- 1Department of Neuroscience, Erasmus MC, Rotterdam, Netherlands
- 2Netherlands Institute for Neuroscience, Royal Academy of Arts and Sciences, Amsterdam, Netherlands
The investigation of the dynamics of Purkinje cell (PC) activity is crucial to unravel the role of the cerebellum in motor control, learning and cognitive processes. Within the cerebellar cortex (CC), these neurons receive all the incoming sensory and motor information, transform it and generate the entire cerebellar output. The relatively homogenous and repetitive structure of the CC, common to all vertebrate species, suggests a single computation mechanism shared across all PCs. While PC models have been developed since the 70′s, a comprehensive review of contemporary models is currently lacking. Here, we provide an overview of PC models, ranging from the ones focused on single cell intracellular PC dynamics, through complex models which include synaptic and extrasynaptic inputs. We review how PC models can reproduce physiological activity of the neuron, including firing patterns, current and multistable dynamics, plateau potentials, calcium signaling, intrinsic and synaptic plasticity and input/output computations. We consider models focusing both on somatic and on dendritic computations. Our review provides a critical performance analysis of PC models with respect to known physiological data. We expect our synthesis to be useful in guiding future development of computational models that capture real-life PC dynamics in the context of cerebellar computations.
1 Introduction
The cerebellum plays a central role in motor learning and cognitive processes as it crucially contributes to motor coordination, precision and timing. Purkinje cells (PC) receive all incoming sensory and motor information and generate the sole output of the cerebellar cortex (CC) (Figure 1). PCs serve as pivotal mediators, receiving and processing diverse and rich sensory and motor information. For example, it has been shown that during the eyeblink conditioning task—a classical associative learning paradigm where a previously inert stimulus, such as light, is paired with a salient air puff to evoke eyelid closure—PCs adaptively adjust their firing rates based on the timing of the stimulus by integrating input from the inferior olive (IO), eliciting blinks (Gilbert and Thach, 1977; ten Brinke et al., 2015; Cook et al., 2021). In fact, there are several examples of sensorimotor behavior where a timely adaptation of PC activity is crucial for the correct execution of movement (Gao et al., 2012). There is consensus in the field of neuroscience that the investigation of PC's responses and plasticity is essential for understanding the cerebellar circuit and motor learning.
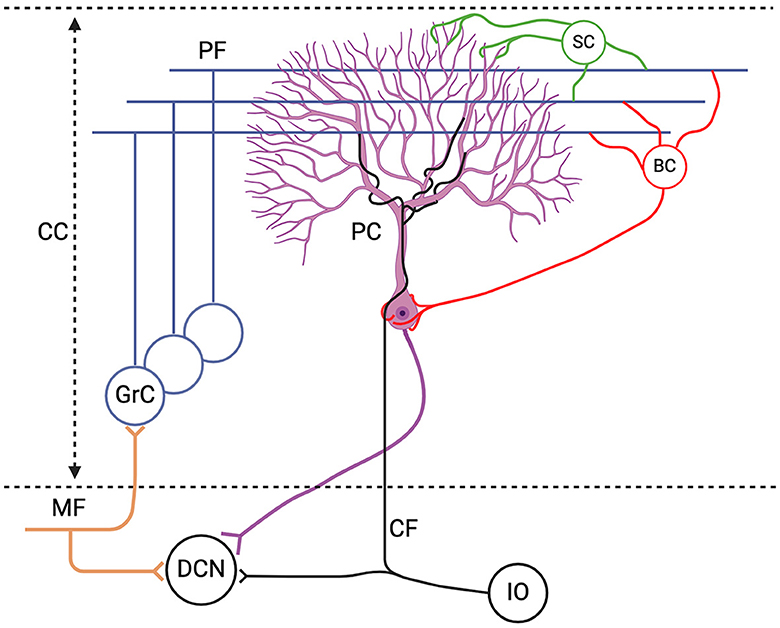
Figure 1. Schematic representation of the excitatory inputs to the Purkinje cell (PC). The PC receives excitatory inputs from: (1) parallel fibers (PF), axonal extension of granule cells (GrC) which receive excitatory signals from the mossy fibers (MF), and (2) climbing fibers (CF) from the inferior olive (IO); as well as inhibitory inputs from stellate cells (SC) and basket cells (BC) which are collectively referred to as molecular layer interneurons (MLIs). The only output of the cerebellar cortex (CC) is the PC axon which converges onto the deep cerebellar nuclei (DCN). Figure generated with BioRender.
The high degree of morphological homogeneity of the CC across vertebrate species hints at a shared computational framework. Physiologically, PCs seem to produce a wide spectrum of spiking activity involving tonic firing, different types of bursts and wide distribution of pauses. Part of this spectrum can be related to differences in excitability in connection with Aldolase C, a glycolytic enzyme related to energetic metabolism of the cell (Voogd and Ruigrok, 2004; Zhou et al., 2014). The fact that cells can be categorized in two classes—Zebrin positive (Z+) and Zebrin negative (Z-)—invites a deeper exploration of the nuanced intricacies of PC dynamics, via computational models. Notably, experimental studies have pointed to other regional differences in the cerebellum such as: differences in the connectivity patterns between PCs and GrCs across anterior and posterior lobules (Guo et al., 2016) and different PC morphologies (Busch and Hansel, 2023). For all of these divergent properties, the biophysical models can help identify the crucial parameters that distinguish these PC populations, and potentially relate them to the morphological and molecular differences.
In the mammalian nervous system, the PC stands out among the most complex and largest of neurons (Sugimori and Llinás, 1990; Miyakawa et al., 1992). Discovered in the 19th century by Jan Evangelista Purkinje (Zárský, 2012), its unique structure was later described by Golgi and Cajal. The dendritic arbor of the PC is highly elaborate, consisting of numerous branching dendrites that have a surface area 100x the size of the cell body. The PC membrane has a complex set of ion channels and gating mechanisms that regulate its activity (Raman and Bean, 1999). It emits two distinct kinds of action potentials (AP): simple spikes (SS), triggered by mossy fiber signals (MF) which are relayed via granule cells (GrC) and reach PCs via the parallel fibers (PFs), and complex spikes (CS) which are triggered by the CF input from the IO (Schmolesky et al., 2002) (Figure 1). The SS activity is also modulated by the inhibitory MLI input (Wulff et al., 2009). Experimental studies have demonstrated substantial voltage-dependent dendritic conductances in the PC, emphasizing the independent contribution of the soma and the dendritic arbors to PC activity (Llinás and Sugimori, 1980b; Davie et al., 2008).
These unique features have drawn significant attention to the PC, establishing it as one of the most frequently modeled neurons in the brain. Over the years multiple PC models have been developed, ranging from highly detailed morphologies with hundreds of compartments to more simplified models. Understanding the intricate dynamics of PC—spiking patterns, trimodal firing patterns, current x frequency behavior, multistable dynamics, plateau potentials, plasticity dynamics, and input/output computations—is crucial for unraveling the complexities of cerebellar function and advancing our knowledge of motor learning and cognitive processes. It is imperative to conduct a thorough examination of modern models to foster the future development of computational PC models. This review aims to fill this need by providing an overview of contemporary PC models. Our objective is to facilitate the critical analysis of existing proposals and ideas, enabling researchers to adapt and redesign models based on current neurophysiological and molecular data. The ultimate goal is to offer a comprehensive understanding of the relationship between PCs' dynamics and functions, allowing for the contextualization of future models and providing an overview of the current state of thought in the field.
2 Purkinje cell models
Several types of PC models exist, which we will group into a few categories. First, we distinguish between detailed (>10 compartments) and simplified (< 10 compartments) models (Tables 1, 2). As is the case for any type of model, the choice of level of detail depends on the research question(s) being tackled. Detailed models attempt to capture the rich phenomenology of PCs, while simplified models of reduced complexity capture either a specific part of behavior or explain a given phenomenon in abstract terms, often resorting to dynamical systems theory. At least two more levels of analysis are crucial to understand the role of the CC in motor control, the plasticity of the PC in response to the GrC input and the activity of PCs across cerebellar networks at large. These levels give rise to the categories of synaptic and network models (Tables 3, 4). Importantly, experimental data has shown that connections with molecular layer interneurons (MLIs) are also plastic (Bao et al., 2010; Brown et al., 2019; Arlt and Häusser, 2020), but these properties have not yet been included in most of the PC models. Finally, we give an overview of a category of more traditional, abstract models based on the perceptron analogy, or what is now called a feed forward neural network. Perceptron-like models are useful to quantify metrics like the information capacity (in bits) of the PC to encode patterns (Table 5).
2.1 Detailed models
In the 1970s, the increasing understanding of the composition, dynamics and cellular structure of the PC led to models incorporating high levels of biological detail. This development was largely possible thanks to the detailed Purkinje cell morphologies revealed by Golgi (Pellionisz and Llinás, 1977; Shelton, 1985) and horseradish peroxidase stainings (Rapp et al., 1994) as well as the advancements in the electrophysiological recordings. The integration of information in the complex dendritic tree of the PC was a focus of the early models, which incorporated passive electrical properties and detailed information about active ion channels kinetics. Some of the earlier models studying these properties with a realistic full scale morphology were based on the frog PC anatomy (Pellionisz and Llinás, 1977), or that of a guinea pig PC (Shelton, 1985). The Shelton model used Hodgkin-Huxley (HH) equations for channel kinetics, which at that time were gaining popularity among their contemporaries but still lacked experimentally verified parameters and were very computationally demanding. Bush and Sejnowski (1991) introduced Markov schemes to model channel kinetics, while using the same morphology as Shelton. Following these two, a landmark PC model was developed by De Schutter and Bower—the DB model (De Schutter and Bower, 1994a,b) comprising 1,600 compartments—this large detailed model was reconstructed from earlier morphological observations (Rall, 1964). Ten different ion channels were differentially distributed over the soma, the smooth dendrites and the spiny dendrites. Channel kinetics were computed using HH equations, using parameters observed in different in vitro voltage-clamp studies done in different animal species and different neuronal cell types. One of the model's breakthrough accomplishments was an accurate reproduction of spiking frequency to current injection amplitude. The model started to fire abruptly with a minimum firing frequency around 30–40 Hz, after which it produced linear firing rate increases up to saturation. This very closely reproduced data recorded in vitro from acute cerebellar slices (Raman and Bean, 1999). Dendritic and somatic spiking features were effectively captured by the model explaining the dynamics of increase of Ca2+ concentration over the dendritic arbor upon CF input, which had previously been shown experimentally (Miyakawa et al., 1992).
Miyasho took the DB model as their starting point and implemented new experimental insights into PC physiology, resulting in a 1,088 compartmental model (Miyasho et al., 2001). Several ion channel kinematic equations were modified, such as the P-type Ca2+ channels and delayed rectifier potassium (K+) channels. Furthermore, two low threshold channels, class-E Ca2+ and D-type K+ channels, were added to the model. Sensitivity of Ca2+ activated K+ channels was lowered compared to the DB model, allowing repetitive Ca2+ spiking. These updates made the PC replicate in vitro behavior, where, upon blocking voltage dependent sodium (Na+) channels with tetrodotoxin (TTX), the cell showed repetitive Ca2+ firing (Miyasho et al., 2001). The Miyasho model especially reliably mimicked the dynamics around the generation of Ca2+ spikes in the dendrites, replicating pharmacologically induced changes of channel dynamics seen in vitro (Llinás and Sugimori, 1980b). However, despite the updates, clear differences still remained with respect to experimentally measured PC physiology. Specifically, (1) the intracellular Ca2+ concentrations were not realistically estimated, and (2) the sensitivity to calcium of Ca2+ activated K+ channels was incorrectly estimated, and the channel distributions were considered uniform over dendrites. Furthermore, the complexities of axial and radial calcium diffusion were not incorporated in this model. Those were later shown to account for CS waveform variability, and non-linear dendritic spiking and signal amplification in another detailed PC model published in 2013 (Anwar et al., 2012).
The dendritic model of Miyasho was updated in 2012 to simulate 21 gated ion channels using the morphology earlier published by Shelton (Forrest et al., 2012). Forrest combined two models to make a 1,089 compartmental model: Miyasho's dendritic channels with a highly detailed dendritic model, and the somatic model from Khaliq (Khaliq et al., 2003). The latter simulated TEA-sensitive, TEA-moderately sensitive and TEA-insensitive voltage-gated K+ currents, BK voltage-gated Ca2+-gated K+ channels, hyperpolarization-activated mixed cation current (Ih), I-leak, resurgent Na+ current, P-type Ca2+ currents as well as intracellular Ca2+ dynamics. Moreover, the authors added the SK Ca2+-gated K channels to the soma, previously modeled in the midbrain neurons (Komendantov et al., 2004). Forrest also incorporated the Ih currents (Saraga et al., 2003), as well as the IKv1 channels (Akemann and Knöpfel, 2006), to the dendritic channels of the Miyasho model. Further additions by Forrest were: the inclusion of the Na+/K+ pumps (both Na+ and voltage dependent), with slight dynamical differences between the pumps located in the soma and the dendrites; a Na+/Ca2+ exchanger; an abstract representation of intracellular Na+ and extracellular K+ concentrations. Notably, Forrest's model was constructed to explain activity of isolated PC's from in vitro studies, in which synaptic inputs were compromised. PCs in this disembodied state show a repeating trimodal firing pattern, alternating between tonic firing, bursty firing and quiescence (Womack and Khodakhah, 2002b, 2003, 2004). Furthermore, this model incorporated Na+ spikes in the soma and Ca2+ spikes in dendrites and replicated experimental results in which pharmacological block of Kv1.2 channels increased the Ca2+ spike frequency in the dendrites and lowered the number of somatic spike bursts in the trimodal pattern. Forrest's results uncovered the intracellular PC dynamics governing the trimodal spiking phases, showing the impact of the Na+/K+ pump in trimodal firing and replicated in vitro results in which the pump was pharmacologically blocked. In the same paper, Forrest also reduced the 1089 compartmental model to two simpler models-−41 and 5 compartments respectively—being able to show the same spontaneous firing in the trimodal pattern activity. Forrest further expanded the 41 compartmental model in 2014, where he described a PC model with modifications to the implementation of the tonic to burst transition in the trimodal pattern by removal of one Na+/K+ pump and of the SK channel, as well as adding other HH dynamics (Forrest, 2014). This model reproduced the quiescent periods as observed in vivo (Loewenstein et al., 2005), and showed that CF input could dictate both the timing and duration of these periods.
