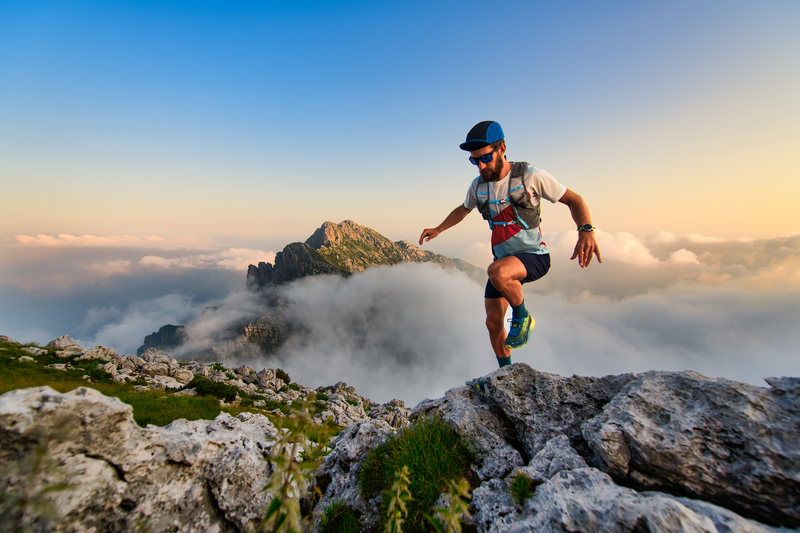
94% of researchers rate our articles as excellent or good
Learn more about the work of our research integrity team to safeguard the quality of each article we publish.
Find out more
ORIGINAL RESEARCH article
Front. Comput. Sci. , 28 January 2022
Sec. Human-Media Interaction
Volume 4 - 2022 | https://doi.org/10.3389/fcomp.2022.773256
This article is part of the Research Topic Recognizing the State of Emotion, Cognition and Action from Physiological and Behavioural Signals View all 11 articles
The Component Process Model is a well-established framework describing an emotion as a dynamic process with five highly interrelated components: cognitive appraisal, expression, motivation, physiology and feeling. Yet, few empirical studies have systematically investigated discrete emotions through this full multi-componential view. We therefore elicited various emotions during movie watching and measured their manifestations across these components. Our goal was to investigate the relationship between physiological measures and the theoretically defined components, as well as to determine whether discrete emotions could be predicted from the multicomponent response patterns. By deploying a data-driven computational approach based on multivariate pattern classification, our results suggest that physiological features are encoded within each component, supporting the hypothesis of a synchronized recruitment during an emotion episode. Overall, while emotion prediction was higher when classifiers were trained with all five components, a model without physiology features did not significantly reduce the performance. The findings therefore support a description of emotion as a multicomponent process, in which emotion recognition requires the integration of all the components. However, they also indicate that physiology per se is the least significant predictor for emotion classification among these five components.
Emotions play a central role in human experience by changing the way we think and behave. However, our understanding of the complex mechanisms underlying their production still remains incomplete and debated. Various theoretical models have been proposed to deconstruct emotional phenomena by highlighting their constituent features, as well as the particular behaviors and particular feelings associated with them. Despite ongoing disagreements, there is a consensus at least in defining an emotion as a multicomponent response, rather than a unitary entity (Moors, 2009). This conceptualization concerning the componential nature of emotion is not only central in appraisal theories (Scherer, 2009) and constructivist theories (Barrett et al., 2007), but also found to some extent in dimensional (Russell, 2009) and basic categorical models (Matsumoto and Ekman, 2009) that consider emotions as organized along orthogonal factors of “core affect” (valence and arousal), or as discrete and modular adaptive response patterns (fear, anger, etc.), respectively. Among these, appraisal theories, such as the Component Process Model (CPM) of emotion proposed by Scherer (1984), provide an explicit account of emotion elicitation in terms of a combination of a few distinct processes that evaluate the significance and context of the situation (e.g., relevance, novelty, controllability, etc.) and triggers a set of synchronized and interdependent responses at different functional levels in both the mind and body (Scherer, 2009). Hence, it is suggested that multiple and partly parallel appraisal processes operate to modify the motivational state (i.e., action tendencies such as approach, avoidance, or domination behaviors), the autonomic system (i.e., somatovisceral changes), as well as the somatic system (i.e., motor expression in face or voice and bodily actions). Eventually, synchronized changes in all these components—appraisal, motivation, physiology, and motor expression—may be centrally integrated in a multimodal representation (see Figure 1) that eventually becomes conscious and constitutes the subjective feeling component of the emotion (Grandjean et al., 2008).
Figure 1. Component model of emotion with five components. As suggested by Scherer (1984), it starts with an evaluation of an event (Appraisal component) which leads to changes in Motivation, Physiology and Expression components. Changes in all these four components modulate the Feeling component.
Because the CPM proposes to define an emotion as a bounded episode characterized by a particular pattern of component synchronization, whereby the degree of coherence among components is a central property of emotional experience (Scherer, 2005a), it offers a valuable framework to model emotions in computationally tractable features. Yet, previous studies often relied on physiological changes combined with subjective feeling measures, either in the perspective of discrete emotion categories (e.g., fear, anger, joy, etc.) or more restricted dimensional descriptors (e.g., valence and arousal) (see Gunes and Pantic, 2010). As a consequence, such approaches have generally overlooked the full componential view of emotion. On the other hand, studies inspired by the appraisal framework have often analyzed emotional response with linear analyses and simple linear models (Smith and Ellsworth, 1985; Frijda et al., 1989; Fontaine et al., 2013). Yet, based on the interactional and multicomponent account of emotions in this framework (Sander et al., 2005), non-linear classification techniques from the field of machine learning may be more appropriate and indeed provide better performances in the discrimination of emotions (Meuleman and Scherer, 2013; Meuleman et al., 2019). However, in the few studies using such approaches, classification analyses were derived from datasets depicting the semantic representation of major emotion words, but participants were not directly experiencing genuine emotions.
In parallel, while physiology is assumed to be one of the major components of emotion, the most appropriate channels of physiological activity to assess or to differentiate a particular emotion is still debated (see Harrison et al., 2013). For example, dimensional and constructivist theories do not assume that different emotions present specific patterns of physiological outputs (Quigley and Barrett, 2014) or argue that evidence is minimal for supporting specific profiles in each emotional category, spotlighting the insufficient consistency and specificity in patterns of activation within the peripheral and central nervous systems (Wager et al., 2015; Siegel et al., 2018). It has also been advocated that an emotion emerges from an ongoing constructive process that involves a set of basic affect dimensions and psychological components that are not specific to emotions (Barrett et al., 2007; Lindquist et al., 2013). Therefore, the modulation of autonomic nervous system (ANS) activity might be tailored to the specific demand of a situation and not to a discrete emotion. Peripheral physiological state occurring during a given emotion type is therefore expected to be highly variable in its physiological nature.
