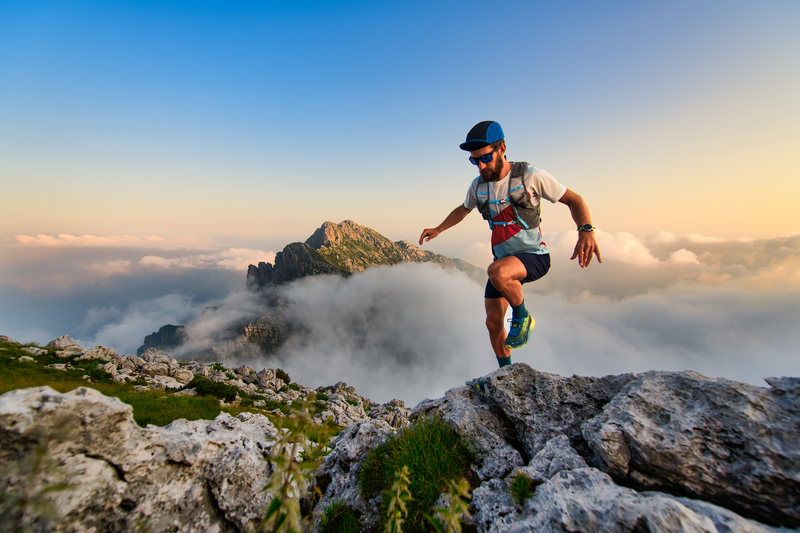
95% of researchers rate our articles as excellent or good
Learn more about the work of our research integrity team to safeguard the quality of each article we publish.
Find out more
ORIGINAL RESEARCH article
Front. Chem. , 18 March 2025
Sec. Theoretical and Computational Chemistry
Volume 13 - 2025 | https://doi.org/10.3389/fchem.2025.1543588
This article is part of the Research Topic Design of Extended Networks for Tuning Functionality of Materials View all 3 articles
Zeolites are extremely massive mineral crystals with complex frameworks, composed of internal porous structures with channels and cages. Open-framework aluminophosphates (AlPOs) are a significant class of inorganic crystalline compounds known for their diverse properties. Our study focuses on the topological aspects of zeolite frameworks using graph theoretical techniques, providing insights into computational chemistry. In this article, we compute various degree-based topological indices, information entropy, and spectral entropies of zeolite AWW using the bond partitioning approach to assess the complexity of the framework. Additionally, we present the HOMO–LUMO gap measures to evaluate the global chemical descriptors using the eigenvalues of the adjacency matrix of zeolite structures. Furthermore, we developed exponential and polynomial regression models using the obtained information entropy and spectral values to predict various potential energies of the framework. Based on the outcomes of the study, we infer that the information entropies and spectral value have a significant relationship with the potential energies.
Zeolites are minerals with crystalline structures that have regular frameworks constructed by channels and pores an the molecular level. Zeolite catalysts are employed throughout gasoline production and in the fields of adsorption, ion exchange, heterogeneous catalysis, sensors, and medicine. These crystals are also frequently used in solar energy conversion (Mihaela and Ildiko, 2012). Zeolite frameworks have Si tetrahedral nodes (T-atoms) and T-O-T, a bond referring to the arrangement where T stands for a tetrahedrally coordinated atom, usually silicon (Si) or aluminum (Al), and O stands for oxygen. The oxygen atom acts as a bridge between two tetrahedrally coordinated sites. Replacing Si with Al or other tetrahedral atoms significantly alters the characteristics of zeolites. This changes the framework’s affinity to other cations, allowing for customization of ion-exchange characteristics and adsorption surfaces. The tetrahedral atoms’ three-dimensional geometry forms rings, cages, channels, and pores, resulting in different frameworks (Kapko et al., 2010; Liebau, 1983; Barrer, 1979). Because there were many distinct materials, some with a common framework but differing by the chemical proposition, we needed to categorize all the different crystalline aluminosilicate materials. As a result, the concept of structural variation came into existence (Liebau, 1983; Barrer, 1979).
The aluminophosphate crystalline sieves, denoted as
A chemical composition graph is used to demonstrate the basic structure of a chemical compound (Balaban, 1985; Sato, 1991; Chandler, 2019). The vertices represent each of the atoms of the compound, and the edges indicate the bonds that occur among them. A topological index is used to measure the relationship between a chemical compound’s structure and various physical, chemical, or biological characteristics. Degree-based descriptors are extensively used topological descriptors with applications in computational chemistry, whereas topological indices based on neighborhood degree sum are capable of accurately determining most physicochemical parameters (Gutman and Tošović, 2013; Mondal et al., 2019; Ramane et al., 2021; Ullah et al., 2024; Mondal et al., 2021).
Topological descriptors for zeolites were developed to enhance machine learning, optimization, and algorithmic techniques (Krivovichev, 2013; Arockiaraj et al., 2022a; Arockiaraj et al., 2022b; Arockiaraj et al., 2021; Jacob et al., 2023). To better understand the basis for relating the structure and property to the synthesis technique, experimental and computational methods have been closely linked in modern zeolite synthesis. The zeolite framework influences its physicochemical properties such as adsorption, phase transformation, complexity, and chirality of molecules. Recent studies indicate that the topological index quantifies the relationship between zeolite molecular structures and a wide range of physicochemical characteristics (Jacob et al., 2023; Peter and Clement, 2023; Jacob and Clement, 2024; Peter and Clement, 2024; Prabhu et al., 2020).
