- 1Department of Hepatobiliary Surgery, The Second Affiliated Hospital of Chongqing Medical University, Chongqing, China
- 2Department of General Surgery, The First Affiliated Hospital of Hunan University of Medicine, Huaihua, Hunan, China
- 3Department of Gastroenterology and Hepatology, Shanghai Ninth People’s Hospital, Shanghai Jiao Tong University School of Medicine, Shanghai, China
- 4Center for Digestive Diseases Research and Clinical Translation, Shanghai Jiao Tong University, Shanghai, China
- 5Shanghai Key Laboratory of Gut Microecology and Associated Major Diseases Research, Shanghai Jiao Tong University, Shanghai, China
Background: Prior studies have established correlations between gut microbiota (GM) dysbiosis, circulating metabolite alterations, and gastric cancer (GC) risk. However, the causal nature of these associations remains uncertain.
Methods: We utilized summary data from genome-wide association studies (GWAS) on GM (European, n=8,956), blood metabolites (European, n=120,241; East Asian, n=4,435), and GC (European, n=476,116; East Asian, n=167,122) to perform a bidirectional Mendelian randomization (MR) analysis, investigating the causal effects of GM and metabolites on GC risk. Additionally, we conducted mediation analysis (two-step MR) to identify potential metabolite mediators in the GM-GC relationship.
Results: We identified twelve negative and seven positive associations between specific GM taxa and GC risk. For blood metabolites, seven traits were found to be significantly associated with reduced GC risk in the European population, with these findings subsequently validated in the East Asian cohort. Three GM taxa showed potential causal associations with five metabolic traits: the Bacteroidia class and Bacteroidales order were positively correlated with five metabolites (all P < 0.013), while Bacteroides OTU97_27 exhibited a negative correlation with one metabolite (P = 0.007). Two-step MR analysis indicated that total lipids in intermediate-density lipoprotein (IDL), IDL particle concentration, phospholipids in medium low-density lipoprotein (LDL), phospholipids in small LDL, and free cholesterol in small LDL indirectly influenced the association between Bacteroidia class/Bacteroidales order and GC, with mediation proportions of 1.71% (P = 0.048), 1.69% (P = 0.048), 2.05% (P = 0.045), 1.85% (P = 0.048), and 1.99% (P = 0.045), respectively.
Conclusion: The present study provides suggestive evidence of a causal relationship between specific GM, blood metabolites, and GC risk, potentially offering new insights into GC etiology.
1 Introduction
Gastric cancer (GC) ranked as the fifth most common cancer and cause of cancer-related deaths globally, with 968,000 new cases and 660,000 deaths reported worldwide in 2022 (Bray et al., 2024). East Asia and Eastern Europe are the regions with the highest incidence rates globally. Extensive research suggests that gastric carcinogenesis is associated with genetic factors, Helicobacter pylori infection, dietary habits, and regional environmental factors, all interacting within a complex network (López et al., 2023; Noto et al., 2022; Smyth et al., 2020). The dynamic balance of human gut microbiota (GM) is closely linked to both physiological and pathological conditions and can directly or indirectly influence the carcinogenesis, treatment, and prognosis of GC (Wang et al., 2023b; Wang et al., 2023d). Increasing evidence indicates that GC patients experience changes in GM diversity and abundance, with alterations in specific microbial communities associated with GC risk (Yu et al., 2024). Additionally, some GM metabolites, such as short-chain fatty acids (SCFAs) and bile acid metabolites, have been associated with the formation and progression of GC (Noto et al., 2022; Yu et al., 2024). These metabolic products can enter systemic circulation via the gut-liver and gut-blood axes, exerting significant effects on systemic metabolism (Ahlawat et al., 2021; Hsu and Schnabl, 2023).
Blood metabolites, as critical indicators of the body’s metabolic status, serve as biomarkers for the diagnosis and prognosis of various diseases (Kurilshikov et al., 2019; Xu Z. et al., 2023). Previous studies have shown that changes in blood metabolites are closely linked to GM dysbiosis and its metabolic byproducts (Vojinovic et al., 2019). Consequently, the GM and its metabolites, along with blood metabolites, may play important roles in the carcinogenesis and development of GC (Cao et al., 2023; Lario et al., 2017). Further investigation into these relationships could not only clarify the pathogenesis of GC but also provide novel insights and methodologies for its early diagnosis and prevention. However, as current research is predominantly observational, it is prone to confounding factors such as dietary patterns, environmental influences, and reverse causality, often with small sample sizes. Additionally, ethical and practical limitations pose challenges to conducting randomized controlled studies that involve all bacterial strains.
Mendelian randomization (MR) is a powerful data analysis method for inferring causal relationships and has been widely applied in epidemiological research (Sekula et al., 2016). With advancements in sequencing technologies and the public availability of large-scale genome-wide association study (GWAS) data, numerous reliable genetic variations, such as single nucleotide polymorphisms (SNPs), are now accessible for MR studies. In this study, we used the latest GWAS datasets to conduct bidirectional MR and mediation analyses, aiming to explore the causal associations among GM, circulating metabolites, and GC risk. Additionally, we integrated metabolomics and microbiomics data to better elucidate the potential mediating role of circulating metabolites in the relationship between GM and GC.
2 Methods
2.1 Study design
Figure 1 presents the framework and the three core assumptions underlying this MR analysis. Firstly, we conducted bidirectional MR to examine the impact of GM on GC risk. Secondly, we evaluated the association between blood metabolites and GC risk, with comparative analyses conducted in European and East Asian populations. Thirdly, we assessed the reciprocal relationship between GM and blood metabolites through bidirectional MR. Finally, a two-step MR approach (Burgess et al., 2015a) was used to investigate whether GM influences GC through the mediation of blood metabolites. A detailed analysis flow is provided in Supplementary Figure 1. This study was conducted in accordance with the STROBE-MR checklist for the reporting of observational studies using Mendelian randomization (Supplementary Table 1) (Skrivankova et al., 2021).