In contrast, some authors argue that measures of peripheral autonomic activity may contain diagnostic information enabling the representation of discrete emotions, that is, a shared pattern of bodily changes within the same category of emotion that becomes apparent only when considering a multidimensional configuration of simultaneous measures (Kragel and LaBar, 2013). Because univariate statistical approaches, which evaluate the relationship between a dependent variable and one or more experimental independent variables, have shown inconsistent results in relating physiology measures to discrete emotions (Kreibig, 2010), the development of multivariate statistical approaches to discriminate multidimensional patterns offers new perspectives to address these issues. By assessing the correlation between both dependent and independent variables and by jointly considering a set of multiple variables, multivariate analyses can reveal a finer organization in data as compared with univariate analyses where variables are treated independently. Accordingly, several recent studies used multivariate techniques and described separate affective states based on physiological measures including cardiovascular, respiratory and electrodermal activity (Christie and Friedman, 2004; Kreibig et al., 2007; Stephens et al., 2010). Such results support theoretical accounts from both basic (Ekman, 1992) and appraisal models (Scherer, 1984) suggesting that information carried in autonomic responses is useful to distinguish between emotional states. In this view, by using the relationships between multiple physiological responses in different emotional situations, it should be possible to infer which emotion is elicited. However, empirical evidence suggests that it is still complicated to figure out from patterned physiological responses, whether ANS measures are differentiated among specific emotion categories or more basic dimensions (Mauss and Robinson, 2009; Quigley and Barrett, 2014). Moreover, it is often observed that self-reports of emotional experience discriminate between discrete emotions with a much better accuracy than autonomic patterns (Mauss and Robinson, 2009).
In sum, there is still no unanimous conclusion about distinguishable patterns of activation in ANS, due to the difficulty to identify and associate reliable response patterns to discrete emotions. As a consequence, the debate is not closed concerning the functionality of physiology during an emotional experience. Based on the CPM model, physiology is involved in shaping emotion and can contribute to differentiating emotion. However, while relevant, we hypothesize that the use of physiology alone is limited in discriminating emotion but could be better understood if integrated with the other major components of emotion. Therefore, to provide further insights about the contribution of physiology in emotion differentiation, we propose here to examine how a full componential model can account for the multiple and concomitant changes in physiological and behavioral measures observed during emotion elicitation. In addition, we examine the added information by each component and hypothesize that considering the synchronized changes in all components, the information in each component is already encoded in the other components. To the best of our knowledge, the present work represents one of the first attempts to investigate the componential theory by explicitly considering a combination of multiple, theoretically defined, emotional processes that occur in response to naturalistic emotional events (from cinematic film excerpts). By deploying a data-driven computational approach based on multivariate pattern classification, we aim at performing detailed analyses of physiological data in order to distinguish and predict the engagement of different emotion components across a wide range of eliciting events. On the grounds of such multicomponent response patterns, we also aim at determining to what extent discrete emotion categories can be predicted from information provided by these components, and what is the contribution of each component in such predictions. We hypothesize that a multicomponent account, as proposed by the CPM (Scherer, 1984, 2009), may allow us to capture the variability of physiological activity during emotional episodes, as well as their differentiation across major categories of emotions.
Assuming that a wide range of emotional sequences will engage a comprehensive range of component processes, we selected a number of highly emotional film excerpts taken from different sources (see below). Physiological measures were recorded simultaneously during the initial viewing of movie clips, with no instructions other than be spontaneously absorbed by the movies. Participants were asked, during a second presentation, to fill out a detailed questionnaire with various key descriptors of emotion-eliciting episodes derived from the componential model (i.e., CoreGRID items) that assess several dimensions of appraisal, motivation, expression, physiology, and feeling experiences (Fontaine et al., 2013). We then examined whether the differential patterns of physiological measures observed across episodes could be linked to a corresponding distribution of ratings along the CoreGRID items, and whether the combined assessment of these items and physiological measures could be used together to distinguish between discrete emotions.
A total of 20 French-speaking and right-handed students (9 women, 11 men) between 19 and 25 years old (mean age = 20.95, SD = 1.79) took part in the main study. All of them reported no history of neurological or psychiatric disorder, gave a written informed consent after a full explanation of the study and were remunerated. One participant completed only 2 sessions out of 4, but the data collected were nevertheless included in the study. This work was approved by Geneva Cantonal Research Committee and followed their guidelines in accordance with Helsinki declaration.
To select a set of emotionally engaging film excerpts which could induce variations along different dimensions of the component model, a first preliminary study was conducted in separate study (for more details, see Mohammadi and Vuilleumier, 2020; Mohammadi et al., 2020). We selected a set of 139 film clips from the previous literature on emotion elicitation, matching in terms of time and visual quality (Gross and Levenson, 1995; Soleymani et al., 2009; Schaefer et al., 2010; Gabert-Quillen et al., 2015). Emotion assessment was collected in terms of discrete emotion labels and componential model descriptors. Initially, clips were evaluated over 14 discrete emotions (fear, anxiety, anger, shame, warm-hearted, joy, sadness, satisfaction, surprise, love, guilt, disgust, contempt, calm) based on a modified version of the Differential Emotion Scale (McHugo et al., 1982; Izard et al., 1993). For the component model, 39 descriptive items were selected from the CoreGRID instrument, capturing emotion features along the five components of interest: appraisal, motivation, expression, physiology, and feeling (Fontaine et al., 2013). This selection was performed based on the applicability to emotion elicitation scenarios while watching an event in a clip. The study was performed on Crowdflower, a crowdsourcing platform, and a total number of 638 workers participated. Based on average ratings and discreteness, 40 film clips were selected for this study (for more details, see Mohammadi and Vuilleumier, 2020). Shame, warm-hearted, guilt and contempt were excluded from the list of elicited emotions because no clips received high ratings for these four emotions.
Finally, another preliminary study was conducted to isolate the highest emotional moments in each clip. To this aim, five different participants watched the full clips and rated the emotional intensity of the scene using CARMA, a software for continuous affect rating and media annotation (Girard, 2014). The five annotations were integrated to find the most intense emotional events in each time series.
The final list of film excerpts was thus represented by 4 clips for each of the 10 selected discrete emotions, with a total duration of 74 min (average length of 111 seconds per clip). Moreover, between 1 and 4 highly emotional segments of 12 seconds were selected in each film excerpt, for a total of 119 emotional segments. The list of the 40 selected films in our final dataset is presented in Supplementary Table S1. The duration, the initially assigned emotion label, and the number of highly emotional segments are indicated for each film excerpt.
The whole experiment consisted of four sessions scheduled on different days. Each session was divided into two parts, fMRI experiment and behavioral experiment, lasting for about 1 and 2 h, respectively. In the current study we focus only on the behavioral analysis and will not use the fMRI data. Stimuli presentation and assessment were controlled using Psychtoolbox-3, an interface between MATLAB and computer hardware.
