- 1University of Melbourne, Parkville, VIC, Australia
- 2Central South University, Changsha, China
Climate change is driving the traditional development mode to a green, low-carbon, and sustainable development path. Enterprise green transformation is an important path to achieve green and low-carbon. This study, based on evolutionary game theory, analyzes the driving mechanisms of enterprises green transformation, focusing on the interactive relationship between government policy support and enterprise green transformation. By constructing a game model for both government and enterprises, it examines the impacts of different levels of policy support, enterprise transformation costs, market return expectations, and environmental penalties on enterprise green transformation decisions. The results indicate that high government policy support significantly enhances the success rate of enterprise green transformation, while transformation costs, market returns, and environmental penalties play important moderating roles in the decision-making process. Particularly in the context of the “dual carbon” goals, sustained and stable policy support is crucial for enterprise green transformation. This study further suggests promoting enterprise green transformation through market-oriented measures, digital technology empowerment, and long-term incentive mechanisms, providing theoretical foundations and practical guidance for government policy formulation and enterprise green development.
1 Introduction
Climate change is increasingly becoming a global crisis, posing serious threats to the economy, society, human health, national security, and the environment (Stern, 2008; Suter, 2022; Parmesan and Yohe, 2003; Patz et al., 2005; Lal, 2004; Vörösmarty et al., 2000). These issues compel us to shift from traditional development models to green, low-carbon, and sustainable pathways (Wang et al., 2021; Owen et al., 2018; Hou et al., 2018). Against this backdrop, the Paris Agreement was adopted at the 21st UN Climate Change Conference in 2015, with the long-term goal of limiting the global average temperature rise to within 2 degrees Celsius above pre-industrial levels, and striving to limit it to 1.5 degrees Celsius. The Paris Agreement encourages parties to participate in global climate action through “nationally determined contributions, “actively transitioning to green and sustainable growth models, and avoiding the continued threat to natural ecosystems posed by the decades-long reliance on fossil fuels. In 2020, the Chinese government also announced its “dual carbon” goals, aiming for peak carbon emissions and carbon neutrality, which are crucial measures for transforming energy policies toward low-carbon and zero-carbon approaches.
China’s economic development faces severe resource constraints, environmental limitations, and immense energy consumption (Tang et al., 2021; Wen and Chen, 2008; Xu et al., 2002; MacBean, 2007). The shift toward green and low-carbon development is urgent and represents an inevitable path for high-quality growth. As fundamental economic organizations and units of society, enterprises need to pursue economic benefits while also assuming social responsibilities for sustainable economic, environmental, and social development. Enterprises can reduce their environmental impact by promoting clean energy, energy-saving and emission-reduction technologies, and environmentally friendly products, while also stimulating their innovative capabilities and driving technological progress and industrial upgrades.
Among these, innovative enterprises play a leading role in green transformation, making their transition to green practices particularly significant. Innovative enterprises view technological innovation as the core driving force of green transformation. By introducing and developing new technologies, these enterprises can reduce energy consumption and pollution during production processes, improve resource utilization efficiency, and achieve green, low-carbon production methods (Zhou et al., 2022; Ma et al., 2022; Zhang et al., 2022; Zhao et al., 2022). This, in turn, can guide more enterprises onto the right path of green transformation. Moreover, the continuous exploration of green transformation by innovative enterprises is a crucial prerequisite for successfully achieving this transition. Given this context, the core issue that this study aims to explore is: Under government policy support, what is the driving mechanism behind enterprises’ sustained engagement in green transformation?
2 Literature review
Currently, many studies focus on the impact mechanisms of digital innovation on enterprise green transformation. Numerous studies using panel data correlation analysis have shown that digital transformation facilitates enterprise green innovation (Guo B. et al., 2024). They have identified significant mediating mechanisms between enterprise R&D investment and government green subsidies. Furthermore, digital transformation can enhance companies’ levels of environmental responsibility through micro-level pathways, such as improving internal disclosures and increasing external investor attention, ultimately advancing enterprise green transformation (Li et al., 2023; Liang et al., 2023; Shen et al., 2023; Zhang et al., 2024; Shang et al., 2023). The application of industrial robots can also promote enterprise green innovation by improving production efficiency, human capital, and environmental governance levels. The government plays a significant role in this process. On one hand, government-guided funds have a notable positive effect on enterprise green transformation (Sun et al., 2024). On the other hand, the promoting effect of digital transformation on green investment is greater in regions with strong government support and stringent environmental regulations (Xu et al., 2024). Overall, enterprise green innovation is influenced by a combination of policy environment, market environment, and digital culture.
In addition, some studies have explored enterprise green transformation mechanisms through evolutionary game analysis. For instance, Fan et al. analyzed the tripartite game among the government, enterprises, and consumers during the green supply chain transformation process. The results indicate that, under certain constraints, the green behaviors of the government and consumers incentivize supply chain enterprises (Fan et al., 2024). Liu et al. applied the evolutionary game model to a two-tier green supply chain consisting of green suppliers and green manufacturers to analyze various internal and external influencing factors, aiming to avoid “free-riding” behavior (Liu et al., 2022b). Cui et al. constructed an evolutionary game model involving the government, coal-fired power enterprises, and banks to clarify the key factors and mechanisms influencing green transformation under green credit for coal power enterprises (Cui et al., 2022). Wu developed an evolutionary game model involving industrial enterprises, local governments, and the central government to analyze the dynamic interactions among vertical decentralization, environmental regulation, and enterprise pollution (Wu et al., 2024). This study identified that fiscal incentives from the central government to local governments are crucial drivers for promoting green transformation, while the additional social benefits arising from local government oversight and governance are key factors in managing green transformation.
Based on existing research findings, the current research object primarily focus on how digital transformation drives green transformation in enterprises, while there is a lack of systematic responses regarding the driving mechanisms from the perspective of government policy support. At the same time, previous studies mostly focused on enterprises in different fields. Under the current background of rapid development of digital technology, innovative enterprises play an important role in green transformation, but they have not been taken as research objects in the past. Additionally, when comparing the two research methods, panel data analysis can simultaneously control for time effects and individual effects, providing more accurate estimates and inferences. In contrast, evolutionary game analysis is better suited for handling interactive behaviors within complex adaptive systems, where individual actions and strategies influence and adapt to one another, ultimately forming overall evolutionary dynamics (Liu et al., 2022a; Yang et al., 2023; Guo S. et al., 2024). In comparison, the evolutionary game method is more effective for digging deeply into the mechanism of green transformation of enterprises generated by multi-agent actions. Compared with the current research using the evolutionary game method, this study combined with field research to construct the evolutionary game model and numerical simulation more scientifically, to promote the practice more pertinently. This study leans toward starting from practical systems, focusing on the dynamic strategic changes and interactive processes of enterprises during their green transformation, which offers advantages in exploring the driving mechanisms under multiple influencing factors.