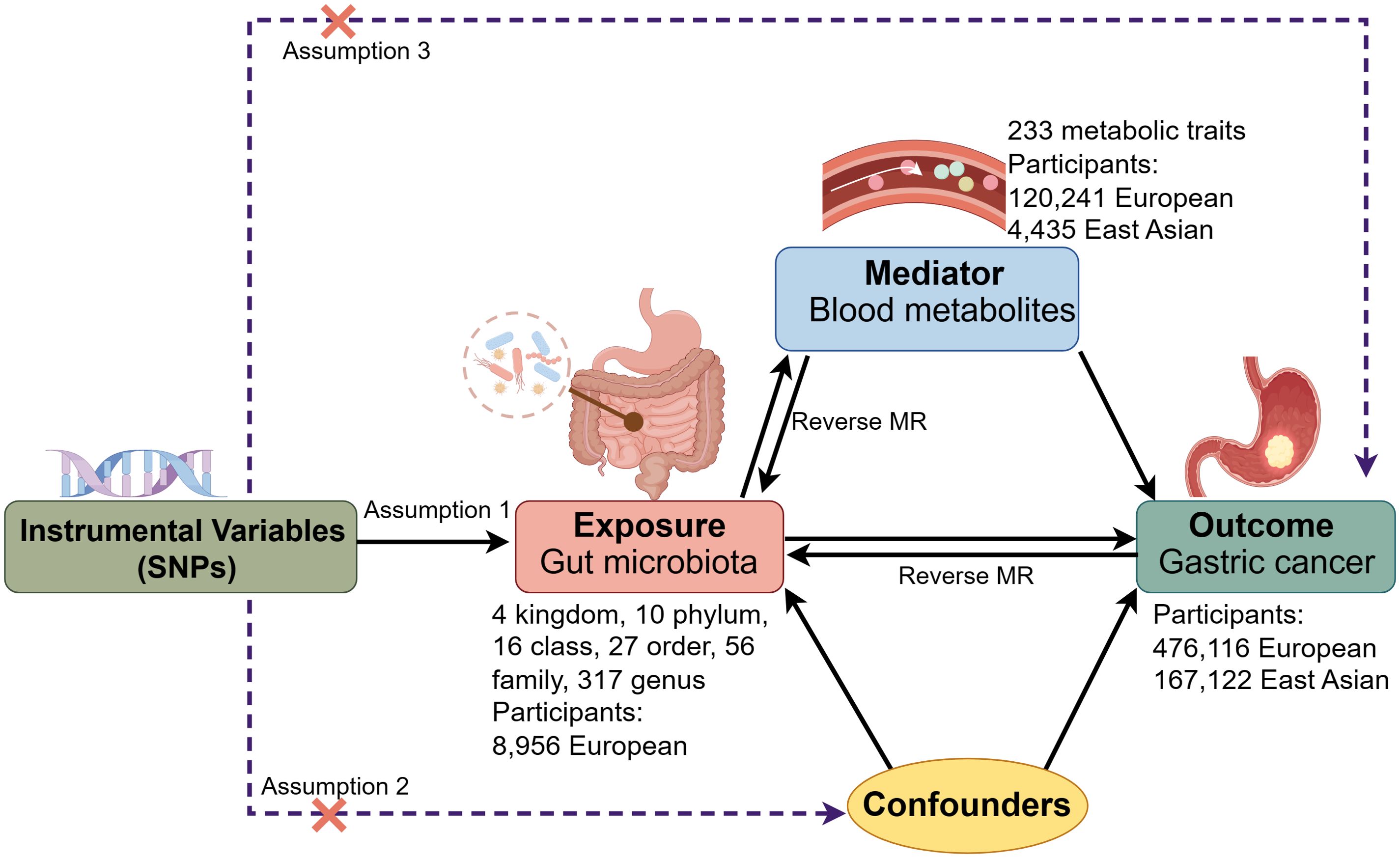
Figure 1. Framework and key assumptions of the Mendelian randomization (MR) analysis. SNPs, single nucleotide polymorphisms.
2.2 Data sources and selection of genetic instruments
Detailed characteristics of all GWAS data sources are provided in Supplementary Table 2. Summary statistics for GM were obtained from a large-scale, single-country GWAS (Rühlemann et al., 2021). This project included 8,956 participants of European ancestry from five independent cohorts (FoCus, PopGen, KORA, SHIP, and SHIP-TREND) in Germany, with fecal samples collected from all participants. Microbial data were generated through bacterial 16S rRNA gene amplicon sequencing conducted in a wet lab in Kiel, Germany, following a standardized protocol. GWAS data from all cohorts were analyzed using a generalized linear model with logistic regression, adjusting for the top 10 genetic principal components, body mass index, sex, and age as covariates. The analysis identified 430 taxonomic units, encompassing 4 kingdoms, 10 phyla, 16 classes, 27 orders, 56 families, and 317 genera.
GWAS summary statistics for blood metabolites were collected from a large-scale genome-wide meta-analysis (Karjalainen et al., 2024), including 136,016 participants across 33 cohorts (120,241 European, 4,435 East Asian, and 11,340 South Asian individuals). This study used a consistent nuclear magnetic resonance (NMR) metabolomics platform to quantify up to 233 plasma or serum metabolic traits, including lipoprotein and lipid parameters, fatty acids and their compositions, as well as non-lipid traits (such as ketone bodies, amino acids, glycolysis/gluconeogenesis, and inflammation-related metabolites). For our analysis, 81 blood metabolite ratio traits were excluded, resulting in 152 blood metabolite concentration traits included in the final MR analysis.
Summary statistics for GC were obtained from the IEU Open GWAS Project (Elsworth et al., 2020). The GWAS data were derived from a cross-population meta-analysis incorporating phenotype data from BioBank Japan, UK Biobank, and FinnGen, with a total of 476,116 participants of European ancestry (1,029 cases and 475,087 controls) and 167,122 participants of East Asian ancestry (7,921 cases and 159,201 controls) (Sakaue et al., 2021). GC was defined based on the International Classification of Diseases (ICD) codes, specifically limited to confirmed cases of gastric carcinoma, using ICD-10: C16, ICD-9: 151, and ICD-8: 151.
When selecting instrumental variables (IVs) significantly associated with GM, we applied a relaxed significance threshold of P < 1×10-5, following previous MR studies (Li et al., 2022; Wang et al., 2023a), to ensure suitable IVs for analysis. For screening IVs significantly associated with blood metabolites and GC, we applied the conventional GWAS threshold (P < 5×10-8) to select SNPs. Subsequently, we ensured independent IV through linkage disequilibrium (LD) clumping with a stringent threshold (r2 < 0.001, window size =10,000kb) (Ma et al., 2023). We manually filtered out SNPs associated with outcome risk factors from PhenoScanner V2 to further ensure the independence of IVs (Kamat et al., 2019). We computed the F-statistic (a measure of IVs’ strength) and removed SNPs with F < 10 to mitigate bias from weak IVs (Bowden et al., 2019; Burgess et al., 2011). After harmonizing the data, SNPs with palindromic sequences were also removed.
2.3 MR analysis and sensitivity analysis
Inverse variance-weighted (IVW) was used as the primary method for estimating causal effects in the two-sample MR analysis (Burgess et al., 2013; Burgess et al., 2015b), supplemented by MR-Egger (Bowden et al., 2015), weighted median (Bowden et al., 2016), and weighted mode (Hartwig et al., 2017) as additional analyses. When only one SNP was available, the Wald ratio was used as the definitive measure of causal effects, while the IVW method consolidated the Wald ratios across all genetic variants (Karjalainen et al., 2024). IVW provides the most accurate estimate of causal effects under the assumption of no horizontal pleiotropy.
A series of sensitivity analyses were conducted to detect pleiotropy and heterogeneity, ensuring the robustness of MR results. Potential horizontal pleiotropy was assessed using the Egger regression test (Bowden et al., 2015), where a P-value > 0.05 and an intercept close to zero indicate no evidence of pleiotropy. Heterogeneity was examined using Cochrane’s Q test (Burgess et al., 2013), with a P-value > 0.05 indicating no significant heterogeneity. When heterogeneity was detected, the random-effects IVW method was used for effect estimation, whereas the fixed-effects model was applied in its absence (Bowden et al., 2017). In cases of horizontal pleiotropy, MR Pleiotropy Residual Sum and Outlier (MR-PRESSO) tests were conducted to identify outliers contributing to pleiotropy (Verbanck et al., 2018). Outliers were removed if identified, and the MR analysis was then re-conducted. Additionally, a leave-one-out analysis was performed to detect individual SNP outliers that might disproportionately influence causal inference.