The complexity of networks can be evaluated using statistical information measures (Bonchev, 1983; Anand and Bianconi, 2009; Mowshowitz, 1968a). Shannon’s information theory was used to develop the universal quantitative measurements of structural and chemical complexity, which are helpful for the investigation of several mineralogical, crystallographic, and chemical processes (Jacob et al., 2023; Dehmer and Mowshowitz, 2011; Krivovichev, 2012; Shannon, 1948). The probability of electrons in molecules and the chemical bonds between molecules have been effectively studied using information theory concepts (Nalewajski, 2006; Nalewajski, 2014). The communication theory for the chemical connection employs the basic information entropy of molecular systems in the atoms-in molecules, orbital, or local levels to ascertain electron probability distributions (Nalewajski, 2006; Nalewajski, 2014; Nalewajski, 2011). The information entropy
The model obtained good accuracy and provided information about the connection between the zeolite framework structure and their mechanical stability (Evans and Coudert, 2017). The connections between zeolite frameworks and their characteristics are complicated, making an in-depth experimental investigation for novel zeolites necessary (Burtch et al., 2014). Various density functional theory computational techniques for determining zeolite characteristics have been established (Ranjan et al., 2023; Román-Román and Zicovich-Wilson, 2015; Fischer and Angel, 2017; Balasubramanian, 2023a; Balasubramanian, 2023b). Determining the zeolite lattice energy linked to formation enthalpy can help understand zeolite stability and structure, although computations are expensive but accurate (Román-Román and Zicovich-Wilson, 2015; Fischer and Angel, 2017; Stacey et al., 2023). Machine learning techniques can help determine the characteristics of zeolites by learning from a vast collection of known zeolites and their characteristics. It was demonstrated that some structural descriptors are suitable to characterize the lattice energy of zeolites in a comprehensible manner using a linearized equation, focusing on the intricate connection between structural features (Jacob et al., 2023; Peter and Clement, 2023; Jacob and Clement, 2024; Balestra et al., 2024). Electronic structural calculations help us understand how zeolite structures influence their performance. Long-range and short-range interaction energies significantly affect the geometry of the zeolite framework and their stability of transition states and adsorption behavior. Notably, long-range zeolite electrostatic interactions play a crucial role in describing transition-state structures and in predicting experimentally determined activation energies (Ranjan et al., 2023; Román-Román and Zicovich-Wilson, 2015; Fischer and Angel, 2017; Mansoor et al., 2018).
This article focuses on developing generalized expressions for descriptors such as degree-based and degree-sum-based measures specifically for the AWW zeolite structure, with any arbitrary dimension used to obtain structural data. A detailed analysis of bond information and spectral entropies is presented to evaluate the complexity of the AWW zeolite. Furthermore, we incorporated the exponential regression models that establish relationships between the measured entropies and molecular interaction energies, offering deeper insights into the energy and structural characteristics of zeolite. Global chemical reactivity descriptors and the spectral properties are also calculated using the eigen values of AWW zeolite structures.
Let
For
where
The molecular topological descriptors based on the degree and degree sum are obtained via the atomic valences of the framework’s chemical bonds. Various degree-type topological indices have been developed and employed extensively in molecular modeling of a wide range of zeolite framework characteristics and activities (Arockiaraj et al., 2022a; Jacob et al., 2023; Zhang et al., 2024; Peter and Clement, 2023). Self-powered multiplicative topological indices of chemical structure are derived from the product of edge functions associated with the degrees of end vertices of an edge (Kavitha et al., 2021). These indices have been developed using exponential vertex degree-based descriptors (Rada, 2019). Therefore, one can develop a self-powered multiplicative version of degree and degree sum-based topological indices using the following general form:
The edge partition technique is applied to derive the various degree and degree sum-based molecular topological indices. The sizes of the partitions on the basis of end vertex degrees are denoted by
•
•
By considering
The studies (Krivovichev, 2013; Krivovichev, 2012; Kaußler and Kieslich, 2021; Krivovichev, 2016) used the following equation to determine the quantity of structural Shannon’s information of the crystal structure:
where
Now, Equation 7 will be modified to characterize the structural characteristics of the zeolite framework. The bonds of the zeolite framework are taken into account, and each of them receives a probability value based on the topological indices. The topological information entropy measures for degree type are defined using that molecular topological index
where
The information entropy measure based on the degree sum molecular topological descriptors is obtained by replacing
By reformulating Equation 8 using self-powered multiplicative degree-based indices, as described in (Kavitha et al., 2021), we could establish a relationship between the self-powered multiplicative indices and the entropy computation typically associated with bond additive indices.
The developed Equations 8, 9 are used to measure the various bond information entropy values of AWW zeolite. Furthermore, the measured information entropy was used to develop the possibility of machine learning of the AWW zeolite structure for studying the properties and activities (Arockiaraj et al., 2022a; Peter and Clement, 2023; Jacob and Clement, 2024; Peter and Clement, 2024).
