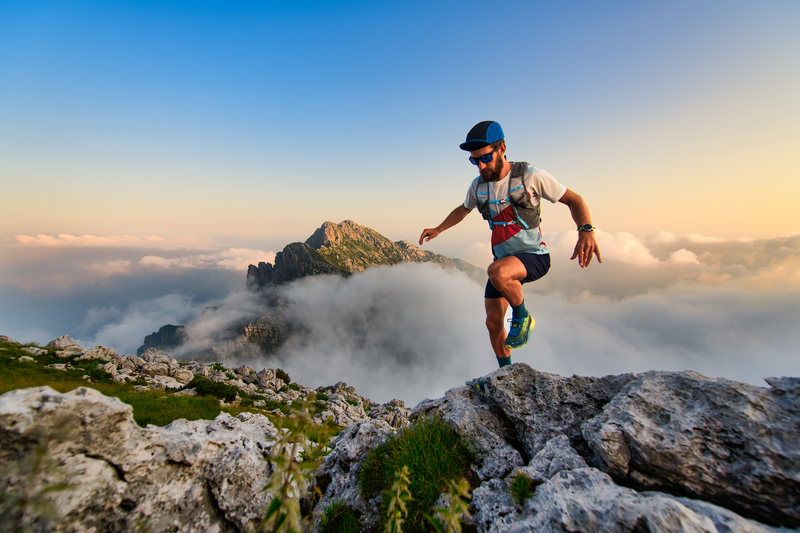
95% of researchers rate our articles as excellent or good
Learn more about the work of our research integrity team to safeguard the quality of each article we publish.
Find out more
ORIGINAL RESEARCH article
Front. Cardiovasc. Med. , 13 January 2025
Sec. Cardiovascular Surgery
Volume 11 - 2024 | https://doi.org/10.3389/fcvm.2024.1514751
Objective: We aimed to explore the application value of unsupervised machine learning in identifying acute gastrointestinal injury (AGI) after extracorporeal circulation for acute type A aortic dissection (ATAAD).
Methods: Patients who underwent extracorporeal circulation for ATAAD at the First Hospital of Lanzhou University from January 2016 to January 2021 were included. Unsupervised machine learning algorithm was used to stratify patients into different phenogroups according to the similarity of their clinical features and laboratory test results. The differences in the incidence of perioperative AGI and other adverse events among different phenogroups were compared. Logistic regression was used to analyze the high-risk factors for AGI in each phenogroups and random forest (RF) algorithms were used to construct diagnostic models for AGI in different phenogroups.
Results: A total of 188 patients were included, with 166 males and 22 females. Unsupervised Machine Learning stratified patients into three phenogroups (phenogroup A, B, and C). Compared with other phenogroups, phenogroup B patients were older (P < 0.01), had higher preoperative lactate and D-dimer levels, and had the highest incidence of AGI (52.5%, P < 0.001) and in-hospital mortality (18.6%, P = 0.002). The random forest model showed that the top four risk factors for AGI in phenogroup B were cardiopulmonary bypass time, operation time, aortic clamping time, and ventilator time, which were significantly different from other phenogroups. The areas under the curve (AUCs) for diagnosing postoperative AGI of phenogroup A, B, and C were 0.943 (0.854–0.992), 0.990 (0.966–1.000), and 0.964 (0.899–0.997) using the RF model, respectively.
Conclusion: Phenogroup stratification based on unsupervised learning can accurately identify high-risk populations for postoperative AGI in ATAAD, providing a new approach for implementing individualized preventive and therapeutic measures in clinical practice.
