- AI R and I Division, DENSO CORPORATION, Kariya, Japan
One solution supporting a healthy mental state for humans is controlling the environment with ambient intelligence technology. We are developing a mental support system for healthy people that automatically changes environmental conditions, such as sound volume and light color, depending on the user’s mental state, which is monitored according to physiological signals such as sympathetic nerve activity. In our previous basic study under laboratory-controlled conditions, the system was applied to improve the user’s concentration level as they performed calculation tasks. Results indicated that the system improved the task performance, but individual variations existed, with some users improving greatly but others much less. For the future practical application of the system, determining the causes of the variation in efficacy is important. Considering that the brain structure and activity differ according to an individual’s personality, we investigated the relationship between the user’s personality and task performance with our system’s support. The results showed a clear correlation between the extraversion score and task performance. Our study presents an example where the system’s efficacy is sensitive to the user’s personality and indicates the importance of considering the user’s personality when designing a mental support system based on ambient intelligence.
Introduction
Maintaining mental well-being is important, especially in light of the COVID-19 pandemic that has forced urgent changes in our life and work styles. WHO (World Health Organization, 2020) has raised concerns over worsening of mental health because of the stress or fear caused by the pandemic. Studies have shown that stressors associated with telework can reduce well-being and eventually performance (Molino et al., 2020; Tarafdar et al., 2007). Thus, an urgent demand for developing a mental support system exists.
Over several decades, different methods and technologies have been studied to improve the mental state. However, these are not always universally effective and have different efficacies depending on the individual. A primary factor for this is the users’ personalities. For instance, the user’s personality plays an important role in accepting socially assistive robotics (SAR) for mental therapy and therapeutic performance (Tapus and Mataric, 2008). SAR affects the user’s mental state through multimodal cues to express empathy, including verbal and nonverbal (body movement) interactions as well as social distance. A social relationship between the robot and user can determine the success of SAR; therefore, user’s and robot’s personalities are considered important for further developing effective SAR (Esterwood and Robert, 2020).
A common method to improve the mental state is to use biofeedback. Many biofeedback schemes were developed for informing users of their autonomic nervous system activity based on their current heart rate and blood pressure (Purwandini Sutarto et al., 2012; Frank et al., 2010). This technology requires users to learn to control their involuntary physiological responses to enhance their mental state. Although compared to SAR, biofeedback involves a different kind of human–machine interaction, and its effectiveness is also related to personal traits (Jafarova et al., 2020). Similar to biofeedback, meditation/mindfulness is a useful self-regulatory approach for mental management. Takahashi et al. (2005) investigated the effects of personality on brain activity and found that changes in brain activity as measured by an electroencephalogram reflecting enhanced mindfulness were associated with personal traits. The mental state results from brain activity, and the abovementioned methods and technologies to improve the mental state ultimately try to affect or stimulate brain activity. Therefore, it is foreseeable that their effectiveness varies among individuals if the basic properties of the brain activity and structure differ with personalities.
Eysenck (1967) suggested a relationship between brain activity and personality by postulating that extraversion is related to cortical arousal. As alpha brain waves are correlated with a wakeful state (DeYoung et al., 2010), several studies investigated the relationship between brain waves and personalities, and their results have supported Eysenck’s claim (Tran et al., 2001; Gale et al., 2001). Research on the relationship between brain activity and personality has been expanded to include the brain structure and brain network activity, and the results revealed that the brain structure and dynamics of the default mode network are associated with personality (DeYoung et al., 2010; Tran et al., 2006). Overall, these studies found that brain activity is fundamentally different among individuals with different personalities. Accordingly, individual differences in brain responses to stimuli have been attributed to differences in personalities (Stenberg, 1994). Thus, the user’s personality is an important consideration when developing a mental support system.
