
Pérez, J., Cerezo, E., Seron, F. J., and Rodriguez, L. F. (2016). A cognitive-affective architecture for ECAs. Bio. Insp. Cog. Arch. 18, 33–40. doi: 10.1016/j.bica.2016.10.002
Romzek, B. S., and Dubnick, M. (1998). “Accountability (Vol. 1),” in International Encyclopedia of Public Policy and Administration Volume 1, ed. J. M Shafritz (Boulder: Westview Press), 6–11.
Roseman, I. J., and Smith, C. A. (2001). “Appraisal theory,” in Appraisal Processes in Emotion: Theory, Methods, Research, eds K. R. Scherer, A. Schorr, and T. Johnstone (Oxford: Oxford University Press), 3–19.
Rousi, R. (2013). From cute to content: user experience from a cognitive semiotic perspective. Jyväskylä Stud. Comput. 171:Jyväskylä: University of Jyväskylä Press.
Rousi, R. (2018). Me, my bot and his other (robot) woman? Keeping your robot satisfied in the age of artificial emotion. Robotics 7:44. doi: 10.3390/robotics7030044
Rousi, R. (2021). “Ethical stance and evolving technosexual culture–a case for human-computer interaction,” in International Conference on Human-Computer Interaction (Cham: Springer), 295–310. doi: 10.1007/978-3-030-77431-8_19
Rousi R. (forth comming). “Will robots know that they are robots? The ethics of utilizing learning machines,” in International Conference on Human-Computer Interaction 2022 (Cham: Springer).
Rousi, R., and Alanen, H. K. (2021). “Socio-emotional experience in human technology interaction design–a fashion framework proposal,” in International Conference on Human-Computer Interaction 2021 (Cham: Springer), 131–150. doi: 10.1007/978-3-030-77431-8_8
Russell, J. A. (2009). Emotion, core affect, and psychological construction. Cog. Em. 23, 1259–1283. doi: 10.1080/02699930902809375
Saariluoma, P. (2003). “Apperception, content-based psychology and design,” in Human Behaviour in Design. (Berlin; Heidelberg: Springer), 72–78.
Saariluoma, P., Karvonen, H., and Rousi, R. (2018). “Techno-trust and rational trust in technology–a conceptual investigation,” in IFIP Working Conference on Human Work Interaction Design, eds J. Abdelnour-Nocera, B. R. Barricelli, T. Clemmensen, V. Roto, P. Campos, A. Lopes, and F. Gonçalves (Cham: Springer), 283–293. doi: 10.1007/978-3-030-05297-3_19
Saariluoma, P., and Rousi, R. (2020). “Emotions and technoethics,” in Emotions in Technology Design: From Experience to Ethics, eds R. Rousi, J. Leikas, and P. Saariluoma (Cham: Springer), 167–189.
Scherer, K. R. (2001). “Appraisal considered as a process of multilevel sequential checking,” in Appraisal Processes in Emotion: Theory, Methods, Research, eds K. R. Scherer, A. Schorr, and T. Johnstone (Oxford: Oxford University Press), 92–120.
Searle, J. R., and Willis, S. (1983). Intentionality: An Essay in the Philosophy of Mind. Cambridge: Cambridge University Press. doi: 10.1017/CBO9781139173452
Seo, H., and Faris, R. (2021). Comparative approaches to mis/disinformation| introduction. Int. J. Comm. 15:8. Available online at: https://ijoc.org/index.php/ijoc/article/view/14799
Shah, J., and Higgins, E. T. (2001). Regulatory concerns and appraisal efficiency: the general impact of promotion and prevention. J. Per. Soc. Psych. 80:693. doi: 10.1037/0022-3514.80.5.693
Shklovski, I., Mainwaring, S. D., Skúladóttir, H. H., and Borgthorsson, H. (2014). “Leakiness and creepiness in app space: perceptions of privacy and mobile app use,” in Proceedings of the SIGCHI Conference on Human Factors in Computing Systems (ACM), 2347–2356. doi: 10.1145/2556288.2557421
Smith, B. C. (2019). The Promise of Artificial Intelligence: Reckoning and Judgment. Cambridge, MA: MIT Press.