During the fMRI experiment, participants were engaged in an emotion elicitation procedure using our 40 emotional film excerpts. No explicit task was required during this phase. They were simply instructed to let themselves feel and express emotions freely rather than controlling feelings and thoughts because of the experiment environment. Movies were presented inside the MRI scanner on an LCD screen through a mirror mounted on the head coil. The audio stream was transmitted through MRI-compatible earphones. Each session was composed of 10 separate runs, each presenting a film clip preceded by a 5-seconds instruction screen warning about the imminent next display and followed by a 30-seconds washout periods introduced as a low-level perceptual control baseline for the fMRI analysis (not analyzed here). Moreover, a session consisted of a pseudo-random choice of 10 unique film clips with high ratings on at least one of the 10 different pre-labeled discrete emotion categories (fear, anxiety, anger, joy, sadness, satisfaction, surprise, love, disgust, calm). This permitted to engage potentially different component processes in every session. To avoid any order effect, the presentation of all stimuli was counterbalanced.
The behavioral experiment was performed at the end of each fMRI session, in a separate room. Participants were let alone with no imposed time constraints to complete the assessment. They were asked to rate their feelings, thoughts, or emotions evoked during the first viewing of the film clips and advised not to report what might be expected to feel in general when watching such kinds of events. To achieve the emotion evaluation, the 10 film excerpts seen in the preceding session were presented on a laptop computer with LCD screen and headphones. However, the previously selected highly emotional segments (see “stimuli selection” above) were now explicitly highlighted in each film excerpts by a red frame surrounding the visual display. In order to ensure that emotion assessment corresponded to a single event and not the entire clip, the ratings were required right after each segment by pausing the clips. The assessment involved a subset of CoreGRID instrument (Fontaine et al., 2013), which is to date the most comprehensive attempt for multi-componential measurement in emotion. The set of 32 items (see Table 1) had been pre-selected based on their applicability to the emotion elicitation scenario with movies, rather than according to an active first-person involvement in an event. Among our set of CoreGRID items, 9 were related to the appraisal component, 6 to the expression component, 7 to the motivation component, 6 to the feeling component, and 4 to the bodily component. Participants had to indicate how much they considered that the description of the CoreGRID items correctly represented what they felt in response to the highlighted segment, using a 7-level Likert scale with 1 for “not at all” and 7 for “strongly.”
Thus, each participant had to complete 119 assessments corresponding to 119 emotional segments. All responses were collected through the keyboard, for a total of 3,808 observations per participant (32 items × 119 emotional segments). Finally, they were also asked to label the segments by selecting one discrete emotion term from the list of 10 emotion categories. Therefore, the same segment may have been classified by participants into different emotion categories, and differently from the pre-labeled category defined during the pilot phase (where ratings were made for the entire film clip). In this study, we always used the subjectively experienced emotions reported by the participants as ground-truth labels for subsequent classification analyses. The frequency histogram showing the categorical emotions selected by the participants is presented in Supplementary Figure S1.
A number of physiological measures were collected during the first part of each session in the MRI scanner, including heart rate, respiration rate, and electrodermal activity. All the measures were acquired continuously throughout the whole scanning time. The data were first recorded with a 5,000 Hz sampling rate using the MP150 Biopac Systems software (Santa Barbara, CA), before being pre-processed with AcqKnowledge 4.2 and MATLAB 2012b.
Heart rate (HR) was recorded with a photoplethysmogram amplifier module (PPG100C). This single channel amplifier designed for indirect measurement of blood pressure was coupled to a TSD200-MRI photoplethysmogram transducer fixed on the index finger of the left hand. Recording artifacts and signal losses were corrected using endpoint function from AcqKnowledge, which interpolates the values of a selected impaired measure portion. Secondly, the pulse signal was exported to MATLAB and downsampled to 120 Hz. To remove scanner artifacts, a comb-pass filter was applied at 17.5 Hz. The pulse signal was then filtered with a band-pass filter between 1 and 40 Hz. Subsequently, the instantaneous heart rate was computed by identifying the peaks in the pulse signal, calculating the time intervals between them and converting this distance into beats per minute (BPM). The standard heart rate in humans goes from 60 to 100 bpm at rest. Hence, it was considered that a rate above 100 bpm was unlikely and the minimum distance between peaks will not exceed this limit. This automatic identification was manually verified by adding, changing or removing the detected peaks and possible outliers.
Respiration rate (RR) was measured using a RSP100C respiration pneumogram amplifier module, designed specifically for recording respiration effort. This differential amplifier worked with a TSD201 respiration transducer, which was attached with a belt around the upper chest near the level of maximum amplitude in order to measure thoracic expansion and contraction. Using a similar procedure as for HR preprocessing, the connect endpoint function of AcqKnowledge was first employed to correct manually the artifacts and losses of signal. After exporting the raw signal to MATLAB, it was downsampled to 120 Hz and then filtered with a band pass filter fixed between 0.05 and 1 Hz. Lastly, the signal was converted to breaths per minute using the same procedure as above. The standard respiration rate in human goes from 12 to 20 breaths per minute at rest. Since participants were performing a task inside a scanner which could be an unusual environment, the higher maximum rate was increased at 35 cycles per minute. Therefore, it was estimated that a rate above 35 was unlikely and the minimum distance between peaks will not exceed this limit. Again, this information was used in the automatic detection of the signal peaks. The respiration rate was then manually verified by looking at the detected signal peaks and corrected, with outliers being removed when it was necessary.
Electrodermal activity (EDA) was registered using an EDA100C electrodermal activity amplifier module, a single-channel, high-gain, differential amplifier designed to measure skin conductance via the constant voltage technique. The EDA100C was connected to Adult ECG Cleartrace 2 LT electrodes. Electrodes were placed on the index and the median fingers of the participants left hand. Following the manual correction of artifacts and losses of signal with the connect endpoint function on AcqKnowledge, the raw signal was exported to MATLAB. Similar to the two other physiological signals, the EDA signal was downsampled to 120 Hz. This signal, recorded by BIOPAC in microSiemes (μS), was then filtered with a 1 Hz low pass filter. An IIR (infinite impulse response) high-pass filter fixed at 0.05 Hz was applied to derive the Skin Conductance Responses (phasic component of EDA) representing the rapidly changing peaks, while a FIR (finite impulse response) low-pass filter fixed at 0.05 Hz was applied to derive the Skin Conductance Levels (tonic component of EDA) corresponding to the smooth underlying slowly-changing levels (AcqKnowledge 4 Software Guide, 2011).
MATLAB was used to select physiological values during the 12-s duration of high emotional segments. From these values, the means, variances, and ranges of each physiological signals (HR, RR, phasic and tonic EDA) were calculated. We chose to focus specifically on the mean and variance of these physiological signals, as these are the most reliable and frequently reported features in studies associating discrete emotions and physiological responses (Kreibig, 2010). For HR and RR measures, respectively 4 and 17 responses during highly emotional segments had to be removed in one participant due to a corrupted signal, potentially induced by movements. For EDA, 267 values had to be removed due to temporary losses of signal, resulting in flat and useless measures. In particular, EDA responses of two subjects were completely removed as the EDA sensor could not capture their response. In order to handle the missing values in the physiological data, dropouts were replaced by mean value of the whole session during which the signal loss has happened (i.e., missing value imputation). Furthermore, the variance in physiological responses could be very large and different across participants. Because it was particularly important to reduce such variability in order to avoid inter-individual biases, all physiological measures were normalized within-subject using RStudio (1.1.383). To achieve this, standardized z-scores were calculated from the physiological data during the 4 sessions of each participant.