Acute type A aortic dissection (ATAAD) is a life-threatening condition characterized by a tear in the inner layer of the aortic wall, resulting in the separation of the aortic layers and the formation of a true and false lumen (1, 2). The incidence of ATAAD has been increasing annually, and it is associated with high morbidity and mortality rates (3). Surgical intervention is one of the crucial therapeutic modalities for patients with ATAAD (4, 5). However, acute gastrointestinal injury (AGI), a common postoperative complication, severely compromises the surgical outcome and represents a significant contributor to the high postoperative mortality rate in ATAAD patients (6, 7). AGI can manifest as various gastrointestinal complications, such as gastrointestinal bleeding, intestinal obstruction, and ischemic bowel disorders, further exacerbating the postoperative course (8). Low cardiac output, inflammatory responses, surgical trauma, and prolonged extracorporeal circulation are considered the primary risk factors for the development of AGI in ATAAD patients undergoing surgical repair (9). The occurrence of AGI is often insidious, and clinical manifestations lack specificity, frequently leading to delayed diagnosis and treatment, thereby exacerbating the condition (10). Therefore, early identification and intervention for high-risk populations of AGI are crucial for improving the surgical prognosis of ATAAD patients.
Personalized medicine aims to optimize treatment strategies for each individual patient to maximize therapeutic efficacy. Accurate patient stratification is a prerequisite for achieving personalized medicine. The postoperative AGI population in ATAAD patients exhibits high heterogeneity, and some standard treatment protocols have limited efficacy (11). Unsupervised machine learning techniques can perform patient clustering analysis based on multidimensional features (such as demographics, medical history, and laboratory indices), thereby identifying intrinsically similar patient Phenogroups. These approaches have been utilized for Phenogroup clustering in heart failure and sepsis patients to identify the effectiveness of different interventions (12, 13). By analyzing the associations between specific Phenogroups and outcomes or treatment responses, unsupervised machine learning can provide a basis for developing personalized treatment strategies.
Therefore, this study proposes to apply unsupervised machine learning methods to exploit the heterogeneous data of ATAAD patients and identify intrinsic similarities, thereby enabling the recognition of high-risk populations for postoperative AGI. This approach aims to provide guidance for early interventions in this patient population.
Patient studies were conducted according to the guiding principles of the Helsinki Declaration. This study was approved by the Ethics Committee of the First Hospital of Lanzhou University (LDYYLL-2021-422). Due to the retrospective nature of the study, written informed consent was abandoned. The framework of this study is shown in Figure 1.
Patients hospitalized for ATAAD from January 2016 to January 2021 were identified from the institutional database. Exclusion criteria: (1) Patients with severe hematological, respiratory, or other severe comorbidities; (2) Patients with preoperative acute or chronic gastrointestinal disorders (gastrointestinal hemorrhage, diarrhea, pancreatitis, cholecystitis, cholelithiasis, peptic ulcers, history of abdominal trauma surgery, and gastrointestinal malignancies); (3) Perioperative mortalities and mortalities within 3 days postoperatively; (4) Patients with missing data exceeding 15%.
AGI diagnostic criteria and severity assessment were based on the 2012 AGI guidelines from the European Society of Intensive Care Medicine (14). The severity is graded as follows: Grade 0: Absence of gastrointestinal symptoms. Grade 1: Self-limiting condition, but with a high risk of progression to gastrointestinal dysfunction or failure. Grade 2: Interventional management is necessitated to restore gastrointestinal function (gastrointestinal dysfunction). Grade 3: Persistent gastrointestinal failure despite interventional management (gastrointestinal failure). Grade 4: Acute, life-threatening gastrointestinal insult. Supplementary Table S1 list the evaluation criteria that AGI patients. The AGI grading system was utilized for daily assessment in accordance with standard care during the initial seven days of follow-up. The patients were divided into two groups by the maximum AGI grade: Grade 0 and Grade 1 as the non-AGI group, and Grade ≥2 as the AGI group.
Laboratory tests parameters were obtained by reviewing patients' medical records. Which included:
(1) Baseline patient characteristics: gender, age, body mass index (BMI), medical history, and aortic dissection (AD) risk score. (2) Preoperative and postoperative laboratory examinations, including white blood cell and neutrophil percentage (N%), as well as serum levels of amylase, total cholesterol, triglycerides, total bilirubin, direct and indirect bilirubin, alkaline phosphatase (ALP), alanine aminotransferase (ALT), aspartate aminotransferase (AST), and γ-glutamyl transferase (γ-GT). (3) Imaging parameters, including the results of computed tomography angiography (CTA) performed upon admission. (4) Records of the surgical procedure, including cardiopulmonary bypass (CPB) time, circulatory arrest time, and intraoperative red blood cell transfusion. Postoperative clinical outcome data: postoperative complications (renal dysfunction, liver dysfunction, and nosocomial infections), intensive care unit (ICU) length of stay, AGI, and in-hospital mortality.