Smyth, S. M. (2019). The facebook conundrum: is it time to usher in a new era of regulation for big tech?. Int. J. Cyb. Crim. 13, 578–595. doi: 10.5281/zenodo.3718955
Sobe, N. W., and Kowalczyk, J. (2017). “Context, entanglement and assemblage as matters of concern in comparative education research,” in World Yearbook of Education 2018, eds J. McLeod, N. W. Sobe, and T. Seddon (London: Routledge), 197–204. doi: 10.4324/9781315363813-12
Somerville, H., and Shepardson, D. (2018). Uber car's ‘safety' Driver Streamed TV Show Before Fatal Crash: Police. Available online at: https://www.reuters.com/article/us-uber-selfdriving-crash/uber-cars-safety-driver-streamed-tv-show-before-fatal-crash-police-idUSKBN1JI0LB [Accessed November 20, 2021].
Stenros, A. (2022). Think Like a Futurist [workshop]. VME Interaction Design Environment. University of Vaasa, Finland.
Stone, Z. (2017). Everything You Need to Know About Sophia, the World's First Robot Citizen. Forbes. Available online at: https://www.forbes.com/sites/zarastone/2017/11/07/everything-you-need-to-know-about-sophia-the-worlds-first-robot-citizen/?sh=146e4e9f46fa (accessed December 10 2021).
Ulnicane, I., Knight, W., Leach, T., Stahl, B. C., and Wanjiku, W. G. (2021). Framing governance for a contested emerging technology: insights from AI policy. Pol. Soc. 40, 158–177. doi: 10.1080/14494035.2020.1855800
Vakkuri, V., Kemell, K. K., and Abrahamsson, P. (2020). “ECCOLA-a method for implementing ethically aligned AI systems,” in 2020 46th Euromicro Conference on Software Engineering and Advanced Applications (SEAA). IEEE, 195–204. doi: 10.1109/SEAA51224.2020.00043
Wagner, C. (2018). Sexbots: the ethical ramifications of social robotics' dark side. AI Matters 3, 52–58. doi: 10.1145/3175502.3175513
West, S. M. (2019). Data capitalism: redefining the logics of surveillance and privacy. Bus. Soc. 58, 20–41. doi: 10.1177/0007650317718185
Wheeler, M. (2008). “God's machines: Descartes on the mechanization of mind,” in The Mechanical mind in History, eds P. Husbands, O. Hollan, and M. Wheeler (Cambridge, MA: MIT Press), 307–330.
Wilner, A. S. (2018). Cybersecurity and its discontents: Artificial intelligence, the Internet of Things, and digital misinformation. Int. J. 73, 308–316. doi: 10.1177/0020702018782496
Winkielman, P., Berridge, K. C., and Wilbarger, J. L. (2005). “Emotion, behavior, and conscious experience: once more without feeling,” in Emotion and Consciousness, eds L. F. Barrett, P. M. Niedenthal, and P. Winkielman (New York City, NY: The Guilford Press), 335–362.
Wirtz, B. W., Weyerer, J. C., and Sturm, B. J. (2020). The dark sides of artificial intelligence: An integrated AI governance framework for public administration. Int. J. Pub. Admin. 43, 818–829. doi: 10.1080/01900692.2020.1749851
Keywords: robots, AI, governance, ethics, framework, machine learning, intentionality
Citation: Rousi R (2022) With Clear Intention—An Ethical Responsibility Model for Robot Governance. Front. Comput. Sci. 4:852528. doi: 10.3389/fcomp.2022.852528
Received: 11 January 2022; Accepted: 28 February 2022;
Published: 01 April 2022.
Edited by:
Anton Nijholt, University of Twente, NetherlandsReviewed by:
Galena Pisoni, University of Nice Sophia Antipolis, FranceAdamantios Koumpis, RWTH Aachen University, Germany
Copyright © 2022 Rousi. This is an open-access article distributed under the terms of the Creative Commons Attribution License (CC BY). The use, distribution or reproduction in other forums is permitted, provided the original author(s) and the copyright owner(s) are credited and that the original publication in this journal is cited, in accordance with accepted academic practice. No use, distribution or reproduction is permitted which does not comply with these terms.
*Correspondence: Rebekah Rousi, rebekah.rousi@uwasa.fi