To define the spectral entropies for each edge
where
The study of graph energy obtained from the topological matrix indices offers insights into the structural properties of graphs, with potential applications in chemistry and communication networks (Bozkurt et al., 2010; Ghanbari, 2022; Rodríguez and Sigarreta, 2016; Chen et al., 2014; Hayat et al., 2024; Hui et al., 2022; Kosar et al., 2023). The novelty of this section is that we use the computed eigen values of the topological matrix indices of the zeolite framework to measure the spectral information entropies. Let
where the ratio
The AWW zeolite structures are characterized by using the measurements provided in Section 2. As depicted in Figure 2, the cavities serve as the primary building component for the zeolite AWW molecular communication system, which has 32 vertices and 48 edges connected by eight squares and two octagons, such that some bonds are shared. In order to form a three-dimensional molecular structure, the cavities of AWW are arranged in the
Figure 3 depicts the polyhedral structural growth of AWW. Figure 2 shows the primary cavities that are interconnected to generate the
We employed the edge partition technique according to the degrees of the end atoms of bonds, and the degree sum of the end atoms of bonds used to generate different degree-based molecular topological descriptors of zeolite
Bond degree partitions:
•
•
•
•
Bond degree-sum partitions (when
•
•
•
•
•
•
•
•
•
•
•
•
•
Now let us obtain the molecular topological indices using Equation 1, and the estimated bond degree and bond degree sum partition are obtained from the above-listed partitions. The results of the zeolite
Since the results from Theorem 3.2 are applicable for
Bond degree-sum partitions (when
•
•
•
•
•
•
•
•
By using the same procedure and the above-listed partitions, we can calculate the neighborhood degree sum-based indices of two-dimensional zeolite AWW.
Theorem 3.1. Let
1.
2.
3.
4.
5.
6.
7.
8.
9.
10.
11.
12.
13.
Theorem 3.2. Let
1.
2.
3.
4.
5.
6.
7.
8.
9.
10.
11.
12.
13.
14.
For computing the degree and degree sum-type multiplicative self-powered molecular descriptors of the AWW zeolite, Equations 5, 6 were employed to generate the corresponding indices using the listed bond degree and bond degree sum partitions. For instance, the analytical expression for degree-based self-powered sum connectivity index is
Furthermore, the information entropy measures multiplicative self-powered for bond additive degree, and bond additive degree sum topological indices are easily obtained using Equation 9.
This section summarizes the information entropy of AWW zeolite and suggests possible development using the degree, degree sum-based information, and spectral entropies of Equations 8, 11 by assessing the mineral complexity given in Tables 1, 2. It can be very useful for selected dimensions for training in an attempt to analyze the information entropies for larger complex molecules. For all entropy measures, the
The characteristics of the AWW zeolite structure can be studied using the data provided in Table 1. This allows for the investigation of atomic chemical properties, structural complexity, diversity, similarity, modularity, chirality, and molecular structure information in crystal systems. In addition, in this study, degree spectral entropies were measured to assess the structural complexity of zeolite frameworks. The spectral degree-based entropies have the lowest discriminative values compared to degree and degree sum-based information entropies. This implies that spectral entropy focuses on specific modes or energy distributions within the structure rather than the overall disorder. The zero eigenvalue of the zeolite adjacency matrix is indeterminate in the spectral information entropy calculations from Equation 11 as it causes issues in logarithmic terms. To ensure accuracy, the zero eigenvalue must be excluded or addressed through normalization techniques.
Section 4.2 will address the prediction abilities of our measured bond information and spectral entropies obtained from the AWW zeolite structure.
Density functional theory (DFT) has limitations in calculating the molecular interaction energies for large AWW zeolite systems due to its high computational costs, difficulty in capturing long-range interactions, and challenges in modeling non-covalent interactions and strongly correlated electron systems. It also struggles with the flexibility of zeolite frameworks and dynamic effects (Cohen et al., 2012). These issues make DFT impractical for application in large zeolite systems, underscoring the need for predictive models like machine learning to efficiently estimate interaction energies, so there is another computational method to calculate the molecular energies of zeolites: force field method. Force-field methods, in contrast, offer an efficient, cost-effective alternative for studying zeolite structures. These methods allow for scalable simulations of large, complex frameworks and can provide insights into time-dependent behaviors such as adsorption, diffusion, and framework flexibility. Although they rely on parameterized potentials and may struggle with modeling larger zeolite structures, force-field methods, when tailored to specific systems, complement experimental data and fill gaps in case of limited experiments, thus playing a crucial role in advancing our understanding of zeolite properties (Dubbeldam et al., 2019; Jaramillo and Auerbach, 1999).
Determining the properties of zeolites experimentally is challenging due to their structural complexity, which includes intricate pore networks, large unit cells, and diverse atomic environments. These challenges are further compounded by the limited crystallinity, structural defects, and difficulties in synthesizing high-quality samples, all of which impede accurate measurements (Price et al., 2021). The unique crystal structure of the AWW zeolite, characterized by intricate cages and novel configurations, exemplifies this complexity and contributes to the limited availability of experimental data. Additionally, zeolite properties are highly sensitive to environmental factors such as temperature and pressure, complicating the reproducibility. Advanced techniques like X-ray diffraction, neutron diffraction, and nuclear magnetic resonance add to the difficulty, given their high costs and time requirements (Van Vreeswijk and Weckhuysen, 2022).