We have been developing a mental support system for healthy people based on ambient intelligence (AmI). AmI is broadly defined as an information technology focused on supporting people using a digital environment that is sensitive, adaptive, and responsive to human needs and emotions (Friedewald et al., 2005). This technology is expected to provide solutions in the healthcare domain to both healthy and/or unhealthy people such as patients suffering from the Alzheimer’s disease (Acampora et al., 2013; Cavallo et al., 2015; Machado et al., 2021). In our previous basic study conducted under laboratory-controlled conditions (Iwashita and Ishikawa, 2021), we validated our system experimentally using the system to improve the user’s concentration (mental state), while measuring their current mental state; a sound speaker, an aroma diffuser, and colored lights were used to control the environment, while users conducted a calculation task for 45 min (Iwashita and Ishikawa, 2021). Our system helped many users improve their calculation scores, but the improvement varied significantly among individuals. For the future practical application of the current basic system, determining the causes of the variation in efficacy is important. In our system, the environmental changes are likely to stimulate the brain. Neuroscience research has revealed the relationship between neural response to stimuli and personality (Gurrera et al., 2001). Therefore, personality may alter the effectiveness of our system. So far, little research has been conducted on quantifying the effect of personality on the efficacy of AmI-based systems. As a case study, we investigated the relationship between the effectiveness of our system and user’s personality by analyzing the data from our previous study (Iwashita and Ishikawa, 2021).
Methods
Here, we briefly describe the methodology of our previous study where the experimental data were obtained (Iwashita and Ishikawa, 2021) and the new statistical analysis performed in this study.
Experimental Design
Our AmI-based system was applied to improving the concentration of participants. Participants played the countdown 25 square (C25S) calculation game (see below) for 45 min under different conditions with and without the aid of the AmI-based system while their sympathetic nerve activity was monitored according to the nasal skin temperature. During the first 8 min, the participants played the game with the three environmental devices (sound speaker, aroma diffuser, and lights) at their initial settings, which were set by the participants according to their preferences prior to the game. For the remaining time, participants played the game under one of three conditions: (1) user-only control (User), (2) random control (With Random), and (3) with the AmI-based system (With AmI). Under the User condition, environmental changes were controlled by participants without the aid of any system. Under the With Random condition, the system’s actions were determined randomly. The participants partook in three experiments under three different conditions at three different morning hours. Every experiment was executed at least 1 day apart in randomized order. After all the experiments took place, participants were asked to take the Maudsley personality inventory (MPI) test, which is a questionnaire designed to measure the two major dimensions of personality: extraversion and neuroticism (Eysenck, 1947).
Participants
Eleven healthy males aged 18–42 years, employees of DENSO CORPORATION, participated in the experiment. The experiment was approved by the local ethics committee at the DENSO CORPORATION. After receiving detailed written and oral instructions, participants provided their informed written consent to participate in the study. The experimental results of 9 of the 11 participants were described previously (Iwashita and Ishikawa, 2021). For the remaining two participants, one of the results from three experiments was excluded because they did not meet our criteria (i.e., they had enough sleep and no significant personal issue is ongoing). In the present study, we utilized the results of all 11 participants, except in two experiments because of the rationale described above. Accordingly, we had 10 participants’ data for the User condition, 11 participants’ data for the With Random condition, and 10 participants’ data for the With AmI condition.
AmI-Based System
The AmI-based system developed by us in a previous study works in an interactive way between the user and system (Iwashita and Ishikawa, 2021). Because the mental state is stochastic in nature, we employed an interactive method for realizing effective environmental control. This method monitors real-time biological signals (sympathetic nerve activity) to infer and improve the user’s current mental state and adapt the online environmental controls to the user’s preferences, which usually vary with the situation (e.g., work or leisure).