The K-means clustering algorithm, an unsupervised machine learning technique, was applied to cluster ATAAD patients based on their laboratory test results and clinical baseline information. The K-means algorithm aims to partition n observations into k clusters, minimizing the sum of squared distances between data points and their assigned cluster centroids (15). The algorithm initializes by randomly selecting k cluster centers, assigns each data point to the nearest centroid, and iteratively recalculates the new centroids until convergence is achieved. To determine the optimal number of clusters k, the study evaluated the clustering quality with different k values ranging from 2 to 8 based on the silhouette coefficient. The silhouette coefficient measures the ratio of the similarity of a data point to its own cluster compared to its dissimilarity to other clusters, ranging from −1 to 1, with higher values indicating better clustering performance. The k value corresponding to the maximum silhouette coefficient and its associated clustering result were selected as the optimal clustering solution.
Based on the optimal clustering outcome, the study analyzed and compared the incidence of AGI and other postoperative complications among different ATAAD patient subgroups. Additionally, univariate logistic regression was employed to identify risk factors associated with postoperative AGI occurrence in each subgroup. The Random Forest algorithm was then utilized to rank the importance of these risk factors and elucidate the relative weights of predictor variables influencing the risk of AGI across different phenogroups.
Python (Version 3.9.0) was used for statistical analysis. Continuous variables were reported as the median and interquartile range (IQR) or mean and standard deviation (SD) and were compared using the Mann-Whitney test or Student's t test. Categorical data, presented as numbers and frequencies (%), were compared using the chi-square test or Fisher's exact test. A two-sided P value < 0.05 indicated that the corresponding difference was statistically significant.
The study included 188 ATAAD patients, with 166 males and 22 females. Postoperatively, 60 patients developed AGI, while 128 patients did not (no-AGI group). Figure 2 illustrates the optimal clustering solution and the silhouette coefficient values in the K-means clustering model. Based on the silhouette coefficient, the optimal number of clusters was determined to be 3, and these phenotypic subgroups were labeled as Phenogroup-A, B, and C. Table 1 presents the baseline characteristics of the three main phenogroups (A, B, and C), comprising 81, 59, and 48 patients, respectively. Compared to the other phenogroups, patients in Phenogroup-B were older and had a higher prevalence of pre-existing heart failure. Additionally, the Phenogroup-B exhibited the highest number of patients with impaired vascular perfusion on computed tomographic angiography (CTA). Laboratory tests revealed significantly elevated lactate and D-dimer levels in Phenogroup-B compared to Phenogroups-A and C.
Figure 2. The optimal silhouette coefficient values (A) and clustering solution (B) in the K-means clustering model. In the panel (B), phenogrophs are visualized using the T-distributed Random Neighborhood Embedding (t-SNE) technique, visualizing the differences between the three phenogroups.
The operative time was longest for Phenogroup-B, followed by Phenogroup-A, and shortest for Phenogroup-C (Table 2). However, the cardiopulmonary bypass (CPB) times were not significantly different among the three groups (P = 0.169). The aortic cross-clamp time was significantly longer in Phenogroup-B compared to the other two groups (P = 0.02). Patients in Phenogroup-B required the highest intraoperative red blood cell transfusion volumes, prolonged mechanical ventilation duration, and longer hospital stays when compared to Phenogroup-A and C. Figure 3 depicts the combined illustration of postoperative complications across the three phenogroups along with the mapping relationship between different phenogroups and the complications. The in-hospital mortality rate was highest in Phenogroup-B (18.6%), followed by Phenogroup-C (4.17%), and lowest in Phenogroup-A (2.47%), the difference was statistically significant (P = 0.02). Phenogroup-B had the highest proportion of patients requiring postoperative enteral nutrition (P < 0.01) and the highest incidence of infections (59.3%, P = 0.001). Additionally, this group was most prone to developing neurological complications. The occurrence of AGI was significantly different among the three groups, with 13, 31, and 16 cases in Phenogroup-A, B, and C, respectively (P < 0.001), indicating that Phenogroup-B represents a high-risk phenogroups for AGI.