Another model based on a detailed reconstruction of the PC morphology was proposed in 2015 (Masoli et al., 2015). It presented an extensive multicompartmental PC model with an intricate axonal compartment. The authors added Axonal Initial Segments (AIS), the site of action potential (AP) initiation located at the base of axons (Somogyi and Hámori, 1976). Moreover, the Masoli model also included three nodes of Ranvier (RN) separated by myelinated sections. This detailed axon representation was modeled with a set of 15 different subtypes of Na+, Ca2+ and K+ ionic channels, combined with internal Ca2+ dynamics. The authors showed that the firing of the PC is intrinsic, and relies on Na+ channel dynamics in the AIS, and P-type Ca2+ channels in the dendrites. Absence of either one of these channel types caused the model to become bistable, resulting in the possibility of the PC to switch to a quiescent state, as had been observed in anesthetized mice (Loewenstein et al., 2005). Masoli's model also exhibited a switch from tonic firing to complex bursting at higher input frequencies in response to high current injections, corroborating experimental findings (Kim et al., 2012). Intriguingly, in Masoli's model the APs could be triggered during complex bursts by dendritic depolarization currents. This could imply secondary spike generation in RNs, though it currently lacks experimental verification.
In 2018, Zang et al. (2018) published a substantially modified model based on the DB model. Here, the authors extensively updated the model and tuned it to recent experimental data, integrating new knowledge about ionic channels distributions and Ca2+ diffusion. Among other predictions, this allowed the authors to calculate the metabolic cost of PC activity. In the Zang model, CS consumed more adenosine triphosphate (ATP), resulting in the energy equivalent of ~40 SS. This high energetic cost of CS activity might help explain the negative correlation found experimentally between the firing rates of SS and those of CS (Cerminara and Rawson, 2004). This fact could reflect a homeostatic, protective mechanism inside the cerebellum to abide by its limited energy supply. The model also showcased that voltage-dependent K+ currents in the PC dendrites were essential in gating the spatial range of dendritic responses evoked by CFs. According to the Zang model, all synaptic inputs to the PC and the SS firing rate contribute to learning occurring inside the PC. Furthermore, the time interval between SSs and the CF input modulated the amplitudes of the spikelets after CF input, indicating that the response to CF input depends on the SS activity. According to this model, the dendritic arbors exhibit heterogeneous excitability, implying the existence of computational units at the level of individual branches. The question of PC dendritic compartmentalization was recently examined in more detail by the same authors (Zang and De Schutter, 2021). With slight changes to spiny dendrite conductances, this updated model was able to reproduce PF-evoked dendritic spike pauses similar to the experimental data (Rancz and Häusser, 2010), without affecting the findings of the 2018 version of the model. Individual dendritic branches could either exhibit linear or burst-pause coding behavior, showcasing the computational unit of the individual branch. According to the updated model, the PF-evoked dendritic spikes trigger Ca2+ influx in the branch, leading to long and/or short term depression (STD) at the PF-PC synapse. The modeled dendritic dynamics were context dependent, which allowed the PC to encode a wide range of behavioral properties by generating somatic SS burst-pause sequences when dendritic spikes occurred. When paired with CF input, Zang's 2021 model initiated cerebellar LTD in line with recent experimental findings (Rowan et al., 2018; Silva et al., 2024).
While detailed models are able to capture many aspects of PC dynamics, they are computationally expensive even today, a fact that limits their applicability to small- to medium-scale simulations and leads many researchers to focus on simplified modeling approaches.
2.2 Simplified models
The goal of the simplified models is to reduce complexity so as to capture either a specific part of PC spiking behavior or explain the phenomena in abstract terms. Usually this implies a heavily simplified morphology and/or ion channel make-up. This renders the models less biologically accurate but better suited to dynamical systems analysis of spike generation mechanisms, and in addition, due to reduced computational costs, usable in larger scale networks.
Khaliq, Gouwens and Raman constructed a single-compartment model of the soma to study the high-frequency SS firing observed in the PCs [(Khaliq et al., 2003); the KGR model]. This model is based on experimental results and previous modeling efforts from the same group that showed that resurgent Na+ currents were associated with a rapid recovery from inactivation and were thus important in sustaining high SS firing frequencies (Raman and Bean, 1997, 2001). The resurgent dynamics were incorporated in the model with TTX-sensitive Na+ channel kinetics, mainly the NaV1.6 channels. The authors decided to leave several ion channels out of their model. T-type and A-type currents were not incorporated due to the fact that their voltage-based activation and inactivation kinetics were unlikely to play a major role in spontaneous and current evoked firing of isolated PCs. This simplified model encompassed in total 8 ionic currents. The TTX-sensitive Na+ currents (of which the NaV1.6 channel was the main contributor) were calculated using Ohm's law, multiplying the ratio of open channels by the driving force and the maximal conductance observed (Raman and Bean, 1997). All other channels were modeled using HH simulations with parameters obtained from experimental data; the P-type Ca2+ current dynamics were modeled based on an older experimental dataset (Raman and Bean, 1999). The KGR model simulated the Ca2+ concentration in a 100 nm shell underneath the cell membrane. Resulting spontaneous SS firing was ~27 Hz, and the Na+ currents with a resurgent component contributed to PC excitability. Finally, the KGR model was used to simulate PC activity in an ataxic knockout mouse line lacking the expression of the NaV1.6 channels PCs. To simulate these mutant PC cells, the Na+ channels were tweaked to have faster inactivation rates and slower transition to a blocked state, so that they closely mimicked the recorded currents in mutant cells. This altered model indeed showed that a reduction in SS firing frequency depends on the Na+ current with a resurgent component, mediated by the NaV1.6 channels.
An adjusted version of the KGR model was later used to study the effect of a K+ channel, Kv3.3, on spontaneous firing in PCs (Akemann and Knöpfel, 2006). The authors updated the three voltage-gated K+ channels, used in the KGR model, to molecularly identified K+ channels. Furthermore, they included both the resurgent and non resurgent Na+ channels, which were simulated separately in the KGR model. The Akemann model also included a P-type Ca2+ current, a Ca2+-activated K+ current, and an Ih current, with conductances based on kinetics previously established by the KGR model. The K+ channels of the Kv1 and Kv4 type were simulated using HH equations. The Kv3.3 currents—characterized by high activation threshold, fast activation and deactivation—recapitulated findings from the dynamic patch clamp experiments. Among several important findings of this model, the simulations revealed that Kv3.3 currents could only substantiate the higher firing frequencies by cooperation with resurgent Na+ currents, inducing a tonic inward Na+ current which drove the intrinsic firing of PCs.
Simplified models were also developed to explore computational principles in the PC dendrites. Genet and Delord used a single-compartment model to simulate nonlinear dynamics underlying plateau potentials and Ca2+ spikes in the PCs dendrites [(Genet and Delord, 2002); GD model]. Plateaus are depolarizations that outlast the end of their triggering stimulus and last from tens of milliseconds to seconds (Llinás and Sugimori, 1980b). These plateaus, as well as dendritic spiking, rely on voltage dependent P/Q-Ca2+ channels. The GD compartmental model further included a simplified calculation of internal Ca2+ regulation and three ionic currents: P-type Ca2+ current, delayed-rectifier K+ current and a generic class of K+ channels activating sharply in the sub-threshold voltage range. It successfully replicated experimentally observed spontaneous transitions between Ca2+ plateaus and Ca2+ valleys, which for example the DB model failed to show (De Schutter and Bower, 1994a,b). Using a dynamical systems approach the GD model explained how the plateau and valley states in the dendrites were correlated to the input currents. Interestingly, the model's only Ca2+ channel was the P-type Ca2+ channel, in agreement with experimental data showing that indeed the P-type Ca2+ channels constitute the major part of the Ca2+ currents (Usowicz et al., 1992). In comparison, the detailed model of Miyasho discussed above, also included E and D type Ca2+ currents. The GD model showed that brief depolarizing currents could trigger long after-depolarizations that resemble experimental plateaus in the absence of the ‘long' inactivating time constants provided by the E and D type Ca2+ channels. Explorations of the model showed that slow intracellular Ca2+ increases in the sub-threshold voltage range decreased the Ca2+ Nernst potential, thereby reducing the magnitude of Ca2+ current on a time scale of hundreds of milliseconds. Notably the K+ channels in the GD model were generic, as the authors argued that the dendritic function of distinct K+ channel subclasses was not understood well enough to be modeled separately.
Interest in the Ca2+ channel dynamics in the dendritic arbor led to the development of a new single dendritic compartment model (Anwar et al., 2012), which was later computationally optimized to simulate realistic Ca2+ diffusion (Anwar et al., 2013). This updated model incorporated stochastic spatially arranged ion channels, and stochastic intracellular Ca2+ dynamics. Most full-scale models assume voltage-gated computations that represent average kinetics over many channels. For whole-cell models this is justified because fluctuations resulting from stochastic gating kinetics in small systems tend to become negligible. However, the stochastic behavior of channels in the PC dendrites, together with the complex morphology of the PC dendritic arbor led to high variability in dendritic spikes triggered by CF-mimicking input (Anwar et al., 2013). This high temporal and spatial variability in Ca2+ levels observed in dendritic arbor simulations could have great functional significance on e.g. short-term plasticity mechanisms.
As mentioned above in the description of the detailed models, the 2012 Forest model, also reduced the full 1089 compartment model to 2 simplified models of smaller morphological complexity to decrease the computational demand (Forrest et al., 2012). Both reduced models were able to conserve reproducibility of the full model with simpler channel compositions. The simplified models were used to study CF input-dependent changes in the stable-to-bistable PC activity state, as observed in experimental studies (Loewenstein et al., 2005). The reduced models explored the Ca2+ dynamics, in which intracellular Ca2+ concentration prior to the CF input determined the balance between excitation or hyperpolarization upon CF input. Forrest's simulations assumed a CF-specific activation of Ca2+ responsive SK K+ channels by means of channel co-localization, resulting in a hyperpolarizing current. If prior to the CF input, the neuron was tonically firing there was a high dendritic internal Ca2+ concentration. The CF input, opening Ca2+ channels, resulted in high Ca2+ concentrations leading to a large Ca2+ activated K+ current hyperpolarizing the PC, which then entered the quiescent state. In the quiescent state the Ca2+ concentration receded, and therefore the Ca2+ concentration in the dendrites upon CF input in this state is relatively smaller. This results in subsequent hyperpolarizing current through Ca2+ activated SK potassium channels being smaller than in the Akemann 2006 model (Akemann and Knöpfel, 2006).
More recently, two single-compartmental models were developed to examine if essential aspects of the PC CS-waveform can be generated by a limited number of somatic channels (Burroughs et al., 2020). The models examined were (1) a 5-current model which contained Ca2+, Na+ and K+dynamics; and (2) a reduced 3-current model, which only contained Na+ and K+ dynamics. The Na+ current was modeled after the Markovian scheme of Raman and Bean (1997) while the K+ current was modeled after the previously discussed Masoli model (Masoli et al., 2015). The Ca2+ dynamics in the 5-current model were based on the Miyasho model (Miyasho et al., 2001) with an addition of an SK channel implemented with dynamics from Gillies and Willshaw (2006). The final addition was a base input current combined with a CF input current. This CF current was modeled as a combination of two exponentially decaying inputs in line with experimental data on CF synaptic input (Davie et al., 2008). Both the 3- and 5-current models were able to simulate general CS behavior, causing a high amplitude of the initial spike followed by spikelets. Additionally, the 5-current model allowed for the CF input to influence the SS rate, causing depression after a CS and suggesting that the SK and Ca2+ dynamics are essential for CS induced depression as observed in vivo (Schmolesky et al., 2002).
2.2.1 Bistability
In vitro experimental data show that the passive firing of PCs can follow a trimodal firing pattern of tonic firing, burst firing and quiescence (Llinás and Sugimori, 1980b; Raman and Bean, 1997). Bistable patterns toggling between tonic firing and quiescence have also been observed in anesthetized mice (Loewenstein et al., 2005) as well as awake cats (Yartsev et al., 2009). However, despite sparking interest guided by these initial observations, bistability—switching between prolonged depolarized (up) state, and periods of hyperpolarization (down state)—has never been reported as a correlate of behavior, nor has it been observed in awake behaving mice (Schonewille et al., 2006) as it seems to be modulated by anesthesia (Engbers et al., 2013). Nevertheless, in vitro experiments show that bistability is within the scope of PC dynamics, and gives clues to its dynamical responses.
A few reduced dynamical models have been used to explain bistability by using slow state variables such as Ih or Ca2+ currents (Loewenstein et al., 2005). Fernandez produced a model with only four voltage-gated conductances (Fernandez et al., 2007), and electrically coupled dendrites and soma, to reproduce the relative depolarized state of the dendrites during the AP measured in the soma (Stuart and Häusser, 1994; McKay and Turner, 2004). The Fernandez model depended on a saddle node bifurcation to account for transitions between rest and firing state (such as the low firing range < 40 Hz in the bistable range, expected from the dynamical system). This model displayed tonic activity with frequencies up to 150 Hz and replicated dynamical system characteristics underlying spike behavior as observed experimentally in PCs, such as a highly nonlinear I-V relationship near threshold and the generation of long delays to first spike compared to the average ISI time
To study the dynamical states of the PC, Fernandez and colleagues constructed a simplified two-channel model to investigate if the CF input could trigger state transitions in a bistable region (Engbers et al., 2013). This resulted in transitions from rest to firing, and vice-versa, together with a long delay for the first spike. The commanding factors underlying bistability in this model were: (1) the (large) membrane time constant, and (2) spike refractory dynamics that generated large enough depolarizing after potentials that triggered the following spikes leading to a persistent activity. The positive current flowing from dendrites into the soma was also found to contribute to bistability. The Ih current was not directly involved in establishing bistability dynamics, but rather limited the range of bistability in the phase plane. This somewhat contradicts model simulations by Loewenstein et al. (2005) that assumed I h to be a main player in establishing bistability.
In 2010 Genet expanded on the 2002 GD single-compartment simplified model adding a full dendritic morphology (Genet et al., 2010). Analysis performed with the 2010 Genet model suggests that the dendritic Ca2+ plateaus and valleys, triggered upon PF stimulation, respectively, causes periods of firing and silencing of PCs (bistable state).
An altered and reduced version of the original 2012 Forrest model also implemented bistable PC behavior (Forrest, 2014). Here, the authors altered the mechanisms of the tonic to burst transition using a D-Type K+ current, called ID with an added inactivation rate modulation parameter (k), as well as additional mechanics for plasticity in the baseline intracellular Ca2+ concentrations. The updated Forrest model showed that PF inputs could alter the frequency of PC SS firing, but not the pattern of firing. Moreover, the updated internal Ca2+ dynamics allowed the simulated PCs to toggle between up and down states.
The most recent model of bistability was proposed by Buchin et al. using an experimentally tuned adaptive exponential integrate-and-fire model (aEIF) with type II excitability and nonlinear voltage dynamics (Buchin et al., 2016). In contrast to Forrest's models, Buchin's model relied upon an inverse stochastic resonance depending on noise variability for bistability rather than an internal Ca2+ dynamics. Whether these mechanisms best explain PC bistability remains an open question.