The AmI-based system estimates the user’s concentration level by monitoring the nasal skin temperature, which is known to increase as sympathetic nerve activity decreases (Wallin, 1981; Mizuno and Kume, 2015; Nozawa and Tacano, 2009). The estimated concentration level is then used to control environmental devices to enhance the concentration level as desired. Figure 1 shows the structure of the AmI-based system. The nasal skin temperature is normalized by subtracting the forehead skin temperature. The average nasal skin temperature for 2 min is used to determine control commands every 2 min, which was assumed as sufficient for the temperature to reflect sympathetic nerve activity. Only when the averaged nasal skin temperature increases, the system executes control commands to increase sympathetic nerve activity. The system is designed to adapt to a user’s environmental preferences based on their control commands. If the user changes the sound volume often, the system tends to change the sound volume rather than the aroma intensity and light color. Furthermore, the system evaluates the effects of control commands on the nasal skin temperature in real time, which it uses to update the function to generate effective control commands. If increasing the sound volume is not as effective as reducing the nasal skin temperature, the next command will decrease the sound volume. The detailed algorithm of each module is described in our previous paper (Iwashita and Ishikawa, 2021).
Random System
The random system determines the control commands for environmental devices randomly, without monitoring the user’s current nasal skin temperature. In the experiments, the control commands were executed with a 50% chance for any control command every 2 min (same as with the AmI-based system), and the degree of change was selected randomly.
Experimental Setup
Figure 2A displays the experimental setup. The environmental devices were a natural sound speaker (i.e., a smartphone application), an aroma emitter (Aroma Join Corporation, Japan), and three smart lightbulbs (PHILIPS, the Netherlands). The participants selected two of five natural sounds (wave, rain, café, village, and magma) as their preferences beforehand. The sound volume, aroma intensity, and light color were controlled by the device controller application (Figure 2B). The sound volume was scaled to 0–100, which corresponded to ∼15–70 dB. The volumes of the two sounds could be specified separately. The aroma intensity was scaled 0–5, which corresponded to spray times of 0–5 s in increments of 1 s. The light color was scaled as 0–100, which ranged from red to purple. The system output was the sound volume for the louder of the two chosen sounds (the volume of the softer sound was determined by keeping the volume ratio of two ongoing sounds same), the aroma intensity, which was converted to a 0–100 scale from the 0–5 scale displayed on the controller, and the light color. Both the nasal and forehead skin temperatures were measured with Polymate I, which is a biological signal recorder (Miyuki Giken Corporation, Japan).
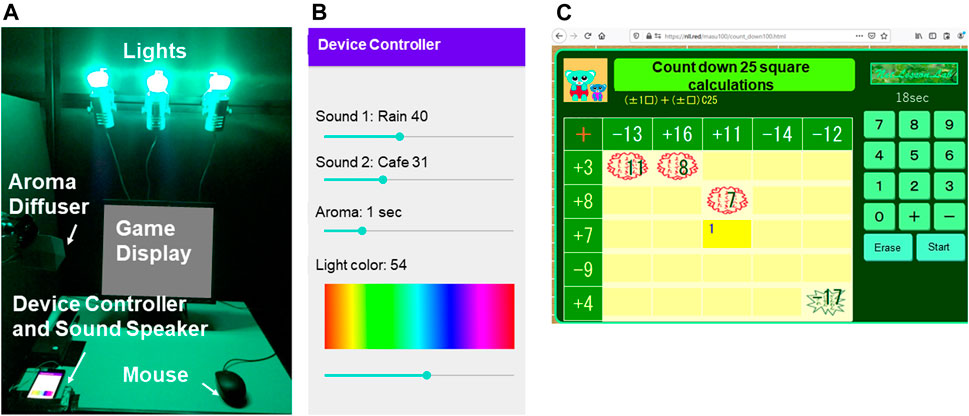
FIGURE 2. Experimental setup, device controller display, and Countdown Twenty-five Square (C25S) calculation game.(A) Experimental setup equipped with sound speakers, aroma diffuser, and three smart lights.(B) Device controller implemented on a smartphone.(C) C25S calculation game. When the 25 calculations of the game have been completed, the score, elapsed time, and total points are displayed (not shown in the figure).