Table 2. Subsequent intraoperative and postoperative outcome characteristics of the three phenogroups.
Figure 3. The postoperative complications in the three phenogroups (A) and mapping relationship between phenogroups and the complications (B).
Postoperatively, the profiles of inflammatory biomarkers exhibited distinct patterns across the three phenogroups, as shown in Figure 4. Platelet counts declined to varying degrees in Phenogroup-A, B, and C. All three groups demonstrated elevated C-reactive protein (CRP) levels, with the most pronounced upward trend observed in Phenogroup-B, indicating a more robust inflammatory response in this subgroup compared to Phenogroup-A and C. Lactate dehydrogenase (LDH) levels were significantly higher in Phenogroup-B compared to Phenogroup-A and C (P = 0.005), while creatine kinase (CK) levels were highest in Phenogroup-C. Overall, no significant differences were observed in neutrophil, lymphocyte, monocyte, neutrophil-to-lymphocyte ratio, or lymphocyte-to-monocyte ratio among the three phenogroups, as presented in Table 3.
Figure 4. The heat map shows the logarithm of the median of various blood markers with their hierarchical clustering relationship. Neu, neutrophils; Lym, lymphocytes; mono, monocytes; N/L, neutrophils/lymphocytes; L/M, lymphocytes/monocytes; Lc, lactic dehydrogenase; CK, creatine kinase.
Table 3. Subsequent intraoperative and postoperative outcome characteristics of the three phenogroups.
To evaluate the risk factors contributing to AGI development in the three phenogroups, univariate logistic regression were constructed (Supplementary Table S2). Additionally, the RF algorithm was employed to rank the importance of risk factors influencing AGI occurrence, as shown in Figure 5. For the Phenogroup-B, which exhibited the highest incidence rate of AGI, the top four risk factors identified by the RF analysis were: CPB time, aortic clamping time, operation time, and ventilator time, respectively. This risk factors were distinctly different from the other phenogroups. The ROC curves for diagnosing postoperative AGI of three phenogroups using the RF model are shown in the Supplementary Figure S1. The AUCs for phenogroup-A, B, and C were 0.943 (0.854–0.992), 0.990 (0.966–1.000), and 0.964 (0.899–0.997), respectively. Different risk factors for various phenogroups demonstrate promising diagnostic value for postoperative AGI.
Figure 5. The random forest algorithm was employed to rank the importance of risk factors influencing AGI occurrence. (A) phenogroup-A, (B) phenogroup-B, (C) phenogroup-C.
In this study, we applied an unsupervised machine learning algorithm for phenogroups stratification of ATAAD patients undergoing cardiopulmonary bypass, rather than relying solely on individual data points. Three phenogroups were identified, providing motivation for further evaluation of postoperative AGI in ATAAD patients. Patients of different phenogroups exhibited significant differences in the risk and influencing factors of AGI. Notably, phenogroup-B patients had the highest incidence of AGI at 52.5%, significantly higher than the other two phenogroups. This result attests to the accuracy and clinical value of phenogroups stratification in identifying high-risk populations and underscores the potential of machine learning techniques in improving patient stratification and outcomes. This novel approach offers significant advantages for clinical practice, enabling more accurate prediction and management of high-risk patients.