This subsection investigates the entropies of zeolite AWW using both the bond additive and bond additive sum indices. The results are then correlated with the physical properties of zeolites. For force-field calculations, the Molecular Mechanics 3 (MM3) and Universal Force Field (UFF) approaches are employed within the CRYSTAL computing tools. These tools are essential for analyzing the zeolite’s structural and energetic properties, enabling accurate calculation of molecular interaction energies such as
A detailed exponential fit analysis was performed between the properties provided in Table 3 and the information entropy values to determine the possible molecular energies and construct models for prediction. The AWW zeolite’s bond degree, degree sum, and spectral information entropy are taken into account for developing the following exponential fit prediction model:
where
The exponential model is chosen for it accurately captures the rapid, non-linear growth of entropy as molecular interaction energies increase in large zeolite frameworks. Unlike polynomial models, the exponential model reflects the exponential increase in the complexity and entropy, providing a more accurate and meaningful representation of their relationship, particularly in complex systems where small energy changes lead to significant increases in entropy.
Table 4 presents the significant coefficients for exponential fit of entropies with molecular energies. The proposed model obtained from Table 4 shows that
Figure 4. Exponential fitting of bond and spectral information entropy with properties of AWW zeolite.
Table 5 presents a comparison between properties and predicted molecular interaction energies for AWW zeolites. The table also highlights consistent trends across different zeolite frameworks, particularly for larger structures, demonstrating the robustness of entropy-based models in capturing complex molecular interactions and effectively predicting energy properties.
Table 5. Comparison of molecular interaction energy values for AWW zeolite using the force-field method and predictive model
Assessing global chemical reactivity descriptors such as ionization potential (IP), electron affinity (EA), hardness
In this section, we propose the measurement of the global chemical reactivity descriptors using the eigen values of the zeolite graph as the HOMO–LUMO gap. Therefore, we employ the graph-theoretic approach to compute the HOMO–LUMO gap, which involves modeling the zeolite structure as a graph, constructing adjacency, and calculating the eigenvalues of the zeolite matrix. The difference between the maximum negative and minimum positive eigenvalues of the characteristic polynomial gives the HOMO–LUMO gap, which is a key indicator of the molecule’s electronic properties and reactivity (Arockiaraj et al., 2022a; Fowler and Pisański, 2010; Dias and Guirgis, 2002; Aihara, 1999; Bacalis and Zdetsis, 2009; Mushtaq et al., 2022; Ghosh, 2019).
The HOMO–LUMO gap in the zeolite structure reflects their chemical reactivity. A larger HOMO–LUMO gap typically indicates greater stability and lower chemical reactivity as it requires more energy to excite an electron from the HOMO to the LUMO. Conversely, a smaller gap implies higher chemical reactivity as less energy is needed for electronic transitions, facilitating interactions with adsorbates or reactants (Yin et al., 2021). Furthermore, the spectral properties (SP) of chemical structure such as spectral diameter (SD), graph-energy (GE), and spectral entropy (SGE) (Arockiaraj et al., 2022a; Balasubramanian, 2023b; Balasubramanian, 2023c) are computed using the eigen values of characteristic polynomials for the different AWW zeolites listed in Table 6. The global chemical reactivity descriptors are calculated using Equations 13–18, and the summarized values in Table 6 are measured using Python code. One can observe from Table 6 that as the AWW zeolite structure increases, the global descriptors such as hardness, chemical potential, and electrophilicity index decrease. On the other hand, the softness of the AWW zeolite structure is increasing.
Table 7 presents molecular energy properties of various AWW zeolite structures, serving as a benchmark to validate predictive models developed using the measured spectral values of AWW zeolite. It establishes a critical link between computed molecular energies and spectral values, demonstrating the reliability of theoretical calculations in predicting energy properties. The table also highlights how structural variations in zeolites influence energy behavior, emphasizing the practical utility of spectral and entropy-based analyses in evaluating the stability and reactivity.
The data in Table 8 summarize the significant coefficients of the developed polynomial fit Equation 19. By using the molecular energies and spectral values of zeolite AWW listed in Tables 6, 7, the models demonstrate their effectiveness in predicting potential energies, as evidenced by the high
where
Table 8. Significant coefficients for polynomial fit of spectral properties with molecular energies.
Figure 5 highlights the polynomial fitting of spectral values with AWW zeolite properties, demonstrating a strong correlation between spectral entropy and molecular characteristics. The model shows high accuracy, validating the use of spectral properties for predicting molecular energies.
The obtained data, such as the HOMO–LUMO gap and spectral information entropy, directly relate to the applications of AWW zeolites. A smaller HOMO–LUMO gap suggests higher reactivity, enhancing catalytic activity, while spectral information entropy reflects the structural complexity, influencing adsorption capacity through pore connectivity and active site diversity. These comparisons contextualize the findings, linking structural properties to their functional performance in industrial applications.
Predicting the characteristics of complicated multicomponent zeolite materials and extremely high-molecular weight systems of molecules is an essential step in the development of QSPR advances in technology. We have developed the degree-sum-based molecular descriptors for zeolite AWW, analyzing three information entropies obtained from topological indices and their spectral aspects. Furthermore, we proposed the exponential regression and polynomial models to predict its potential energies using the computed information measures. The eigenvalue difference approximation of the HOMO–LUMO gap reduces computational demands compared to more complex DFT calculations, enabling faster reactivity predictions in large systems or high-throughput screening. The results of the present investigation illustrate the usefulness and efficacy of the quantitative structure–property relationship approach for the prediction of an extensive variety of properties of the zeolite material.
The raw data supporting the conclusions of this article will be made available by the authors, without undue reservation.
PP: investigation, methodology, software, validation, and writing–original draft. JC: conceptualization, formal analysis, resources, supervision, validation, and writing–review and editing. MA: formal analysis, supervision, validation, and writing–review and editing. KJ: formal analysis, investigation, methodology, visualization, and writing–original draft.