This study will focus on innovative enterprises in China as the research object and use evolutionary game theory to analyze the mechanisms of corporate green transformation. First, based on the clarification of the research methods and data sources (Section 3), an evolutionary game model will be constructed, and the evolutionary stable strategies of each stakeholder will be analyzed (Section 4). Finally, appropriate cases will be selected to verify the evolutionary stable strategies and explore the role of different factors in the mechanism (Section 5). The discussion and conclusion are presented in Sections 6 and 7, respectively.
3 Research methods and data sources
This study employs a combined approach of semi-structured interviews and evolutionary game analysis. Semi-structured interviews are a method that in enterprises both structured and open-ended elements (Kvale and Brinkmann, 2009). Researchers design a series of key questions in advance but can adjust or expand upon these questions during the interview based on the respondents’ answers. This approach helps gather quantitative data and in-depth qualitative information. For example, semi-structured interviews can assist researchers in collecting real feedback about the strategy choices, decision-making processes, and interactive behaviors of various parties.
Evolutionary game theory focuses on how participants’ strategies evolve over time within dynamic and complex systems (McNamara, 2013; Ji et al., 2015; Fan and Hui, 2020). It is particularly suitable for analyzing how parties adjust their strategies in response to environmental changes across multiple games. The evolutionary game model describes the strategy updating and selection processes of individuals or groups through mechanisms such as replication dynamics, simulating the long-term evolutionary trends of different strategies.
The semi-structured interviews provide valuable qualitative information for the evolutionary game analysis, especially in complex social or organizational contexts, revealing the motivations, strategies, and preferences of actors. These qualitative data help researchers set initial conditions, strategy sets, and payoff functions—key parameters within the game model. The case data and contextual knowledge collected during interviews provide realistic foundations for the assumptions and structural design of the evolutionary game model, ensuring that the model is closely aligned with actual circumstances. Additionally, the qualitative data obtained through semi-structured interviews can serve as references for verifying and adjusting subsequent numerical analyses, thereby enhancing the model’s accuracy and predictive capability. After the research conclusion is drawn, the interview data can further verify the results to improve the scientific nature of the research.
The data for this study comes from interviews with 10 management personnel from 5 technology companies in Hunan Province. Firstly, Hunan Province was selected for this study due to its unique economic and industrial context. As one of the key provinces in central China, Hunan has made significant strides in developing its technology sector, and this provides a valuable case study for understanding the dynamics of green transformation within technology firms. Moreover, the provincial government has been actively promoting green initiatives and sustainability, which makes it an interesting and relevant context for examining the impact of policy support on green transformation. Therefore, the selection of Hunan is grounded in its representativeness of an emerging, rapidly developing region where technology firms are increasingly facing pressures to align with national sustainability goals.
For the interview questions, it is gradually in-depth layer by layer. First, we know the basic situation of the enterprise, including industry sector, establishment date, scale (number of employees, annual revenue), location, and green transformation efforts. Secondly, we further understand the influencing factors of enterprises’ green transformation, such as policy support, government punishment, and so on. Some of these factors were revealed by the staff in explaining why the green transformation was carried out, and some were determined by our active inquiry. Finally, the important data involved in the study were collected, including the amount of investment, the amount of government support funds, the amount of government punishment, and so on.
4 Construction and analysis of evolutionary game model
4.1 Theoretical model construction
To implement the new development philosophy, meet the intrinsic requirements of high-quality development, and promote the green transformation of the economy and society, enterprises are undertaking green transformation with policy support. Among them, the green transformation of enterprises refers to the process in which businesses actively adopt green low-carbon technologies, optimize resource utilization, and reduce environmental pollution in their production, operation, and management activities to achieve sustainable development. Green transformation not only focuses on improving the enterprise’s own environmental performance but also covers enhancing social responsibility, economic benefits, and corporate competitiveness. Its core goal is to transition enterprises from traditional high-energy consumption and high-emission production methods to energy-saving, environmentally friendly, and low-carbon approaches, thereby promoting sustainable development in economic, environmental, and social dimensions. Government policy support refers to the various policy tools and measures used by the government to drive businesses or society as a whole toward achieving sustainable development goals. This policy support manifests mainly in five areas: fiscal and tax policy support, government procurement support, technological innovation support, financial support, and infrastructure development.
1. Fiscal and tax policy support refers to government measures, such as fiscal subsidies and tax incentives, aimed at fostering the development of green and low-carbon industries to promote high-quality economic growth (Li, 2009). Government procurement support involves increasing the procurement of green products by the government, such as promoting photovoltaic and solar thermal products, new energy vehicles, and green building materials. This encourages large retail enterprises and e-commerce platforms to enrich green consumption scenarios and optimize the purchasing environment.
2. Technological innovation support entails the government’s establishment of a green low-carbon technology innovation system, supporting enterprises in green technology R&D, and creating pilot bases for the conversion of green technology achievements, while also promoting the development of specialized technology transfer institutions (Cai et al., 2015). Financial support involves utilizing national industrial-financial cooperation platforms to leverage financial resources for the green low-carbon transformation of manufacturing, establishing a project library for green technology upgrades and benchmark enterprises, and increasing support for green and transitional finance.
3. Infrastructure development focuses on accelerating the green upgrade of infrastructure, promoting the low-carbon transformation of energy systems, and improving the living environment in urban and rural areas (Ma and Lin, 2023). Policy support not only provides external incentives and guidance for enterprise green transformation but also influences enterprise decisions and behaviors through legal, market, and technological channels.
4. For enterprises, green transformation offers multiple positive effects, enhancing market competitiveness while providing long-term economic and social benefits (Jiang et al., 2020; Han et al., 2023). From a competitive standpoint, green transformation helps companies gain consumer favor in the increasingly growing environmental protection market, establish a green brand image, and seize more business opportunities (Lin et al., 2023). Additionally, the application of green technologies can optimize production processes, reduce energy consumption, and minimize resource waste, effectively lowering operational costs (Shi et al., 2021). While significant initial investments may be required for the transition, enterprises can achieve substantial cost savings in the long run by improving resource utilization and reducing emissions and energy consumption. Moreover, green transformation drives continuous technological innovation within enterprises to meet environmental requirements, promoting the development and application of new technologies and opening up new market opportunities. The transformation also enhances risk management capabilities, enabling companies to adapt to increasingly stringent environmental regulations, reduce compliance risks, and mitigate social pressures, while improving their capacity to respond to external environmental changes such as climate change. Green transformation also enhances a company’s sense of social responsibility, boosting brand value and public recognition, and earning support from society and communities. Additionally, by improving the work environment and reducing the use of harmful substances, enterprises can enhance employee health and job satisfaction, fostering a sense of belonging among staff. Driven by global sustainable development strategies, green transformation aligns with the long-term needs of enterprises, helping them reap policy benefits such as tax reductions and green financing. Furthermore, the green transformation of enterprises contributes positively to environmental protection and global climate change mitigation, supporting the construction of a sustainable economy and promoting collective progress in society and the economy.