Experimental Tasks
Figure 2C shows the C25S calculations. C25S is an educational PC game/free application comprising simple 25 summation or subtraction calculations and is available at https://nll.red/masu100/index_S.html. We obtained permission from the developer to use it. Once a participant clicked on the start button, they had to calculate an equation, which was selected randomly by the system from 25 possible choices. They had to input the answer by clicking a number displayed on the right side of the screen within 5 s. If the participant could not answer correctly within 5 s, it was counted as a “fail,” and the next equation was selected randomly. After all 25 equations were done (i.e., the game ended), participants repeated the game by clicking the start button again until a total time of 45 min had elapsed. The content of the game refreshed each time the start button was clicked. Participants could only manipulate the device controller before starting each game, and they were allowed to perform shoulder and arm stretches between games to reduce the fatigue caused by long-term mouse operation. The points scored in each game were calculated as follows:
where
Analysis
To perform a statistical analysis on the effect of personality on the system efficacy, we used a linear mixed-effect model, which can integrate continuous (personality scores) and categorical (condition—User, With Random, and With AmI) predictors under a repeated experimental design. The linear mixed-effect model was tested in R (version 4.0.2) with the lmer4, lmerTest, and car packages, with the alpha set to 0.05. The predictors were the conditions (User, With Random, and With AmI) and one of the MPI scores (extraversion or neuroticism), including the interaction between them. The task performance was the reference variable, and the participant was included as a random intercept. The task performance was defined as the trend line of the time series of the points. Post hoc analysis was adjusted with the Bonferroni method. The correlation coefficient (r) was calculated using Pearson’s correlation in R (“cor.test” function; version 4.0.2).
Results
As an example, Figures 3A–C show the nasal skin and environmental changes of a participant, and Figures 3D–F show the corresponding task performance. The task performance was evaluated according to the slope of the trend line of the time series of points (i.e., a of y = ax + b). The points tended to increase under the With AmI condition, but not under the other conditions. Our previous study showed that the task performance statistically improved under the With AmI condition (Iwashita and Ishikawa, 2021), but individual differences were observed in the level of improvement.
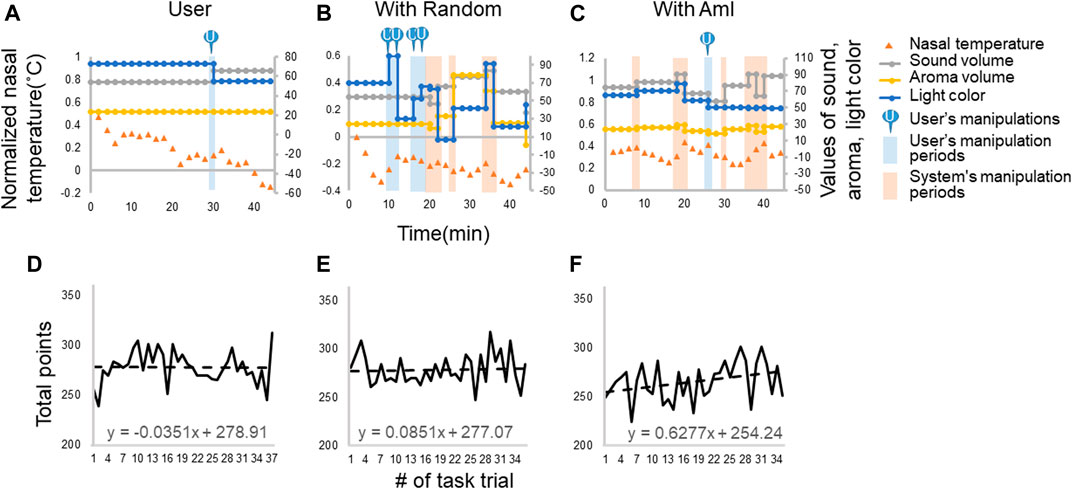
FIGURE 3. Example of a participant’s time courses for the nasal skin temperature, environmental manipulations, and task performance. (A–C) Time courses for the nasal skin temperature and environmental manipulations of the sound volume, aroma intensity, and light color under three conditions (User, With Random, and With AmI). (D–F) Time courses for the task performances according to the points scored in each C25S game. The trend lines (dotted line) and approximation formulas are also shown.