2.3 Synaptic models
Due to its imputed role in learning, plasticity at the PF synapses (Figure 2) has been the focus of multiple modeling forays. Whereas some models focused solely on the integration mechanisms in the PC dendritic arbor, others simulated the dynamics of the molecular pathways underlying the plasticity rules in dendritic spines. One of the first synaptic models examined how glutamate activates metabolic glutamate receptors (mGluRs), leading to cytoplasmic Ca2+ fluctuations, which vary greatly in timing across different PCs and potentially encode the timing for stimuli in classical eyeblink conditioning (Fiala et al., 1996). This model suggested that effective eyeblink learning occurred in PCs when Ca2+ response and cyclic guanosine monophosphate (cGMP) production were simultaneous, a result of cerebellar input. It also showed that the response delay—ranging from 100 ms to several seconds—is due to the sequential activation of phospholipase C (PLC), the generation of inositol (1,4,5)-trisphosphate (IP3), and the release of IP3-induced Ca2+. Steuber later simplified this model, identifying critical elements like Ca2+ dependent feedback and autocatalysis that influence these delays, which also vary with mGluR density (Steuber et al., 2006). In parallel, efforts were made to refine the dendritic model to explore the synaptic detection input coincidence and the role of the IP3-induced Ca2+ release in synaptic plasticity (Doi et al., 2005).
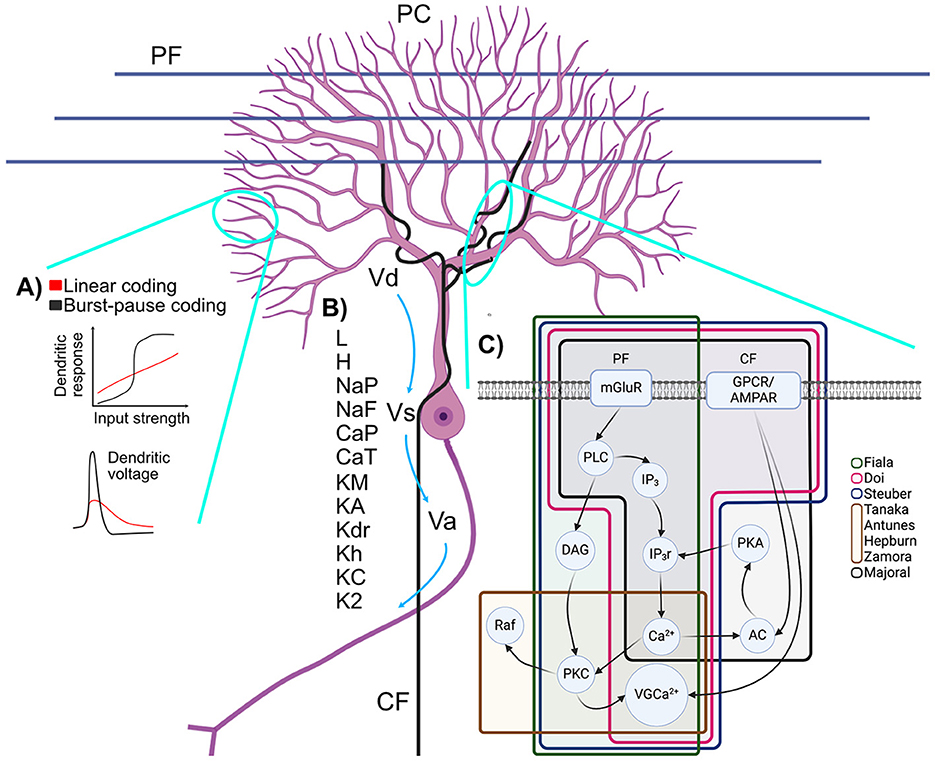
Figure 2. Schematic representation of (A) branch-dependent bimodal computations, (B) membrane potentials and ionic channels of PC models and (C) simplified molecular pathway involved in LTD and the PF-PC synapse. (A) (TOP) Multiple encoding mechanisms have been proposed for the PC: a linear firing rate response to increasing current and a pause duration mechanism. (BOTTOM) EPSCs in spiny dendrites are nonlinear and may or may not produce spikelets (adapted from Zang and De Schutter, 2021). (B) Dendritic (Vd), Somatic (Vs) and Axonic (Va) membrane potentials and their various ionic channels: leak current (L), hyperpolarization-activated current (H), persistent Na+ current (NaP), fast Na+ current (NaF), P Ca2+ current (CaP), T Ca2+ current (CaT), persistent K+ current (KM), A K+ current (KA), delayed K+ rectifier (Kdr), anomalous K+ rectifier (Kh), BK calcium-activated K+ current (KC), K2 calcium-activated K+ current (K2). (C) Starting from PF: mGluR activates the PLC enzyme which yields two products, IP3 and diacylglycerol (DAG). IP3 activates the opening of IP3r which liberates Ca2+. DAG and Ca2+ activate PKC. Ca2+ from CF and from IP3r stimulate AC which activates PKA, opening more IP3r through positive feedback. PKC and signals from GPCR or AMPAR from the CF activate voltage-gated Ca2+ channels (VGCa2+). Figure generated with BioRender.
A highly detailed biochemical dynamical model of long-term depression (LTD) at the PF-PC synapse was introduced in 2012 (Antunes and De Schutter, 2012). It was based on an earlier model by Tanaka et al., which highlighted a feedback loop involving protein kinase C (PKC), extracellular signal-regulated kinase (ERK) and cytosolic phospholipase A2 (cPLA2), critical for LTD (Tanaka et al., 2007). The Tanaka model underscored the probabilistic nature of LTD induction, as demonstrated in experimental Ca2+ uncaging experiments performed by the same research group. The critical modification of the Antunes model was the inclusion of α-amino-3-hydroxy-5-methyl-4-isoxazole propionic acid (AMPA) receptor trafficking and the prediction that the induction of LTD is all or none. A competing LTD model that combined in a single model the biochemical pathways leading to LTD and the active ion conductances—described in a previously published detailed model (Miyasho et al., 2001)—was described a year earlier (Brown et al., 2011). The Brown model explored the dynamics of phosphatidylinositol-4,5-bisphosphate (PIP2) signaling in PCs, which is a molecular pathway element needed for the production of IP3 (Brown et al., 2008). However, in contrast to the previously described models (Tanaka et al., 2007; Antunes and De Schutter, 2012), it did not include the positive forward loop in the LTD induction.
Next to models that focused on LTD-relevant molecular pathways, attention was also given to the morphology of the individual dendrite diameters and their impact on the Ca2+ diffusion (Anwar et al., 2014). Notably, earlier work from the same research group did not account for the variability of the diameter of the compartment. In the 2014 Anwar et al. paper, the De Schutter laboratory re-examined their earlier work (De Schutter and Bower, 1994b; Anwar et al., 2012), comparing the diffusion errors of 3D and 1D models. They found that the errors from incorrect 3D morphology were higher than the errors caused by the 1D simplification. They also concluded that 3D diffusion is essential for synaptic plasticity and proper modeling of Ca2+ signaling pathways.
Multiple synaptic models focused on the mechanisms with which the PCs adapt to external stimuli. Hepburn updated the 2012 synaptic model from De Schutter (Hepburn et al., 2017), to include the Raf kinase inhibitory protein (RKIP) to align with experimental findings (Yamamoto et al., 2012). It also updated the Ca2+ dynamics with a fitted rate response to correspond better to existing experimental data. The Hepburn model showed that AMPA receptor (AMPAR) interactions increased robustness of the LTD at the PF-PC synapse, and that LTD probability depended on the amount of PKC. In 2018, the Hepburn model was updated by adding the activation and regulation of the α-calcium/calmodulin-dependent protein kinase II (αCaMKII) (Zamora Chimal and De Schutter, 2018). In accordance with the experimental data (Hansel et al., 2006), LTD in this model was abolished when αCaMKII was not present. Moreover, because ERK, PKC and αCaMKII responded differently to stimuli with varying frequencies, the modeled molecular dynamics of these proteins caused cerebellar LTD to be frequency sensitive.
The TRACE model (Narain et al., 2018), which is based on experimental findings, indicates that interval learning in eyeblink conditioning results in Bayesian-like estimation computed in the cerebellum. The model by Narain has two components: the commonly described LTD, and the less frequently considered long-term potentiation (LTP). The LTD and LTP changes at the GrC-PC synapse changed the PC's SS activity, resulting in a Bayesian Least Squares like estimation through integration of the PC input downstream at the deep cerebellar nuclei (DCN) level. While the Narain model successfully reproduced experimental eyeblink conditioning results, several experimental investigations had shown that the LTD at the PF-PC synapse is not required for cerebellar learning (Schonewille et al., 2011; Johansson et al., 2018). The extent to which LTD is required for cerebellar learning remains the subject of a debate in the cerebellar field.
The experimental data questioning the role of PC-LTD in cerebellar learning led to the development of models which rely on intrinsic plasticity. Majoral and colleagues designed a model in which the Adenylyl cyclase (AC) functioned as a coincidence detector between Ca2+ generated from PF input and the G-protein mechanisms triggered by the CF input (Majoral et al., 2020). This coincidence detector functions as a positive feedback loop for low Ca2+concentrations, while the higher Ca2+ concentrations inhibit the G-protein preventing further activation. The combination of positive and negative feedback loops allows for successful modeling of time intervals. However, the Majoral model simplified the connection between the CF and the dendritic arbor to just the G-protein connection and currently still lacks in vivo experimental verification.
The PC has been observed to produce pauses of different durations during eyeblink conditioning, in the absence of MLI inhibition (Johansson et al., 2016). This led Johansson and colleagues to propose intrinsic PC molecular mechanisms as the source of learning. A biochemical pathway that could regulate intrinsic plasticity capable of simulating timing intervals was related to the activation of mGluR7 channels (Mandwal et al., 2021). In this Mandwal's model, response time could be encoded in the rate constants arising from mGluR7 mechanisms. Unique to this model is the description and implementation of the G-protein inward rectifier K+ (GIRK) ion channels, whose role in PC-dependent learning also is supported by experimental data (Johansson et al., 2016).
2.4 Network models
Purkinje cells' responses vary widely in terms of firing rate modulation, pause duration and morphology. As populations of PCs converge on DCN cells, it becomes relevant to examine collective PC behavior in network models. It is likely that neighboring PCs aligned to PF beams have the highly shared input, which was shown to lead to correlated PC activity in vivo (Heck et al., 2007; Cao et al., 2017). Simulations of PC activation over PF beams with the unchanged detailed DB model were used to see if volleys traveling along the beams leads to synchronous active PCs (Jaeger, 2003). About 3 % (~4,500 inputs) of all PFs being active would be sufficient to trigger time-locked PC firing along the PF beam, a fact somewhat aligned with experimental data (Isope and Barbour, 2002). Notably, experimental work presented in the article by Jaeger showed a lack of synchronicity and no narrow cross-correlation peaks between PCs at distances >0.1 mm along the PFs. This discrepancy likely reflects the influence of experimental parameters, notably the PF's feedforward inhibition provided by MLIs (Santamaria et al., 2007). Recent experimental data suggest that even though the PF input might be sparse it can still support very rich stimulus representations (Lanore et al., 2021).
The DB model was also used in a spatial integration study, which analyzed spiking behavior of a single PC receiving different input patterns from PFs and MLIs (Santamaria and Bower, 2005). This study into network dynamics showed that the baseline somatic firing rate of PC is independent from the response to the synaptic inputs. The modulatory effects of the inputs on the SS activity were found to be dominated by dendritic Ca2+ and calcium-activated K+ currents.
A network model consisting of 50 PCs inhibited by parasagittal projecting MLIs and excited by PFs, yielded PC responses to PF outlasting its input for up to 10 s (Maex and Steuber, 2013). Within this network, PFs delivered transient activity to ‘on-beam' PCs upon a continuous background input over all PFs. Single PCs were based on a trimmed version of the DB model with 196 compartments, enhanced with a modeled axon (Maex and De Schutter, 2007) and an Ih-current (Roth and Häusser, 2001) introduced to all compartments. The trimmed PC model further lacked the resurgent Na+ current (Khaliq et al., 2003), and non-inactivating persistent Na+ channel. It did however increase the conductance of several channels: (1) the peak conductance of fast Na+ channel (NaF) was amplified by 20%; (2) delayed rectifier K+ current was doubled; (3) P-type Ca2+ and Ca2+-dependent K+ channels were increased by 40% and 20%, respectively.
The spontaneous firing rate of the 2013 Maex model was set to 71 Hz, but negative current injections would result in very low firing rates. Simulations showed that the PC response only outlasted its stimulus if the PC basic firing rate was in a low frequency range (< 30 Hz). The prolonged excitable states resulted in an increase in membrane voltage compared to simulation runs lacking these excitable states. Further investigation into the observed increased excitability of the PC in this model, revealed that the fast Na+ channels (NaF) were responsible for this more depolarised PC state. The level of prolonged excitability was dependent on the PCs basal SS firing rate controlled by PF background activity. Interestingly, the PCs in the 2013 Maex network model showed bistability but only in the sub-millivolt range, which is in stark contrast to the large membrane voltage differences reported by the “classic bistability” PC models (Loewenstein et al., 2005; Fernandez et al., 2007).
Remarkably, other detailed PC models have also been used to study network effects. For example, simulations using the previously described 2012 Forrest model showed that MLI-like GABAergic inputs can switch the PC from a trimodal firing mode into a bimodal firing mode, a finding consistent with experimental observations. Specifically, the GABAergic MLI-like network input was found to be able to block dendritic Ca2+ spikes, which would trigger the quiescence.
While the focus of this review is on the PC synapses, other sites of cerebellar plasticity are likely to contribute to motor adaptation. A network model from Clopath et al. (2014) with LTD/LTP plasticity at the PF-PC and plasticity at MF-medial vestibular nuclei (MVN) synapse, simulated vestibular ocular reflex (VOR) adaptation experiments, reliably reproducing experimental data from wildtype mice (Clopath et al., 2014). This model was later used to study learning impairments in six cerebellar mutant mice with impaired motor learning (Badura et al., 2016). The 2014 Clopath model reliably reproduced behavioral deficits as well as PC SS activity abnormalities observed experimentally. Importantly, lack of LTP at the PF-PC broke the Clopath model —where the model does not show any activity— suggesting that it plays an important role in cerebellar-dependent motor learning. This is in line with other experimental evidence showing that LTP is required for procedural learning (Schonewille et al., 2010; Gutierrez-Castellanos et al., 2017).