However, the green transformation of enterprises is not guaranteed to succeed; its success is influenced by multiple factors, which in turn affect the effectiveness of policy support and the decision to pursue transformation. The uncertainty of green transformation refers to the risks and challenges that may arise during the process of driving businesses or society toward green transformation due to various changes and unpredictable factors. These uncertainties stem from multiple aspects, such as policy, market, technology, funding, and the environment, often making the path and outcomes of green transformation difficult to predict, and potentially affecting the effectiveness and success of decision-making. Firstly, internal resources, technological capabilities, and management models are critical factors. Small and medium-sized enterprises with limited resources may struggle to bear the substantial costs associated with green transformation. Additionally, whether management has a long-term sustainable development strategy directly impacts the effectiveness of the transformation. The market environment and industry competition landscape are equally important. In a mature market with strong demand for green products, companies are more likely to benefit from transformation; however, in a market with weak demand, maintaining transformation can be challenging. The strength and continuity of policy support are also key driving forces; if policies lack stability or are insufficiently robust, enterprises may fail to secure ongoing external support, affecting transformation outcomes. The uncertainty surrounding technological innovation presents further challenges, as technological bottlenecks or difficulties in applying new technologies can lead to unmet transformation expectations. Social and public environmental awareness is another vital factor, as green transformation often requires positive societal feedback to achieve market returns. The relationship between policy support and enterprise transformation is reciprocal; if enterprises encounter obstacles during transformation, the strength of policy support may diminish, subsequently affecting the transformation motivation of other companies. Overall, promoting green transformation requires careful consideration of the real conditions of enterprises, market demand, and technological development, while also providing long-term and stable policy support to ensure the sustainability of green development.
Based on the analysis above, an evolutionary game theory model of the driving mechanism for green transformation in enterprises is constructed, as illustrated in Figure 1.
4.2 Interest matrix construction
In an environment characterized by information asymmetry and opportunism, both enterprises and the government are considered bounded rational players in the game. Enterprises have two strategies: “transformation” and “non-transformation,” while the government has two strategies: “high policy support” and “low policy support.” Based on the analysis, the key factors influencing the strategy choices of both the government and enterprises are identified as follows: (1) the probability of successful transformation affects the strategy choices of both government and enterprises; (2) the government’s strategy choice is influenced by the investment in policy support and the expected social benefits brought about by the enterprises’ green transformation; (3) the enterprises’ strategy choice is influenced by the investment in green transformation, the expected increase in market returns from transformation, and the differences in government penalties before and after transformation. The corresponding parameters and their descriptions are summarized in Table 1.
For enterprises, when choosing the “transformation” strategy and the government opts for a “high policy support investment” strategy, the overall impact of the probability of successful green transformation under high policy support, the investment in green transformation under high policy support, the expected increase in market returns from transformation, and the anticipated penalties related to safety and environmental protection results in a payoff of p1×(E3+E4−E5)−E1−E4p1×(E3+E4−E5)−E1−E4 . When the enterprise chooses the “transformation” strategy and the government selects a “low policy support investment” strategy, the combined effects of the probability of successful green transformation under low policy support, the investment in green transformation under low policy support, the expected increase in market returns from transformation, and the anticipated penalties related to safety and environmental protection result in a payoff of p2×(E3+E4−E5)−E2−E4p2×(E3+E4−E5)−E2−E4 . When the enterprise chooses the “non-transformation” strategy and the government opts for a “high policy support investment” strategy, the payoff is influenced by the penalties associated with not transforming in terms of safety and environmental protection, resulting in a payoff of −E4−E4 .
For the government, when it chooses a “high policy support investment” strategy and the enterprise selects the “transformation” strategy, the overall impact of the probability of successful green transformation under high policy support, the investment in high policy support, and the expected social benefits from successful transformation results in a payoff of p1×G3−G1p1×G3−G1 . When the government opts for a “low policy support investment” strategy while the enterprise chooses the “transformation” strategy, the combined effects of the probability of successful green transformation under high policy support, the investment in high policy support, and the expected social benefits from successful transformation yield a payoff of p2×G3−G2p2×G3−G2 . When the enterprise chooses the “non-transformation” strategy, the government’s payoff is 0.
Additionally, the probability of the enterprise’s transformation is denoted as x, and the probability of the government providing high policy support is denoted as y. Based on this, the payoff matrix for the game between the enterprise and the government is shown in Table 2.
4.3 Evolutionary game model analysis
4.3.1 Revenue expectation function construction
According to Table 2, the expected returns and average expected returns of enterprises in green transformation and non-transformation are shown in Equations 1–3:
Expected returns and average expected returns of high and low government policy support are shown in Equations 4–6:
Under the replicator dynamic thought, the replication dynamic equation are shown in Equations 7, 8:
4.4 Evolutionary strategy stability analysis
4.4.1 ESS of enterprise
Find the first partial derivative with respect to x of the replication dynamic equation F(x,y)F(x,y) of the enterprise in Equation 7, as shown in Equation 9:
Let y×[(p1−p2)×(E3+E4−E5)+(E2−E1)]−p2×(E2+E3+2×E4−E5)−E4=y×[(p1−p2)×(E3+E4−E5)+(E2−E1)]−p2×(E2+E3+2×E4−E5)−E4=0, y∗=p2×(E2+E3+2×E4−E5)+E4(p1−p2)×(E3+E4−E5)+(E2−E1)y∗=p2×(E2+E3+2×E4−E5)+E4(p1−p2)×(E3+E4−E5)+(E2−E1) . That is, when y=y∗y=y∗ , F(x,y)=0F(x,y)=0 , It shows that all the levels are evolutionarily stable states, and there is no difference between the benefits of transformation and non-transformation. At this time, the ESS of the enterprise cannot be determined. At that time of y≠y∗y≠y∗ , to make F(x,y)=0F(x,y)=0 , you can get two stable points of x=0x=0 and x=1x=1 . If the probability of an enterprise’s response to strategy selection is in a stable state, the conditions to be met are F(x,y)=0F(x,y)=0 , and ∂F(x,y)∂x<0∂F(x,y)∂x<0 . In order to find the stable point, we need to find the solution that makes the whole expression zero. As can be seen, the solution of the equation depends on the relationship between 𝑦 (government support) and the parameters.