In this paper, a statistical analysis was performed to evaluate the effectiveness of the system with regard to personality. The linear mixed-effects model indicated that neuroticism had insignificant statistical effect on task performance [condition: degree of freedom (df) = 2, chi-square = 0.9, p-value = 0.89930; neuroticism-score: df = 1, chi-square = 0.1, p-value = 0.69860; condition*neuroticism-score: df = 2, chi-square = 1.1, p-value = 0.58530]. In contrast, the model indicated that the conditions and interaction between conditions and extraversion score had a significant effect on the task performance (condition: df = 2, chi-square = 9.1, p-value = 0.01067; extraversion score: df = 1, chi-square = 2.0, p-value = 0.15231; condition* extraversion score: df = 2, chi-square = 18.2, p-value = 0.00011). First, we compared task performance under various conditions, as in our previous study (Iwashita and Ishikawa, 2021). Figure 4A shows the averaged slope of the trend line of the points for all participants under the three conditions. The slope value was significantly higher under the With AmI condition than under the User condition (Wilcoxon test, Z-score = 2.3, p-value = 0.0391, adjusted by the Bonferroni correction, effect size r = 0.77). The slope value did not differ significantly between the With Random and User conditions (Wilcoxon test, Z-score = 0.7, p-value = 0.5703, adjusted by Bonferroni correction). These results were consistent with those of our previous study (Iwashita and Ishikawa, 2021). Next, we investigated the relationship between task performance and extraversion. Figures 4B–D show the correlation between the performance and extraversion score under each experimental condition. The relationship between extraversion and task performance differed depending on the condition. No correlation was observed under the User condition (r = 0.031), a negative correlation was observed under the With Random condition (r = −0.574), and a positive correlation was observed under the With AmI condition (r = 0.672). These results suggest that, when the environmental control was automated, an association existed between extraversion and task performance, whether positive or negative.
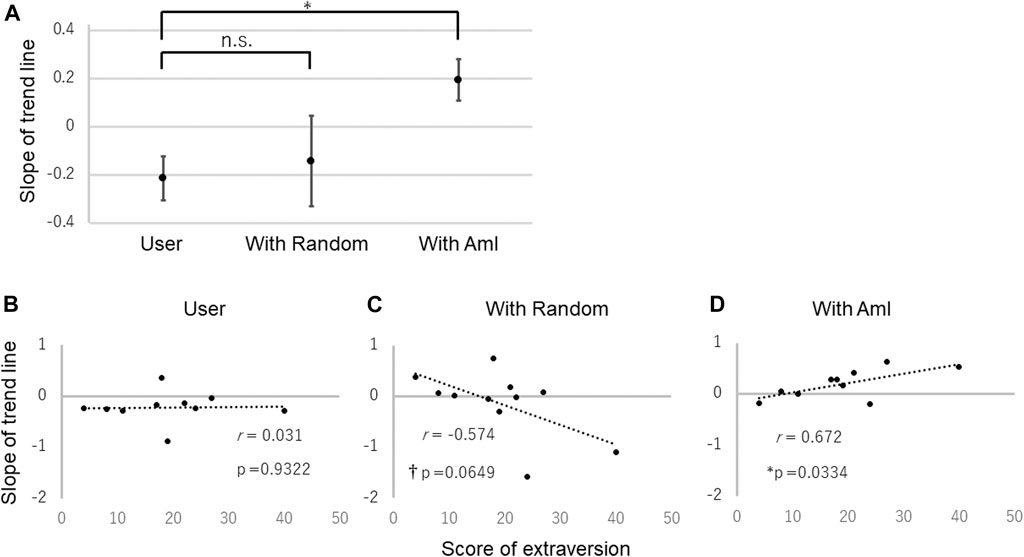
FIGURE 4. Effect of the system on the task performance and relationship between the task performance and personality. (A) Effects of the three conditions on task performance as the slope of the trend line; the error bars represent the SEM of the slope of the trend line. Relationship between the extraversion score and task performance as the slope of the trend line: (B) User, (C) With Random, and (D) With AmI.