Electrophysiological measurements of the excitatory (GrC-driven) and inhibitory (MLI- driven) inputs onto the PC were incorporated into a model that explored pre- and postsynaptic plasticity dynamics at the PC synapse (Grangeray-Vilmint et al., 2018). It demonstrated that SS PC firing rate was sensitive to the duration of the GrC burst activity, and that different PCs could either decrease or increase the SS firing upon a similar GrC input, based on the state of the PC synapse. Interestingly, these features were also observed in vivo in rhesus macaque PCs during saccadic eye movements (Herzfeld et al., 2015).
In 2019 a spiking cerebellar network model was introduced, where the PCs were based on a modified 2001 Miyasho model, simplified to allow for fast spiking neural network simulations (Luque et al., 2019). This simulated cerebellar network consisted of 100 MFs, 2 CFs, 1,000 GrCs, 20 PCs and 2 MVN cells. It was used to examine how the spike-burst mechanisms can cause LTP blockades during rapid eye movement sleep. This finding was highly relevant to the experimental cerebellar researchers as it offered an explanation for the consolidation of the VOR phase reversal learning, which had been shown to occur overnight (Clopath et al., 2014; Badura et al., 2016). The Luque model also suggested that the pauses following CS bursts in PCs gate the vestibular signals, which accounts for early stages of VOR learning. Altogether, these results predicted that PC SS burst-pause dynamics are instrumental to VOR learning and reversal adaptation. In 2022, a network model for a VOR experiment was performed by Luque and colleagues using Luque's 2019 model. Here, they studied VOR during aging and found that LTP is likely acting as a global homeostatic mechanism that counters age-related vestibular neuroanatomical losses. The 2022 analysis also suggested that VOR is sustained at older ages because the intrinsic plasticity of the PC synapses can operate as a local homeostatic mechanism (Luque et al., 2022).
A different approach to simulating large-scale cerebellar dynamics used modified integrate-and-fire neurons (Fourcaud-Trocmé et al., 2003) to model a network of 50 PCs, 500 MLIs, 500 MFs and 1,000 GrCs (Tang et al., 2021). In this model, each PC received excitatory synaptic input from 100 GrCs and inhibitory input from 8 MLIs. The PCs firing rate and pausing was modulated through a mixture of short term potentiation (STP) on the GrC-PC synaptic connection combined with feedforward inhibition through the MLIs. Extensive simulations showed that the nonlinear characteristics of excitatory STP dynamics significantly modulated PC spiking, mediated by inhibition. In fact, the feedforward inhibition was essential to the PC modulation as the pause response shown in the PC network can only emerge with the interaction of both pathways. This is in line with experimental findings that underscore the essential role of the interaction between excitation and inhibition in the cerebellar cortex (Kim and Augustine, 2021).
In 2023, a network model based on the 2018 Grangeray-Vilmint model was used to investigate a temporal organization of the feedforward inhibitory microcircuit between GrCs, MLIs and PCs (Binda et al., 2023). Using ex vivo patch-clamp recordings of PCs and the Grangeray-Vilmint model, it was shown that the encoding of specific MF inputs by PCs can be aided by specific excitatory and inhibitory delays.
The PF-PC synapses undergo both LTP and LTD (though not simultaneously), and these plasticity mechanisms in general counterbalance each other. It has been demonstrated experimentally that different cerebellar zones undergo different opposite directions of plasticity (De Zeeuw, 2021). A recently published olivocerebellar network model incorporated bidirectional plasticity in cerebellar learning by embedding upbound (mainly Z+ PCs) and downbound (mainly Z- PCs) zones that have different plasticity rules (Geminiani et al., 2024). This model showed that plasticity can regulate the cascade of precise spiking patterns spread throughout the CC and DCN.
2.5 Perceptron-like models
The earliest neural network that could be trained to distinguish patterns was the perceptron, described in 1958 (Rosenblatt, 1958), building on the earlier work by McCulloch and Pitts (1943). Essentially, a perceptron is a feedforward neural network with threshold units, trained weights and a binary transfer function. The perceptron linearly separates patterns on the basis of supervised learning, and it was taken as the departure point for a tremendously influential Marr-Albus-Ito (MAI) theory of the cerebellum. In short, MAI-based models are based on the premise that the PF-PC synapse undergoes LTP (Eccles et al., 1967) and/or LTD (Albus, 1971) plasticity during learning, which is guided by the CF activity. Within the perceptron framework, the MAI models assume that the modulation of the PF-PC synapse is guided by ‘supervised learning' (CF input), where the weights of each individual synapse are summated and subsequently translated into a PC response. Remarkably, the MAI-type models can also be viewed through the lens of adaptive filter theory (Dean et al., 2010).
When considering the PC as a perceptron, it becomes possible to study the capacity of the cell to encode patterns (Brunel et al., 2004; Clopath et al., 2012; Clopath and Brunel, 2013). An early analysis of the PC as a perceptron model with binary inputs and outputs demonstrated that such a PC can distinguish up to 40,000 patterns, equivalent to 50 KB of storage (Brunel et al., 2004). Furthermore, this model also suggested that in order for the PC to have such a high capacity, many PF-PC synapses would have to be nearly silent (~80% of synapses), in line with experimental data (Isope and Barbour, 2002). Later models improved the estimation of encoding capacity by relaxing the assumption about binary output, allowing firing rate modulation (Clopath et al., 2012; Clopath and Brunel, 2013). Both binary and non-binary models achieved maximum PC storage capacity with up to 80% of the PF-PC synapses being silent. The 2012 model of Clopath also quantified the capacity for pattern separation afforded by bistable firing. The CF signal not only affected supervised learning triggering the weight changes, but could also switch the PCs from up to down states. Bistability enhanced the PC storage capacity and predicted that for maximal storage capacity the bistable range must increase when correlation between input patterns decreases. Clopath and Brunel updated the perceptron model one more time in their 2013 paper to incorporate more physiologically relevant input and output patterns represented by frequency trains (analog signals) rather than binary on/off signals. Interestingly, the learning capacity of the 2013 model was highest when the input variance was the largest. This type of variance can be found experimentally in GrC firing behavior—low mean firing rate with high frequency bursts (Chadderton et al., 2004; Jörntell and Hansel, 2006; Rancz et al., 2007).
It is also possible to study PC encoding capacity in highly detailed compartmental PC models (Steuber and Schutter, 2001; Steuber et al., 2007). The Steuber models relied on the standard MAI-based LTD induction principle, where the PF input pattern was ‘trained' under the CF supervision. These studies were based on the morphologically and biophysically detailed PC model discussed previously (De Schutter and Bower, 1994a,b). Continuous excitatory background input to the DB model resulted in biologically realistic PC firing rates of 48 Hz. Novel input patterns were found to trigger higher responses than learned input patterns, and active membrane conductances in the dendrites were found to increase the discrimination between novel and learned input patterns (Steuber and Schutter, 2001). Whereas, both novel and learned stimuli triggered an initial SS burst, the pause length following the burst was predictive of the type of the stimulus. Analysis of PC responses in the 2007 Steuber model found that a hyperpolarizing Ca2+ triggered K+ efflux underlied the SS pause. Thus, LTD would encode patterns by shortening pauses, a prediction that has not yet been experimentally verified. Pattern recognition based on pause length showed that PF patterns between 750 to 8,000 active PFs allowed reliable discrimination. Patterns with PF activity levels outside of this range failed to reliably differentiate between input based on the pause length.
There are also other encoding schemes available to the PC beyond the duration of pause modulation. In 2009 Walter analyzed firing frequency as coding strategy of the PC to differentiate learned from novel input patterns (Walter and Khodakhah, 2009). The perceptron-like network model from which the coding capacity was deduced was based on the 2007 Steuber model. The Walter model simulated a PC innervated by 150,000 independent GrC synapses. A number of 650 synapses in a specific pattern was calculated to be able to trigger the maximal firing rate increase in PCs. In other words, increasing input from 0 to 650 PF's would take a PC from 50 to 250 Hz, revealing the large and linear dynamic range of the PC response. The authors convincingly argued that the firing rate frequency output gave the PC with a better coding capacity than pause duration. Moreover, asynchronous activation of synaptic input patterns over 10–25 ms windows showed a significantly better coding capacity if the PC used the firing rate coding strategy as compared to pause induction. A firing rate strategy also showed increased performance if a base level firing was introduced, which was not present in the 2007 Steuber model.
3 Discussion
Models of the PC capture much of the complex phenomenology of this cerebellar cell, though not all at the same time. Modeling is the art of selecting what and how to explain. In the context of the PC, as with many other biological systems, there are many levels at which a given phenomenon can be elucidated. Sometimes a simple model is the most effective method to understand the mechanism underlying a behavior, but in other cases the full phenomenology can be captured accurately only with added detail. There is an undeniable tension between the goals of simple vs accurate (detailed) models, but as we see in this review, their explanatory powers are often complementary. In a sense, it is this model “ensemble” (Figure 3) that describes intrinsic PC activity as well as its function in the context of a network.
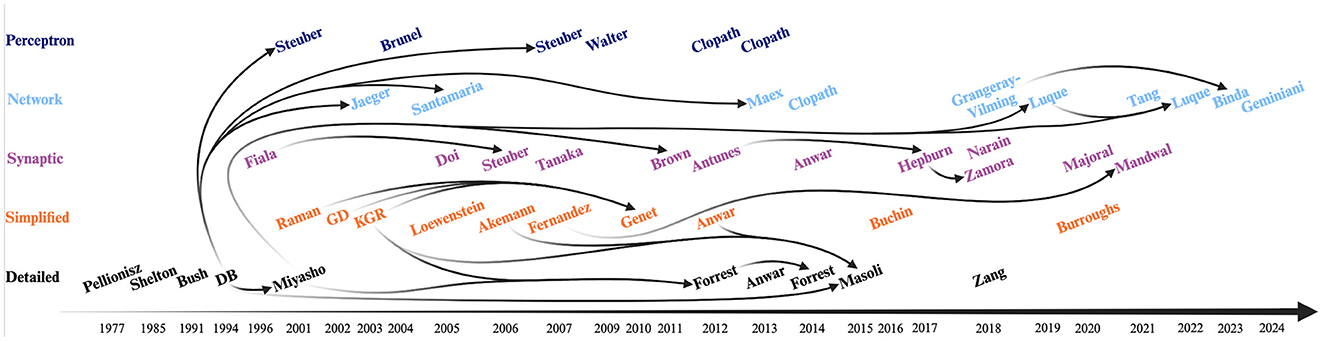
Figure 3. Timeline of selected Purkinje cell (PC) models. Each color represents a different category: detailed (black), simplified (orange), synaptic (purple), network (light blue) and perceptron-like (dark blue) models. Initial models were all detailed models. The Zang et al. (2018) model was designed as a modern update to the original 1994 De Schutter and Bower (DB) model. The DB model was the basis not only for the first network (Jaeger, 2003; Santamaria and Bower, 2005; Maex and Steuber, 2013) and perceptron-like (Steuber and Schutter, 2001; Steuber et al., 2007) models, but as well directly or indirectly for the majority of the next detailed models—except for the Anwar model. The Miyasho et al. (2001) model (based on DB model) was the basis for further synaptic (Brown et al., 2011; Luque et al., 2019, 2022) network models. The appearance of simplified models such as the Raman and Bean (2001), Genet and Delord (2002) (GD), Khaliq et al. (2003) (KGR), and Fernandez et al. (2007) models allowed for further advancement for detailed models such as the Forrest et al. (2012) and Masoli et al. (2015) models, for other simplified models such as the Genet et al. (2010) model, and for synaptic models such as the Mandwal et al. (2021) model. The Fiala et al. (1996) and Antunes and De Schutter (2012) synaptic models were used as basis for other synaptic models: the Steuber et al. (2006) and Hepburn et al. (2017) (in turn used for the Zamora Chimal and De Schutter, 2018 model), respectively. Finally, the Binda et al. (2023) network model was based on the Grangeray-Vilmint et al. (2018) model of the same type. Figure generated with BioRender.
A common criticism of biophysically plausible computational modeling is that much of the modeling work is geared toward reproducing behavior known from experiment. It is also often suggested that biophysically plausible modeling scarcely produces experimentally testable hypotheses. This criticism is sufficiently prevalent that merits discussion. What is gained by capturing complex behavior via parameters that represent specific conductances is a quantitative understanding of the spectrum of neuronal dynamics, which cannot be fully captured in one experiment. The goal of modeling is to generalize to cover a whole class of neurons, and their potential transitions across modes of activity. All models are incomplete, in the sense that not all relevant mechanisms that explain cell activity are incorporated in a single model (i.e. metabolism, transcription, regulation), and many important factors are, by necessity, excluded. Nevertheless, modeling work has also led to a profusion of experimental hypotheses. All the same, one can argue that one of the principal roles of biophysically plausible modeling is to produce mechanistic and computational understanding. Indeed, we argue that many models discussed in this review offer explanations for specific biologically observed phenomena.
In terms of the PCs computational role, modeling studies often converge on three main coding mechanisms: (1) a rather linear firing rate response to current input, (2) a complex set of intracellular Ca2+ dynamics that induce plasticity, and (3) tuneable SS pause durations. The fact that a relatively small number of active PF synapses can drive the PC response from minimum to maximum means that PCs exhibit a relatively narrow dynamical range, in the sense of signal analysis. It is still unknown whether silent synapses are a transient condition, or if they can be rapidly enlisted in short timeframes (Barbour, 1993). In any case, the small proportion of active PF synapses is still a befuddling fact of PC physiology and function.
One important point about levels of analysis is that the computational properties of the cell are not equal to its dynamics and mechanisms. Regardless of the functional roles of a PC in a network, their models may not need to capture the entire repertoire of biological detail. It is conceivable that adding specific detail may actually be detrimental to its computational role in the circuit, even if it reproduces experiment with exquisite precision. On the one hand, exploring the parameter space in which a model can no longer produce meaningful data is important to understanding its limitations. On the other hand, it is important to be aware of the bias contained in the statement that “because something exists in biology, it must play a functional role”, what Gould and Lewontin called “adaptationism” (S. J. Gould and Lewontin, 1979). Nevertheless, the value of an accurate description of a neuron cannot be understated, as it gives insight into healthy cellular dynamics and gives us confidence that the mechanisms we observe contribute to the overall electrophysiological behavior of the cell.