When y = 0 (i.e., when the government does not provide any support): the equation simplifies to −p2×(E2+E4)+E4=0−p2×(E2+E4)+E4=0 , get p2=E4E2+E4p2=E4E2+E4 . That is, when the critical value is reached, the enterprise’s transformation motivation will disappear, that is, 𝑥 will tend to 0. At this time, the stability strategy is x = 0, that is, the enterprise chooses not to carry out green transformation.
When 𝑦=1 (i.e., when the government provides the highest support): the equation is simplified to (p1−p2)×(E3+E4−E5)+(E2−E1)−p2×(E2+E3+2×E4−E5)−E4=0,(p1−p2)×(E3+E4−E5)+(E2−E1)−p2×(E2+E3+2×E4−E5)−E4=0, which means that the transformation probability x of the enterprise will depend on the combination of policy support and market conditions. If it is larger and the market income is larger, the enterprise will be more inclined to choose the transformation strategy. This means that when it is significantly greater than and the market returns are high, the enterprise has a strong motivation for transformation, and the government’s policy support has become the main factor affecting the decision of the enterprise. At this time, the stability strategy is x = 1, that is, the enterprise chooses to carry out green transformation.
Therefore, when y = 0 (that is, there is no policy support from the government), the transformation probability of an enterprise mainly depends on p2 and related transformation costs and penalties. When the threshold is reached E4E2+E4E4E2+E4 , the enterprise will not transform. When y = 1 (that is, the government provides the highest support), the transformation probability of the enterprise mainly depends on p1, market expected revenue E3, transformation cost E2, and penalty E4. If p1 is large enough and expected returns are high, companies will choose green transformation.
4.4.2 ESS of government
Find the first partial derivative with respect to y of the replicated dynamic equation G(x,y) of the emergency department in Equation 8, as shown in Equation 10:
At that time of x=x∗=0x=x∗=0 , it shows that all levels are evolutionarily stable states at this time, and there is no difference in the benefits of high policy support strategies and low policy support strategies adopted by the government. At this time, the ESS of government departments cannot be determined. At that time of x≠0x≠0 , to make G(x,y)=0G(x,y)=0 , you can get two stable points of y=0y=0 and y=1y=1 . If the probability of government policy selection is in a stable state, the conditions to be met are G(x,y)=0G(x,y)=0 and ∂G(x,y)∂x<0∂G(x,y)∂x<0 . Then analyze different situations:
1. When (p1−p2)×G3−G2−G1>0,(p1−p2)×G3−G2−G1>0, ∂G(x,y)∂x|y=0>0,∂G(x,y)∂x|y=1<0∂G(x,y)∂x∣∣y=0>0,∂G(x,y)∂x∣∣y=1<0 . Therefore, when p1−p2∈(G1+G2G3,1)p1−p2∈(G1+G2G3,1) and y=1y=1 , ∂G(x,y)∂x<0∂G(x,y)∂x<0 and G(x,y)=0G(x,y)=0 , this indicates the ESS point is y=1y=1 .
2. When (p1−p2)×G3−G2−G1<0,∂G(x,y)∂x|y=0<0,∂G(x,y)∂x|y=1>0(p1−p2)×G3−G2−G1<0,∂G(x,y)∂x∣∣y=0<0,∂G(x,y)∂x∣∣y=1>0
Therefore, when p1−p2∈(0,G1+G2G3)p1−p2∈(0,G1+G2G3) , and y=0y=0 , ∂G(x,y)∂x<0∂G(x,y)∂x<0 and G(x,y)=0G(x,y)=0 , this indicates the ESS point is y=0y=0 .
Based on the above analysis, it can be concluded that when the difference in the probability of successful green transformation under high policy support and low policy support exceeds a certain threshold, the government will choose the high policy support strategy. Conversely, when the difference in the probability of successful green transformation under high policy support and low policy support is below that threshold, the government will opt for the low policy support strategy.
Therefore, when the gap in the probability of successful green transformation between high policy support and low policy support exceeds a critical value, the government is likely to adopt a high policy support strategy. In other words, if an increase in policy support significantly improves the probability of successful green transformation for enterprises, the government will be inclined to enhance policy support. This logic reflects the government’s decision-making mechanism for effectively promoting enterprises’ green transformation through stronger policy support. On the other hand, when the difference in the probability of successful green transformation between high policy support and low policy support is less than a critical value, the government will choose the low policy support strategy. This means that if the impact of increasing policy support on the success of enterprise transformation is minimal, the government will refrain from providing high policy support. This reflects the rational choice of the government, indicating a reluctance to invest excessive resources when the success rate of transformation is unlikely to improve significantly.
5 Numerical analysis based on a case
5.1 Case introduction
Zhongqing Changtai (Changsha) Intelligent Technology Co., Ltd. was established in August 1999 and is a holding subsidiary of China National Light Industry Group Co., Ltd. The company currently employs 484 people and holds 442 independent intellectual property rights, achieving an annual output value of 1.8 billion RMB. It is a leading supplier of integrated intelligent equipment systems for machinery, electronics, instrumentation, and networking, providing comprehensive solutions covering system planning, R&D design, manufacturing, installation, commissioning, and after-sales service. The company focuses on the research and manufacturing of intelligent logistics warehousing systems, intelligent equipment systems, and digital factories. With years of technical accumulation and market experience, its performance spans various industries including new energy, steel, timber, rail transportation, hardware manufacturing, machinery manufacturing, food and pharmaceuticals, pulp and paper, printing and packaging, textile and chemical fibers, and household paper, capturing over 80% of the market share in the domestic pulp and paper industry’s completed product segments.
According to research, with strong government support, the company has invested approximately 25 million RMB in green innovation. The green transformation has increased the company’s market returns by 2 percentage points, indicating that E1 = 2,500 and E3 = 18,000 × 0.2 = 3,600. Assuming that low government policy support increases the company’s investment by 5 million RMB, then E2 = 3,000. Regarding environmental and safety penalties for the company, prior to the green transformation, it faced penalties of about 5 million RMB annually; after the transformation, this decreased to approximately 1 million RMB per year. Thus, E4 = 500 and E5 = 100.
For the government, high policy support for the enterprise is reflected in fiscal and tax policy support, government procurement support, technical innovation support, financial support, and infrastructure construction, amounting to around 5 million RMB. Low policy support is shown as partial support, amounting to approximately 1 million RMB. Therefore, G1 = 500 and G2 = 100. The social benefits generated by the enterprise’s green transformation are long-term, with an annual benefit of 3 million RMB, leading to a total of 15 million RMB over 5 years. Hence, G3 = 1,500.