Regarding responses collected for the 32 CoreGRID items for each high emotional segment, a within-subject normalization into z-score was also performed. These normalized behavioral data and the discrete emotion labels selected by the participants for each emotional segment were incorporated to the related physiological measures. In the end, for each of the 119 emotional segments, we obtained a set of observations including 32 standardized CoreGRID items and 1 discrete emotion label, as well as 8 standardized physiological values calculated offline. However, the final dataset included 19 participants who attended all 4 sessions (19 x 119 = 2,261), while 1 participant completed 2 sessions out of 4 (1 × 55 = 55). Also, for 11 participants, the assessment of one of the emotional segments did not get recorded due to a technical issue. Therefore, in total, there were (2,261 + 55 – 11 =) 2,305 sets of observations instead of the possible maximum of (20 × 119 =) 2,380 (~3% of points loss).
To investigate the relationship between physiology and the component model descriptors, two analyses were performed. First, we examined whether the physiology measures allowed predicting component model descriptors and vice versa. Second, we assessed whether distinct features from the componential model allowed predicting discrete emotion categories and compared the value of different components for this prediction. For both analyses, multivariate pattern classifications using machine learning algorithms were undertaken to predict the variables of interest. Linear and non-linear classifiers including Logistic Regression (LR) and Support Vector Machine (SVM) with different kernels (linear, radial basis function, polynomial and sigmoid) were applied. All analyses were carried out using the RStudio statistical software, Version 1.1.383. Logistic regressions were conducted with the “caret” package, Version 6.0 and multinomial logistic regressions with the “nnet” package, Version 7.3. The binary and multiclass classifications using Support Vector Machine were conducted with the “e1071” package, Version 1.7.
First, the CoreGRID items were used as predictor variables to predict the dependent variable, which was either the mean or the variance of each physiological measure. To enable such analyses and to simplify the computational problem, the scores of the dependent variable were converted into two classes of “High” and “Low” using the median value across all the participants as a cutoff threshold. LR and SVM with linear and non-linear kernels using 10-fold cross-validation were applied. To guarantee test and training independence, each participant's assessment was included in either a test set or a training set. Conversely, similar analyses were carried out to determine whether physiology measures could encode the component model descriptors, but now using the physiology measures as independent variables in an attempt to predict the ratings of each CoreGRID item as either above or below the median.
Secondly, to examine the relationship between the component process model and discrete emotion types, multiclass classifications using SVM were performed on different combinations of CoreGRID items and physiological measures in order to predict specific emotion categories as labeled by the participants. Given the large number of classes and limited number of samples per class with too many predictors, a leave-one-subject-out cross-validation was used to guarantee a complete independence between the training and testing datasets.
For all analyses, we used a grid search method to optimize the parameters, but no significant improvement was observed, so the default parameters were kept. Moreover, SVM with radial basis function (RBF) kernel outperformed LR and other SVM models. Therefore, we will only report the result from SVM with RBF kernel.
It should be mentioned that the classes used for binary classifications were pretty balanced since they were defined based on the median value of the dependent variable, resulting in a distribution close to 50–50 split. However, in the case of multi-class classification, although the number of movie clips for each pre-labeled emotion category was balanced, the final dataset was not since we used the subjectively experienced emotions reported by each participant as emotional labels. This imbalance may have slightly affected the classification performance for some under- or over-represented categories. To account for an effect of class distribution, we reported the chance level in all comparisons as well as the confusion matrix.
Our first predictive analyses aimed to assess classification based on multivariate patterns using either physiology measures or CoreGRID items for different emotional movie segments. The variables to be discriminated were treated as binary dependent variables (High vs. Low), the classes being defined with respect to the median value. One of the main assumptions of the CPM is that emotions rely on interdependent and synchronized changes within the five major components, suggesting that changes in any component might partly result from or contribute to changes in other components. Therefore, our initial exploratory analyses intended to examine whether physiological data (which represent an objective proxy to some of the CoreGRID items related to the physiology component) can be predicted using the CoreGRID items (which evaluate the five components of emotions), and vice versa. In other words, the idea was to investigate whether physiological changes are encoded in other emotional components. Based on the CPM, we expected to observe that physiological responses could predict not only physiology-related CoreGRID items, but also the items assessing the other components.
In the first instance, we deployed SVM classifications with 10-fold cross-validation to predict each physiological measure (mean or variance) as high or low from responses to the 32 CoreGRID items. Cross validation was applied to evaluate the generalizability of the results to some extent. This classifier yielded accuracies significantly greater than the chance rate of 50% for all physiological measures (Table 2). However, while these binary classifications were statistically significant and effect sizes were large, their discriminative performance remained weak (on average, about 58% of correct responses).
Conversely, using the same classification approach, the ratings of each CoreGRID item were predicted from the combination of physiological measures and physiology items in the CoreGRID questionnaire. Results from SVM showed that a majority of the CoreGRID items could be predicted significantly better than the chance level (Table 3), even though the classification accuracies were still relatively low (on average, about 55% of correct responses). The most reliable discrimination levels (highest t values relative to chance rate) were observed for appraisals and feelings of unpleasantness (55% of correct responses) as well as action tendencies (want to destroy / to do damage, 58% of correct responses).
Our second and main aim was to investigate whether discrete emotion categories as indicated by the participants can be predicted from ratings of their componential profiles. Here, we wanted to test whether the discrimination of discrete emotions is supported by one particular component (e.g., the appraisal component), distributed (equally) across the components, or requires the full combination of all components. The first step was to test how the entire data (physiological measures and behavioral responses) could predict discrete emotion labels. This more global pattern analysis for a multiclass variable required to go further than simple binary classification. To achieve this, a multiclass SVM classifications with leave-one-subject-out cross-validation was performed, taking the combination of within-subject normalized mean and variance measures from the four physiological signals and all behavioral responses to the CoreGRID items for the five emotional components as predictors. Applying the SVM classifiers (generated with training datasets) on separate testing datasets, we obtained an average accuracy rate of 45.4% in comparison to a rate of 17.6% for the chance level [t(19) = 10.852, p < 0.001, Cohen's d = 3.41, 95% CI (1.75 5.06)] (Figure 2A).
Figure 2. (A) Predictions of discrete emotion labels from physiological measures and responses to the 32 CoreGRID features. Cross-subject multiclass SVM classification with leave-one-subject-out cross-validation. Accuracy rate represents the percentage of correct classifications. The error bars show the standard deviation. Paired t-tests were conducted to assess significant differences between SVM classifier and chance level, as highlighted by asterisks indicating the p-value (***p < 0.001). (B) Confusion matrix of emotion labels. The diagonal running from the lower left to the upper right represents the correct predicted emotion. (C) Statistical measures of classification performances across emotions. Average of statistical measures assessing the performances from the 20 classifiers. The error bars show the standard deviation.