The potential mediating effect of blood metabolites was assessed using a two-step MR approach. The direct effect of each exposure on the outcome was estimated, including the effect of GM on GC risk (α), the effect of GM on blood metabolites (β1), and the effect of blood metabolites on GC risk (β2). The indirect effect was estimated using the product of coefficients method (β1 × β2), with the mediation proportion calculated as (β1 × β2)/α to quantify the mediating effect’s magnitude.
All P-values of IVW results were corrected using the False Discovery Rate (FDR) method. After FDR correction, P-values < 0.05 were considered strong causal associations, while exposures losing significance post-correction were regarded as having potential causal associations with the outcome (Shi et al., 2022). Data analyses were performed utilizing the “Mendelian Randomization” (version 0.9.0), “TwoSampleMR” (version 0.6.0), “forestplot” (version 1.1.1), and “MRPRESSO” (version 1.0) package in R Software 4.3.3.
3 Results
3.1 Genetic correlation between GM and GC
After excluding confounding-related SNPs (Supplementary Table 3), the number of SNPs utilized as instruments in the MR analysis ranged from 3 to 22 for the 430 GM taxa and from 3 to 6 for GC. The F-statistics ranged from 19.51 to 38.85 and from 990.65 to 11,306.03, respectively, indicating no evidence of weak instrument bias. Detailed information on genetic instruments is provided in Supplementary Tables 4, 5.
In assessing the causal impact of GM on GC, MR analysis showed that two phyla, two classes, one order, and seven genera were negatively correlated with GC, while two phyla, four families, and one genus were positively correlated with GC (Figure 2, Supplementary Table 6). Among these taxa, Butyrivibrio OTU99_155 (odds ratio [OR], 95% confidence interval [CI]: 0.94, 0.91-0.98; P = 0.002) and Phascolarctobacterium OTU99_123 (OR, 95% CI: 0.87, 0.80-0.95; P = 0.002) had the strongest protective effects against GC, while Ruminococcaceae OTU99_121 (OR, 95% CI: 1.11, 1.02-1.22; P = 0.018) exhibited the most significant association with increased GC risk.
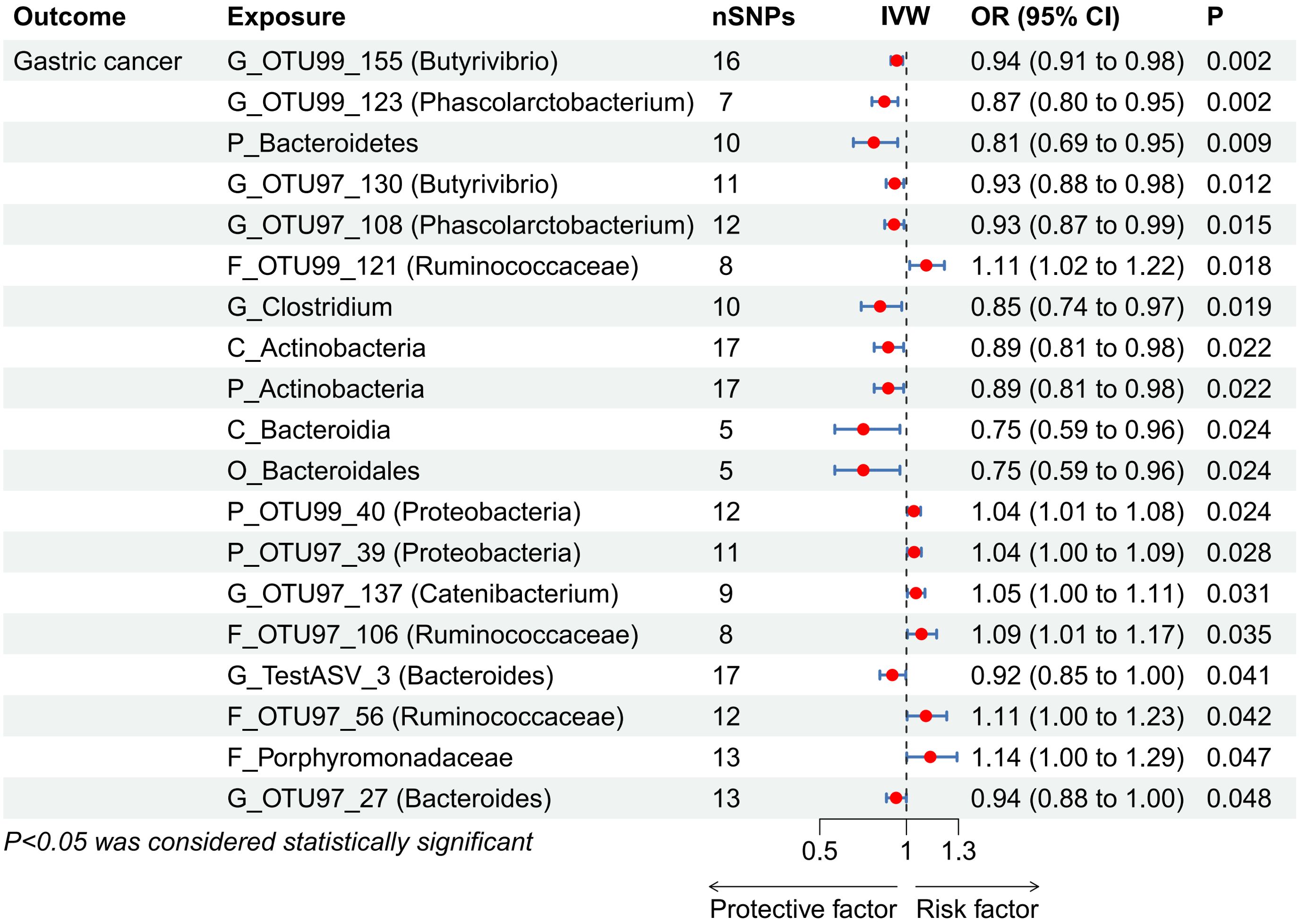
Figure 2. Forest plot showing associations between gut microbiota taxa and gastric cancer risk. CI, confidence interval; IVW, inverse-variance weighted; OR, odds ratios; SNPs, single nucleotide polymorphisms. “P_/C_/O_/F_/G_” represents phylum/class/order/family/genus, respectively.
In the reverse MR analysis, evaluating the causal impact of GC on GM revealed no significant associations between GC and any GM taxa (Supplementary Table 7).
3.2 Genetically predicted blood metabolites and GC risk
For blood metabolites, the number of SNPs utilized as instruments in the MR analysis ranged from 6 to 81 in the European population and from 6 to 90 in the East Asian population. The F-statistics ranged from 30.34 to 6,183.51 in both populations, indicating no evidence of weak instrument bias. Detailed information on genetic instruments is provided in Supplementary Table 8.