Discussion
The present research showed that our AmI-based system had a positive correlation with the extraversion score, whereas the random system had a negative correlation. Our results can be interpreted by considering the work of (Matthews and Amelang, 1993), who investigated the relationship between task performance and brain waves. They found that extraverts performed better when their alpha waves were low in power, whereas introverts performed better when their alpha waves were high in power. Generally, alpha wave activity is low when cortical arousal is high and vice versa. Moreover, sympathetic nerve activity increases with high cortical arousal and vice versa (Lim et al., 1996). Therefore, extraverts may perform well when sympathetic nerve activity is high (i.e., high arousal state), whereas introverts perform well when sympathetic nerve activity is low (i.e., low arousal state). Our system is designed to manipulate environmental devices to increase the sympathetic nerve activity while the activity was decreasing. Thus, our AmI-based system may be more effective for extraverts than introverts. For introverts, a system controlling environmental devices to reduce sympathetic nerve activity may help improve task performance.
The random system showed a negative correlation between the task performance and extraversion score. This may be because the randomly timed environmental control did not effectively increase sympathetic nerve activity, which seems to be helpful for extraverts. The random system seemed to be slightly more effective for introverts than our AmI-based system, which indicates that the environmental control was sometimes effective. Thus, some of the random controls had a positive effect on the task performance of introverts.
When the environment was controlled by the user without any support from a system, there was no correlation between the task performance and personality. These results highlight that task performance is sensitive to personality when the environment control is supported by a system. Therefore, to develop an effective AmI-based mental support system fitting each user, personality is an important consideration. Especially, utilizing the known differences in brain activity for each personality will be helpful for system design. For example, we can design a system that reduces sympathetic nerve activity for introverts. Alternatively, moderate changes to the sound volume may help prevent stimulating excessive brain activity because introverts tend to demonstrate higher tonic cortical arousal than extraverts (Kumari et al., 2004).
To apply the current study in practical situations, our system must be validated under not only laboratory conditions but also real-life conditions. Moreover, the system must employ more user-friendly sensors such as noninvasive sensors to monitor sympathetic nerve activity. In future work, we aim to extend this study to a larger and more diverse sample under diverse real-life situations/scenarios to further validate the system.
Here, we presented only a case study on the relationship between personality and the efficacy of a system trying to improve concentration. However, personality should also have an effect when systems are trying to improve other mental states, such as relaxation. Future studies on the correlation between personality and the efficacy of mental support systems can help lead to a better understanding of human–machine interactions and the development of better AmI for mental support.
Data Availability Statement
The raw data supporting the conclusions of this article will be made available by the authors, without undue reservation.
Ethics Statement
The studies involving human participants were reviewed and approved by DENSO CORPORATION. The patients/participants provided their written informed consent to participate in this study.
Author Contributions
All authors designed the research. MoI performed the research and wrote first draft of the manuscript. All authors contributed to manuscript revision.
Conflict of Interest
Authors MoI, KI, and MaI were employed by the company DENSO CORPORATION.
Publisher’s Note
All claims expressed in this article are solely those of the authors and do not necessarily represent those of their affiliated organizations, or those of the publisher, the editors and the reviewers. Any product that may be evaluated in this article, or claim that may be made by its manufacturer, is not guaranteed or endorsed by the publisher.
Acknowledgments
The authors would like to thank Enago (www.enago.jp) for the English language review.