AGI is a severe complication after cardiac surgery, with an extremely high mortality rate (16, 17). The occurrence of AGI is influenced by various perioperative factors, primarily including cardiopulmonary bypass time and the extent of surgical trauma (6). If ischemic hypoperfusion in patients is not diagnosed and addressed promptly in the early stage, it can lead to reduced tissue and organ oxygenation and metabolism, subsequently triggering multiple organ dysfunction and increased mortality (18). Moreover, systemic inflammatory responses constitute a crucial pathophysiological component of postoperative AGI (19). In this study, involving 188 patients with ATAAD undergoing cardiopulmonary bypass, we applied an unsupervised machine learning algorithm to perform phenogroups stratification and evaluated the risk of AGI across different Phenogroups. Utilizing K-means clustering based on clinical and laboratory data, we categorized patients into three distinct phenotypic phenogroups. The results demonstrated significant differences in baseline characteristics among the three phenogroups. The phenogroup-A comprised patients of intermediate age and relatively stable preoperative conditions. Phenogroups-B exhibited the highest risk of AGI, including elderly patients with elevated preoperative lactate and D-dimer levels, 91.5% of patients in this group showed poor mesenteric perfusion. This group also displayed prolonged operative times, increased intraoperative red blood cell transfusion requirements, prolonged mechanical ventilation duration, and extended hospital stays. Computed tomography angiography (CTA) revealed a higher prevalence of preexisting heart failure and impaired vascular perfusion in this phenogroup. Phenogroups-C included younger patients with fewer comorbidities and better preoperative clinical profiles. These differences highlighted the importance of phenotypic heterogeneity in understanding patients' risk profiles. Notably, not only did phenogroups-B patients have the highest incidence of AGI (52.5%), significantly higher than the other two phenogroups, but they also exhibited the highest in-hospital mortality rate. This finding underscored the close association between AGI and poor postoperative outcomes, emphasizing the critical importance of preventing and treating AGI to improve prognosis (20). Such phenogroups stratification is crucial as it allows for personalized, tailored patient care approaches, reflecting the principles of precision medicine. Furthermore, we employed a random RF to analyze the high-risk factors and their weights for AGI in phenogroups-B patients. The top four influencing factors were identified as CPB time, aortic clamping time, operation time, and ventilator time. These factors, to some extent, reflected the magnitude of surgical trauma and the degree of ischemia-reperfusion injury, suggesting a close association between AGI occurrence and surgical trauma as well as ischemia-reperfusion injury (21).
The analysis of postoperative inflammatory biomarkers revealed distinct patterns among the phenogroups. Phenogroup-B showed the most robust inflammatory response, with significantly elevated CRP and LDH levels, indicating a higher degree of systemic inflammation. Previous studies have established that elevated CRP and LDH levels are associated with poor outcomes in gastrointestinal injuries, further corroborating our findings (22, 23). These biomarkers can thus serve as valuable indicators for early intervention and monitoring in high-risk patients. Additionally, the distinct inflammatory profiles suggest that different inflammatory pathways might be involved in AGI pathogenesis among the phenogroups, which could inform the development of targeted therapies.
Traditional risk assessment methods suffer from inherent subjectivity and limitations of single-dimensional analysis. This study pioneered the application of unsupervised machine learning for risk stratification of postoperative AGI in patients with ATAAD. By automatically discovering heterogeneous subgroups based on patients’ multidimensional clinical features, this innovative approach circumvents the need for manual threshold setting and avoids subjectivity. The results revealed significant differences in the incidence of AGI and other postoperative complications across different phenogroups, highlighting the advantages of machine learning in individualized risk assessment. This approach lays the foundation for tailored preventive and therapeutic measures, underscoring its significant clinical translational value. For future research, integrating intraoperative and postoperative dynamic data could further enhance the performance of phenogroup classification and risk prediction models through the development of multi-label classification models for comprehensive evaluation of various postoperative complications. Additionally, extending the application of these models to preoperative assessments could support decision-making for individualized surgical plans and perioperative management strategies, broadening the potential applications of machine learning in perioperative risk assessment (24).