In assessing the causal impacts of blood metabolites on GC risk in the European population, MR analysis identified 37 blood metabolic traits negatively correlated with GC risk, with 31 traits remaining significant after FDR correction (Supplementary Table 9). A repeat analysis conducted in the East Asian population revealed that 11 blood metabolic traits were significantly associated with GC risk (Supplementary Table 10). When combining results from both populations, seven metabolic traits (Table 1) were consistently associated with reduced GC risk in both European and East Asian populations. These included the concentration of intermediate-density lipoprotein (IDL) particles (OR, 95% CI: 0.85, 0.77-0.92; P < 0.001), total lipids in IDL (OR, 95% CI: 0.83, 0.76-0.92; P < 0.001), phospholipids in medium low-density lipoprotein (LDL) (OR, 95% CI: 0.82, 0.74-0.91; P < 0.001), phospholipids in small LDL (OR, 95% CI: 0.83, 0.75-0.91; P < 0.001), free cholesterol in small LDL (OR, 95% CI: 0.83, 0.75-0.91; P < 0.001), free cholesterol in medium LDL (OR, 95% CI: 0.84, 0.76-0.93; P = 0.001), and cholesterol esters in IDL (OR, 95% CI: 0.87, 0.79-0.96; P = 0.005).
3.3 Genetic correlation between GM and blood metabolites
Bidirectional MR analysis was conducted to assess correlations between 19 GM taxa with causal associations to GC and 7 blood metabolic traits. After FDR correction, IVW results identified significant associations between 3 GM taxa and 5 metabolic traits. Specifically, the Bacteroidia class/Bacteroidales order was positively correlated with 5 metabolic traits, while Bacteroides OTU97_27 showed a negative correlation with free cholesterol in small LDL (Table 2, Supplementary Table 11). In the reverse MR analysis, no significant effect of metabolites on GM was observed (Supplementary Table 12).
3.4 Mediation analysis linking GM with GC via blood metabolites
Mediation MR was performed to examine the mediating effect of blood metabolites on the association between GM and GC. Results indicated that total lipids in IDL, concentration of IDL particles, phospholipids in medium LDL, phospholipids in small LDL, and free cholesterol in small LDL exhibited indirect effects on the relationship between Bacteroidia class/Bacteroidales order and GC, with mediation proportions of 1.71%, 1.69%, 2.05%, 1.85%, and 1.99%, respectively. Free cholesterol in small LDL did not show a mediating effect between Bacteroides OTU97_27 and GC (P = 0.100) (Table 3).
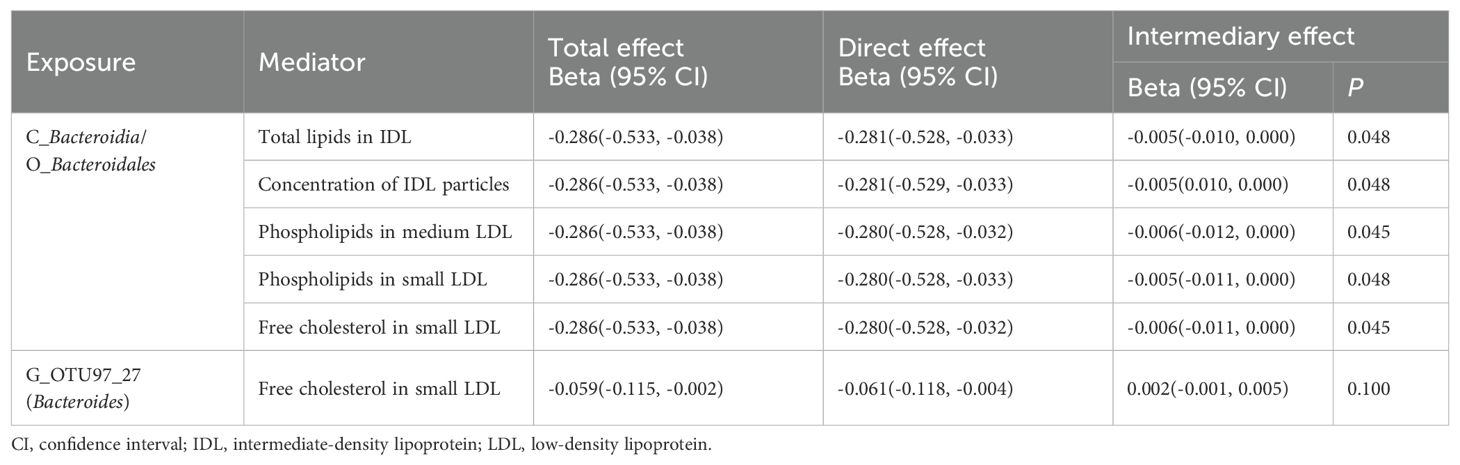
Table 3. Mediation analysis of the effect of gut microbiota on gastric cancer via blood metabolites.
3.5 Sensitivity analyses
Sensitivity analyses, including Cochrane Q test and MR-Egger test, revealed no evidence of horizontal pleiotropy or significant heterogeneity in the MR analyses (Supplementary Table 13). Additionally, the MR-PRESSO test identified no outliers or pleiotropy (Supplementary Table 13). Scatter plots illustrating the linear regression for each SNP provide a visual overview of the association effects between SNPs and phenotypes (Supplementary Figures 2-4). Funnel plots show that most MR analyses are free from significant bias (Supplementary Figures 5-7). However, when GM was used as the exposure variable, certain microbiota taxa displayed uneven SNP distribution in the funnel plot, likely due to the limited number of SNPs available for these taxa, suggesting the possibility of small-sample bias. The forest plot illustrates the causal effect of individual SNPs on the outcome (Supplementary Figures 8-10). Leave-one-out analysis did not identify any anomalous SNPs (Supplementary Figures 11-13). Collectively, these sensitivity analyses support the statistical reliability of the analytical results and enhance the credibility of the conclusions.
4 Discussion
In this large-scale MR study, we identified potential causal relationships between 19 GM taxa and 7 blood metabolic traits with GC and simultaneously explored their potential interactions. Using a two-step MR method for mediation analysis, we found that five blood metabolic traits may mediate the causal relationship between GM and GC. These findings suggest that the protective effects of the Bacteroidia class and Bacteroidales order in reducing GC risk may be partially attributed to their influence on the concentrations of five blood metabolites.
In our study, Bacteroides exhibited a negative correlation with GC across taxonomic levels from phylum to genus, suggesting a potential protective role for Bacteroides in GC patients. Its reduced abundance may be associated with gastric carcinogenesis. An observational study examining fecal samples from 22 healthy participants and 20 GC patients reported findings consistent with ours, revealing a significant decrease in Bacteroides abundance from phylum to genus in GC patients compared to healthy controls (Liang et al., 2019). Additionally, an MR study conducted in a different population further supports our findings (Xie et al., 2023). Bacteroides is a key commensal bacterium in the human gut and a major producer of SCFAs, which play a crucial role in maintaining immune homeostasis within the gastrointestinal tract (Raoul et al., 2024; Wexler, 2007). In gastrointestinal neoplasms, both SCFA levels and the abundance of SCFA-producing bacteria are significantly reduced (Dong et al., 2023; Yu et al., 2024). Previous studies have shown that direct supplementation of dietary fiber and probiotics or the transplantation of fecal microbiota to alter GM composition can enhance SCFA levels and suppress neoplasm development (Dong et al., 2023; Yu et al., 2024).