This results in P2 = 0.14 and P1–P2 = 0.4, which are the thresholds affecting the decisions of both the enterprise and the government. The above data will facilitate the numerical analysis. When the initial willingness of both the enterprise and the government is 0.5, i.e., x = y = 0.5, this study will use Python software to explore the dynamics of the green transformation in the case study.
5.2 Numerical analysis under different scenarios
This study conducts numerical analysis based on different scenarios of p1 and p2 (where p1 is significantly greater than p2, p1 and p2 are close, both p1 and p2 are high, and both p1 and p2 are low). The six scenarios are “p1 = 0.3, p2 = 0.1,” “p1 = 0.5, p2 = 0.1,” “p1 = 0.7, p2 = 0.1,” “p1 = 0.5, p2 = 0.3,” “p1 = 0.7, p2 = 0.3,” and “p1 = 0.8, p2 = 0.3,” as shown in Figure 2.
Scenario 1 (p1 = 0.3, p2 = 0.1): In this combination, the probability of the enterprise’s transformation rapidly rises to nearly 1, while the government’s probability of providing high policy support almost immediately drops to nearly 0. Despite the government opting for lower policy support, the enterprise still chooses to transform. This may be due to strong internal motivations for transformation, such as considerations of market competition and environmental regulations. The low level of government support indicates that, in this scenario, the government does not perceive sufficient social benefits to justify continued support.
Scenario 2 (p1 = 0.5, p2 = 0.1): The probability of the enterprise’s transformation also quickly rises and approaches 1, while the government’s probability of high policy support remains at 0.5. As p1 increases further, the enterprise’s likelihood of green transformation significantly increases, but the government is currently unable to determine the optimal policy solution.
Scenario 3 (p1 = 0.7, p2 = 0.1): The probability of the enterprise’s transformation rapidly rises to nearly 1, and the government’s probability of high policy support also swiftly increases to 1. With further increases in p1, the enterprise’s likelihood of green transformation significantly increases, leading the government to choose a high policy support strategy due to the anticipated social benefits of the relevant policies.
Scenario 4 (p1 = 0.5, p2 = 0.3): The probability of the enterprise’s transformation initially drops rapidly to 0, and the government’s probability of high policy support also immediately falls to nearly 0. As the low support level p2 increases, the government’s support remains low. This indicates that in this scenario, the enterprise can complete its green transformation through its own motivation even when policy support is low. Thus, the government is less inclined to provide high policy support.
Scenario 5 (p1 = 0.7, p2 = 0.3): Similar to the previous scenario, the enterprise’s probability of transformation quickly drops to 0, while the government’s probability of high policy support remains at 0.5. As p2 increases further, the likelihood of the enterprise’s green transformation continues to decrease, and the government is unable to determine the optimal policy solution.
Scenario 6 (p1 = 0.8, p2 = 0.3): The probability of the enterprise’s transformation rapidly reaches 1, and the government’s probability of high policy support also quickly rises to 1. At the highest values of p1 and p2, the enterprise is almost certain to pursue green transformation, and the government’s policy support is maximized. This indicates that under extremely high success probabilities and strong internal motivation, the enterprise’s transformation is almost independent of government policy support.
Overall, these conclusions validate the stable evolutionary strategy proposed in this study. Moreover, Scenario 6 aligns more closely with the actual circumstances of the aforementioned case. The employee clearly stated in the interview that: “Even with limited government support, we can still make progress. However, government policy support is still crucial for us. In the case of high policy support, there are clear incentives—such as subsidies, tax breaks, and favorable regulations—that make it easier for us to invest in green technologies and processes. This high level of support significantly increases the likelihood of our green transformation’s success.” This further validates the conclusion of this paper.
5.3 Numerical analysis of parameter variation
This study intends to further explore the impact of parameter changes on government and enterprise strategy choice based on the background of Scenario 4. This study analyzes the impact of changes in E1, E2, E3, and E4 on x and y, with results shown in Figure 3. The specifics are as follows:
1. Impact of E1 on x and y: When E1 is large, enterprises face higher investments under the government’s high policy support, leading them to prefer a non-transformation strategy. By reducing the value of E1, enterprises can be encouraged to choose green transformation. Therefore, with other factors held at their initial values (p1 = 0.5, p2 = 0.3, E2 = 3,000, E3 = 3,600, E4 = 500, E5 = 100, G1 = 500, G2 = 100, G3 = 1,500), lowering the value of E1 (E1 = 2,500, 2000, 1,500, 1,000, 500) results in the findings shown in Figure 3a. The results indicate that E1 must be reduced to 500 to promote green transformation.
2. Impact of E2 on x and y: A larger E2 means that enterprises face greater investments under the government’s low policy support, further pushing them toward a non-transformation strategy. Therefore, with other factors maintained at their initial values (p1 = 0.5, p2 = 0.3, E1 = 2,500, E3 = 3,600, E4 = 500, E5 = 100, G1 = 500, G2 = 100, G3 = 1,500), lowering E2 (E2 = 3,000, 2,900, 2,800, 2,700, 2,600) leads to the results shown in Figure 3b. The results suggest that reducing E2 does not effectively promote green transformation.
3. Impact of E3 on x and y: A larger E3 indicates greater market benefits from successful transformation, making enterprises more likely to choose green transformation strategies. Therefore, with other factors held at their initial values (p1 = 0.5, p2 = 0.3, E1 = 2,500, E2 = 3,000, E4 = 500, E5 = 100, G1 = 500, G2 = 100, G3 = 1,500), increasing E3 (E3 = 4,000, 4,500, 5,000, 5,500, 6,000, 6,500) results in findings shown in Figure 3c. The results indicate that increasing E3 does not effectively promote green transformation.
4. Impact of E4 on x and y: A larger E4 means that enterprises face greater penalties related to safety and environmental protection, potentially leading them to choose green transformation strategies. Therefore, with other factors held at their initial values (p1 = 0.5, p2 = 0.3, E1 = 2,500, E2 = 3,000, E3 = 3,600, E4 = 500, E5 = 100, G1 = 500, G2 = 100, G3 = 1,500), increasing E4 (E4 = 500, 1,000, 1,500, 2,000) leads to the results shown in Figure 3d. The results indicate that once E4 reaches 1,500, the enterprise chooses the green transformation strategy.