Our study has some limitations. First, the retrospective single-center study design lacks prospective multi-center data, which may limit the extrapolation and generalizability of the results. Second, the sample size is relatively small (188 cases), and after stratification by phenogroups, the sample size of each phenogroups may be further reduced, affecting the statistical power of the analysis. Third, although the high-risk factors for different AGI phenogroups were analyzed, the potential interactions among these factors were not explored, which could aid in the refinement of risk stratification. Fourth, the absence of an external validation cohort may lead to overly optimistic results from the internal validation, and external data is needed to validate the model's generalizability. Finally, although our study provides valuable insights into patient stratification using unsupervised machine learning, the absence of more specific preoperative and intraoperative management strategies (e.g., preoperative restoration of mesenteric perfusion and cardiorespiratory variables) limits the potential for tailoring individualized treatments for high-risk patients identified in this study. Future prospective studies with more comprehensive data collection, including hemodynamic, renal, and perfusion parameters, are necessary to validate our findings and provide more targeted recommendations for clinical practice.
In conclusion, Phenogroups stratification based on unsupervised learning can accurately identify high-risk populations for postoperative AGI in ATAAD, providing a new approach and basis for implementing individualized preventive and therapeutic measures in clinical practice. This approach has certain innovative value and potential for clinical translation.
The raw data supporting the conclusions of this article will be made available by the authors, without undue reservation.
The studies involving humans were approved by the Ethics Committee of the First Hospital of Lanzhou University (LDYYLL-2021-422). The studies were conducted in accordance with the local legislation and institutional requirements. The ethics committee/institutional review board waived the requirement of written informed consent for participation from the participants or the participants' legal guardians/next of kin due to the retrospective nature of the study. Written informed consent was not obtained from the individual(s) for the publication of any potentially identifiable images or data included in this article due to the retrospective nature of the study.
YM: Conceptualization, Data curation, Funding acquisition, Methodology, Project administration, Resources, Writing – original draft, Writing – review & editing. XY: Conceptualization, Formal Analysis, Investigation, Methodology, Validation, Writing – original draft. CW: Conceptualization, Investigation, Methodology, Supervision, Validation, Writing – original draft. XW: Data curation, Investigation, Methodology, Project administration, Software, Writing – original draft. BZ: Conceptualization, Formal Analysis, Funding acquisition, Methodology, Writing – original draft. YiL: Conceptualization, Formal Analysis, Investigation, Project administration, Supervision, Writing – original draft. RW: Conceptualization, Formal Analysis, Investigation, Methodology, Validation, Writing – original draft. ZB: Conceptualization, Methodology, Project administration, Writing – original draft. PY: Conceptualization, Formal Analysis, Investigation, Methodology, Resources, Writing – original draft. HZ: Methodology, Project administration, Resources, Validation, Writing – original draft, Writing – review & editing, Conceptualization, Investigation. YaL: Conceptualization, Formal Analysis, Funding acquisition, Investigation, Methodology, Resources, Validation, Writing – original draft, Writing – review & editing.
The author(s) declare financial support was received for the research, authorship, and/or publication of this article. This study was supported by Foundation of The First Hospital of Lanzhou University (ldyyyn2023-95); High-end foreign project Plan of the Ministry of Science and Technology (G2023175006l); Gansu Province Joint Research Fund project (23JRRA1496).
We thank Professor Yaolong Chen, Dan Xu, Junxian Zhao, Tianhu Liang from the Research Center for Clinical Medical of the First Hospital of Lanzhou University for their valuable comments and suggestions, which provided important support for the design and implementation of this study.
The authors declare that the research was conducted in the absence of any commercial or financial relationships that could be construed as a potential conflict of interest.
The author(s) declare that no Generative AI was used in the creation of this manuscript.
All claims expressed in this article are solely those of the authors and do not necessarily represent those of their affiliated organizations, or those of the publisher, the editors and the reviewers. Any product that may be evaluated in this article, or claim that may be made by its manufacturer, is not guaranteed or endorsed by the publisher.