The author(s) declare that no financial support was received for the research, authorship, and/or publication of this article.
The authors declare that the research was conducted in the absence of any commercial or financial relationships that could be construed as a potential conflict of interest.
The author(s) declared that they were an editorial board member of Frontiers, at the time of submission. This had no impact on the peer review process and the final decision.
The author(s) declare that no Generative AI was used in the creation of this manuscript.
All claims expressed in this article are solely those of the authors and do not necessarily represent those of their affiliated organizations, or those of the publisher, the editors and the reviewers. Any product that may be evaluated in this article, or claim that may be made by its manufacturer, is not guaranteed or endorsed by the publisher.
Abraham, J., Arockiaraj, M., Jency, J., Kavitha, S. R. J., and Balasubramanian, K. (2022). Graph entropies, enumeration of circuits, walks and topological properties of three classes of isoreticular metal organic frameworks. J. Math. Chem. 60 (4), 695–732. doi:10.1007/s10910-021-01321-8
Aihara, J. I. (1999). Weighted HOMO-LUMO energy separation as an index of kinetic stability for fullerenes. Theor. Chem. Acta 102, 134–138. doi:10.1007/s002140050483
Anand, K., and Bianconi, G. (2009). Entropy measures for networks: toward an information theory of complex topologies. Phys. Rev. E. 80, 045102. doi:10.1103/physreve.80.045102
Arockiaraj, M., Clement, J., Paul, D., and Balasubramanian, K. (2021). Quantitative structural descriptors of sodalite materials. J. Mol. Struct. 1223, 128766. doi:10.1016/j.molstruc.2020.128766
Arockiaraj, M., Paul, D., Klavžar, S., Clement, J., Tigga, S., and Balasubramanian, K. (2022a). Relativistic topological and spectral characteristics of zeolite SAS structures. J. Mol. Struct. 1270, 133854. doi:10.1016/j.molstruc.2022.133854
Arockiaraj, M., Paul, D., Klavžar, S., Clement, J., Tigga, S., and Balasubramanian, K. (2022b). Relativistic distance based and bond additive topological descriptors of zeolite RHO materials. J. Mol. Struct. 1250, 131798. doi:10.1016/j.molstruc.2021.131798
Bacalis, N. C., and Zdetsis, A. D. (2009). Properties of hydrogen terminated silicon nanocrystals via a transferable tight-binding Hamiltonian, based on ab-initio results. J. Math. Chem. 46, 962–970. doi:10.1007/s10910-009-9557-x
Balaban, A. T. (1985). Applications of graph theory in chemistry. J. Chem. Inf. Comput. 25 (3), 334–343. doi:10.1021/ci00047a033
Balasubramanian, K. (2023a). Density functional and graph theory computations of vibrational, electronic, and topological properties of porous nanographenes. J. Phys. Org. Chem. 36 (12), e4435. doi:10.1002/poc.4435
Balasubramanian, K. (2023b). Topological indices, graph spectra, entropies, Laplacians, and matching polynomials of n-dimensional hypercubes. Symmetry 15 (2), 557. doi:10.3390/sym15020557
Balasubramanian, K. (2023c). Characteristic polynomials, spectral-based Riemann-Zeta functions and entropy indices of n-dimensional hypercubes. J. Math. Chem. 61 (7), 1570–1591. doi:10.1007/s10910-023-01479-3
Balestra, S. R., Rodríguez-Sánchez, N., Mena-Torres, D., and Ruiz-Salvador, A. R. (2024). Structural features and zeolite stability: a linearized equation approach. Cryst. Growth Des. 24 (3), 938–946. doi:10.1021/acs.cgd.3c00893
Barrer, R. M. (1979). Chemical nomenclature and formulation of compositions of synthetic and natural zeolites. Pure Appl. Chem. 51 (5), 1091–1100. doi:10.1351/pac197951051091
Bonchev, D. (1983). Information theoretic indices for characterization of chemical structures. Chichester: Research Studies Press.
Bozkurt, S. B., Güngör, A. D., Gutman, I., and Cevik, A. S. (2010). Randić matrix and Randić energy. MATCH Commun. Math. Comput. Chem. 64 (1), 239–250.
Burtch, N. C., Jasuja, H., and Walton, K. S. (2014). Water stability and adsorption in metal–organic frameworks. Chem. Rev. 114 (20), 10575–10612. doi:10.1021/cr5002589
Chandler, D. L. (2019). A new mathematical approach to understanding zeolites. Cambridge, MA: MIT News Office. Available at: https://www.materialstoday.com/computation-theory/news/graph-theory-transformative-discovery-zeolites.
Chen, Z., Dehmer, M., and Shi, Y. (2014). A note on distance-based graph entropies. Entropy 16 (10), 5416–5427. doi:10.3390/e16105416
Cohen, A. J., Mori-Sánchez, P., and Yang, W. (2012). Challenges for density functional theory. Chem. Rev. 112 (1), 289–320. doi:10.1021/cr200107z
Dehmer, M. (2008). Information processing in complex networks: graph entropy and information functionals. Appl. Math. Comput. 201, 82–94. doi:10.1016/j.amc.2007.12.010
Dehmer, M., and Mowshowitz, A. (2011). A history of graph entropy measures. Inf. Sci. 181, 57–78. doi:10.1016/j.ins.2010.08.041
Dias, J. R., and Guirgis, G. A. (2002). π-molecular orbitals of conjugated linear polyene molecules as molecular orbital functional groups in the design of near-infrared dyes. Croat. Chem. Acta. 75 (2), 621–632.