The confusion matrix showed that five emotion categories (anger, calm, sadness and surprise) were correctly predicted more than half of the times, with an accuracy range from 55 to 59.4% (Figure 2B). By contrast, predictions were extremely unsuccessful for fear (misclassified as anxiety) and satisfaction (misclassified as joy or calm). However, it is worth noticing that these categorical emotions had a smaller number of instances since they were less often selected by the participants (see Supplementary Figure S1). Interestingly, incorrect predictions for these two emotions were still related to some extent to the target category. Indeed, mainly anxiety but also disgust and surprise were predicted instead of fear, whereas joy and calm were predicted instead of satisfaction. Love was also frequently misclassified as joy and calm.
Concomitantly, statistical measures allowing the assessment of prediction performance indicated that specificity and negative predictive value were particularly high for all emotions (Figure 2C). This suggests that the classification algorithm had a notable ability to correctly reject observations that did not belong to the emotion of interest, that is, to provide a good degree of certainty and reliability for true negatives. In contrast, sensitivity and positive predictive value were not as good and fluctuated substantially across the emotions, with the best performance for calm and the worst for fear and satisfaction (Figure 2C).
The second step consisted in investigating the added information brought by each component in the classification performance. To better identify the relation between discrete emotions and interactions of different component processes, we began by examining the effect on overall performance when one component was excluded. Five multiclass SVM classifications with leave-one-subject-out cross-validation were performed using different combinations of these components (Figure 3). In comparison to the accuracy rate of the complete model using physiology and all the CoreGRID items (45.4%), the accuracy rate of the reduced model without the body physiology component (4 CoreGRID items and all physiological measures) was lower but did not significantly change [45.2%, t(19) = −0.17, p = 0.866, Cohen's d = −0.01, 95% CI (−0.13 0.11)]. These results suggest that information coming from features of the body physiology component in our study may have already been encoded in other components. On the other hand, with respect to performance with the full model, reduced models without the appraisal component [41.4%, t(19) = −4.598, p < 0.001, Cohen's d = −0.33, 95% CI (−0.48 −0.18)], without the expression component [41.9%, t(19) = −4.358, p < 0.001, Cohen's d = −0.29, 95% CI (−0.43 −0.15)], without the motivation component [43%, t(19) = −2.489, p = 0.022, Cohen's d = −0.21, 95% CI (−0.37 −0.03)] or without the feeling component [44%, t(19) = −2.349, p = 0.029, Cohen's d = −0.12, 95% CI (−0.22 −0.02)] were statistically less predictive, even though the effects sizes remained relatively small.
Figure 3. Emotion classification using one-component-out models. Cross-subject multiclass SVM classifications with leave-one-subject-out cross-validation. Accuracy rate represents the percentage of correct classifications. The error bars show the standard deviation. Paired t-tests have been conducted and significant differences between SVM classifier and chance level are highlighted (asterisks indicate the p-value: ***p < 0.001).
In addition, we examined the specific contribution in emotion classification of all major components. These contributions were assessed by predicting discrete emotion labels from each component separately. Five multiclass SVM classifications with leave-one-subject-out cross-validation were performed from the appraisal, expression, motivation, feeling, and body components (body items and all physiological measures). Since prediction performance from each of the five emotion components yielded accuracies significantly greater than the chance rate of 17.6% (Figure 4), we also analyzed the average sensitivity rate across the different classifiers in order to determine more precisely the power of each component to distinguish the different discrete emotions.
Figure 4. Emotion classification using the components independently. Cross-subject multiclass SVM classifications with leave-one-subject-out cross-validation. Accuracy rate represents the percentage of correct classifications. The error bars show the standard deviation. Paired t-tests have been conducted and significant differences between SVM classifier and chance level are highlighted (asterisks indicate the p-value: **p < 0.01, ***p < 0.001).
While the results above suggested that the percentage of correct predictions was generally similar regardless of the particular component used to train the classifiers, these additional analyses indicate that the pattern of features from specific components may yield a more reliable detection of particular emotions relative to others (Figure 5A). Moreover, it appeared also that some emotion classes were consistently well-discriminated by all components (e.g., calm and sadness), while others (fear, love, and satisfaction) were poorly predicted by any component. Conversely, some components could have more importance for particular emotions (e.g., surprise is well-predicted by appraisal features but not by the combination of body and physiological features, while motivation features seem best at predicting anger and joy).
Figure 5. (A) Sensitivity of emotion detection using the components independently. (B) Sensitivity of emotion detection using body and physiology components separately. Sensitivity rate represents the average of the sensitivity measures for each emotion label across the leave-one-subject-out cross-validation. The error bars show the standard deviation.
Furthermore, since we observed that the body and physiology component was the least effective in discriminating discrete emotions, we also examined the sensitivity rates for each emotion and compared the performance of models using either the body-related CoreGRID items (i.e., subjective ratings), the physiological measures (i.e., objective recordings), or both information (Figure 5B). Consistent with the results above, we found that the sensitivity rates obtained with these models all showed a very poor discrimination for the majority of emotions, except for calm which was more successfully discriminated in comparison to predictions based on other components. Interestingly, the subjective body-related items from CoreGRID tended to surpass the objective physiology data [t(19) = 3.448, p = 0.002, Cohen's d = 0.88, 95% CI (0.27 1.49)].
The CPM defines emotions by assuming that they are multicomponent phenomena, comprising changes in appraisal, motivation, expression, physiology, and feeling. A considerable advantage of this theory is that it offers the possibility of computational modeling based on a specific parameter space, in order to account for behavioral (Wehrle and Scherer, 2001; Meuleman et al., 2019) and neural (Leitão et al., 2020; Mohammadi et al., 2020) aspects of emotion in terms of dynamic and interactive responses among components. The current research applied multivariate pattern classification analyses for assessing the CPM framework with a range of emotions experienced during movie watching. Through this computational approach, we first investigated the links between physiology manifestations and the five emotion components proposed by the CPM to determine predictive relationships between them. Second, we investigated whether discrete emotion types can be discriminated from the multicomponent pattern of responses and assessed the importance of each component.
Assuming that physiological responses are intertwined with all components of emotion, we expected that ratings on the 32 CoreGRID features would carry information sufficient to predict corresponding physiological changes. Effectively, SVM classifications provided prediction accuracies significantly better than the chance level. However, information from the CoreGRID items did not allow a high accuracy, even though prediction was simplified by being restricted to a binary distribution. This modest accuracy may be explained by a great variability across participants, which could reduce the generalizability of classifiers when they were applied to all individuals rather than within subject. It might also reflect heterogeneity in intra-individual physiological responses among emotions with similar componential patterns. In parallel, the opposite approach to predict ratings of CoreGRID features based on the means and variances of physiological responses also yielded a performance significantly higher than chance level but still relatively low. Because each CoreGRID item focuses on quite specific behavioral features, it is however not surprising that the sole use of physiology would be insufficient to precisely determine the ratings.