Growing evidence suggests that SCFAs, as natural histone deacetylase (HDAC) inhibitors produced by GM, play a critical role across various immune cells. SCFAs can promote macrophage differentiation and enhance antimicrobial activity by inhibiting HDAC3 (Schulthess et al., 2019). They also induce IL-10 expression in T cells and enhance B cell activation, thus promoting immune tolerance and maintaining gut microbial balance (Kim, 2023). Recent experimental studies have found that butyrate regulates CD8+ T cell cytotoxicity through the G protein-coupled receptor 109A (GPR109A) and homologous domain protein homologous box (HOPX) pathway, resulting in enhanced anti-cancer activity against tumor cells in the GC microenvironment (Yu et al., 2024). Notably, butyrate and other SCFAs have direct effects on the immune system and also indirectly suppress the development of gastrointestinal tumors, including GC, by preserving gut barrier function and preventing microbial dysbiosis (Gou et al., 2024; Kaźmierczak-Siedlecka et al., 2022). These findings suggest that increasing SCFA levels through dietary or probiotic interventions may have significant potential in reducing GC risk (Gou et al., 2024; Yu et al., 2024).
In our study, Butyrivibrio and Phascolarctobacterium genera exhibited the most significant negative correlations with GC risk. Both genera belong to the Firmicutes phylum and are producers of SCFAs. Butyrivibrio, a beneficial gut bacterium, generates butyrate, a metabolite linked to numerous health-promoting effects, including the maintenance of the intestinal barrier, energy homeostasis, and anti-inflammatory and antioxidant activities (Hodgkinson et al., 2023; Zhang et al., 2021). A cross-sectional study reported a significant increase in Butyrivibrio abundance in patients’ intestines following subtotal gastrectomy for early-stage GC (Lin et al., 2018). However, research on the association between Butyrivibrio abundance and GC risk remains limited, warranting further clinical studies to elucidate their relationship. Phascolarctobacterium, primarily utilizing succinate produced by Bacteroides as a nutritional substrate, may interact with Bacteroides in the gut (Ikeyama et al., 2020; Watanabe et al., 2012). We therefore hypothesize that Phascolarctobacterium abundance may decrease along with the reduction of Bacteroides in the gut of GC patients. A recent study indeed found a significant reduction in Phascolarctobacterium abundance among GC patients (Yu et al., 2024), which indirectly supports its protective effect against GC.
The Ruminococcus genus includes both beneficial and harmful species, and its impact on GC risk may vary across populations and classification levels. Our findings indicate a positive correlation between the Ruminococcaceae family and GC risk. A fecal microbiota analysis of a European cohort similarly revealed a marked increase in Ruminococcus abundance among GC patients (Youssef et al., 2018). Conversely, studies analyzing fecal samples from East Asian populations reported conflicting results (Lee et al., 2024; Yu et al., 2024). Consequently, additional research with larger, ethnically diverse samples is necessary to clarify the relationship between Ruminococcus and GC risk.
Our MR study identified several blood metabolic traits with causal effects on GC risk, providing insights from a genetic perspective. For instance, elevated levels of phospholipids and free cholesterol in small and medium LDL were associated with a decreased GC risk. Similarly, higher levels of total lipids, cholesterol esters in IDL, and increased IDL particle concentrations correlated with reduced GC risk. Prior research has predominantly examined traditional blood markers linked to lipoproteins and lipids in relation to GC (Qiu et al., 2023). A meta-analysis summary has demonstrated that serum high-density lipoprotein cholesterol (HDL-C) and total cholesterol (TC) levels are negatively associated with GC risk, while low-density lipoprotein cholesterol (LDL-C) and triglycerides (TG) levels show no association with GC risk (Xu S. et al., 2023). Our study is the first to examine the causal relationship between circulating lipoprotein subclasses and GC risk, providing new insights into the intricate biochemical mechanisms underlying lipid metabolism dysregulation in GC patients.
To date, no studies have directly linked the Bacteroidia class or the Bacteroidales order to circulating lipoprotein subfractions. Previous observational studies have confirmed associations between specific GM taxa and subfractions of very-low-density lipoprotein (VLDL) and HDL, offering some understanding of the GM’s influence on host systemic metabolism (Vojinovic et al., 2019). The mechanisms by which GM may influence circulating metabolites remain incompletely understood, but they likely involve GM’s role in bile acid and SCFA metabolism (Ghazalpour et al., 2016). Prior studies suggest that bacteria-derived bile acids entering the bloodstream can participate in systemic lipid metabolism (Allayee and Hazen, 2015), while SCFAs such as butyrate, acetate, and propionate may also influence lipid biosynthesis (Allayee and Hazen, 2015). Through mediation analysis, we further explored the potential mediating effects of circulating metabolites on the association between GM and GC. Overall, the mediation proportions of these blood metabolite categories in the GM-GC relationship were relatively low. These modest mediation percentages likely reflect the complex and multifactorial nature of GC etiology, suggesting that blood metabolites play a limited mediating role in the relationship between GM and GC. Furthermore, the similarity in mediation proportions observed among certain metabolite categories may be attributed to shared lipid metabolism pathways and the overlapping biological functions of these lipid components, leading to parallel mediating effects on GC risk. From the funnel plots in our study, we observed that when GM was used as the exposure, the SNP distribution for certain taxa was uneven, indicating potential heterogeneity in the results. As with most MR studies on GM (Chen et al., 2023; Li et al., 2023; Zhou et al., 2023), this heterogeneity may be due to the limited number of available IVs. Consequently, the observed associations between these taxa and GC cannot entirely rule out the influence of chance or unmeasured confounding factors. Further research is therefore needed to confirm the definitive causal relationships between these taxa and GC.
This study has several strengths. We utilized the most recent and largest GWAS summary data available for blood metabolites and GC, with over 136,000 participants for metabolite data, 476,116 European participants, and 167,122 East Asian participants for GC data. Furthermore, we validated associations between blood metabolites and GC in both European and East Asian populations, ensuring robust statistical power. This study is also the first to investigate causal relationships among human GM, blood metabolites, and GC from a genetic standpoint, minimizing the confounding effects commonly encountered in observational studies.
Conversely, several potential limitations of this study should be acknowledged. First, our analysis relied exclusively on GM data from European populations. Given the substantial variability in GM composition across populations and ethnic groups, the generalizability of our findings may be restricted. Second, the GM data were generated using 16S rRNA sequencing, which inherently limits taxonomic resolution and hampers differentiation between closely related bacterial species (Rühlemann et al., 2021). Additionally, our study was restricted to bacterial taxa, while other non-bacterial microbiome constituents, such as fungi, viruses, archaea, and protozoa, are increasingly recognized for their roles in gastrointestinal health and disease (Jaswal et al., 2023). Expanding future analyses to encompass these groups could provide a more comprehensive understanding of the microbiome’s role in GC. Third, although we used a bidirectional MR approach to evaluate causal relationships, the power of the reverse MR analysis may be limited by the relatively low number of GC cases, potentially impacting the robustness of these reverse causal findings. Thus, we recommend cautious interpretation of these results. Additionally, although our MR analysis detected no pleiotropy, it cannot be entirely ruled out, especially as GM influences luminal metabolites that may not persist in the bloodstream or be detectable at all sampling points. Lastly, we identified GM taxa with nominally significant associations with GC, though these associations lost significance after applying multiple correction tests. Given the complex interdependencies among GM taxa (Faust and Raes, 2012), multiple testing corrections may be overly conservative in high-dimensional datasets (Wang et al., 2023c). Therefore, while not meeting the threshold for strict significance, the nominal associations identified should not be dismissed entirely, particularly as prior studies have corroborated that some of these taxa are associated with GC risk. Future studies with larger sample sizes and refined correction techniques may help to confirm these associations more robustly.