The Supplementary Material for this article can be found online at: https://www.frontiersin.org/articles/10.3389/fcvm.2024.1514751/full#supplementary-material
1. Rylski B, Schilling O, Czerny M. Acute aortic dissection: evidence, uncertainties, and future therapies. Eur Heart J. (2023) 44(10):813–21. doi: 10.1093/eurheartj/ehac757
2. Rady MY, Ryan T, Starr NJ. Perioperative determinants of morbidity and mortality in elderly patients undergoing cardiac surgery. Crit Care Med. (1998) 26(2):225–35. doi: 10.1097/00003246-199802000-00016
3. Pape LA, Awais M, Woznicki EM, Suzuki T, Trimarchi S, Evangelista A, et al. Presentation, diagnosis, and outcomes of acute aortic dissection: 17-year trends from the international registry of acute aortic dissection. J Am Coll Cardiol. (2015) 66(4):350–8. doi: 10.1016/j.jacc.2015.05.029
4. Elsayed RS, Cohen RG, Fleischman F, Bowdish ME. Acute type A aortic dissection. Cardiol Clin. (2017) 35(3):331–45. doi: 10.1016/j.ccl.2017.03.004
5. Malaisrie SC, Szeto WY, Halas M, Girardi LN, Coselli JS, Sundt TM 3rd, et al. 2021 the American Association for thoracic surgery expert consensus document: surgical treatment of acute type A aortic dissection. J Thorac Cardiovasc Surg. (2021) 162(3):735–58.e732. doi: 10.1016/j.jtcvs.2021.04.053
6. Ohri SK, Velissaris T. Gastrointestinal dysfunction following cardiac surgery. Perfusion. (2006) 21(4):215–23. doi: 10.1191/0267659106pf871oa
7. Hashemzadeh K, Hashemzadeh S. Predictors and outcome of gastrointestinal complications after cardiac surgery. Minerva Chir. (2012) 67(4):327–35.23022757
8. Dong G, Liu C, Xu B, Jing H, Li D, Wu H. Postoperative abdominal complications after cardiopulmonary bypass. J Cardiothorac Surg. (2012) 7:108. doi: 10.1186/1749-8090-7-108
9. Lu R, Yang B. Incidence and influencing factors of acute gastrointestinal injury after cardiac surgery. BMC Cardiovasc Disord. (2023) 23(1):437. doi: 10.1186/s12872-023-03475-6
10. Allen SJ. Gastrointestinal complications and cardiac surgery. J Extra Corpor Technol. (2014) 46(2):142–9. doi: 10.1051/ject/201446142
11. Naar L, Dorken Gallastegi A, Kongkaewpaisan N, Kokoroskos N, Tolis G, Melnitchouk S, et al. Risk factors for ischemic gastrointestinal complications in patients undergoing open cardiac surgical procedures: a single-center retrospective experience. J Card Surg. (2022) 37(4):808–17. doi: 10.1111/jocs.16294
12. Qin Y, Kernan KF, Fan Z, Park HJ, Kim S, Canna SW, et al. Machine learning derivation of four computable 24-h pediatric sepsis phenotypes to facilitate enrollment in early personalized anti-inflammatory clinical trials. Critical Care (London, England). (2022) 26(1):128. doi: 10.1186/s13054-022-03977-3
13. Cikes M, Sanchez-Martinez S, Claggett B, Duchateau N, Piella G, Butakoff C, et al. Machine learning-based phenogrouping in heart failure to identify responders to cardiac resynchronization therapy. Eur J Heart Fail. (2019) 21(1):74–85. doi: 10.1002/ejhf.1333
14. Reintam Blaser A, Malbrain ML, Starkopf J, Fruhwald S, Jakob SM, De Waele J, et al. Gastrointestinal function in intensive care patients: terminology, definitions and management. Recommendations of the ESICM working group on abdominal problems. Intensive Care Med. (2012) 38(3):384–94. doi: 10.1007/s00134-011-2459-y
15. Hashimoto DA, Witkowski E, Gao L, Meireles O, Rosman G. Artificial intelligence in anesthesiology: current techniques, clinical applications, and limitations. Anesthesiology. (2020) 132(2):379–94. doi: 10.1097/ALN.0000000000002960
16. Chaudhry R, Zaki J, Wegner R, Pednekar G, Tse A, Sheinbaum R, et al. Gastrointestinal complications after cardiac surgery: a nationwide population-based analysis of morbidity and mortality predictors. J Cardiothorac Vasc Anesth. (2017) 31(4):1268–74. doi: 10.1053/j.jvca.2017.04.013