There are several potential caveats to the PC models presented in this review. First, most PC models are based on electrophysiological data obtained from ex-vivo slice recordings or in-vivo recordings under anesthesia. As discussed briefly in section 2.2.1 “Bistability”, anesthesia affects neuronal channel conductances, most often leading to reduced firing. The most commonly used method is isoflurane inhalation anesthesia, which at clinically relevant concentrations, leads to membrane hyperpolarization via its effects on the Na+ voltage-dependent channels (Qiu et al., 2023). Notably, isoflurane replaced an older method of urethane inhalation anesthesia, which acted on K+ voltage-dependent channels, and was shown to lead to cycling between up and down states (Yagishita et al., 2020). The second most commonly used sedation method is a ketamine/xylazine cocktail injected intraperitoneally, which is a mixture on an NMDA receptor antagonist (ketamine) and an α2-adrenergic receptor agonist (xylazine). On the one hand, all these methods affect neuronal electrophysiological properties, which in turn might lead to improper inputs to the PC models. On the other hand the very same models could be used to study different classes of the anesthetics and their effects on cerebellar function. Nevertheless, anesthesia is a factor that can introduce many unforeseen variables. Therefore newer models, particularly the ones that describe network activity during learning, are largely based on data from in-vivo awake recordings from behaving animals. Second, our review focuses primarily on models of the PC itself, and thereby excludes most of the circuit resonances feeding back into the CC such as: the Golgi cell oscillations, PC to PC inhibition, DCN feedback to GrCs, the IO dynamics and the feedforward inhibition of MLIs. Particularly, the significant impact of the MLI inputs on PC activity and cerebellar learning has been extensively researched in the last decade (Badura et al., 2013; Brown et al., 2019; Ma et al., 2020), but has not yet been investigated in depth in the modeling work, with the notable exception of network models. Overall, complex network dynamics make the interpretation of the experimental data, restricted to PC recordings, difficult. However, a good grasp of the mechanisms underlying activity of the PC in isolation, is instrumental to disentangle complex cerebellar network responses. Third, even when models include large numbers of conductances and other dynamics, publications often focus on a few mechanisms at a time. This is partly due to the difficulty of visualizing high dimensional parameter spaces, and partly due to the fact that adding new mechanisms exponentially expands parameter space, which is computationally costly. This is one of the reasons why there is no ‘final model' of the PC, which includes ‘everything'. Computational scientists have a preference to study only the mechanisms that are required for a given behavior of interest. Finally, with the rise of the new era of big omics, cerebellar sequencing data has revealed that there might in fact be several categories of PCs with different characteristics and properties (Kozareva et al., 2021; Apsley and Becker, 2022). However, at present these new categories are not yet linked to excitability differences, or other functional or encoding correlates. One fundamental open question for computational modeling of the PC is the extent to which excitability differences found in distinct Zebrin zones can be linked to specific model parameters. It seems promising that highly constrained biophysical models can help us express the different categories of cells as they relate to parameter ranges. We foresee a future in which complex neuron models will be able to help us link results from the genomics and proteomics studies with neuronal dynamics.
As computational neuroscience moves forward, the key issue for upcoming research is the development of accurate models that give us insight into necessary homeostatic mechanisms of complex neurons such as the PC. Tuning accurate models reproducing specific behaviors at the level of cells and organisms, with plausible models of cellular dynamics, gives researchers a handle on how to interpret neuronal activity in health and disease. Future models must tackle the question of how to extrapolate from single detailed models to entire populations close to the biological constraints, particularly across different brain regions. As neurons need to “change to stay the same” (Abadia et al., 2021), the inclusion of homeostatic mechanisms in the current models is a promising research direction, so that we better understand how a cell changes within functional constraints.
Finally, the question should be addressed of whether abstract PC models are sufficient to represent motor learning. Though robotics applications have been using the Perceptron analogy for a long time (Vijayan et al., 2017), there is no “full spectrum” theory of motor learning on the basis of PCs as Perceptrons. It seems that the ways in which PCs are used in biological brains will still be providing ample insight to develop computational models and potential future AI applications to represent biological learning.
Author contributions
EF: Writing – original draft, Writing – review & editing. AK: Writing – original draft, Writing – review & editing. PW: Writing – original draft, Writing – review & editing. CD: Funding acquisition, Writing – original draft, Writing – review & editing. AB: Funding acquisition, Project administration, Resources, Supervision, Writing – original draft, Writing – review & editing. MN: Conceptualization, Funding acquisition, Investigation, Methodology, Project administration, Resources, Supervision, Writing – original draft, Writing – review & editing.
Funding
The author(s) declare financial support was received for the research, authorship, and/or publication of this article. This work was supported by the Netherlands Organization for Scientific Research (NWO) VIDI/917.18.380,2018/ZonMw (AB), NWO NWA-ORC 2022 SCANNER (AB), the Erasmus MC Convergence Health and Technology Integrative Neuromedicine Flagship Program (AB and MN), the 2021 H2020 ArborIO project (no. 650003) (MN). Financial support to CD was provided by the Netherlands Organization for Scientific Research (NWO-ALW 824.02.001), the Dutch Organization for Medical Sciences (ZonMW 91120067), Medical Neuro-Delta (MD 01092019-31082023), INTENSE LSH-NWO (TTW/00798883), ERC-adv (GA-294775), and ERC-POC (nrs. 737619 and 768914) and The NIN Vriendenfonds for Albinism as well as the Dutch NWO Gravitation Program (DBI2).
Acknowledgments
The authors would like to thank Erik De Schutter, Egidio D'Angelo, Sungho Hong, and Michael Häusser for insights on the encoding properties and physiological responses of the Purkinje cell.
Conflict of interest
The authors declare that the research was conducted in the absence of any commercial or financial relationships that could be construed as a potential conflict of interest.
The author(s) declared that they were an editorial board member of Frontiers, at the time of submission. This had no impact on the peer review process and the final decision.
Publisher's note
All claims expressed in this article are solely those of the authors and do not necessarily represent those of their affiliated organizations, or those of the publisher, the editors and the reviewers. Any product that may be evaluated in this article, or claim that may be made by its manufacturer, is not guaranteed or endorsed by the publisher.
References
Abadia, I., Naveros, F., Garrido, J. A., Ros, E., and Luque, N. R. (2021). On robot compliance: a cerebellar control approach. IEEE Trans. Cybern. 51, 2476–2489. doi: 10.1109/TCYB.2019.2945498
Akemann, W., and Knöpfel, T. (2006). Interaction of Kv3 potassium channels and resurgent sodium current influences the rate of spontaneous firing of Purkinje neurons. J. Neurosci. 26, 4602–4612. doi: 10.1523/JNEUROSCI.5204-05.2006
Albus, J. S. (1971). A theory of cerebellar function. Math. Biosci. 10, 25–61. doi: 10.1016/0025-5564(71)90051-4
Aldrich, R. W., Corey, D. P., and Stevens, C. F. (1983). A reinterpretation of mammalian sodium channel gating based on single channel recording. Nature 306, 436–441. doi: 10.1038/306436a0
Angelo, K., London, M., Christensen, S. R., and Häusser, M. (2007). Local and global effects of I(h) distribution in dendrites of mammalian neurons. J. Neurosci. 27, 8643–8653. doi: 10.1523/JNEUROSCI.5284-06.2007
Antunes, G., and De Schutter, E. (2012). A stochastic signaling network mediates the probabilistic induction of cerebellar long-term depression. J. Neurosci. 32, 9288–9300. doi: 10.1523/JNEUROSCI.5976-11.2012
Anwar, H., Hepburn, I., Nedelescu, H., Chen, W., and De Schutter, E. (2013). Stochastic calcium mechanisms cause dendritic calcium spike variability. J. Neurosci. 33, 15848–15867. doi: 10.1523/JNEUROSCI.1722-13.2013
Anwar, H., Hong, S., and De Schutter, E. (2012). Controlling Ca2+-activated K+ channels with models of Ca2+ buffering in Purkinje cells. Cerebellum 11, 681–693. doi: 10.1007/s12311-010-0224-3
Anwar, H., Roome, C. J., Nedelescu, H., Chen, W., Kuhn, B., and De Schutter, E. (2014). Dendritic diameters affect the spatial variability of intracellular calcium dynamics in computer models. Front. Cell. Neurosci. 8:168. doi: 10.3389/fncel.2014.00168
Apsley, E. J., and Becker, E. B. E. (2022). Purkinje cell patterning-insights from single-cell sequencing. Cells 11:2918. doi: 10.3390/cells11182918
Arlt, C., and Häusser, M. (2020). Microcircuit rules governing impact of single interneurons on purkinje cell output in vivo. Cell Rep. 30, 3020–3035.e3. doi: 10.1016/j.celrep.2020.02.009
Badura, A., Clopath, C., Schonewille, M., and De Zeeuw, C. I. (2016). Modeled changes of cerebellar activity in mutant mice are predictive of their learning impairments. Sci. Rep. 6, 36131. doi: 10.1038/srep36131
Badura, A., Schonewille, M., Voges, K., Galliano, E., Renier, N., Gao, Z., et al. (2013). Climbing fiber input shapes reciprocity of Purkinje cell firing. Neuron 78, 700–713. doi: 10.1016/j.neuron.2013.03.018
Bao, J., Reim, K., and Sakaba, T. (2010). Target-dependent feedforward inhibition mediated by short-term synaptic plasticity in the cerebellum. J. Neurosci. 30, 8171–8179. doi: 10.1523/JNEUROSCI.0276-10.2010
Barbour, B. (1993). Synaptic currents evoked in Purkinje cells by stimulating individual granule cells. Neuron 11, 759–769. doi: 10.1016/0896-6273(93)90085-6
Benton, M. D., Lewis, A. H., Bant, J. S., and Raman, I. M. (2013). Iberiotoxin-sensitive and -insensitive BK currents in Purkinje neuron somata. J. Neurophysiol. 109, 2528–2541. doi: 10.1152/jn.00127.2012
Benton, M. D., and Raman, I. M. (2009). Stabilization of Ca current in Purkinje neurons during high-frequency firing by a balance of Ca-dependent facilitation and inactivation. Channels 3, 393–401. doi: 10.4161/chan.3.6.9838
Bezprozvanny, I., Watras, J., and Ehrlich, B. E. (1991). Bell-shaped calcium-response curves of Ins(1,4,5)P3- and calcium-gated channels from endoplasmic reticulum of cerebellum. Nature 351, 751–754. doi: 10.1038/351751a0
Bhalla, U. S., and Iyengar, R. (1999). Emergent properties of networks of biological signaling pathways. Science 283, 381–387. doi: 10.1126/science.283.5400.381
Bichet, D., Haass, F. A., and Jan, L. Y. (2003). Merging functional studies with structures of inward-rectifier K(+) channels. Nat. Rev. Neurosci. 4, 957–967. doi: 10.1038/nrn1244
Binda, F., Spaeth, L., Kumar, A., and Isope, P. (2023). Excitation and inhibition delays within a feedforward inhibitory pathway modulate cerebellar Purkinje cell output in mice. J. Neurosci. 43, 5905–5917. doi: 10.1523/JNEUROSCI.0091-23.2023
Blackstone, C. D., Supattapone, S., and Snyder, S. H. (1989). Inositolphospholipid-linked glutamate receptors mediate cerebellar parallel-fiber-Purkinje-cell synaptic transmission. Proc. Natl. Acad. Sci. U. S. A. 86, 4316–4320. doi: 10.1073/pnas.86.11.4316
Boele, H.-J., Peter, S., Ten Brinke, M. M., Verdonschot, L., IJpelaar, A. C. H., Rizopoulos, D., et al. (2018). Impact of parallel fiber to Purkinje cell long-term depression is unmasked in absence of inhibitory input. Sci Adv 4:eaas9426. doi: 10.1126/sciadv.aas9426
Brown, A. M., Arancillo, M., Lin, T., Catt, D. R., Zhou, J., Lackey, E. P., et al. (2019). Molecular layer interneurons shape the spike activity of cerebellar Purkinje cells. Sci. Rep. 9:1742. doi: 10.1038/s41598-018-38264-1
Brown, S.-A., Moraru, I. I., Schaff, J. C., and Loew, L. M. (2011). Virtual NEURON: a strategy for merged biochemical and electrophysiological modeling. J. Comput. Neurosci. 31, 385–400. doi: 10.1007/s10827-011-0317-0
Brown, S.-A., Morgan, F., Watras, J., and Loew, L. M. (2008). Analysis of phosphatidylinositol-4,5-bisphosphate signaling in cerebellar Purkinje spines. Biophys. J. 95, 1795–1812. doi: 10.1529/biophysj.108.130195
Brunel, N., Hakim, V., Isope, P., Nadal, J.-P., and Barbour, B. (2004). Optimal information storage and the distribution of synaptic weights: perceptron versus Purkinje cell. Neuron 43, 745–757. doi: 10.1016/S0896-6273(04)00528-8
Buchin, A., Rieubland, S., Häusser, M., Gutkin, B. S., and Roth, A. (2016). Inverse stochastic resonance in cerebellar purkinje cells. PLoS Comput. Biol. 12:e1005000. doi: 10.1371/journal.pcbi.1005000
Burroughs, A., Cerminara, N. L., Apps, R., and Houghton, C. (2020). A Purkinje cell model that simulates complex spikes. bioRxiv [preprint]bioRxiv 2020.05.18.102236. doi: 10.1101/2020.05.18.102236
Busch, S. E., and Hansel, C. (2023). Climbing fiber multi-innervation of mouse Purkinje dendrites with arborization common to human. Science 381, 420–427. doi: 10.1126/science.adi1024
Bush, P. C., and Sejnowski, T. J. (1991). Simulations of a reconstructed cerebellar purkinje cell based on simplified channel kinetics. Neural Comput. 3, 321–332. doi: 10.1162/neco.1991.3.3.321
Bushell, T., Clarke, C., Mathie, A., and Robertson, B. (2002). Pharmacological characterization of a non-inactivating outward current observed in mouse cerebellar Purkinje neurones. Br. J. Pharmacol. 135, 705–712. doi: 10.1038/sj.bjp.0704518
Cao, Y., Liu, Y., Jaeger, D., and Heck, D. H. (2017). Cerebellar Purkinje cells generate highly correlated spontaneous slow-rate fluctuations. Front. Neural Circuits 11:67. doi: 10.3389/fncir.2017.00067
Cerminara, N. L., and Rawson, J. A. (2004). Evidence that climbing fibers control an intrinsic spike generator in cerebellar Purkinje cells. J. Neurosci. 24, 4510–4517. doi: 10.1523/JNEUROSCI.4530-03.2004
Chadderton, P., Margrie, T. W., and Häusser, M. (2004). Integration of quanta in cerebellar granule cells during sensory processing. Nature 428, 856–860. doi: 10.1038/nature02442
Clopath, C., Badura, A., De Zeeuw, C. I., and Brunel, N. (2014). A cerebellar learning model of vestibulo-ocular reflex adaptation in wild-type and mutant mice. J. Neurosci. 34, 7203–7215. doi: 10.1523/JNEUROSCI.2791-13.2014
Clopath, C., and Brunel, N. (2013). Optimal properties of analog perceptrons with excitatory weights. PLoS Comput. Biol. 9:e1002919. doi: 10.1371/journal.pcbi.1002919
Clopath, C., Nadal, J.-P., and Brunel, N. (2012). Storage of correlated patterns in standard and bistable Purkinje cell models. PLoS Comput. Biol. 8:e1002448. doi: 10.1371/journal.pcbi.1002448
Connor, J. A., and Stevens, C. F. (1971). Voltage clamp studies of a transient outward membrane current in gastropod neural somata. J. Physiol. 213, 21–30. doi: 10.1113/jphysiol.1971.sp009365
Cook, A. A., Fields, E., and Watt, A. J. (2021). Losing the beat: contribution of purkinje cell firing dysfunction to disease, and its reversal. Neuroscience 462, 247–261. doi: 10.1016/j.neuroscience.2020.06.008
Courtemanche, M., Ramirez, R. J., and Nattel, S. (1998). Ionic mechanisms underlying human atrial action potential properties: insights from a mathematical model. Am. J. Physiol. 275, H301–H321. doi: 10.1152/ajpheart.1998.275.1.H301
Cox, D. H., Cui, J., and Aldrich, R. W. (1997). Allosteric gating of a large conductance Ca-activated K+ channel. J. Gen. Physiol. 110, 257–281. doi: 10.1085/jgp.110.3.257
Davie, J. T., Clark, B. A., and Häusser, M. (2008). The origin of the complex spike in cerebellar Purkinje cells. J. Neurosci. 28, 7599–7609. doi: 10.1523/JNEUROSCI.0559-08.2008
De Schutter, E. (1986). Alternative equations for the molluscan ion currents described by Connor and Stevens. Brain Res. 382, 134–138. doi: 10.1016/0006-8993(86)90120-4
De Schutter, E., and Bower, J. M. (1994a). An active membrane model of the cerebellar Purkinje cell II. Simulation of synaptic responses. J. Neurophysiol. 71, 401–419. doi: 10.1152/jn.1994.71.1.401
De Schutter, E., and Bower, J. M. (1994b). An active membrane model of the cerebellar Purkinje cell. I. Simulation of current clamps in slice. J. Neurophysiol. 71, 375–400. doi: 10.1152/jn.1994.71.1.375
de Solages, C., Szapiro, G., Brunel, N., Hakim, V., Isope, P., Buisseret, P., et al. (2008). High-frequency organization and synchrony of activity in the purkinje cell layer of the cerebellum. Neuron 58, 775–788. doi: 10.1016/j.neuron.2008.05.008
De Zeeuw, C. I. (2021). Bidirectional learning in upbound and downbound microzones of the cerebellum. Nat. Rev. Neurosci. 22, 92–110. doi: 10.1038/s41583-020-00392-x
Dean, P., Porrill, J., Ekerot, C.-F., and Jörntell, H. (2010). The cerebellar microcircuit as an adaptive filter: experimental and computational evidence. Nat. Rev. Neurosci. 11, 30–43. doi: 10.1038/nrn2756
Diwakar, S., Magistretti, J., Goldfarb, M., Naldi, G., and D'Angelo, E. (2009). Axonal Na+ channels ensure fast spike activation and back-propagation in cerebellar granule cells. J. Neurophysiol. 101, 519–532. doi: 10.1152/jn.90382.2008
Doi, T., Kuroda, S., Michikawa, T., and Kawato, M. (2005). Inositol 1,4,5-trisphosphate-dependent Ca2+ threshold dynamics detect spike timing in cerebellar Purkinje cells. J. Neurosci. 25, 950–961. doi: 10.1523/JNEUROSCI.2727-04.2005
Eccles, J. C., Ito, M., and Szentágothai, J. (1967). The Cerebellum as a Neuronal Machine. Cham: Springer Berlin Heidelberg.