5 Conclusions
In summary, our MR analysis using genetic instruments identified several blood metabolites with potential causal associations to GC risk. Additionally, it provided suggestive evidence for a causal relationship between GM and GC, as well as the potential mediating role of blood metabolites in the GM-GC association. While this exploratory study offers valuable insights into the complex interactions between GM, blood metabolites, and GC, further research is essential to validate these causal relationships and elucidate the underlying mechanisms. Such efforts could ultimately inform novel GC treatment strategies centered on GM modulation and targeted metabolite interventions.
Data availability statement
The original contributions presented in the study are included in the article/Supplementary Material. Further inquiries can be directed to the corresponding author.
Author contributions
XL: Conceptualization, Data curation, Formal analysis, Funding acquisition, Investigation, Methodology, Resources, Software, Validation, Visualization, Writing – original draft, Writing – review & editing. HL: Software, Validation, Writing – review & editing. JP: Funding acquisition, Resources, Writing – review & editing. JG: Conceptualization, Project administration, Supervision, Writing – review & editing.
Funding
The author(s) declare financial support was received for the research, authorship, and/or publication of this article. This research was funded by Clinical Medical Technology Demonstration Base for Minimally Invasive Treatment of Hepatobiliary and Pancreatic Diseases in Huaihua, grant number 2018N2503.
Acknowledgments
The authors express their gratitude to Rühlemann MC et al. and Karjalainen MK et al. and all investigators and participants from BioBank Japan, FinnGen, and UK Biobank for sharing summary statistics data.
Conflict of interest
The authors declare that the research was conducted in the absence of any commercial or financial relationships that could be construed as a potential conflict of interest.
Publisher’s note
All claims expressed in this article are solely those of the authors and do not necessarily represent those of their affiliated organizations, or those of the publisher, the editors and the reviewers. Any product that may be evaluated in this article, or claim that may be made by its manufacturer, is not guaranteed or endorsed by the publisher.
Supplementary material
The Supplementary Material for this article can be found online at: https://www.frontiersin.org/articles/10.3389/fcimb.2024.1453286/full#supplementary-material
Abbreviations
CI, confidence interval; FDR, False Discovery Rate; GC, gastric cancer; GM, gut microbiota; GWAS, genome-wide association study; HDL, high-density lipoprotein; IVW, inverse variance-weighted; IVs, instrumental variables; IDL, intermediate-density lipoprotein; LD, linkage disequilibrium; LDL, low-density lipoprotein; MR, Mendelian randomization; MR-PRESSO, MR Pleiotropy Residual Sum and Outlier; NMR, nuclear magnetic resonance; OR, odds ratio; SCFA, short-chain fatty acids; SNPs, single nucleotide polymorphisms; TG, triglycerides; TC, total cholesterol; VLDL, very-low-density lipoprotein.
References
Ahlawat, S., Asha, Sharma, K. K. (2021). Gut-organ axis: a microbial outreach and networking. Lett. Appl. Microbiol. 72, 636–668. doi: 10.1111/lam.13333
Allayee, H., Hazen, S. L. (2015). Contribution of gut bacteria to lipid levels: another metabolic role for microbes? Circ. Res. 117, 750–754. doi: 10.1161/circresaha.115.307409
Bowden, J., Davey Smith, G., Burgess, S. (2015). Mendelian randomization with invalid instruments: effect estimation and bias detection through Egger regression. Int. J. Epidemiol. 44, 512–525. doi: 10.1093/ije/dyv080
Bowden, J., Davey Smith, G., Haycock, P. C., Burgess, S. (2016). Consistent estimation in Mendelian randomization with some invalid instruments using a weighted median estimator. Genet. Epidemiol. 40, 304–314. doi: 10.1002/gepi.21965
Bowden, J., Del Greco, M. F., Minelli, C., Davey Smith, G., Sheehan, N., Thompson, J. (2017). A framework for the investigation of pleiotropy in two-sample summary data Mendelian randomization. Stat. Med. 36, 1783–1802. doi: 10.1002/sim.7221
Bowden, J., Del Greco, M. F., Minelli, C., Zhao, Q., Lawlor, D. A., Sheehan, N. A., et al. (2019). Improving the accuracy of two-sample summary-data Mendelian randomization: moving beyond the NOME assumption. Int. J. Epidemiol. 48, 728–742. doi: 10.1093/ije/dyy258
Bray, F., Laversanne, M., Sung, H., Ferlay, J., Siegel, R. L., Soerjomataram, I., et al. (2024). Global cancer statistics 2022: GLOBOCAN estimates of incidence and mortality worldwide for 36 cancers in 185 countries. CA Cancer J. Clin. 74, 229–263. doi: 10.3322/caac.21834
Burgess, S., Butterworth, A., Thompson, S. G. (2013). Mendelian randomization analysis with multiple genetic variants using summarized data. Genet. Epidemiol. 37, 658–665. doi: 10.1002/gepi.21758
Burgess, S., Daniel, R. M., Butterworth, A. S., Thompson, S. G. (2015a). Network Mendelian randomization: using genetic variants as instrumental variables to investigate mediation in causal pathways. Int. J. Epidemiol. 44, 484–495. doi: 10.1093/ije/dyu176
Burgess, S., Scott, R. A., Timpson, N. J., Davey Smith, G., Thompson, S. G., Consortium, E.-I. (2015b). Using published data in Mendelian randomization: a blueprint for efficient identification of causal risk factors. Eur. J. Epidemiol. 30, 543–552. doi: 10.1007/s10654-015-0011-z
Burgess, S., Thompson, S. G., Collaboration, C. C. G (2011). Avoiding bias from weak instruments in Mendelian randomization studies. Int. J. Epidemiol. 40, 755–764. doi: 10.1093/ije/dyr036
Cao, K., Lyu, Y., Chen, J., He, C., Lyu, X., Zhang, Y., et al. (2023). Prognostic implication of plasma metabolites in gastric cancer. Int. J. Mol. Sci. 24, 12774. doi: 10.3390/ijms241612774
Chen, Z., Shi, W., Chen, K., Lu, C., Li, X., Li, Q. (2023). Elucidating the causal association between gut microbiota and intrahepatic cholangiocarcinoma through Mendelian randomization analysis. Front. Microbiol. 14. doi: 10.3389/fmicb.2023.1288525