References
Acampora, G., Cook, D. J., Rashidi, P., and Vasilakos, A. V. (2013). A Survey on Ambient Intelligence in Healthcare. Proc. IEEE 101 (12), 2470–2494. doi:10.1109/JPROC.2013.2262913.A
Cavallo, F., Aquilano, M., and Arvati, M. (2015). An Ambient Assisted Living Approach in Designing Domiciliary Services Combined with Innovative Technologies for Patients with Alzheimer's Disease. Am. J. Alzheimers. Dis. Other Demen. 30 (1), 69–77. doi:10.1177/1533317514539724
DeYoung, C. G., Hirsh, J. B., Shane, M. S., Papademetris, X., Rajeevan, N., and Gray, J. R. (2010). Testing Predictions from Personality Neuroscience. Psychol. Sci. 21 (6), 820–828. doi:10.1177/0956797610370159
Esterwood, C., and Robert, L. P. (2020). Personality in Healthcare Human Robot Interaction (H-HRI). Proc. 8th Int. Conf. Human-agent Interact., 87–95. doi:10.1145/3406499.3415075
Frank, D. L., Khorshid, L., Kiffer, J. F., Moravec, C. S., and McKee, M. G. (2010). Biofeedback in Medicine: Who, when, Why and How? Ment. Health Fam. Med. 7 (2), 85–91.
Friedewald, M., Costa, O. D., Punie, Y., Alahuhta, P., and Heinonen, S. (2005). Perspectives of Ambient Intelligence in the home Environment. Telematics Inform. 22 (3), 221–238. doi:10.1016/j.tele.2004.11.001
Gale, A., Edwards, J., Morris, P., Moore, R., and Forrester, D. (2001). Extraversion-introversion, Neuroticism-Stability, and EEG Indicators of Positive and Negative Empathic Mood. Personal. Individual Differences 30 (3), 449–461. doi:10.1016/s0191-8869(00)00036-2
Gurrera, R. J., O’Donnell, B. F., Nestor, P. G., Gainski, J., and McCarley, R. W. (2001). The P3 Auditory Event-Related Brain Potential Indexes Major Personality Traits. Biol. Psychiatry 49 (11), 922–929. doi:10.1016/S0006-3223(00)01067-2
Iwashita, M., and Ishikawa, M. (2021). Buddy System: An Adaptive Mental State Support System Based on Active Inference and Free Energy Principles. IEEE Transactions on Cognitive and Developmental Systems in press.
Jafarova, O., Mazhirina, K., Sokhadze, E., and Shtark, M. (2020). Self-regulation Strategies and Heart Rate Biofeedback Training,” Appl. Psychophysiol. Biofeedback, 45. 87–98. doi:10.1007/s10484-020-09460-5
Kumari, V., Ffytche, D. H., Williams, S. C. R., and Gray, J. A. (2004). Personality Predicts Brain Responses to Cognitive Demands. J. Neurosci. 24 (47), 10636–10641. doi:10.1523/JNEUROSCI.3206-04.2004
Lim, C. L., Barry, R. J., Gordon, E., Sawant, A., Rennie, C., and Yiannikas, C. (1996). The Relationship between Quantified EEG and Skin Conductance Level. Int. J. Psychophysiology 21 (2–3), 151–162. doi:10.1016/0167-8760(95)00049-6
Machado, S. D., Tavares, J. E. d. R., Martins, M. G., Barbosa, J. L. V., González, G. V., and Leithardt, V. R. Q. (2021). Ambient Intelligence Based on IoT for Assisting People with Alzheimer's Disease through Context Histories. Electronics 10 (11), 1260–1278. doi:10.3390/electronics10111260
Matthews, G., and Amelang, M. (1993). Extraversion, Arousal Theory and Performance: A Study of Individual Differences in the Eeg. Personal. Individual Differences 14 (2), 347–363. doi:10.1016/0191-8869(93)90133-N
Mizuno, T., and Kume, Y., “Development of a Glasses-like Wearable Device to Measure Nasal Skin Temperature,”. In Proceedings of the International Conference on Human-Computer Interaction, Bamberg, Germany, September 2015, 727–732. 2015, doi:10.1007/978-3-319-21380-4_123