Dovesi, R., Orlando, R., Civalleri, B., Roetti, C., Saunders, V. R., and Zicovich-Wilson, C. M. (2005). CRYSTAL: a computational tool for the ab initio study of the electronic properties of crystals. Z. Krist. Cryst. Mater. 220 (5-6), 571–573. doi:10.1524/zkri.220.5.571.65065
Dubbeldam, D., Walton, K. S., Vlugt, T. J., and Calero, S. (2019). Design, parameterization, and implementation of atomic force fields for adsorption in nanoporous materials. Adv. Theory. Simul. 2 (11), 1900135. doi:10.1002/adts.201900135
Evans, J. D., and Coudert, F. X. (2017). Predicting the mechanical properties of zeolite frameworks by machine learning. Chem. Mater. 29 (18), 7833–7839. doi:10.1021/acs.chemmater.7b02532
Fischer, M., and Angel, R. J. (2017). Accurate structures and energetics of neutral-framework zeotypes from dispersion-corrected DFT calculations. J. Chem. Phys. 146 (17), 174111. doi:10.1063/1.4981528
Fowler, P. V., and Pisański, T. (2010). HOMO-LUMO maps for chemical graphs. MATCH. Commun. Math. Comput. Chem. 64, 373–390.
Gázquez, J. L. (1993). “Hardness and softness in density functional theory,” in Chemical hardness. Editor K. D. Sen (Berlin, Heidelberg: Springer Berlin Heidelberg), 27–43.
Ghanbari, N. (2022). On the Sombor characteristic polynomial and Sombor energy of a graph. Comput. Appl. Math. 41 (6), 242. doi:10.1007/s40314-022-01957-5
Ghosh, B. C. (2019). Approach to zero band gap graphene sheets: a graph theoretical analysis. J. Indian Chem. Soc. 96 (6), 693–698. doi:10.5281/zenodo.5647856
Gutman, I. (2013). Degree-based topological indices. Croat. Chem. Acta. 86 (4), 351–361. doi:10.5562/cca2294
Gutman, I., and Tošović, J. (2013). Testing the quality of molecular structure descriptors. Vertex-degree-based topological indices. J. Serbian Chem. Soc. 78, 805–810. doi:10.2298/jsc121002134g
Hayat, S., Mahadi, H., Alanazi, S. J., and Wang, S. (2024). Predictive potential of eigenvalues-based graphical indices for determining thermodynamic properties of polycyclic aromatic hydrocarbons with applications to polyacenes. Comput. Mater. Sci. 238, 112944. doi:10.1016/j.commatsci.2024.112944
Hemelsoet, K., Lesthaeghe, D., Van Speybroeck, V., and Waroquier, M. (2007). Global DFT-based reactivity indicators: an assessment of theoretical procedures in zeolite catalysis. J. Phys. Chem. C 111 (7), 3028–3037. doi:10.1021/jp0656227
Hui, Z. H., Kanwal, S., Aslam, A., Saeed, S., and Idrees, N. (2022). A mathematical investigation for appraisal of crystal structure lattices through eccentricity-based topological invariants, QSPR analysis, and MCDA. Eur. Phys. J. Plus. 137 (11), 1277. doi:10.1140/epjp/s13360-022-03495-9
Jacob, K., and Clement, J. (2024). Zeolite ATN: topological characterization and predictive analysis on potential energies using entropy measures. J. Mol. Struct. 1299, 137101. doi:10.1016/j.molstruc.2023.137101
Jacob, K., Clement, J., Arockiaraj, M., Paul, D., and Balasubramanian, K. (2023). Topological characterization and entropy measures of tetragonal zeolite merlinoites. J. Mol. Struct. 1277, 134786. doi:10.1016/j.molstruc.2022.134786
Jaramillo, E., and Auerbach, S. M. (1999). New force field for Na cations in faujasite-type zeolites. J. Phys. Chem. B 103 (44), 9589–9594. doi:10.1021/jp991387z
Kalaam, A. A., Greeni, A. B., and Arockiaraj, M. (2024). Modified reverse degree descriptors for combined topological and entropy characterizations of 2D metal organic frameworks: applications in graph energy prediction. Front. Chem. 12, 1470231. doi:10.3389/fchem.2024.1470231
Kapko, V., Dawson, C., Treacy, M. M. J., and Torpe, M. F. (2010). Flexibility of ideal zeolite frameworks. Phys. Chem. Chem. Phys. 12, 8531–8541. doi:10.1039/c003977b
Kaußler, C., and Kieslich, G. (2021). crystIT: complexity and configurational entropy of crystal structures via information theory. J. Appl. Crystallogr. 54 (1), 306–316. doi:10.1107/s1600576720016386
Kavitha, S. R. J., Abraham, J., Arockiaraj, M., Jency, J., and Balasubramanian, K. (2021). Topological characterization and graph entropies of tessellations of kekulene structures: existence of isentropic structures and applications to thermochemistry, nuclear magnetic resonance, and electron spin resonance. J. Phys. Chem. 125 (36), 8140–8158. doi:10.1021/acs.jpca.1c06264
Kazemi, R. (2016). Entropy of weighted graphs with the degree-based topological indices as weights. MATCH Commun. Math. Comput. Chem. 76, 69–80.