17. Viana FF, Chen Y, Almeida AA, Baxter HD, Cochrane AD, Smith JA. Gastrointestinal complications after cardiac surgery: 10-year experience of a single Australian centre. ANZ J Surg. (2013) 83(9):651–6. doi: 10.1111/ans.12134
18. Elgharably H, Gamaleldin M, Ayyat KS, Zaki A, Hodges K, Kindzelski B, et al. Serious gastrointestinal complications after cardiac surgery and associated mortality. Ann Thorac Surg. (2021) 112(4):1266–74. doi: 10.1016/j.athoracsur.2020.09.034
19. Chor CYT, Mahmood S, Khan IH, Shirke M, Harky A. Gastrointestinal complications following cardiac surgery. Asian Cardiovasc Thorac Ann. (2020) 28(9):621–32. doi: 10.1177/0218492320949084
20. Vohra HA, Farid S, Bahrami T, Gaer JA. Predictors of survival after gastrointestinal complications in bypass grafting. Asian Cardiovasc Thorac Ann. (2011) 19(1):27–32. doi: 10.1177/0218492310394803
21. Shimizu K, Ogura H, Hamasaki T, Goto M, Tasaki O, Asahara T, et al. Altered gut flora are associated with septic complications and death in critically ill patients with systemic inflammatory response syndrome. Dig Dis Sci. (2011) 56(4):1171–7. doi: 10.1007/s10620-010-1418-8
22. Wang X, Deng C, Guo F, Cao X, Yan Y. Relationship between the postoperative lactate dynamic levels, the acute gastrointestinal injury and the prognosis among patients who undergo surgical treatment for acute type A aortic dissection. Heliyon. (2023) 9(6):e17128. doi: 10.1016/j.heliyon.2023.e17128
23. Zhang QC, Hastings C, Johnson K, Slaven E. Metformin-Associated lactic acidosis presenting like acute mesenteric ischemia. J Emerg Med. (2019) 57(5):720–2. doi: 10.1016/j.jemermed.2019.04.024
Keywords: acute type A aortic dissection, unsupervised machine learning, phenogroups, random forest, prediction
Citation: Ma Y, Yang X, Weng C, Wang X, Zhang B, Liu Y, Wang R, Bao Z, Yang P, Zhang H and Liu Y (2025) Unsupervised machine learning model for phenogroup-based stratification in acute type A aortic dissection to identify postoperative acute gastrointestinal injury. Front. Cardiovasc. Med. 11:1514751. doi: 10.3389/fcvm.2024.1514751
Received: 21 October 2024; Accepted: 30 December 2024;
Published: 13 January 2025.
Edited by:
Luca Paolo Weltert, Saint Camillus International University of Health and Medical Sciences, ItalyReviewed by:
Franco Turani, Aurelia Hospital, ItalyCopyright: © 2025 Ma, Yang, Weng, Wang, Zhang, Liu, Wang, Bao, Yang, Zhang and Liu. This is an open-access article distributed under the terms of the Creative Commons Attribution License (CC BY). The use, distribution or reproduction in other forums is permitted, provided the original author(s) and the copyright owner(s) are credited and that the original publication in this journal is cited, in accordance with accepted academic practice. No use, distribution or reproduction is permitted which does not comply with these terms.
*Correspondence: Yatao Liu, ZG9jdG9ybGl1eXRAMTYzLmNvbQ==; Hong Zhang, bGR5eV96aGFuZ2d5eUBsenUuZWR1LmNu
†These authors have contributed equally to this work
Disclaimer: All claims expressed in this article are solely those of the authors and do not necessarily represent those of their affiliated organizations, or those of the publisher, the editors and the reviewers. Any product that may be evaluated in this article or claim that may be made by its manufacturer is not guaranteed or endorsed by the publisher.
Research integrity at Frontiers
Learn more about the work of our research integrity team to safeguard the quality of each article we publish.