Dong, Y., Zhang, K., Wei, J., Ding, Y., Wang, X., Hou, H., et al. (2023). Gut microbiota-derived short-chain fatty acids regulate gastrointestinal tumor immunity: a novel therapeutic strategy? Front. Immunol. 14. doi: 10.3389/fimmu.2023.1158200
Elsworth, B., Lyon, M., Alexander, T., Liu, Y., Matthews, P., Hallett, J., et al. (2020). The MRC IEU OpenGWAS data infrastructure. bioRxiv 2020, 8.10.244293. doi: 10.1101/2020.08.10.244293
Faust, K., Raes, J. (2012). Microbial interactions: from networks to models. Nat. Rev. Microbiol. 10, 538–550. doi: 10.1038/nrmicro2832
Ghazalpour, A., Cespedes, I., Bennett, B. J., Allayee, H. (2016). Expanding role of gut microbiota in lipid metabolism. Curr. Opin. Lipidol. 27, 141–147. doi: 10.1097/mol.0000000000000278
Gou, H., Zeng, R., Lau, H. C. H., Yu, J. (2024). Gut microbial metabolites: Shaping future diagnosis and treatment against gastrointestinal cancer. Pharmacol. Res. 208, 107373. doi: 10.1016/j.phrs.2024.107373
Hartwig, F. P., Davey Smith, G., Bowden, J. (2017). Robust inference in summary data Mendelian randomization via the zero modal pleiotropy assumption. Int. J. Epidemiol. 46, 1985–1998. doi: 10.1093/ije/dyx102
Hodgkinson, K., El Abbar, F., Dobranowski, P., Manoogian, J., Butcher, J., Figeys, D., et al. (2023). Butyrate’s role in human health and the current progress towards its clinical application to treat gastrointestinal disease. Clin. Nutr. 42, 61–75. doi: 10.1016/j.clnu.2022.10.024
Hsu, C. L., Schnabl, B. (2023). The gut-liver axis and gut microbiota in health and liver disease. Nat. Rev. Microbiol. 21, 719–733. doi: 10.1038/s41579-023-00904-3
Ikeyama, N., Murakami, T., Toyoda, A., Mori, H., Iino, T., Ohkuma, M., et al. (2020). Microbial interaction between the succinate-utilizing bacterium Phascolarctobacterium faecium and the gut commensal Bacteroides thetaiotaomicron. Microbiologyopen 9, e1111. doi: 10.1002/mbo3.1111
Jaswal, K., Todd, O. A., Behnsen, J. (2023). Neglected gut microbiome: interactions of the non-bacterial gut microbiota with enteric pathogens. Gut Microbes 15, 2226916. doi: 10.1080/19490976.2023.2226916
Kamat, M. A., Blackshaw, J. A., Young, R., Surendran, P., Burgess, S., Danesh, J., et al. (2019). PhenoScanner V2: an expanded tool for searching human genotype-phenotype associations. Bioinformatics 35, 4851–4853. doi: 10.1093/bioinformatics/btz469
Karjalainen, M. K., Karthikeyan, S., Oliver-Williams, C., Sliz, E., Allara, E., Fung, W. T., et al. (2024). Genome-wide characterization of circulating metabolic biomarkers. Nature 628, 130–138. doi: 10.1038/s41586-024-07148-y
Kaźmierczak-Siedlecka, K., Daca, A., Roviello, G., Catalano, M., Połom, K. (2022). Interdisciplinary insights into the link between gut microbiome and gastric carcinogenesis-what is currently known? Gastric Cancer 25, 1–10. doi: 10.1007/s10120-021-01260-y
Kim, C. H. (2023). Complex regulatory effects of gut microbial short-chain fatty acids on immune tolerance and autoimmunity. Cell Mol. Immunol. 20, 341–350. doi: 10.1038/s41423-023-00987-1
Kurilshikov, A., van den Munckhof, I. C. L., Chen, L., Bonder, M. J., Schraa, K., Rutten, J. H. W., et al. (2019). Gut microbial associations to plasma metabolites linked to cardiovascular phenotypes and risk. Circ. Res. 124, 1808–1820. doi: 10.1161/circresaha.118.314642
Lario, S., Ramírez-Lázaro, M. J., Sanjuan-Herráez, D., Brunet-Vega, A., Pericay, C., Gombau, L., et al. (2017). Plasma sample based analysis of gastric cancer progression using targeted metabolomics. Sci. Rep. 7, 17774. doi: 10.1038/s41598-017-17921-x
Lee, S. Y., Jhun, J., Woo, J. S., Lee, K. H., Hwang, S. H., Moon, J., et al. (2024). Gut microbiome-derived butyrate inhibits the immunosuppressive factors PD-L1 and IL-10 in tumor-associated macrophages in gastric cancer. Gut Microbes 16, 2300846. doi: 10.1080/19490976.2023.2300846
Li, P., Wang, H., Guo, L., Gou, X., Chen, G., Lin, D., et al. (2022). Association between gut microbiota and preeclampsia-eclampsia: a two-sample Mendelian randomization study. BMC Med. 20, 443. doi: 10.1186/s12916-022-02657-x
Li, Y., Liang, X., Lyu, Y., Wang, K., Han, L., Wang, Y., et al. (2023). Association between the gut microbiota and nonalcoholic fatty liver disease: A two-sample Mendelian randomization study. Dig Liver Dis. 55, 1464–1471. doi: 10.1016/j.dld.2023.07.014
Liang, W., Yang, Y., Wang, H., Wang, H., Yu, X., Lu, Y., et al. (2019). Gut microbiota shifts in patients with gastric cancer in perioperative period. Med. (Baltimore) 98, e16626. doi: 10.1097/md.0000000000016626
Lin, X. H., Huang, K. H., Chuang, W. H., Luo, J. C., Lin, C. C., Ting, P. H., et al. (2018). The long term effect of metabolic profile and microbiota status in early gastric cancer patients after subtotal gastrectomy. PloS One 13, e0206930. doi: 10.1371/journal.pone.0206930
López, M. J., Carbajal, J., Alfaro, A. L., Saravia, L. G., Zanabria, D., Araujo, J. M., et al. (2023). Characteristics of gastric cancer around the world. Crit. Rev. Oncol. Hematol. 181, 103841. doi: 10.1016/j.critrevonc.2022.103841
Ma, J., Li, J., Jin, C., Yang, J., Zheng, C., Chen, K., et al. (2023). Association of gut microbiome and primary liver cancer: A two-sample Mendelian randomization and case-control study. Liver Int. 43, 221–233. doi: 10.1111/liv.15466
Noto, J. M., Piazuelo, M. B., Shah, S. C., Romero-Gallo, J., Hart, J. L., Di, C., et al. (2022). Iron deficiency linked to altered bile acid metabolism promotes Helicobacter pylori-induced inflammation-driven gastric carcinogenesis. J. Clin. Invest. 132, e147822. doi: 10.1172/jci147822
Qiu, Y., Xu, Z., Xie, Q., Zhang, R., Wang, L., Zhao, L., et al. (2023). Association of plasma lipid metabolism profiles with overall survival for patients with gastric cancer undergoing gastrectomy based on (1)H-NMR spectroscopy. Nutr. Metab. (Lond) 20, 7. doi: 10.1186/s12986-023-00728-1
Raoul, P., Maccauro, V., Cintoni, M., Scarpellini, E., Ianiro, G., Gasbarrini, A., et al. (2024). Microbiota-gastric cancer interactions and the potential influence of nutritional therapies. Int. J. Mol. Sci. 25, 1679. doi: 10.3390/ijms25031679