Engbers, J. D. T., Fernandez, F. R., and Turner, R. W. (2013). Bistability in Purkinje neurons: ups and downs in cerebellar research. Neural Netw. 47, 18–31. doi: 10.1016/j.neunet.2012.09.006
Etzion, Y., and Grossman, Y. (1998). Potassium currents modulation of calcium spike firing in dendrites of cerebellar Purkinje cells. Exp. Brain Res. 122, 283–294. doi: 10.1007/s002210050516
Etzion, Y., and Grossman, Y. (2001). Highly 4-aminopyridine sensitive delayed rectifier current modulates the excitability of guinea pig cerebellar Purkinje cells. Exp. Brain Res. 139, 419–425. doi: 10.1007/s002210100788
Fernandez, F. R., Engbers, J. D. T., and Turner, R. W. (2007). Firing dynamics of cerebellar purkinje cells. J. Neurophysiol. 98, 278–294. doi: 10.1152/jn.00306.2007
Fiala, J. C., Grossberg, S., and Bullock, D. (1996). Metabotropic glutamate receptor activation in cerebellar Purkinje cells as substrate for adaptive timing of the classically conditioned eye-blink response. J. Neurosci. 16, 3760–3774. doi: 10.1523/JNEUROSCI.16-11-03760.1996
Forrest, M. D. (2014). Intracellular calcium dynamics permit a Purkinje neuron model to perform toggle and gain computations upon its inputs. Front. Comput. Neurosci. 8:86. doi: 10.3389/fncom.2014.00086
Forrest M. D. Wall M. J. Press D. A. Feng J. (2012). The sodium-potassium pump controls the intrinsic firing of the cerebellar Purkinje neuron. PLoS ONE 7:e51169. doi: 10.1371/journal.pone.0051169
Fourcaud-Trocmé, N., Hansel, D., van Vreeswijk, C., and Brunel, N. (2003). How spike generation mechanisms determine the neuronal response to fluctuating inputs. J. Neurosci. 23, 11628–11640. doi: 10.1523/JNEUROSCI.23-37-11628.2003
Fujiwara, A., Hirose, K., Yamazawa, T., and Iino, M. (2001). Reduced IP3 sensitivity of IP3 receptor in Purkinje neurons. Neuroreport 12, 2647–2651. doi: 10.1097/00001756-200108280-00012
Gähwiler, B. H., and Llano, I. (1989). Sodium and potassium conductances in somatic membranes of rat Purkinje cells from organotypic cerebellar cultures. J. Physiol. 417, 105–122. doi: 10.1113/jphysiol.1989.sp017793
Gao, Z., van Beugen, B. J., and De Zeeuw, C. I. (2012). Distributed synergistic plasticity and cerebellar learning. Nat. Rev. Neurosci. 13, 619–635. doi: 10.1038/nrn3312
Geminiani, A., Casellato, C., Boele, H.-J., Pedrocchi, A., De Zeeuw, C. I., and D'Angelo, E. (2024). Mesoscale simulations predict the role of synergistic cerebellar plasticity during classical eyeblink conditioning. PLoS Comput. Biol. 20:e1011277. doi: 10.1371/journal.pcbi.1011277
Genet, S., and Delord, B. (2002). A biophysical model of nonlinear dynamics underlying plateau potentials and calcium spikes in purkinje cell dendrites. J. Neurophysiol. 88, 2430–2444. doi: 10.1152/jn.00839.2001
Genet, S., Sabarly, L., Guigon, E., Berry, H., and Delord, B. (2010). Dendritic signals command firing dynamics in a mathematical model of cerebellar Purkinje cells. Biophys. J. 99, 427–436. doi: 10.1016/j.bpj.2010.04.056
Gilbert, P. F., and Thach, W. T. (1977). Purkinje cell activity during motor learning. Brain Res. 128, 309–328. doi: 10.1016/0006-8993(77)90997-0
Gillies, A., and Willshaw, D. (2006). Membrane channel interactions underlying rat subthalamic projection neuron rhythmic and bursting activity. J. Neurophysiol. 95, 2352–2365. doi: 10.1152/jn.00525.2005
Giovannucci, A., Badura, A., Deverett, B., Najafi, F., Pereira, T. D., Gao, Z., et al. (2017). Cerebellar granule cells acquire a widespread predictive feedback signal during motor learning. Nat. Neurosci. 20, 727–734. doi: 10.1038/nn.4531
Glantz, R. M., and Viancour, T. (1983). Integrative properties of crayfish medial giant neuron: steady-state model. J. Neurophysiol. 50, 1122–1142. doi: 10.1152/jn.1983.50.5.1122
Gould, S. J., and Lewontin, R. C. (1979). The spandrels of San Marco and the Panglossian paradigm: a critique of the adaptationist programme. Proc. Royal Soc. London. Series B. Biol. Sci. 205, 581–598. doi: 10.1098/rspb.1979.0086
Grangeray-Vilmint, A., Valera, A. M., Kumar, A., and Isope, P. (2018). Short-term plasticity combines with excitation-inhibition balance to expand cerebellar purkinje cell dynamic range. J. Neurosci. 38, 5153–5167. doi: 10.1523/JNEUROSCI.3270-17.2018
Gruol, D. L., Jacquin, T., and Yool, A. J. (1991). Single-channel K+ currents recorded from the somatic and dendritic regions of cerebellar Purkinje neurons in culture. J. Neurosci. 11, 1002–1015. doi: 10.1523/JNEUROSCI.11-04-01002.1991
Guo, C., Witter, L., Rudolph, S., Elliott, H. L., Ennis, K. A., and Regehr, W. G. (2016). Purkinje cells directly inhibit granule cells in specialized regions of the cerebellar cortex. Neuron 91, 1330–1341. doi: 10.1016/j.neuron.2016.08.011
Gutierrez-Castellanos, N., Da Silva-Matos, C. M., Zhou, K., Canto, C. B., Renner, M. C., Koene, L. M. C., et al. (2017). Motor learning requires purkinje cell synaptic potentiation through activation of AMPA-receptor subunit GluA3. Neuron 93, 409–424. doi: 10.1016/j.neuron.2016.11.046
Hansel, C., de Jeu, M., Belmeguenai, A., Houtman, S. H., Buitendijk, G. H. S., Andreev, D., et al. (2006). alphaCaMKII Is essential for cerebellar LTD and motor learning. Neuron 51, 835–843. doi: 10.1016/j.neuron.2006.08.013
Hardy, W. L. (1973). Propagation speed in myelinated nerve. II theoretical dependence on external Na and on temperature. Biophys. J. 13, 1071–1089. doi: 10.1016/S0006-3495(73)86046-1
Heck, D. H., Thach, W. T., and Keating, J. G. (2007). On-beam synchrony in the cerebellum as the mechanism for the timing and coordination of movement. Proc. Natl. Acad. Sci. U. S. A. 104, 7658–7663. doi: 10.1073/pnas.0609966104
Hepburn, I., Jain, A., Gangal, H., Yamamoto, Y., Tanaka-Yamamoto, K., and De Schutter, E. (2017). A model of induction of cerebellar long-term depression including RKIP inactivation of Raf and MEK. Front. Mol. Neurosci. 10, 19. doi: 10.3389/fnmol.2017.00019
Herzfeld, D. J., Kojima, Y., Soetedjo, R., and Shadmehr, R. (2015). Encoding of action by the Purkinje cells of the cerebellum. Nature 526, 439–442. doi: 10.1038/nature15693
Hirano, T., and Hagiwara, S. (1989). Kinetics and distribution of voltage-gated Ca, Na and K channels on the somata of rat cerebellar Purkinje cells. Pflugers Arch. 413, 463–469. doi: 10.1007/BF00594174
Hirschberg, B., Maylie, J., Adelman, J. P., and Marrion, N. V. (1998). Gating of recombinant small-conductance Ca-activated K+ channels by calcium. J. Gen. Physiol. 111, 565–581. doi: 10.1085/jgp.111.4.565
Hodgkin, A. L., and Huxley, A. F. (1952). A quantitative description of membrane current and its application to conduction and excitation in nerve. J. Physiol. 117, 500–544. doi: 10.1113/jphysiol.1952.sp004764
Hounsgaard, J., and Midtgaard, J. (1988). Intrinsic determinants of firing pattern in Purkinje cells of the turtle cerebellum in vitro. J. Physiol. 402, 731–749. doi: 10.1113/jphysiol.1988.sp017231
Huguenard, J. R., and McCormick, D. A. (1992). Simulation of the currents involved in rhythmic oscillations in thalamic relay neurons. J. Neurophysiol. 68, 1373–1383. doi: 10.1152/jn.1992.68.4.1373
Iftinca, M., McKay, B. E., Snutch, T. P., McRory, J. E., Turner, R. W., and Zamponi, G. W. (2006). Temperature dependence of T-type calcium channel gating. Neuroscience 142, 1031–1042. doi: 10.1016/j.neuroscience.2006.07.010
Indriati, D. W., Kamasawa, N., Matsui, K., Meredith, A. L., Watanabe, M., and Shigemoto, R. (2013). Quantitative localization of Cav2.1 (P/Q-type) voltage-dependent calcium channels in Purkinje cells: somatodendritic gradient and distinct somatic coclustering with calcium-activated potassium channels. J. Neurosci. 33, 3668–3678. doi: 10.1523/JNEUROSCI.2921-12.2013
Isope, P., and Barbour, B. (2002). Properties of unitary granule cell–>Purkinje cell synapses in adult rat cerebellar slices. J. Neurosci. 22, 9668–9678. doi: 10.1523/JNEUROSCI.22-22-09668.2002
Jacquin, T. D., and Gruol, D. L. (1999). Ca2+ regulation of a large conductance K+ channel in cultured rat cerebellar Purkinje neurons. Eur. J. Neurosci. 11, 735–739. doi: 10.1046/j.1460-9568.1999.00478.x
Jaeger, D. (2003). No parallel fiber volleys in the cerebellar cortex: evidence from cross-correlation analysis between Purkinje cells in a computer model and in recordings from anesthetized rats. J. Comput. Neurosci. 14, 311–327. doi: 10.1023/A:1023217111784
Jaeger, D., and Bower, J. M. (1999). Synaptic control of spiking in cerebellar Purkinje cells: dynamic current clamp based on model conductances. J. Neurosci. 19, 6090–6101. doi: 10.1523/JNEUROSCI.19-14-06090.1999
Jelitai, M., Puggioni, P., Ishikawa, T., Rinaldi, A., and Duguid, I. (2016). Dendritic excitation-inhibition balance shapes cerebellar output during motor behaviour. Nat. Commun. 7:13722. doi: 10.1038/ncomms13722
Johansson, F., Hesslow, G., and Medina, J. F. (2016). Mechanisms for motor timing in the cerebellar cortex. Curr Opin Behav Sci 8, 53–59. doi: 10.1016/j.cobeha.2016.01.013