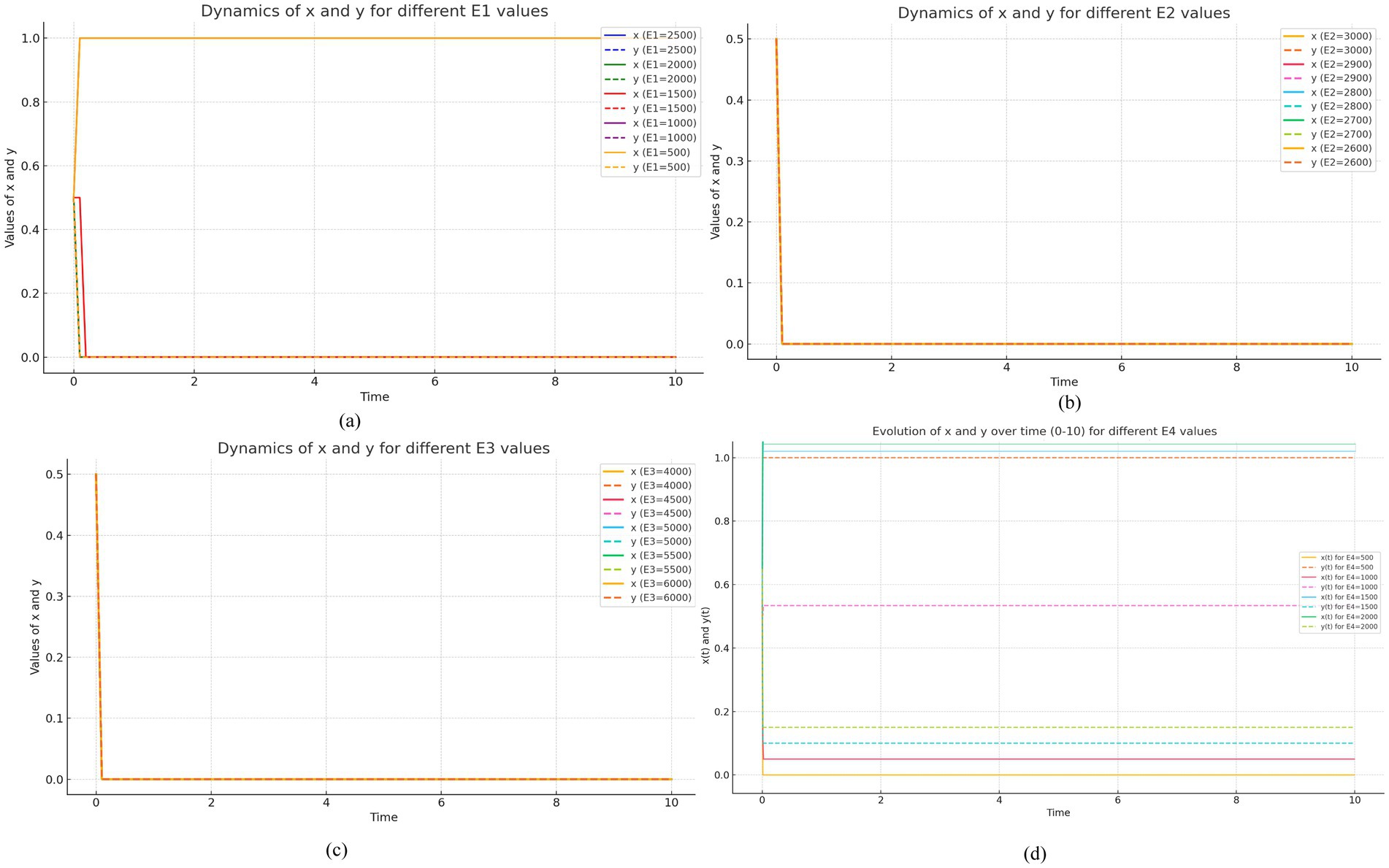
Figure 3. Numerical analysis of parameter changes for enterprises. ((a–d) represent the dynamics of x and y for different E1, E2, E3, and E4, respectively).
6 Discussion
This study delves into the role of government policy support in driving the green transformation strategies of enterprises. Our findings offer several insights into how government interventions, particularly in the form of policy support, interact with enterprise decision-making processes. These insights have significant implications for both policymakers and businesses seeking to align their strategies with sustainability goals.
Firstly, our analysis confirms that when government support is low, enterprises’ probability of choosing a green transformation is primarily determined by factors such as transformation costs and the associated penalties. In such a scenario, enterprises are often deterred from pursuing green transformation unless they are faced with significant penalties or high costs. This suggests that, under low policy support, enterprises are more likely to view the transformation process as a financial burden, and without the necessary incentives or regulatory pressure, they may opt against it. However, when government support reaches its maximum, the situation shifts. Under high policy support, market expected returns, transformation costs, and penalties become the dominant factors influencing the transformation decision. If the market is favorable and expected returns are high enough, enterprises are more likely to commit to green transformation. This suggests that while government policy is important, market conditions also play a crucial role in driving sustainable practices within enterprises. These findings highlight the need for a balanced approach, where both policy incentives and favorable market conditions work synergistically to promote green transformation.
Secondly, our study investigates how the government adjusts its policy strategy based on the differential impact of policy support on enterprises. We find that if the difference in the probability of successful green transformation between high and low policy support exceeds a certain threshold, the government is inclined to choose a high policy support strategy. Conversely, when the difference is minimal, a low policy support strategy is preferred. This suggests that government decisions regarding policy support are dynamic and responsive to the effectiveness of those policies in driving transformation. In essence, the government is likely to adopt a high support strategy when it can significantly improve the likelihood of successful transformations, thus ensuring a more substantial impact on green initiatives. This observation emphasizes the importance of continuously assessing the effectiveness of policy support mechanisms and adjusting them accordingly to optimize their impact.
Lastly, the numerical analyses conducted in this study reveal that reducing the financial input required from enterprises under high policy support can encourage green transformation. Additionally, increasing government-imposed penalties for environmental and safety violations appears to be an effective means of motivating enterprises to adopt green transformation strategies. These findings suggest that a dual approach—lowering the financial burden on businesses while strengthening regulatory penalties—can create a favorable environment for green transformation. It also underscores the need for governments to implement policies that not only incentivize but also enforce compliance with environmental and safety standards.
In conclusion, our study reinforces the idea that government policy support plays a pivotal role in the adoption of green transformation strategies by enterprises. However, the effectiveness of such policies depends on a combination of factors, including market conditions, transformation costs, penalties, and the overall balance between incentives and regulations. For policy to be most effective, it must be adaptable, data-driven, and responsive to the needs and behaviors of enterprises.