Rühlemann, M. C., Hermes, B. M., Bang, C., Doms, S., Moitinho-Silva, L., Thingholm, L. B., et al. (2021). Genome-wide association study in 8,956 German individuals identifies influence of ABO histo-blood groups on gut microbiome. Nat. Genet. 53, 147–155. doi: 10.1038/s41588-020-00747-1
Sakaue, S., Kanai, M., Tanigawa, Y., Karjalainen, J., Kurki, M., Koshiba, S., et al. (2021). A cross-population atlas of genetic associations for 220 human phenotypes. Nat. Genet. 53, 1415–1424. doi: 10.1038/s41588-021-00931-x
Schulthess, J., Pandey, S., Capitani, M., Rue-Albrecht, K. C., Arnold, I., Franchini, F., et al. (2019). The short chain fatty acid butyrate imprints an antimicrobial program in macrophages. Immunity 50, 432–445.e7. doi: 10.1016/j.immuni.2018.12.018
Sekula, P., Del Greco, M. F., Pattaro, C., Köttgen, A. (2016). Mendelian randomization as an approach to assess causality using observational data. J. Am. Soc. Nephrol. 27, 3253–3265. doi: 10.1681/asn.2016010098
Shi, X., Wei, T., Hu, Y., Wang, M., Tang, Y. (2022). The associations between plasma soluble Trem1 and neurological diseases: a Mendelian randomization study. J. Neuroinflamm. 19, 218. doi: 10.1186/s12974-022-02582-z
Skrivankova, V. W., Richmond, R. C., Woolf, B. A. R., Yarmolinsky, J., Davies, N. M., Swanson, S. A., et al. (2021). Strengthening the reporting of observational studies in epidemiology using Mendelian randomization: the STROBE-MR statement. JAMA 326, 1614–1621. doi: 10.1001/jama.2021.18236
Smyth, E. C., Nilsson, M., Grabsch, H. I., van Grieken, N. C., Lordick, F. (2020). Gastric cancer. Lancet 396, 635–648. doi: 10.1016/s0140-6736(20)31288-5
Verbanck, M., Chen, C. Y., Neale, B., Do, R. (2018). Detection of widespread horizontal pleiotropy in causal relationships inferred from Mendelian randomization between complex traits and diseases. Nat. Genet. 50, 693–698. doi: 10.1038/s41588-018-0099-7
Vojinovic, D., Radjabzadeh, D., Kurilshikov, A., Amin, N., Wijmenga, C., Franke, L., et al. (2019). Relationship between gut microbiota and circulating metabolites in population-based cohorts. Nat. Commun. 10, 5813. doi: 10.1038/s41467-019-13721-1
Wang, Q., Dai, H., Hou, T., Hou, Y., Wang, T., Lin, H., et al. (2023c). Dissecting causal relationships between gut microbiota, blood metabolites, and stroke: A Mendelian randomization study. J. Stroke 25, 350–360. doi: 10.5853/jos.2023.00381
Wang, M., Fan, J., Huang, Z., Zhou, D., Wang, X. (2023a). Causal relationship between gut microbiota and gout: A two-sample Mendelian randomization study. Nutrients 15, 4260. doi: 10.3390/nu15194260
Wang, Y., Han, W., Wang, N., Han, M., Ban, M., Dai, J., et al. (2023d). The role of microbiota in the development and treatment of gastric cancer. Front. Oncol. 13. doi: 10.3389/fonc.2023.1224669
Wang, M., Yang, G., Tian, Y., Zhang, Q., Liu, Z., Xin, Y. (2023b). The role of the gut microbiota in gastric cancer: the immunoregulation and immunotherapy. Front. Immunol. 14. doi: 10.3389/fimmu.2023.1183331
Watanabe, Y., Nagai, F., Morotomi, M. (2012). Characterization of Phascolarctobacterium succinatutens sp. nov., an asaccharolytic, succinate-utilizing bacterium isolated from human feces. Appl. Environ. Microbiol. 78, 511–518. doi: 10.1128/aem.06035-11
Wexler, H. M. (2007). Bacteroides: the good, the bad, and the nitty-gritty. Clin. Microbiol. Rev. 20, 593–621. doi: 10.1128/cmr.00008-07
Xie, N., Wang, Z., Shu, Q., Liang, X., Wang, J., Wu, K., et al. (2023). Association between gut microbiota and digestive system cancers: A bidirectional two-sample Mendelian randomization study. Nutrients 15, 2937. doi: 10.3390/nu15132937
Xu, S., Fan, Y., Tan, Y., Zhang, L., Li, X. (2023). Association between blood lipid levels and risk of gastric cancer: A systematic review and meta-analysis. PloS One 18, e0288111. doi: 10.1371/journal.pone.0288111
Xu, Z., Huang, Y., Hu, C., Du, L., Du, Y. A., Zhang, Y., et al. (2023). Efficient plasma metabolic fingerprinting as a novel tool for diagnosis and prognosis of gastric cancer: a large-scale, multicentre study. Gut 72, 2051–2067. doi: 10.1136/gutjnl-2023-330045
Youssef, O., Lahti, L., Kokkola, A., Karla, T., Tikkanen, M., Ehsan, H., et al. (2018). Stool microbiota composition differs in patients with stomach, colon, and rectal neoplasms. Dig Dis. Sci. 63, 2950–2958. doi: 10.1007/s10620-018-5190-5
Yu, X., Ou, J., Wang, L., Li, Z., Ren, Y., Xie, L., et al. (2024). Gut microbiota modulate CD8(+) T cell immunity in gastric cancer through Butyrate/GPR109A/HOPX. Gut Microbes 16, 2307542. doi: 10.1080/19490976.2024.2307542
Zhang, L., Liu, C., Jiang, Q., Yin, Y. (2021). Butyrate in energy metabolism: there is still more to learn. Trends Endocrinol. Metab. 32, 159–169. doi: 10.1016/j.tem.2020.12.003
Keywords: gut microbiota, metabolites, gastric cancer, Mendelian randomization, causal association
Citation: Li X, Lin H, Peng J and Gong J (2025) Exploring the mediating role of blood metabolites in the relationship between gut microbiota and gastric cancer risk: a Mendelian randomization study. Front. Cell. Infect. Microbiol. 14:1453286. doi: 10.3389/fcimb.2024.1453286
Received: 22 June 2024; Accepted: 12 December 2024;
Published: 07 January 2025.
Edited by:
Yolanda López-Vidal, National Autonomous University of Mexico, MexicoReviewed by:
Aaron Balasingam Koenig, Celia Scott Weatherhead School of Public Health and Tropical Medicine, United StatesMingzhe Guo, University of Nevada, United States
Copyright © 2025 Li, Lin, Peng and Gong. This is an open-access article distributed under the terms of the Creative Commons Attribution License (CC BY). The use, distribution or reproduction in other forums is permitted, provided the original author(s) and the copyright owner(s) are credited and that the original publication in this journal is cited, in accordance with accepted academic practice. No use, distribution or reproduction is permitted which does not comply with these terms.
*Correspondence: Jianping Gong, Z29uZ2ppYW5waW5nMTFAMTI2LmNvbQ==