Johansson, F., Jirenhed, D.-A., Rasmussen, A., Zucca, R., and Hesslow, G. (2018). Absence of parallel fibre to Purkinje cell LTD during eyeblink conditioning. Sci. Rep. 8:14777. doi: 10.1038/s41598-018-32791-7
Jörntell, H., and Hansel, C. (2006). Synaptic memories upside down: bidirectional plasticity at cerebellar parallel fiber-Purkinje cell synapses. Neuron 52, 227–238. doi: 10.1016/j.neuron.2006.09.032
Kaneda, M., Wakamori, M., Ito, C., and Akaike, N. (1990). Low-threshold calcium current in isolated Purkinje cell bodies of rat cerebellum. J. Neurophysiol. 63, 1046–1051. doi: 10.1152/jn.1990.63.5.1046
Khaliq, Z. M., Gouwens, N. W., and Raman, I. M. (2003). The contribution of resurgent sodium current to high-frequency firing in Purkinje neurons: an experimental and modeling study. J. Neurosci. 23, 4899–4912. doi: 10.1523/JNEUROSCI.23-12-04899.2003
Khaliq, Z. M., and Raman, I. M. (2006). Relative contributions of axonal and somatic Na channels to action potential initiation in cerebellar Purkinje neurons. J. Neurosci. 26, 1935–1944. doi: 10.1523/JNEUROSCI.4664-05.2006
Khodakhah, K., and Ogden, D. (1993). Functional heterogeneity of calcium release by inositol trisphosphate in single Purkinje neurones, cultured cerebellar astrocytes, and peripheral tissues. Proc. Natl. Acad. Sci. U. S. A. 90, 4976–4980. doi: 10.1073/pnas.90.11.4976
Khodakhah, K., and Ogden, D. (1995). Fast activation and inactivation of inositol trisphosphate-evoked Ca2+ release in rat cerebellar Purkinje neurones. J. Physiol. 487, 343–358. doi: 10.1113/jphysiol.1995.sp020884
Kim, C.-H., Oh, S.-H., Lee, J. H., Chang, S. O., Kim, J., and Kim, S. J. (2012). Lobule-specific membrane excitability of cerebellar Purkinje cells. J. Physiol. 590, 273–288. doi: 10.1113/jphysiol.2011.221846
Kim, J., and Augustine, G. J. (2021). Molecular layer interneurons: key elements of cerebellar network computation and behavior. Neuroscience 462, 22–35. doi: 10.1016/j.neuroscience.2020.10.008
Komendantov, A. O., Komendantova, O. G., Johnson, S. W., and Canavier, C. C. (2004). A modeling study suggests complementary roles for GABAA and NMDA receptors and the SK channel in regulating the firing pattern in midbrain dopamine neurons. J. Neurophysiol. 91, 346–357. doi: 10.1152/jn.00062.2003
Kozareva, V., Martin, C., Osorno, T., Rudolph, S., Guo, C., Vanderburg, C., et al. (2021). A transcriptomic atlas of mouse cerebellar cortex comprehensively defines cell types. Nature 598, 214–219. doi: 10.1038/s41586-021-03220-z
Kubota, Y., and Bower, J. M. (2001). Transient versus asymptotic dynamics of CaM kinase II: possible roles of phosphatase. J. Comput. Neurosci. 11, 263–279. doi: 10.1023/A:1013727331979
Kuroda, S., Schweighofer, N., and Kawato, M. (2001). Exploration of signal transduction pathways in cerebellar long-term depression by kinetic simulation. J. Neurosci. 21, 5693–5702. doi: 10.1523/JNEUROSCI.21-15-05693.2001
Kyriakis, J. M. (2007). The integration of signaling by multiprotein complexes containing Raf kinases. Biochim. Biophys. Acta 1773, 1238–1247. doi: 10.1016/j.bbamcr.2006.11.003
Lanore, F., Cayco-Gajic, N. A., Gurnani, H., Coyle, D., and Silver, R. A. (2021). Cerebellar granule cell axons support high-dimensional representations. Nat. Neurosci. 24, 1142–1150. doi: 10.1038/s41593-021-00873-x
Larkum, M. E., Nevian, T., Sandler, M., Polsky, A., and Schiller, J. (2009). Synaptic integration in tuft dendrites of layer 5 pyramidal neurons: a new unifying principle. Science 325, 756–760. doi: 10.1126/science.1171958
Lev-Tov, A., Miller, J. P., Burke, R. E., and Rall, W. (1983). Factors that control amplitude of EPSPs in dendritic neurons. J. Neurophysiol. 50, 399–412. doi: 10.1152/jn.1983.50.2.399
Llinas, R., Bloedel, J. R., and Hillman, D. E. (1969). Functional characterization of neuronal circuitry of frog cerebellar cortex. J. Neurophysiol. 32, 847–870. doi: 10.1152/jn.1969.32.6.847
Llinás, R., and Sugimori, M. (1980a). Electrophysiological properties of in vitro Purkinje cell dendrites in mammalian cerebellar slices. J. Physiol. 305, 197–213. doi: 10.1113/jphysiol.1980.sp013358
Llinás, R., and Sugimori, M. (1980b). Electrophysiological properties of in vitro Purkinje cell somata in mammalian cerebellar slices. J. Physiol. 305, 171–195. doi: 10.1113/jphysiol.1980.sp013357
Loewenstein, Y., Mahon, S., Chadderton, P., Kitamura, K., Sompolinsky, H., Yarom, Y., et al. (2005). Bistability of cerebellar Purkinje cells modulated by sensory stimulation. Nat. Neurosci. 8, 202–211. doi: 10.1038/nn1393
Luque, N. R., Naveros, F., Carrillo, R. R., Ros, E., and Arleo, A. (2019). Spike burst-pause dynamics of Purkinje cells regulate sensorimotor adaptation. PLoS Comput. Biol. 15:e1006298. doi: 10.1371/journal.pcbi.1006298
Luque, N. R., Naveros, F., Sheynikhovich, D., Ros, E., and Arleo, A. (2022). Computational epidemiology study of homeostatic compensation during sensorimotor aging. Neural Netw. 146, 316–333. doi: 10.1016/j.neunet.2021.11.024
Ma, M., Futia, G. L., de Souza, F. M. S., Ozbay, B. N., Llano, I., Gibson, E. A., et al. (2020). Molecular layer interneurons in the cerebellum encode for valence in associative learning. Nat. Commun. 11:4217. doi: 10.1038/s41467-020-18034-2
Maeda, H., Ellis-Davies, G. C., Ito, K., Miyashita, Y., and Kasai, H. (1999). Supralinear Ca2+ signaling by cooperative and mobile Ca2+ buffering in Purkinje neurons. Neuron 24, 989–1002. doi: 10.1016/S0896-6273(00)81045-4
Maex, R., and De Schutter, E. (2007). Mechanism of spontaneous and self-sustained oscillations in networks connected through axo-axonal gap junctions. Eur. J. Neurosci. 25, 3347–3358. doi: 10.1111/j.1460-9568.2007.05593.x
Maex, R., and Steuber, V. (2013). An integrator circuit in cerebellar cortex. Eur. J. Neurosci. 38, 2917–2932. doi: 10.1111/ejn.12272
Majoral, D., Zemmar, A., and Vicente, R. (2020). A model for time interval learning in the Purkinje cell. PLoS Comput. Biol. 16:e1007601. doi: 10.1371/journal.pcbi.1007601
Mandwal, A., Orlandi, J. G., Simon, C., and Davidsen, J. (2021). A biochemical mechanism for time-encoding memory formation within individual synapses of Purkinje cells. PLoS ONE 16:e0251172. doi: 10.1371/journal.pone.0251172
Marchant, J. S., and Taylor, C. W. (1997). Cooperative activation of IP3 receptors by sequential binding of IP3 and Ca2+ safeguards against spontaneous activity. Curr. Biol. 7, 510–518. doi: 10.1016/S0960-9822(06)00222-3
Martina, M., Metz, A. E., and Bean, B. P. (2007). Voltage-dependent potassium currents during fast spikes of rat cerebellar Purkinje neurons: inhibition by BDS-I toxin. J. Neurophysiol. 97, 563–571. doi: 10.1152/jn.00269.2006
Masoli, S., Solinas, S., and D'Angelo, E. (2015). Action potential processing in a detailed Purkinje cell model reveals a critical role for axonal compartmentalization. Front. Cell. Neurosci. 9:47. doi: 10.3389/fncel.2015.00047
McCulloch, W. S., and Pitts, W. (1943). A logical calculus of the ideas immanent in nervous activity. Bull. Math. Biophys. 5, 115–133. doi: 10.1007/BF02478259
McKay, B. E., and Turner, R. W. (2004). Kv3 K+ channels enable burst output in rat cerebellar Purkinje cells. Eur. J. Neurosci. 20, 729–739. doi: 10.1111/j.1460-9568.2004.03539.x
Middleton, S. J., Racca, C., Cunningham, M. O., Traub, R. D., Monyer, H., Knöpfel, T., et al. (2008). High-frequency network oscillations in cerebellar cortex. Neuron 58, 763–774. doi: 10.1016/j.neuron.2008.03.030
Miyakawa, H., Lev-Ram, V., Lasser-Ross, N., and Ross, W. N. (1992). Calcium transients evoked by climbing fiber and parallel fiber synaptic inputs in guinea pig cerebellar Purkinje neurons. J. Neurophysiol. 68, 1178–1189. doi: 10.1152/jn.1992.68.4.1178
Miyasho, T., Takagi, H., Suzuki, H., Watanabe, S., Inoue, M., Kudo, Y., et al. (2001). Low-threshold potassium channels and a low-threshold calcium channel regulate Ca2+ spike firing in the dendrites of cerebellar Purkinje neurons: a modeling study. Brain Res. 891, 106–115. doi: 10.1016/S0006-8993(00)03206-6
Narain, D., Remington, E. D., Zeeuw, C. I. D., and Jazayeri, M. (2018). A cerebellar mechanism for learning prior distributions of time intervals. Nat. Commun. 9, 469. doi: 10.1038/s41467-017-02516-x
Otsu, Y., Marcaggi, P., Feltz, A., Isope, P., Kollo, M., Nusser, Z., et al. (2014). Activity-dependent gating of calcium spikes by A-type K+ channels controls climbing fiber signaling in Purkinje cell dendrites. Neuron 84, 137–151. doi: 10.1016/j.neuron.2014.08.035
Pellionisz, A., and Llinás, R. (1977). A computer model of cerebellar Purkinje cells. Neuroscience 2, 37–48. doi: 10.1016/0306-4522(77)90066-5
Qiu, J., Yang, Y., Liu, J., Zhao, W., Li, Q., Zhu, T., et al. (2023). The volatile anesthetic isoflurane differentially inhibits voltage-gated sodium channel currents between pyramidal and parvalbumin neurons in the prefrontal cortex. Front. Neural Circuits 17:1185095. doi: 10.3389/fncir.2023.1185095
Rall, W. (1964). “Theoretical significance of dendritic trees for neuronal input-output relations (1964),” in The Theoretical Foundation of Dendritic Function (Cambridge, MA: The MIT Press) 122–146.
