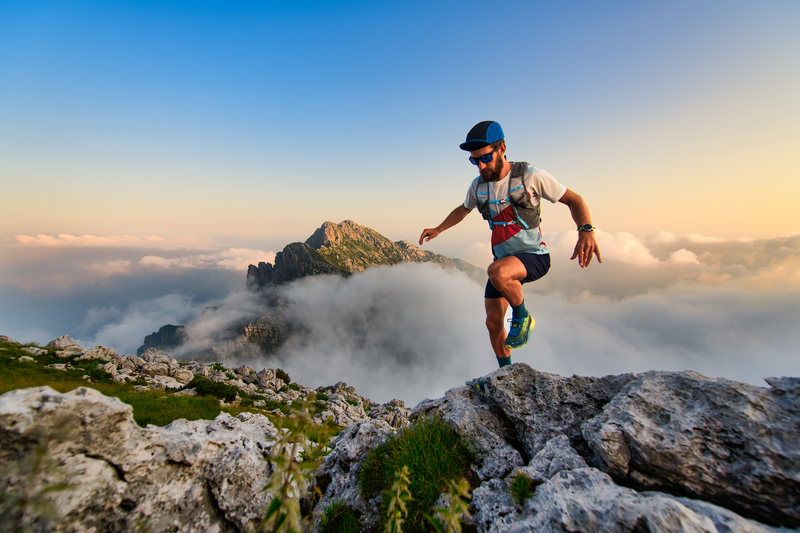
94% of researchers rate our articles as excellent or good
Learn more about the work of our research integrity team to safeguard the quality of each article we publish.
Find out more
ORIGINAL RESEARCH article
Front. Cardiovasc. Med.
Sec. Pediatric Cardiology
Volume 12 - 2025 | doi: 10.3389/fcvm.2025.1471989
This article is part of the Research Topic Pediatric and Perinatal Cardiology; Insights, Advances and Updates View all 7 articles
The final, formatted version of the article will be published soon.
You have multiple emails registered with Frontiers:
Please enter your email address:
If you already have an account, please login
You don't have a Frontiers account ? You can register here
IntroductionThe diagnostic performance of automated analysis of electrocardiograms for screening children with pediatric heart diseases at risk of sudden cardiac death is unknown. In this study, we aimed to develop and validate a deep learning-based model for automated analysis of ECGs in children.MethodsWave data of 12-lead electrocardiograms were transformed into a tensor sizing 2 x 12 x 400 using signal processing methods. A deep learning-based model to classify abnormal electrocardiograms based on age, sex, and the transformed wave data was developed using electrocardiograms performed in patients at the age of 6-18 years during 2003-2006 at a tertiary referral hospital in Japan. Eighty-three percent of the patients were assigned to a training group, and 17% to a test group. The diagnostic performance of the model and a conventional algorithm (ECAPS12C, Nihon Kohden, Japan) for classifying abnormal electrocardiograms were evaluated using the cross-tabulation, McNemar’s test, and decision curve analysis.ResultsWe included 1,842 ECGs performed in 1,062 patients in this study, and 310 electrocardiograms performed in 177 patients were included in the test group. The specificity of the deep learning-based model for detecting abnormal electrocardiograms was not significantly different from that of the conventional algorithm. For detecting electrocardiograms with ST-T abnormality, complete right bundle branch block, and QRS axis abnormality, the specificity of the deep learning-based model was higher than that of the conventional algorithm at the same sensitivity.ConclusionsThe present new deep learning-based method of screening for abnormal electrocardiograms in children showed at least a similar diagnostic performance compared to that of a conventional algorithm. Further studies are warranted to develop an automated analysis of electrocardiograms in school-age children.
Keywords: Electrocardiograms, School-age children, screening, congenital heart disease, deep learning, Arrhythima detection
Received: 28 Jul 2024; Accepted: 11 Feb 2025.
Copyright: © 2025 Toba, Mitani, Sugitani, Ohashi, Sawada, Takeoka, Tsuboya, Ohya, Yodoya, Yamasaki, Nakayama, Ito, Hirayama and Takao. This is an open-access article distributed under the terms of the Creative Commons Attribution License (CC BY). The use, distribution or reproduction in other forums is permitted, provided the original author(s) or licensor are credited and that the original publication in this journal is cited, in accordance with accepted academic practice. No use, distribution or reproduction is permitted which does not comply with these terms.
* Correspondence:
Shuhei Toba, Department of Thoracic and Cardiovascular Surgery, Graduate School of Medicine, Mie University, Tsu, 5148507, Mie, Japan
Yoshihide Mitani, Department of Pediatrics, Graduate School of Medicine, Mie University, Tsu, 5148507, Mie, Japan
Disclaimer: All claims expressed in this article are solely those of the authors and do not necessarily represent those of their affiliated organizations, or those of the publisher, the editors and the reviewers. Any product that may be evaluated in this article or claim that may be made by its manufacturer is not guaranteed or endorsed by the publisher.
Research integrity at Frontiers
Learn more about the work of our research integrity team to safeguard the quality of each article we publish.