More importantly, if experiencing an emotion affects simultaneously more than one major component of emotion, one would expect that componential responses are clustered into qualitatively differentiated patterns (Scherer, 2005a; Fontaine et al., 2013). In the CPM view, an emotion arises when components are coherently organized and transiently synchronized (Scherer, 2005b). Accordingly, subjective emotion awareness might emerge as the conscious product of the feeling component generated by such synchronization (Grandjean et al., 2008). However, verbal accounts of conscious feelings may restrict the richness of emotional experience when using only declarative reports. Therefore, we anticipated that integrating the five components together into multivariate pattern analyses would provide higher accuracy rates in emotion prediction. This hypothesis was effectively confirmed, as the best prediction performances were obtained from non-linear multiclass SVM when the 32 CoreGRID items and the physiological measures were used all together in the model. Nevertheless, it is important to note that through our one-component-out model comparisons, we found that the body and physiology component was negligible in the overall discrimination of discrete emotion labels. Indeed, prediction performances of a model without body and physiology features were not significantly different from those of the complete model, demonstrating that information derived from these data may have already been encoded in other components. However, further analyses could help to better confirm this observation.
Critically, the CPM assumes a strong causal link between appraisal and other components of emotion, since appraisal processes are the primary trigger of emotion and should account for a major part of qualitative differences in feelings (Moors and Scherer, 2013). For example, a cross-cultural study demonstrated that an appraisal questionnaire alone (31 appraisal features) could discriminate between 24 emotion terms with an accuracy of 70% (Scherer and Fontaine, 2013). In our study, we found all components provided relevant information. Moreover, although being the best predictor, the appraisal component did not provide significantly more information compared to the other components, except in comparison to the model using only body and physiology features. Overall, prediction from components did not significantly differ across emotions. At least three components were always predicting one emotion category within the same range of accuracy. These results are consistent with the assumption of a synchronized and combined engagement of these components during emotion elicitation. It is possible, however, that some results were affected by the uneven distribution of events across classes (Supplementary Figure S1), such as for calm (high representation) which stood out as the most recognizable state regardless of the component used, or for fear, love, and satisfaction (low representation) where all classifiers were poorly sensitive.
In line with our data indicating that physiological measures did not reliably discriminate among emotion categories, the relationship between physiological responses and emotions has long fueled conflicting views. Some authors claimed that there is no invariant and unique autonomic signature linked to each category of emotion (Barrett, 2006), or that physiological response patterns may only distinguish dimensional states (Mauss and Robinson, 2009). In contrast, because emotions imply adaptive and goal-directed reactions, they might trigger differentiated autonomic states to modulate behavior (Stemmler, 2004; Kragel and LaBar, 2013). In this vein, Kreibig (2010) reviewed the most typical ANS responses induced across various emotions and pointed to fairly consistent and stable characteristics for particular affective experiences, but without explicitly confirming a strict emotion specificity since no unique physiological pattern could be highlighted as directly diagnostic of a single emotion. It has also been shown that a single or small number of physiological indices are not able to differentiate emotions (Harrison et al., 2013). Our findings support this view by suggesting that a broader set of measures should be recorded to increase discriminative power, including physiology as well as other components.
Our study is not without limitations. First, statistical machine learning methods may be considered as uninterpretable black boxes. Indeed, SVM analysis gives no explicit clue on functional dimensions underlying classification performances. Second, these data-driven methods often need large amounts of data. We acquired data over a large number of videos and events covering a range of different emotions, but discrimination of specific patterns among the different emotion components was relatively limited with our sample of 20 participants. Third, although participants were asked to report their initial feelings during the first viewing, changes in emotional experience due to repetition or potential recall biases may not be completely excluded since each movie segment was played again before rating CoreGRID items. Fourth, we used a restricted number of CoreGRID items due to time and experimental constraints. It would certainly be beneficial to measure each component in more detailed ways by taking more features into account. For instance, motor behaviors (e.g., facial expressions) could be evaluated with direct measures such as EMG rather than self-report items. This could help to provide more objective and perhaps more discriminant measures, particularly concerning variations of pleasantness (Larsen et al., 2003). As another example, given that the appraisal component is crucial for emotion elicitation, a wider range of appraisal dimensions might allow a more precise discrimination of discrete emotions and physiological patterns. In the same way, it is also possible that the set of items selected from the original CoreGRID instrument may account for suboptimal discrimination performances (i.e., improving or degrading the classification of certain categories of emotion). Lastly, even though using film excerpts has many advantages (e.g., naturalistic and spontaneous emotion elicitation, control over stimuli and timing, standardized validation, and concomitant measurement of physiological responses), an ideal experimental paradigm should evoke first-person emotions in the participants to fully test the assumptions of the CPM framework. In other words, the only way to faithfully elicit a genuine emotion is to get participants to experience an event as pertinent for their own concerns, in order to activate the four most important appraisal features (relevance, implication, coping, normative significance) that are thought to be crucial to trigger an emotion episode (Sander et al., 2005). Viewing film excerpts is an efficient (Philippot, 1993) but passive induction technique and, therefore, the meaning of some appraisal components might be ambiguous or difficult to rate. As a result, subjective reports of behaviors and action tendencies were most likely different compared to what they would be for the same event in real life. We also cannot rule out that the correspondence found between CoreGRID items and discrete emotion labels could partially be affected by the order of the measures collected. For example, providing component-related ratings first may have activated knowledge of the emotion construct that was then used to select a label. Future research should develop more ecological scenarios that can be experienced by participants according to their self-relevance and followed by true choices of possible actions. For example, a sophisticated and ecological method was recently developed by connecting a wearable physiological sensor to a smartphone (Larradet et al., 2019). Upon detection of relevant physiological activity, the participant received a notification on her smartphone requesting to report her current emotional state. Alternatively, a study used virtual reality games to assess the CPM across various emotions (Meuleman and Rudrauf, 2018) and found that fear and joy were predicted by appraisal variables better than by other components, whereas these two emotions were generally poorly classified in our study. Other recent studies have also made use of (virtual reality) video games to assess appraisal and other emotion components during brain imaging (Leitão et al., 2020) or physiological (Bassano et al., 2019) measurements.
Taken together, our results support the reliability and the interindividual consistency of CPM in the study of emotion. Multivariate pattern classification analyses generated results better than chance level (with statistical significance) to predict (1) changes in physiological measures from the 32 CoreGRID items, (2) ratings of the majority of CoreGRID items from physiological measures, and (3) discrete emotion labels that refer to conscious feelings experienced by the participants and presumably emerge from a combination of physiological and behavioral parameters. Overall, we observed, however, that physiological features were the least significant predictor for emotion classification. Yet, since our results also suggest that physiology was encoded within each of the other major components of emotion, they support the hypothesis of synchronized recruitment of all components during an emotion episode.
Further work is now required to determine why certain patterns of behavioral and physiological responses were misclassified into incorrect emotion categories and to study more deeply the links between different emotions. Similarly, it is also needed to explain the importance of various components in the recognition of different emotion categories. For instance, it would be valuable to determine whether poor discrimination stems from a too low sensitivity of the CoreGRID items and physiological measures or whether some categories of emotions simply cannot be differentiated into distinct entities with such methods, perhaps due to a high degree of overlap within the different components of emotion. Future developments allowing objective measures for each component during first-person elicitation paradigms are required to limit as much as possible the use of self-assessment questionnaires and ensure ecological validity. Overall, the current study opens a new paradigm to explore the depth of processes involved in emotion formation as well as a means of unfolding the necessary processes to be considered in developing a reliable emotion recognition system.