Raman, I. M., and Bean, B. P. (1997). Resurgent sodium current and action potential formation in dissociated cerebellar Purkinje neurons. J. Neurosci. 17, 4517–4526. doi: 10.1523/JNEUROSCI.17-12-04517.1997
Raman, I. M., and Bean, B. P. (1999). Ionic currents underlying spontaneous action potentials in isolated cerebellar Purkinje neurons. J. Neurosci. 19, 1663–1674. doi: 10.1523/JNEUROSCI.19-05-01663.1999
Raman, I. M., and Bean, B. P. (2001). Inactivation and recovery of sodium currents in cerebellar Purkinje neurons: evidence for two mechanisms. Biophys. J. 80, 729–737. doi: 10.1016/S0006-3495(01)76052-3
Rancz, E. A., and Häusser, M. (2010). Dendritic spikes mediate negative synaptic gain control in cerebellar Purkinje cells. Proc. Natl. Acad. Sci. U. S. A. 107, 22284–22289. doi: 10.1073/pnas.1008605107
Rancz, E. A., Ishikawa, T., Duguid, I., Chadderton, P., Mahon, S., and Häusser, M. (2007). High-fidelity transmission of sensory information by single cerebellar mossy fibre boutons. Nature 450, 1245–1248. doi: 10.1038/nature05995
Rapp, M., Segev, I., and Yarom, Y. (1994). Physiology, morphology and detailed passive models of guinea-pig cerebellar Purkinje cells. J. Physiol. 474, 101–118. doi: 10.1113/jphysiol.1994.sp020006
Rapp, M., Yarom, Y., and Segev, I. (1992). The impact of parallel fiber background activity on the cable properties of cerebellar purkinje cells. Neural Comput. 4, 518–533. doi: 10.1162/neco.1992.4.4.518
Regan, L. J. (1991). Voltage-dependent calcium currents in Purkinje cells from rat cerebellar vermis. J. Neurosci. 11, 2259–2269. doi: 10.1523/JNEUROSCI.11-07-02259.1991
Rosenblatt, F. (1958). The perceptron: a probabilistic model for information storage and organization in the brain. Psychol. Rev. 65, 386–408. doi: 10.1037/h0042519
Roth, A., and Häusser, M. (2001). Compartmental models of rat cerebellar Purkinje cells based on simultaneous somatic and dendritic patch-clamp recordings. J. Physiol. 535, 445–472. doi: 10.1111/j.1469-7793.2001.00445.x
Rowan, M. J. M., Bonnan, A., Zhang, K., Amat, S. B., Kikuchi, C., Taniguchi, H., et al. (2018). Graded control of climbing-fiber-mediated plasticity and learning by inhibition in the cerebellum. Neuron 99, 999–1015.e6. doi: 10.1016/j.neuron.2018.07.024
Rubin, D. B., and Cleland, T. A. (2006). Dynamical mechanisms of odor processing in olfactory bulb mitral cells. J. Neurophysiol. 96, 555–568. doi: 10.1152/jn.00264.2006
Santamaria, F., and Bower, J. M. (2005). Background synaptic activity modulates the response of a modeled purkinje cell to paired afferent input. J. Neurophysiol. 93, 237–250. doi: 10.1152/jn.00458.2004
Santamaria, F., Tripp, P. G., and Bower, J. M. (2007). Feedforward inhibition controls the spread of granule cell-induced Purkinje cell activity in the cerebellar cortex. J. Neurophysiol. 97, 248–263. doi: 10.1152/jn.01098.2005
Saraga, F., Wu, C. P., Zhang, L., and Skinner, F. K. (2003). Active dendrites and spike propagation in multi-compartment models of oriens-lacunosum/moleculare hippocampal interneurons. J. Physiol. 552, 673–689. doi: 10.1113/jphysiol.2003.046177
Schmidt, H., Stiefel, K. M., Racay, P., Schwaller, B., and Eilers, J. (2003). Mutational analysis of dendritic Ca2+ kinetics in rodent Purkinje cells: role of parvalbumin and calbindin D28k. J. Physiol. 551, 13–32. doi: 10.1113/jphysiol.2002.035824
Schmolesky, M. T., Weber, J. T., De Zeeuw, C. I., and Hansel, C. (2002). The making of a complex spike: ionic composition and plasticity. Ann. N. Y. Acad. Sci. 978, 359–390. doi: 10.1111/j.1749-6632.2002.tb07581.x
Scholfield, C. N. (1978). Electrical properties of neurones in the olfactory cortex slice in vitro. J. Physiol. 275, 535–546. doi: 10.1113/jphysiol.1978.sp012206
Schonewille, M., Belmeguenai, A., Koekkoek, S. K., Houtman, S. H., Boele, H. J., van Beugen, B. J., et al. (2010). Purkinje cell-specific knockout of the protein phosphatase PP2B impairs potentiation and cerebellar motor learning. Neuron 67, 618–628. doi: 10.1016/j.neuron.2010.07.009
Schonewille, M., Gao, Z., Boele, H. J., Vinueza Veloz, M. F., Amerika, W. E., Simek, M. T., et al. (2011). Re-evaluating the role of LTD in Cerebellar Motor Learning. Neuron 14, 43–50. doi: 10.1016/j.neuron.2011.02.044
Schonewille, M., Khosrovani, S., Winkelman, B. H. J., Hoebeek, F. E., De Jeu, M. T. G., Larsen, I. M., et al. (2006). Purkinje cells in awake behaving animals operate at the upstate membrane potential. Nat. Neurosci. 9, 459–61; author reply 461. doi: 10.1038/nn0406-459
Shelton, D. P. (1985). Membrane resistivity estimated for the Purkinje neuron by means of a passive computer model. Neuroscience 14, 111–131. doi: 10.1016/0306-4522(85)90168-X
Siegel, J. J., Kalmbach, B., Chitwood, R. A., and Mauk, M. D. (2012). Persistent activity in a cortical-to-subcortical circuit: bridging the temporal gap in trace eyelid conditioning. J. Neurophysiol. 107, 50–64. doi: 10.1152/jn.00689.2011
Silva, N. T., Ramírez-Buriticá, J., Pritchett, D. L., and Carey, M. R. (2024). Climbing fibers provide essential instructive signals for associative learning. Nat. Neurosci. doi: 10.1038/s,41593-024-01594-7
Solinas, S., Forti, L., Cesana, E., Mapelli, J., De Schutter, E., and D'Angelo, E. (2007). Computational reconstruction of pacemaking and intrinsic electroresponsiveness in cerebellar Golgi cells. Front. Cell. Neurosci. 1, 2. doi: 10.3389/neuro.03.002.2007
Solinas, S. M. G., Maex, R., and De Schutter, E. (2006). Dendritic amplification of inhibitory postsynaptic potentials in a model Purkinje cell. Eur. J. Neurosci. 23, 1207–1218. doi: 10.1111/j.1460-9568.2005.04564.x
Somogyi, P., and Hámori, J. (1976). A quantitative electron microscopic study of the Purkinje cell axon initial segment. Neuroscience 1, 361–365. doi: 10.1016/0306-4522(76)90127-5
Somogyi, R., and Stucki, J. W. (1991). Hormone-induced calcium oscillations in liver cells can be explained by a simple one pool model. J. Biol. Chem. 266, 11068–11077. doi: 10.1016/S0021-9258(18)99129-5
Spain, W. J., Schwindt, P. C., and Crill, W. E. (1987). Anomalous rectification in neurons from cat sensorimotor cortex in vitro. J. Neurophysiol. 57, 1555–1576. doi: 10.1152/jn.1987.57.5.1555
Steuber, V., Mittmann, W., Hoebeek, F. E., Silver, R. A., De Zeeuw, C. I., Häusser, M., et al. (2007). Cerebellar LTD and pattern recognition by Purkinje cells. Neuron 54, 121–136. doi: 10.1016/j.neuron.2007.03.015
Steuber, V., and Schutter, E. (2001). Long-term depression and recognition of parallel fibre patterns in a multi-compartmental model of a cerebellar Purkinje cell. Neurocomputing 38–40, 383–388. doi: 10.1016/S0925-2312(01)00458-1
Steuber, V., Willshaw, D., and Van Ooyen, A. (2006). Generation of time delays: simplified models of intracellular signalling in cerebellar Purkinje cells. Network 17, 173–191. doi: 10.1080/09548980500520328
Stuart, G., and Häusser, M. (1994). Initiation and spread of sodium action potentials in cerebellar Purkinje cells. Neuron 13, 703–712. doi: 10.1016/0896-6273(94)90037-X
Sugimori, M., and Llinás, R. R. (1990). Real-time imaging of calcium influx in mammalian cerebellar Purkinje cells in vitro. Proc. Natl. Acad. Sci. U. S. A. 87, 5084–5088. doi: 10.1073/pnas.87.13.5084
Swensen, A. M., and Bean, B. P. (2005). Robustness of burst firing in dissociated purkinje neurons with acute or long-term reductions in sodium conductance. J. Neurosci. 25, 3509–3520. doi: 10.1523/JNEUROSCI.3929-04.2005
Tanaka, K., Khiroug, L., Santamaria, F., Doi, T., Ogasawara, H., Ellis-Davies, G. C. R., et al. (2007). Ca2+ requirements for cerebellar long-term synaptic depression: role for a postsynaptic leaky integrator. Neuron 54, 787–800. doi: 10.1016/j.neuron.2007.05.014
Tang, Y., An, L., Yuan, Y., Pei, Q., Wang, Q., and Liu, J. K. (2021). Modulation of the dynamics of cerebellar Purkinje cells through the interaction of excitatory and inhibitory feedforward pathways. PLoS Comput. Biol. 17:e1008670. doi: 10.1371/journal.pcbi.1008670
ten Brinke, M. M., Boele, H. J., Spanke, J. K., Potters, J. W., Kornysheva, K., Wulff, P., et al. (2015). Evolving models of pavlovian conditioning: cerebellar cortical dynamics in awake behaving mice. Cell Rep. 13, 1977–1988. doi: 10.1016/j.celrep.2015.10.057
Ten Brinke, M. M., Heiney, S. A., Wang, X., Proietti-Onori, M., Boele, H.-J., Bakermans, J., et al. (2017). Dynamic modulation of activity in cerebellar nuclei neurons during pavlovian eyeblink conditioning in mice. Elife 6:e28132. doi: 10.7554/eLife.28132
Tsodyks, M. V., and Markram, H. (1997). The neural code between neocortical pyramidal neurons depends on neurotransmitter release probability. Proc. Natl. Acad. Sci. U. S. A. 94, 719–723. doi: 10.1073/pnas.94.2.719
Usowicz, M. M., Sugimori, M., Cherksey, B., and Llinás, R. (1992). P-type calcium channels in the somata and dendrites of adult cerebellar Purkinje cells. Neuron 9, 1185–1199. doi: 10.1016/0896-6273(92)90076-P
Vandenberg, C. A. (1987). Inward rectification of a potassium channel in cardiac ventricular cells depends on internal magnesium ions. Proc. Natl. Acad. Sci. U. S. A. 84, 2560–2564. doi: 10.1073/pnas.84.8.2560
Vijayan, A., Gopan, V., Nair, B., and Diwakar, S. (2017). “Comparing robotic control using a spiking model of cerebellar network and a gain adapting forward-inverse model,” in 2017 International Conference on Advances in Computing, Communications and Informatics (ICACCI). (Udupi: IEEE) 566–570. doi: 10.1109/ICACCI.2017.8125900
Vincent, P., and Marty, A. (1996). Fluctuations of inhibitory postsynaptic currents in Purkinje cells from rat cerebellar slices. J. Physiol. 494 (Pt 1) 183–199. doi: 10.1113/jphysiol.1996.sp021484
Violin, J. D., DiPilato, L. M., Yildirim, N., Elston, T. C., Zhang, J., and Lefkowitz, R. J. (2008). beta2-adrenergic receptor signaling and desensitization elucidated by quantitative modeling of real time cAMP dynamics. J. Biol. Chem. 283, 2949–2961. doi: 10.1074/jbc.M707009200
Voogd, J., and Ruigrok, T. J. H. (2004). The organization of the corticonuclear and olivocerebellar climbing fiber projections to the rat cerebellar vermis: the congruence of projection zones and the zebrin pattern. J. Neurocytol. 33, 5–21. doi: 10.1023/B:NEUR.0000029645.72074.2b
Wagner, M. J., Kim, T. H., Savall, J., Schnitzer, M. J., and Luo, L. (2017). Cerebellar granule cells encode the expectation of reward. Nature. 544, 96–100. doi: 10.1038/nature21726
Walter, J. T., and Khodakhah, K. (2009). The advantages of linear information processing for cerebellar computation. Proc. Natl. Acad. Sci. U. S. A. 106, 4471–4476. doi: 10.1073/pnas.0812348106
Wang, Y., Strahlendorf, J. C., and Strahlendorf, H. K. (1991). A transient voltage-dependent outward potassium current in mammalian cerebellar Purkinje cells. Brain Res. 567, 153–158. doi: 10.1016/0006-8993(91)91449-B
Womack, M., and Khodakhah, K. (2002b). Active contribution of dendrites to the tonic and trimodal patterns of activity in cerebellar Purkinje neurons. J. Neurosci. 22, 10603–10612. doi: 10.1523/JNEUROSCI.22-24-10603.2002
Womack, M. D., and Khodakhah, K. (2002a). Characterization of large conductance Ca2+-activated K+ channels in cerebellar Purkinje neurons. Eur. J. Neurosci. 16, 1214–1222. doi: 10.1046/j.1460-9568.2002.02171.x
Womack, M. D., and Khodakhah, K. (2003). Somatic and dendritic small-conductance calcium-activated potassium channels regulate the output of cerebellar Purkinje neurons. J. Neurosci. 23, 2600–2607. doi: 10.1523/JNEUROSCI.23-07-02600.2003
Womack, M. D., and Khodakhah, K. (2004). Dendritic control of spontaneous bursting in cerebellar Purkinje cells. J. Neurosci. 24, 3511–3521. doi: 10.1523/JNEUROSCI.0290-04.2004
Wulff, P., Schonewille, M., Renzi, M., Viltono, L., Sassoe-Pognetto, M., Badura, A., et al. (2009). Synaptic inhibition of Purkinje cells mediates consolidation of vestibulo-cerebellar motor learning. Nat. Neurosci. 12, 1042–1049. doi: 10.1038/nn.2348
Xia, X. M., Fakler, B., Rivard, A., Wayman, G., Johnson-Pais, T., Keen, J. E., et al. (1998). Mechanism of calcium gating in small-conductance calcium-activated potassium channels. Nature 395, 503–507. doi: 10.1038/26758
Xu, J., and Clancy, C. E. (2008). Ionic mechanisms of endogenous bursting in CA3 hippocampal pyramidal neurons: a model study. PLoS ONE 3:e2056. doi: 10.1371/journal.pone.0002056
Yagishita, H., Nishimura, Y., Noguchi, A., Shikano, Y., Ikegaya, Y., and Sasaki, T. (2020). Urethane anesthesia suppresses hippocampal subthreshold activity and neuronal synchronization. Brain Res. 1749:147137. doi: 10.1016/j.brainres.2020.147137
Yamamoto, Y., Lee, D., Kim, Y., Lee, B., Seo, C., Kawasaki, H., et al. (2012). Raf kinase inhibitory protein is required for cerebellar long-term synaptic depression by mediating PKC-dependent MAPK activation. J. Neurosci. 32, 14254–14264. doi: 10.1523/JNEUROSCI.2812-12.2012
Yartsev, M. M., Givon-Mayo, R., Maller, M., and Donchin, O. (2009). Pausing purkinje cells in the cerebellum of the awake cat. Front. Syst. Neurosci. 3:2. doi: 10.3389/neuro.06.002.2009
Yuen, G. L., Hockberger, P. E., and Houk, J. C. (1995). Bistability in cerebellar Purkinje cell dendrites modelled with high-threshold calcium and delayed-rectifier potassium channels. Biol. Cybern. 73, 375–388. doi: 10.1007/BF00199473
Zamora Chimal, C. G., and De Schutter, E. (2018). Ca2+ requirements for long-term depression are frequency sensitive in Purkinje cells. Front. Mol. Neurosci. 11:438. doi: 10.3389/fnmol.2018.00438
Zampini, V., Liu, J. K., Diana, M. A., Maldonado, P. P., Brunel, N., and Dieudonn,é, S. (2016). Mechanisms and functional roles of glutamatergic synapse diversity in a cerebellar circuit. Elife 5:15872. doi: 10.7554/eLife.15872
Zang, Y., and De Schutter, E. (2021). The cellular electrophysiological properties underlying multiplexed coding in Purkinje cells. J. Neurosci. 41, 1850–1863. doi: 10.1523/JNEUROSCI.1719-20.2020
Zang, Y., Dieudonné, S., and De Schutter, E. (2018). Voltage- and branch-specific climbing fiber responses in Purkinje cells. Cell Rep. 24, 1536–1549. doi: 10.1016/j.celrep.2018.07.011
Zárský, V. (2012). Jan Evangelista Purkyně/Purkinje (1787-1869) and the establishment of cellular physiology–Wrocław/Breslau as a central European cradle for a new science. Protoplasma 249, 1173–1179. doi: 10.1007/s00709-012-0407-5
Keywords: Purkinje cell, neuron model, climbing fibers, cerebellum, synaptic plasticity, neuraldynamics, computational models, ion channels
Citation: Fernández Santoro EM, Karim A, Warnaar P, De Zeeuw CI, Badura A and Negrello M (2024) Purkinje cell models: past, present and future. Front. Comput. Neurosci. 18:1426653. doi: 10.3389/fncom.2024.1426653
Received: 01 May 2024; Accepted: 24 June 2024;
Published: 10 July 2024.
Edited by:
Jessica L. Verpeut, Arizona State University, United StatesReviewed by:
Fidel Santamaria, University of Texas at San Antonio, United StatesTimothy J. Ebner, University of Minnesota Twin Cities, United States
Copyright © 2024 Fernández Santoro, Karim, Warnaar, De Zeeuw, Badura and Negrello. This is an open-access article distributed under the terms of the Creative Commons Attribution License (CC BY). The use, distribution or reproduction in other forums is permitted, provided the original author(s) and the copyright owner(s) are credited and that the original publication in this journal is cited, in accordance with accepted academic practice. No use, distribution or reproduction is permitted which does not comply with these terms.
*Correspondence: Aleksandra Badura, YS5iYWR1cmEmI3gwMDA0MDtlcmFzbXVtcy5ubA==; Mario Negrello, bS5uZWdyZWxsbyYjeDAwMDQwO2VyYXNtdXNtYy5ubA==