7 Conclusion and prospect
This study analyzes the motivation mechanisms for enterprises to undertake green transformation under government policy support through an evolutionary game model. The research indicates that the intensity of government policy support, the cost of transformation for enterprises, market expected returns, and environmental penalties are key factors influencing the decision-making process for green transformation. Specifically:
1. When government policy support is low, the probability of enterprise transformation primarily relies on p2 and the associated transformation costs and penalties. If a critical value is reached, enterprises will not transform. When the government provides maximum support, the probability of transformation mainly depends on market expected returns (E3), transformation costs (E2), and penalties (E4). If p1 is sufficiently high and expected returns are favorable, enterprises will choose green transformation. This conclusion has been validated.
2. When the difference in the probability of successful green transformation for enterprises under high and low policy support exceeds a certain value, the government will opt for a high policy support strategy. Conversely, if the difference is below that value, the government will choose a low policy support strategy. This conclusion has also been validated.
3. Numerical analyses under varying conditions reveal that reducing the input of enterprises under high policy support and increasing government penalties for safety and environmental issues can effectively promote the choice of green transformation strategies by enterprises.
4. Looking ahead, as the “dual carbon” goals are further advanced, the government will increase its policy support for the green economy, and enterprises will gain more opportunities in green technology innovation. To better promote the green transformation of enterprises, several areas warrant further research and attention: (1) There is a need to study how to combine government policy support with market mechanisms to encourage more enterprises to actively engage in green transformation and incentivize green innovation through market-based methods. (2) The ways in which digital transformation can further enhance the green innovation capabilities of enterprises deserve in-depth exploration. The integration of digital technologies can drive efficiency improvements in green transformation. (3) Beyond policy support, establishing more sustainable incentive mechanisms to promote continuous green transformation among enterprises is crucial. This includes diversified policy tools such as fiscal incentives and tax benefits. Through further model optimization and validation with practical cases, the conclusions of this study can provide valuable decision-making references for both government and enterprises, promoting the sustainable development of green transformation.
Data availability statement
The original contributions presented in the study are included in the article/supplementary material, further inquiries can be directed to the corresponding author.
Author contributions
JL: Data curation, Investigation, Methodology, Writing – original draft. WF: Conceptualization, Formal analysis, Writing – original draft, Writing – review & editing.
Funding
The author(s) declare that no financial support was received for the research and/or publication of this article.
Conflict of interest
The authors declare that the research was conducted in the absence of any commercial or financial relationships that could be construed as a potential conflict of interest.
Generative AI statement
The authors declare that no Gen AI was used in the creation of this manuscript.
Publisher’s note
All claims expressed in this article are solely those of the authors and do not necessarily represent those of their affiliated organizations, or those of the publisher, the editors and the reviewers. Any product that may be evaluated in this article, or claim that may be made by its manufacturer, is not guaranteed or endorsed by the publisher.
References
Cai, L., Liu, Q., Zhu, X., and Deng, S. (2015). Market orientation and technological innovation: the moderating role of entrepreneurial support policies. Int. Entrep. Manag. J. 11, 645–671. doi: 10.1007/s11365-013-0290-3
Cui, F., Han, C., Liu, P., and Teng, M. (2022). Green credit of China's coal power enterprises during Green transformation: a tripartite evolutionary game analysis. Energies 15:5911. doi: 10.3390/en15165911
Fan, K., and Hui, E. C. M. (2020). Evolutionary game theory analysis for understanding the decision-making mechanisms of governments and developers on green building incentives. Build. Environ. 179:106972. doi: 10.1016/j.buildenv.2020.106972
Fan, W., Wu, X., and He, Q. (2024). Digitalization drives green transformation of supply chains: a two-stage evolutionary game analysis. Ann. Oper. Res. 1–20. doi: 10.1007/s10479-024-06050-0
Guo, S., Feng, W., Zhang, G., and Wen, Y. (2024). Evolutionary game analysis of government–Enterprise collaboration in coping with Natech risks. System 12:275. doi: 10.3390/systems12080275
Guo, B., Hu, P., and Lin, J. (2024). The effect of digital infrastructure development on enterprise green transformation. Int. Rev. Financ. Anal. 92:103085. doi: 10.1016/j.irfa.2024.103085
Han, J., Sun, R., Zeeshan, M., Rehman, A., and Ullah, I. (2023). The impact of digital transformation on green total factor productivity of heavily polluting enterprises. Front. Psychol. 14:1265391. doi: 10.3389/fpsyg.2023.1265391
Hou, J., Teo, T. S. H., Zhou, F., Lim, M. K., and Chen, H. (2018). Does industrial green transformation successfully facilitate a decrease in carbon intensity in China? An environmental regulation perspective. J. Clean. Prod. 184, 1060–1071. doi: 10.1016/j.jclepro.2018.02.311
Ji, P., Ma, X., and Li, G. (2015). Developing green purchasing relationships for the manufacturing industry: an evolutionary game theory perspective. Int. J. Prod. Econ. 166, 155–162. doi: 10.1016/j.ijpe.2014.10.009
Jiang, Z., Lyu, P., Ye, L., and Zhou, Y. W. (2020). Green innovation transformation, economic sustainability and energy consumption during China's new normal stage. J. Clean. Prod. 273:123044. doi: 10.1016/j.jclepro.2020.123044
Kvale, S., and Brinkmann, S. (2009). InterViews: Learning the craft of qualitative research interviewing. 2nd Edn. Los angeles: Sage Publications, Inc.