The raw data supporting the conclusions of this article will be made available by the authors, without undue reservation.
The studies involving human participants were reviewed and approved by Geneva Cantonal Research Committee. The participants provided their written informed consent to participate in this study.
MQM, GM, and PV contributed to the study conception and study design. MQM and GM contributed to the data collection, data analysis and statistical analysis. JL also contributed to the data analysis. MQM, GM, JL, and PV contributed to the data interpretation, manuscript drafting and revision. All authors listed have made a substantial, direct, and intellectual contribution to the work and approved it for publication.
This research was supported by a grant from the Swiss National Science Foundation (SNF Sinergia No. 180319) and the National Centre of Competence in Research (NCCR) Affective Sciences (under grant No. 51NF40- 104897). It was conducted on the imaging platform at the Brain and Behavior Lab (BBL) and benefited from the support of the BBL technical staff.
The authors declare that the research was conducted in the absence of any commercial or financial relationships that could be construed as a potential conflict of interest.
All claims expressed in this article are solely those of the authors and do not necessarily represent those of their affiliated organizations, or those of the publisher, the editors and the reviewers. Any product that may be evaluated in this article, or claim that may be made by its manufacturer, is not guaranteed or endorsed by the publisher.
The Supplementary Material for this article can be found online at: https://www.frontiersin.org/articles/10.3389/fcomp.2022.773256/full#supplementary-material
Barrett, L. F. (2006). Are emotions natural kinds? Perspect. Psychol. Sci. 1, 28–58. doi: 10.1111/j.1745-6916.2006.00003.x
Barrett, L. F., Mesquita, B., Ochsner, K. N., and Gross, J. J. (2007). The experience of emotion. Annu. Rev. Psychol. 58, 373–403. doi: 10.1146/annurev.psych.58.110405.085709
Bassano, C., Ballestin, G., Ceccaldi, E., Larradet, F., Mancini, M., Volta, E., et al. (2019). “A VR Game-based system for multimodal emotion data collection,” in 12th Annual ACM SIGGRAPH Conference on Motion, Interaction and Games 2019 38, 1–3. doi: 10.1145/3359566.3364695
Christie, I. C., and Friedman, B. H. (2004). Autonomic specificity of discrete emotion and dimensions of affective space: a multivariate approach. Int. J. Psychophysiol. 51, 143–153. doi: 10.1016/j.ijpsycho.2003.08.002
Ekman, P. (1992). An argument for basic emotions. Cogn. Emot. 6, 169–200. doi: 10.1080/02699939208411068
Fontaine, J. R. J., Scherer, K. R., and Soriano, C. (2013). “The why, the what, and the how of the GRID instrument,” in Components of emotional meaning: a sourcebook, eds J. R. J. Fontaine, K. R. Scherer, and C. Soriano (Oxford: Oxford University Press), 83–97. doi: 10.1093/acprof:oso/9780199592746.003.0006
Frijda, N. H., Kuipers, P., and Ter Schure, E. (1989). Relations among emotion, appraisal, and emotional action readiness. J. Pers. Soc. Psychol. 57, 212. doi: 10.1037/0022-3514.57.2.212
Gabert-Quillen, C. A., Bartolini, E. E., Abravanel, B. T., and Sanislow, C. A. (2015). Ratings for emotion film clips. Behav. Res. Methods 47, 773–787. doi: 10.3758/s13428-014-0500-0
Girard, J. (2014). CARMA: software for continuous affect rating and media annotation. J. Open Res. Softw. 2, e5. doi: 10.5334/jors.ar
Grandjean, D., Sander, D., and Scherer, K. R. (2008). Conscious emotional experience emerges as a function of multilevel, appraisal-driven response synchronization. Consc. Cogn. 17, 484–495. doi: 10.1016/j.concog.2008.03.019
Gross, J. J., and Levenson, R. W. (1995). Emotion elicitation using films. Cogn. Emot. 9, 87–108. doi: 10.1080/02699939508408966
Gunes, H., and Pantic, M. (2010). Automatic, dimensional and continuous emotion recognition. Int. J. Synth. Emot. 1, 68–99. doi: 10.4018/jse.2010101605
Harrison, N. A., Kreibig, S. D., and Critchley, H. D. (2013). “A two-way road,” in The Cambridge Handbook of Human Affective Neuroscience Efferent and Afferent Pathways of Autonomic Activity in Emotion, eds J. Armony, and P. Vuilleumier (Cambridge: Cambridge University Press), 82–106. doi: 10.1017/CBO9780511843716.006
Izard, C. E., Libero, D. Z., Putnam, P., and Haynes, O. M. (1993). Stability of emotion experiences and their relations to traits of personality. J. Pers. Soc. Psychol. 64, 847–860. doi: 10.1037/0022-3514.64.5.847
Kragel, P. A., and LaBar, K. S. (2013). Multivariate pattern classification reveals autonomic and experiential representations of discrete emotions. Emotion 13, 681–690. doi: 10.1037/a0031820
Kreibig, S. D. (2010). Autonomic nervous system activity in emotion: a review. Biol. Psychol. 84, 394–421. doi: 10.1016/j.biopsycho.2010.03.010
Kreibig, S. D., Wilhelm, F. H., Roth, W. T., and Gross, J. J. (2007). Cardiovascular, electrodermal, and respiratory response patterns to fear- and sadness-inducing films. Psychophysiology 44, 787–806. doi: 10.1111/j.1469-8986.2007.00550.x
Larradet, F., Niewiadomski, R., Barresi, G., and Mattos, L. S. (2019). “Appraisal theory-based mobile app for physiological data collection and labelling in the wild,” in Proceedings of the 2019 ACM International Joint Conference on Pervasive and Ubiquitous Computing and Proceedings of the 2019 ACM International Symposium on Wearable Computers (London: ACM), 752–756. doi: 10.1145/3341162.3345595
Larsen, J. T., Norris, C. J., and Cacioppo, J. T. (2003). Effects of positive and negative affect on electromyographic activity over zygomaticus major and corrugator supercilii. Psychophysiology 40, 776–785. doi: 10.1111/1469-8986.00078
Leitão, J., Meuleman, B., Van De Ville, D., and Vuilleumier, P. (2020). Computational imaging during video game playing shows dynamic synchronization of cortical and subcortical networks of emotions. PLoS Biol. 18, e3000900. doi: 10.1371/journal.pbio.3000900
Lindquist, K. A., Siegel, E. H., Quigley, K. S., and Barrett, L. F. (2013). The hundred-year emotion war: are emotions natural kinds or psychological constructions? Comment on Lench, Flores, and Bench (2011). Psychol. Bull. 139, 255–263. doi: 10.1037/a0029038
Matsumoto, D., and Ekman, P. (2009). “Basic emotions,” in The Oxford Companion to Emotion and the Affective Sciences, eds D. Sander and K. R. Scherer (New York, NY: Oxford University Press), 69–72.