Kosar, Z., Zaman, S., and Siddiqui, M. K. (2023). Structural characterization and spectral properties of hexagonal phenylene chain network. Eur. Phys. J. Plus. 138 (5), 415. doi:10.1140/epjp/s13360-023-03997-0
Krivovichev, S. (2012). Topological complexity of crystal structures: quantitative approach. Acta Crystallogr. 68 (3), 393–398. doi:10.1107/s0108767312012044
Krivovichev, S. (2013). Structural complexity of minerals: information storage and processing in the mineral world. Mineral. Mag. 77, 275–326. doi:10.1180/minmag.2013.077.3.05
Krivovichev, S. V. (2016). Structural complexity and configurational entropy of crystals. Acta Crystallogr. Sect. B Struct. Sci. Cryst. Eng. Mater. 72 (2), 274–276. doi:10.1107/s205252061501906x
Liebau, F. (1983). Zeolites and clathrasils-two distinct classes of framework silicates. Zeolites 3, 191–193. doi:10.1016/0144-2449(83)90003-9
Manda, T., Barasa, G. O., Louis, H., Irfan, A., Agumba, J. O., Lugasi, S. O., et al. (2024). A data-guided approach for the evaluation of zeolites for hydrogen storage with the aid of molecular simulations. J. Mol. Model. 30 (2), 43. doi:10.1007/s00894-024-05837-z
Mansoor, E., Van der Mynsbrugge, J., Head-Gordon, M., and Bell, A. T. (2018). Impact of long-range electrostatic and dispersive interactions on theoretical predictions of adsorption and catalysis in zeolites. Catal. Today. 312, 51–65. doi:10.1016/j.cattod.2018.02.007
Mihaela, U., and Ildiko, A. (2012). Zeolite utilization in solar energy storage and heat exchange, Handbook of Natural Zeolites. Romania: Cyprus Open University, 369–398.
Mondal, S., De, N., and Pal, A. (2019). On some general neighborhood degree based topological indices. Int. J. Appl. Math. 32 (6), 1037. doi:10.12732/ijam.v32i6.10
Mondal, S., Dey, A., De, N., and Pal, A. (2021). QSPR analysis of some novel neighbourhood degree-based topological descriptors. Complex. Intell. Syst. 7, 977–996. doi:10.1007/s40747-020-00262-0
Mowshowitz, A. (1968a). Entropy and the complexity of graphs: I. An index of the relative complexity of a graph. Bull. Math. Biophys. 30, 175–204. doi:10.1007/bf02476948
Mowshowitz, A. (1968b). Entropy and the complexity of graphs II: the information content of digraphs and infinite graphs. Bull. Math. Biophys. 30, 225–240. doi:10.1007/bf02476692
Mushtaq, S., Arockiaraj, M., Fiona, J. C., Jency, J., and Balasubramanian, K. (2022). Topological properties, entropies, stabilities and spectra of armchair versus zigzag coronene-like nanoribbons. Mol. Phys. 120 (17), e2108518. doi:10.1080/00268976.2022.2108518
Nalewajski, R. F. (2006). Information theory of molecular systems. Amsterdam, Netherlands: Elsevier.
Nalewajski, R. F. (2011). Entropy/information descriptors of the chemical bond revisited. J. Math. Chem. 49, 2308–2329. doi:10.1007/s10910-011-9888-2
Nalewajski, R. F. (2014). On phase/current components of entropy/information descriptors of molecular states. Mol. Phys. 112 (19), 2587–2601. doi:10.1080/00268976.2014.897394
Padmanabhan, J., Parthasarathi, R., Subramanian, V., and Chattaraj, P. K. (2007). Electrophilicity-based charge transfer descriptor. J. Phys. Chem. A 111 (7), 1358–1361. doi:10.1021/jp0649549
Palmer, D. C. (2015). Visualization and analysis of crystal structures using CrystalMaker software. Z. Krist. Cryst. Mater. 230 (9–10), 559–572. doi:10.1515/zkri-2015-1869
Pearson, R. G. (1988). Chemical hardness and bond dissociation energies. J. Am. Chem. Soc. 110 (23), 7684–7690. doi:10.1021/ja00231a017
Peter, P., and Clement, J. (2023). Potential energy determination of NPT zeolite frameworks by information entropies. J. Mol. Struct. 1292, 136169. doi:10.1016/j.molstruc.2023.136169
Peter, P., and Clement, J. (2024). Predictive models on potential energies of zeolite ZK-5 using bond weighted information entropy measures. J. Mol. Struct. 1307, 137945. doi:10.1016/j.molstruc.2024.137945
Prabhu, S., Murugan, G., Cary, M., Arulperumjothi, M., and Liu, J. B. (2020). On certain distance and degree based topological indices of Zeolite LTA frameworks. Mater. Res. Express 7 (5), 055006. doi:10.1088/2053-1591/ab8b18
Price, L. A., Ridley, C. J., Bull, C. L., Wells, S. A., and Sartbaeva, A. (2021). Determining the structure of zeolite frameworks at high pressures. Cryst. Eng. Comm. 23 (33), 5615–5623. doi:10.1039/d1ce00142f
Rada, J. (2019). Exponential vertex–degree–based topological indices and discrimination, MATCH Commun. Math. Comput. Chem. 82 (1), 29–41.