Lal, R. (2004). Soil carbon sequestration impacts on global climate change and food security. Science 304, 1623–1627. doi: 10.1126/science.1097396
Li, D. (2009). Fiscal and tax policy support for energy efficiency retrofit for existing residential buildings in China's northern heating region. Energy Policy 37, 2113–2118. doi: 10.1016/j.enpol.2008.11.036
Li, L., Yi, Z., Jiang, F., Zhang, S., and Zhou, J. (2023). Exploring the mechanism of digital transformation empowering green innovation in construction enterprises. Develop. Built Environ. 15:100199. doi: 10.1016/j.dibe.2023.100199
Liang, P., Sun, X., and Qi, L. (2023). Does artificial intelligence technology enhance green transformation of enterprises: based on green innovation perspective. Environ. Dev. Sustain. 26, 21651–21687. doi: 10.1007/s10668-023-04225-6
Lin, J., Huang, Y., and Li, M. (2023). Enhancing Green purchase intentions: the effects of product transformation salience and consumer traceability knowledge. Sustain. For. 15:12612. doi: 10.3390/su151612612
Liu, Z., Huang, Y.-Q., Shang, W.-L., Zhao, Y.-J., Yang, Z.-L., and Zhao, Z. (2022a). Precooling energy and carbon emission reduction technology investment model in a fresh food cold chain based on a differential game. Appl. Energy 326:119945. doi: 10.1016/j.apenergy.2022.119945
Liu, Z., Qian, Q., Hu, B., Shang, W.-L., Li, L., Zhao, Y., et al. (2022b). Government regulation to promote coordinated emission reduction among enterprises in the green supply chain based on evolutionary game analysis. Resour. Conserv. Recycl. 182:106290. doi: 10.1016/j.resconrec.2022.106290
Ma, Q., Khan, Z., Tariq, M., Isik, H., and Rjoub, H. (2022). Sustainable digital economy and trade adjusted carbon emissions: evidence from China's provincial data. Econ. Res.-Ekonomska Istrazivanja 35, 5469–5485. doi: 10.1080/1331677X.2022.2028179
Ma, R., and Lin, B. (2023). Digital infrastructure construction drives green economic transformation: evidence from Chinese cities. Human. Soc. Sci. Commun. 10, 1–10. doi: 10.1057/s41599-023-01839-z
MacBean, A. (2007). China's environment: problems and policies. World Econ. 30, 292–307. doi: 10.1111/j.1467-9701.2007.00883.x
McNamara, J. M. (2013). Towards a richer evolutionary game theory. J. R. Soc. Interface 10:20130544. doi: 10.1098/rsif.2013.0544
Owen, R., Brennan, G., and Lyon, F. (2018). Enabling investment for the transition to a low carbon economy: government policy to finance early stage green innovation. Curr. Opin. Environ. Sustain. 31, 137–145. doi: 10.1016/j.cosust.2018.03.004
Parmesan, C., and Yohe, G. (2003). A globally coherent fingerprint of climate change impacts across natural systems. Nature 421, 37–42. doi: 10.1038/nature01286
Patz, J. A., Campbell-Lendrum, D., Holloway, T., and Foley, J. A. (2005). Impact of regional climate change on human health. Nature 438, 310–317. doi: 10.1038/nature04188
Shang, W.-L., Chen, Y., Yu, Q., Song, X., Chen, Y., Ma, X., et al. (2023). Spatio-temporal analysis of carbon footprints for urban public transport systems based on smart card data. Appl. Energy 352:121859. doi: 10.1016/j.apenergy.2023.121859
Shen, Y., Fu, Y., and Song, M. (2023). Does digital transformation make enterprises greener? Evidence from China. Econ. Analysis and Policy 80, 1642–1654. doi: 10.1016/j.eap.2023.11.006
Shi, X., Wang, X., and Chen, P. (2021). A network-based approach for analyzing industrial green transformation: a case study of Beijing, China. J. Clean. Prod. 317:128281. doi: 10.1016/j.jclepro.2021.128281
Sun, J., Qi, B., Wang, J., and Nie, Y. (2024). Government guidance funds and green transformation of enterprises. Appl. Econ. Lett., 1–5. doi: 10.1080/13504851.2024.2332544
Suter, G. (2022). Climate change 2022: impacts, adaptation and vulnerability. Integr. Environ. Assess. Manag. 18, 1117–1118.
Tang, P., Jiang, Q., and Mi, L. (2021). One-vote veto: the threshold effect of environmental pollution in China?S economic promotion tournament. Ecol. Econ. 185:107069. doi: 10.1016/j.ecolecon.2021.107069
Vörösmarty, C. J., Green, P., Salisbury, J., and Lammers, R. B. (2000). Global water resources: vulnerability from climate change and population growth. Science 289, 284–288. doi: 10.1126/science.289.5477.284
Wang, Y., Guo, C.-H., Chen, X.-J., Jia, L.-Q., Guo, X.-N., Chen, R.-S., et al. (2021). Carbon peak and carbon neutrality in China: goals, implementation path and prospects. China Geol. 4, 1–27. doi: 10.31035/cg2021083
Wen, Z., and Chen, J. (2008). A cost-benefit analysis for the economic growth in China. Ecol. Econ. 65, 356–366. doi: 10.1016/j.ecolecon.2007.07.007
Wu, Y., Hu, J., Irfan, M., and Hu, M. (2024). Vertical decentralization, environmental regulation, and enterprise pollution: an evolutionary game analysis. J. Environ. Manag. 349:119449. doi: 10.1016/j.jenvman.2023.119449
Xu, Z. M., Cheng, G. D., Chen, D. J., and Templet, P. H. (2002). Economic diversity, development capacity and sustainable development of China. Ecol. Econ. 40, 369–378. doi: 10.1016/S0921-8009(02)00005-8
Xu, C., Sun, G., and Kong, T. (2024). The impact of digital transformation on enterprise green innovation. Int. Rev. Econ. Financ. 90, 1–12. doi: 10.1016/j.iref.2023.11.001
Yang, L., Zhan, J., Shang, W.-L., Fang, S., Wu, G., Zhao, X., et al. (2023). Multi-lane coordinated control strategy of connected and automated vehicles for on-ramp merging area based on cooperative game. IEEE Trans. Intell. Transp. Syst. 24, 13448–13461. doi: 10.1109/TITS.2023.3275055
Zhang, S., Lai, Y., Li, H., Wang, S., and Ru, X. (2024). Does digital transformation drive enterprise green investment? Evidence from Chinese listed firms. J. Environ. Plan. Manag., 1–34. doi: 10.1080/09640568.2024.2362267
Zhang, T., Shi, Z.-Z., Shi, Y.-R., and Chen, N.-J. (2022). Enterprise digital transformation and production efficiency: mechanism analysis and empirical research. Econ. Res.-Ekonomska Istrazivanja 35, 2781–2792. doi: 10.1080/1331677X.2021.1980731
Zhao, J., Taghizadeh-Hesary, F., Dong, K., and Dong, X. (2022). How green growth affects carbon emissions in China: the role of green finance. Econ. Res.-Ekonomska Istrazivanja 36, 2090–2111. doi: 10.1080/1331677X.2022.2095522
Keywords: climate change, enterprises, green transformation, evolutionary game theory, climate action
Citation: Luo J and Feng W (2025) Promoting green transformation of enterprises in China to adapt to climate change: an evolutionary game analysis. Front. Clim. 7:1504615. doi: 10.3389/fclim.2025.1504615
Edited by:
Maria Josefina Figueroa, Copenhagen Business School, DenmarkReviewed by:
Wen-Long Shang, Imperial College London, United KingdomKennedy Liti Mbeva, University of Oxford, United Kingdom
Copyright © 2025 Luo and Feng. This is an open-access article distributed under the terms of the Creative Commons Attribution License (CC BY). The use, distribution or reproduction in other forums is permitted, provided the original author(s) and the copyright owner(s) are credited and that the original publication in this journal is cited, in accordance with accepted academic practice. No use, distribution or reproduction is permitted which does not comply with these terms.
*Correspondence: Wei Feng, 210201017@csu.edu.cn