Molino, M., Ingusci, E., Signore, F., Manuti, A., Giancaspro, M. L., Russo, V., et al. (2020). Wellbeing Costs of Technology Use during Covid-19 Remote Working: An Investigation Using the Italian Translation of the Technostress Creators Scale. Sustainability 12 (15), 5911–5920. doi:10.3390/SU12155911
Nozawa, A., and Tacano, M. (2009). Correlation Analysis on Alpha Attenuation and Nasal Skin Temperature. J. Stat. Mech. 2009, P01007. doi:10.1088/1742-5468/2009/01/P01007
Purwandini, S. A., Abdul, W. M. N., and Mat, Z. N. (2012). Resonant Breathing Biofeedback Training for Stress Reduction Among Manufacturing Operators. Int. J. Occup. Saf. Ergon. 18 (4), 549–561. doi:10.1080/10803548.2012.11076959
Stenberg, G. (1994). Extraversion and the P300 in a Visual Classification Task. Personal. Individual Differences 16 (4), 543–560. doi:10.1016/0191-8869(94)90182-1
Takahashi, T., Murata, T., Hamada, T., Omori, M., Kosaka, H., Kikuchi, M., et al. (2005). Changes in EEG and Autonomic Nervous Activity during Meditation and Their Association with Personality Traits. Int. J. Psychophysiology 55 (2), 199–207. doi:10.1016/j.ijpsycho.2004.07.004
Tapus, A., and Mataric, M. J. (2008), “Socially Assistive Robots: The Link between Personality, Empathy, Physiological Signals, and Task Performance,”. In Proceedings of the Emotion, Personality, and Social Behavior, Papers from the 2008 AAAI Spring Symposium, Technical Report SS-08-04, Stanford, California, USA Palo Alto, March 26-28, 2008, 133–140.
Tarafdar, M., Tu, Q., Ragu-Nathan, B. S., and Ragu-Nathan, T. S. (2007). The Impact of Technostress on Role Stress and Productivity. J. Manage. Inf. Syst. 24 (1), 301–328. doi:10.2753/MIS0742-1222240109
Tran, Y., Craig, A., Boord, P., Connell, K., Cooper, N., and Gordon, E. (2006). Personality Traits and its Association with Resting Regional Brain Activity. Int. J. Psychophysiology 60 (3), 215–224. doi:10.1016/j.ijpsycho.2005.05.008
Wallin, B. G. (1981). Sympathetic Nerve Activity Underlying Electrodermal and Cardiovascular Reactions in Man. Psychophysiology 18 (4), 470–476. doi:10.1111/j.1469-8986.1981.tb02483.x
World Health Organization (2020). Mental Health and Psychosocial Considerations during COVID-19 Outbreak, World Heal. Organ., 1–6.
Keywords: ambient intelligence, personality, mental support, individual difference, human machine interaction
Citation: Iwashita M, Ishida K and Ishikawa M (2021) Effect of User Personality on Efficacy of a Mental Support System Based on Ambient Intelligence: A Case Study. Front. Comput. Sci. 3:702069. doi: 10.3389/fcomp.2021.702069
Received: 29 April 2021; Accepted: 21 July 2021;
Published: 10 August 2021.
Edited by:
Roberto Therón, University of Salamanca, SpainReviewed by:
Antonio Coronato, Institute for High Performance Computing and Networking (ICAR), ItalyChristophe Soares, Fernando Pessoa University, Portugal
Copyright © 2021 Iwashita, Ishida and Ishikawa. This is an open-access article distributed under the terms of the Creative Commons Attribution License (CC BY). The use, distribution or reproduction in other forums is permitted, provided the original author(s) and the copyright owner(s) are credited and that the original publication in this journal is cited, in accordance with accepted academic practice. No use, distribution or reproduction is permitted which does not comply with these terms.
*Correspondence: Motoko Iwashita, bW9rbzA5MjdAZ21haWwuY29t