Rahul, M. P., Clement, J., Junias, J. S., Arockiaraj, M., and Balasubramanian, K. (2022). Degree-based entropies of graphene, graphyne and graphdiyne using Shannon’s approach. J. Mol. Struct. 1260, 132797. doi:10.1016/j.molstruc.2022.132797
Ramane, H. S., Pisea, K. S., Jummannaverb, R. B., and Patila, D. D. (2021). Applications of neighbors degree sum of a vertex on Zagreb indices, MATCH Commun. Math. Comput. Chem. 85 (2), 329–348.
Ranjan, P., Balasubramanian, K., and Chakraborty, T. (2023). DFT investigation of structural and optoelectronic properties of glassy chalcogenide CuXY2 (X = Sb, Bi; Y = S, Se, Te) molecules. J. Phys. D. Appl. Phys. 56, 405306. doi:10.1088/1361-6463/ace11c
Rashevsky, N. (1960). Life, information theory, probability, and physics. Math. Biophys. 22, 351–364. doi:10.1007/bf02476719
Richardson, J. W., Pluth, J. J., and Smith, J. V. (1989). Microporous aluminophosphate AlPO 4-22: a framework topology with two new building units, and encapsulated phosphate. Sci. Nat. 76, 467–469. doi:10.1007/bf00366224
Rodríguez, J. M., and Sigarreta, J. M. (2016). Spectral properties of geometric–arithmetic index. Appl. Math. Comput. 277, 142–153. doi:10.1016/j.amc.2015.12.046
Román-Román, E. I., and Zicovich-Wilson, C. M. (2015). The role of long-range van der Waals forces in the relative stability of SiO2-zeolites. Chem. Phys. Lett. 619, 109–114. doi:10.1016/j.cplett.2014.11.044
Sato, M. (1991). Hamiltonian graph representation of zeolite frameworks and Si, Al ordering in the framework. J. Math. Chem. 7, 341–352. doi:10.1007/bf01200831
Shannon, C. E. (1948). A mathematical theory of communication. Bell Labs Tech. J. 27 (3), 379–423. doi:10.1002/j.1538-7305.1948.tb01338.x
Stacey, E., Quesne, M. G., and Catlow, C. R. A. (2023). Computational investigation of the structures and energies of microporous materials. Microporous Mesoporous Mater 358, 112382. doi:10.1016/j.micromeso.2022.112382
Ullah, A., Jamal, M., Zaman, S., and Shamsudin, (2024). Connection based novel AL topological descriptors and structural property of the zinc oxide metal organic frameworks. Phys. Scr. 99 (5), 055202. doi:10.1088/1402-4896/ad350c
Van Vreeswijk, S. H., and Weckhuysen, B. M. (2022). Emerging analytical methods to characterize zeolite-based materials. Natl. Sci. Rev. 9 (9), nwac047047. doi:10.1093/nsr/nwac047
Wilson, S. T., Lok, B. M., Messina, C. A., Cannan, T. R., and Flanigen, E. M. (1982). Aluminophosphate molecular sieves: a new class of microporous crystalline inorganic solids. J. Am. Chem. Soc. 104 (4), 1146–1147. doi:10.1021/ja00368a062
Yin, J., Zhang, J., Fu, W., Jiang, D., Lv, N., Liu, H., et al. (2021). Theoretical prediction of the SO2 absorption by hollow silica based porous ionic liquids. J. Mol. Graph. 103, 107788. doi:10.1016/j.jmgm.2020.107788
Yong, Y., Li, X., Hao, X., Cao, J., and Li, T. (2014). Theoretical prediction of low-density nanoporous frameworks of zinc sulfide based on ZnSn (n = 12, 16) nanocaged clusters. RSC Adv. 4 (70), 37333–37341. doi:10.1039/c4ra05169f
Yu, J., and Xu, R. (2006). Insight into the construction of open-framework aluminophosphates. Chem. Soc. Rev. 35 (7), 593–604. doi:10.1039/b505856m
Keywords: degree indices, information entropy, eigenvalue, spectral entropy, HOMO–LUMO gap, global reactivity descriptors, AWW zeolite
Citation: Peter P, Clement J, Arockiaraj M and Jacob K (2025) Predictive modeling of molecular interaction energies using topological and spectral entropies of zeolite AWW. Front. Chem. 13:1543588. doi: 10.3389/fchem.2025.1543588
Received: 11 December 2024; Accepted: 04 February 2025;
Published: 18 March 2025.
Edited by:
Eugeny Alexandrov, Samara State Medical University, RussiaReviewed by:
Adnan Aslam, University of Engineering and Technology, Lahore, PakistanCopyright © 2025 Peter, Clement, Arockiaraj and Jacob. This is an open-access article distributed under the terms of the Creative Commons Attribution License (CC BY). The use, distribution or reproduction in other forums is permitted, provided the original author(s) and the copyright owner(s) are credited and that the original publication in this journal is cited, in accordance with accepted academic practice. No use, distribution or reproduction is permitted which does not comply with these terms.
*Correspondence: Joseph Clement, Y2xlbWVudGptYWlsQGdtYWlsLmNvbQ==
Disclaimer: All claims expressed in this article are solely those of the authors and do not necessarily represent those of their affiliated organizations, or those of the publisher, the editors and the reviewers. Any product that may be evaluated in this article or claim that may be made by its manufacturer is not guaranteed or endorsed by the publisher.
Research integrity at Frontiers
Learn more about the work of our research integrity team to safeguard the quality of each article we publish.