Mauss, I. B., and Robinson, M. D. (2009). Measures of emotion: a review. Cogn. Emot. 23, 209–237. doi: 10.1080/02699930802204677
McHugo, G. J., Smith, C. A., and Lanzetta, J. T. (1982). The structure of self-reports of emotional responses to film segments. Motivat. Emot. 6, 365–385. doi: 10.1007/BF00998191
Meuleman, B., Moors, A., Fontaine, J., Renaud, O., and Scherer, K. (2019). Interaction and threshold effects of appraisal on componential patterns of emotion: a study using cross- cultural semantic data. Emotion 19, 425–442. doi: 10.1037/emo0000449
Meuleman, B., and Rudrauf, D. (2018). Induction and profiling of strong multi-componential emotions in virtual reality. IEEE Trans. Affect. Comput. 12, 189–202. doi: 10.1109/TAFFC.2018.2864730
Meuleman, B., and Scherer, K. R. (2013). Nonlinear appraisal modeling: an application of machine learning to the study of emotion production. IEEE Trans. Affect. Comput. 4, 398–411. doi: 10.1109/T-AFFC.2013.25
Mohammadi, G., Van De Ville, D., and Vuilleumier, P. (2020). Brain networks subserving functional core processes of emotions identified with componential modelling. bioRxiv [Preprint]. doi: 10.1101/2020.06.10.145201
Mohammadi, G., and Vuilleumier, P. (2020). “A multi-componential approach to emotion recognition and the effect of personality,” in IEEE Transactions on Affective Computing. doi: 10.1109/TAFFC.2020.3028109
Moors, A. (2009). Theories of emotion causation: a review. Cogn. Emot. 23, 625–662. doi: 10.1080/02699930802645739
Moors, A., and Scherer, K. R. (2013). “The role of appraisal in emotion,” in Handbook of Cognition and Emotion, eds M. Robinson, E. Watkins, and E. Harmon-Jones (New York, NY: Guilford Press), 135–155.
Philippot, P. (1993). Inducing and assessing differentiated emotion-feeling states in the laboratory. Cogn. Emot. 7, 171–193. doi: 10.1080/02699939308409183
Quigley, K. S., and Barrett, L. F. (2014). Is there consistency and specificity of autonomic changes during emotional episodes? Guidance from the Conceptual Act Theory and psychophysiology. Biol. Psychol. 98, 82–94. doi: 10.1016/j.biopsycho.2013.12.013
Russell, J. A. (2009). Emotion, core affect, and psychological construction. Cogn. Emot. 23, 1259–1283. doi: 10.1080/02699930902809375
Sander, D., Grandjean, D., and Scherer, K. R. (2005). A system approach to appraisal mechanisms in emotion. Neural Netw. 18, 317–352. doi: 10.1016/j.neunet.2005.03.001
Schaefer, A., Nils, F., Sanchez, X., and Philippot, P. (2010). Assessing the effectiveness of a large database of emotion-eliciting films: a new tool for emotion researchers. Cogn. Emot. 24, 1153–1172. doi: 10.1080/02699930903274322
Scherer, K. R. (1984). “On the nature and function of emotion: a component process approach,” in Approaches to Emotion, eds K. R. Scherer and P. Ekman (Hillsdale, NJ: Erlbaum), 293–317.
Scherer, K. R. (2005a). What are emotions? And how can they be measured? Soc. Sci. Inform. 44, 695–729. doi: 10.1177/0539018405058216
Scherer, K. R. (2005b). “Unconscious processes in emotion: the bulk of the iceberg,” in Emotion and Consciousness, eds L. F. Barrett, P. M. Niedenthal, and P. Winkielman (New York, NY: Guilford Press), 312–334.
Scherer, K. R. (2009). The dynamic architecture of emotion: evidence for the component process model. Cogn. Emot. 23, 1307–1351. doi: 10.1080/02699930902928969
Scherer, K. R., and Fontaine, J. R. J. (2013). “Driving the emotion process: the appraisal component, in Components of emotional meaning: a sourcebook,” in Components of Emotional Meaning, eds J. R. J. Fontaine, K. R. Scherer, and C. Soriano (Oxford: Oxford University Press), 186–209. doi: 10.1093/acprof:oso/9780199592746.003.0013
Siegel, E. H., Sands, M. K., Van den Noortgate, W., Condon, P., Chang, Y., Dy, J., et al. (2018). Emotion fingerprints or emotion populations? A meta-analytic investigation of autonomic features of emotion categories. Psychol. Bull. 144, 343–393. doi: 10.1037/bul0000128
Smith, C. A., and Ellsworth, P. C. (1985). Patterns of cognitive appraisal in emotion. J. Pers. Soc. Psychol. 48, 813. doi: 10.1037/0022-3514.48.4.813
Soleymani, M., Chanel, G., Kierkels, J. J., and Pun, T. (2009). Affective characterization of movie scenes based on content analysis and physiological changes. Int. J. Semant. Comput. 3, 235–254. doi: 10.1142/S1793351X09000744
Stemmler, G. (2004). “Physiological processes during emotion,” in The Regulation of Emotion, eds P. Philippot and R. S. Feldman (Mahwah, NJ: Erlbaum).
Stephens, C. L., Christie, I. C., and Friedman, B. H. (2010). Autonomic specificity of basic emotions: evidence from pattern classification and cluster analysis. Biol. Psychol. 84, 463–473. doi: 10.1016/j.biopsycho.2010.03.014
Wager, T. D., Kang, J., Johnson, T. D., Nichols, T. E., Satpute, A. B., and Barrett, L. F. (2015). A bayesian model of category-specific emotional brain responses. PLoS Comput. Biol. 11, e1004066. doi: 10.1371/journal.pcbi.1004066
Keywords: emotion, component model, autonomic nervous system, physiological responses, computational modeling
Citation: Menétrey MQ, Mohammadi G, Leitão J and Vuilleumier P (2022) Emotion Recognition in a Multi-Componential Framework: The Role of Physiology. Front. Comput. Sci. 4:773256. doi: 10.3389/fcomp.2022.773256
Received: 09 September 2021; Accepted: 06 January 2022;
Published: 28 January 2022.
Edited by:
Kun Yu, University of Technology Sydney, AustraliaReviewed by:
Jason Bernard, McMaster University, CanadaCopyright © 2022 Menétrey, Mohammadi, Leitão and Vuilleumier. This is an open-access article distributed under the terms of the Creative Commons Attribution License (CC BY). The use, distribution or reproduction in other forums is permitted, provided the original author(s) and the copyright owner(s) are credited and that the original publication in this journal is cited, in accordance with accepted academic practice. No use, distribution or reproduction is permitted which does not comply with these terms.
*Correspondence: Maëlan Q. Menétrey, bWFlbGFuLm1lbmV0cmV5QGVwZmwuY2g=
†Present address: Maëlan Q. Menétrey, Laboratory of Psychophysics, Brain Mind Institute, École Polytechnique Fédérale de Lausanne (EPFL), Lausanne, Switzerland
Disclaimer: All claims expressed in this article are solely those of the authors and do not necessarily represent those of their affiliated organizations, or those of the publisher, the editors and the reviewers. Any product that may be evaluated in this article or claim that may be made by its manufacturer is not guaranteed or endorsed by the publisher.
Research integrity at Frontiers
Learn more about the work of our research integrity team to safeguard the quality of each article we publish.